3
Designing and Implementing Control Strategies Through the SIP Process
OVERVIEW OF SIP PROCESS
The state implementation plan (SIP) and the tribal implementation plan (TIP)1 are the central organizing elements in the management of criteria air pollutants (see Box 3-1 for a discussion of tribes and the Clean Air Act). The SIP defines the combination of local, state, and federal actions and emission controls needed for an area to bring about compliance with the National Ambient Air Quality Standards (NAAQS) and for an area that has attained the NAAQS to maintain compliance and prevent significant deterioration of air quality. However, the purpose of the SIP extends well beyond the attainment and maintenance of NAAQS. It provides the basic link between state regulations, EPA oversight of state actions, and federal enforcement. In addition to addressing criteria pollutants, SIPs are used by EPA to formally establish state and local agency obligations to meet emission standards and goals related to regional haze, acid rain, and hazardous air pollutants (HAPs).
When EPA approves a plan, the rules specified therein are federally enforceable. When EPA disapproves a plan or finds that the state is delinquent in implementing it, EPA can impose sanctions, such as the loss of highway funds. In extreme cases, EPA can prepare a federal implementation plan (FIP), which takes the role of a SIP.
SIPs are submitted by individual states in accordance with explicit requirements set forth in the Clean Air Act (CAA). The requirements have been interpreted via regulations and guidance promulgated by EPA. The CAA requires each state to produce a single comprehensive SIP; however, in practice, each state produces a separate implementation plan for each criteria pollutant, because of the timing and pollutant-specific requirements set
BOX 3-1 There are more than 550 federally recognized tribes and more than 300 reservations in the United States. Indian tribes and individuals own approximately 55.4 million acres of land in the contiguous United States (Getches et al. 1998). The 1990 CAA Amendments authorized EPA to “treat tribes as states” for purposes of developing, administering, and enforcing air quality regulations within reservation boundaries, irrespective of land ownership (42 USC § 7601(d)(2)(B)). In doing so, Congress recognized the inherent sovereignty of tribes with respect to their land and members. Congress also delegated to the tribes regulatory authority over nonmembers operating on land within reservation boundaries. Pursuant to the CAA, in February 1998, EPA promulgated its tribal authority rule, specifying requirements for tribal eligibility to administer air programs (40 CFR 49). To be eligible, a tribe must apply to the EPA regional administrator and demonstrate that it is “reasonably” capable of administering its program in a manner consistent with the terms and purposes of the CAA. Tribes may develop a full tribal implementation plan (TIP) and seek authority to carry out all the functions that states perform under the act, but they are not required to do so. EPA’s regulations allow tribes to assume primacy over a subset of regulatory functions and to expand their authority gradually. EPA also has the flexibility to alter deadlines for plan submittal and other regulatory requirements. In 1999, the Gila River Indian Community became the first tribe to become eligible for “treatment as a state” status. To date, 14 tribes have received eligibility to implement parts of the CAA. The Mohegan tribe submitted a TIP to EPA in FY 2002, while the Pequot, St. Regis Mohawk, and Gila River have TIPs in progress (EPA 2003d). The tribal council of the Gila River Indian Community adopted ordinances in March 2002 comprising the first section of its TIP. On the basis of a telephone survey of 156 of the 237 federally recognized tribes in the Western Regional Air Partnership (WRAP) region, 60 tribes in this region have some form of air quality program. The scope of activities ranges from education and outreach to monitoring, emissions inventory development, and source permitting (ITEP 2001). Twenty-eight of the surveyed tribes had an emissions inventory and 51 tribes performed some air quality or meteorological monitoring. The survey suggested that if resources were available, the level of activity could double in the next few years. For example, 62 tribes indicated an interest in starting an air monitoring program in the next few years (ITEP 2001). Tribes, like states, are eligible under the CAA for federal grants to support air quality monitoring and management efforts. EPA began seeking tribal participa- |
tion in its grants program in 1995, providing grants to about 20 tribes. In 2002, 121 tribes received air program grants (C. Darrel Harmon, EPA, personal commun., Feb. 19, 2003). The tribal share of EPA-administered state and tribal assistance grants (STAG) for air has been flat at about $11 million since 1999, while tribal interest in developing air programs has increased. Tribes seeking first-time grants are being turned away in Regions 9 and 10.a Additional support for tribal environmental programs is available through EPA’s multimedia Indian environmental general assistance program (GAP) for which $63 million was included in the agency’s 2004 budget request. However, GAP funding is used primarily for environmental infrastructure development, capacity building, education, and outreach and cannot be used to operate environmental management programs. In addition to direct grants to tribes, STAG funding supports the Institute for Tribal Environmental Professionals (ITEP) at Northern Arizona University. This organization offers about 20 technical workshops each year for tribal environmental staff on monitoring and permitting, and other air quality management (AQM) topics and also serves as a forum for interaction and information exchange between tribes and with EPA. In partnership with ITEP, EPA also supports the Tribal Air Monitoring Support Center at the EPA Radiation and Indoor Environments Laboratory to provide air monitoring training and technical support to the tribes. Until a tribe assumes control of its own air pollution programs, the responsibility and authority to regulate air pollution sources on tribal lands falls to EPA. States have no environmental regulatory jurisdiction on tribal lands. EPA administers a Title V operating permits program for major stationary sources on tribal lands along with a prevention of significant deterioration (PSD) preconstruction permit program for new major sources or source modifications in attainment areas. A new-source review (NSR) program under which individual permits can be issued for major sources in tribal lands within nonattainment areas and for minor sources in attainment areas does not exist. EPA is required to develop case-by-case federal implementation plans (FIPs) where these plans must go through the full and lengthy Federal Register rule-making process for each applicable facility. In addition, sources in tribal areas may be competitively disadvantaged by the lack of synthetic minor permitting programs that allow sources to be classified on the basis of actual rather than potential emissions.b The National Tribal Environmental Council (NTEC) estimates that 80 Indian reservations are located within or partly within nonattainment areas for the 1-hr average ozone (O3) standard and that the number is likely to be higher with the 8-hr standard. Some tribes have expressed concern about pending designations of nonattainment areas for O3, because EPA guidance presumes that designations will be made based on metropolitan statistical area (MSA) or consolidated metropolitan statistical area (CMSA) boundaries (NTEC 2002). These default designations ignore the jurisdictional boundaries between tribes and states. EPA will consider recommendations from tribes that their lands be excluded from nonattainment areas, but the exemptions require detailed analysis that may tax tribal resources. In particular, air quality data for tribal lands are often lacking. Tribes are concerned that they will face the burdens of nonattainment designation, including offset requirements, even though they have historically borne little responsibility for air quality problems (or derived little economic benefit from the air pollu- |
tion sources) in the adjacent metropolitan areas. Similar issues are likely to arise with respect to designations for particulate matter of 2.5 micrometers (PM2.5). Since EPA promulgated the tribal authority rule in 1998, tribes have demonstrated increasing interest in developing and administering their own air programs. Federal policy supports tribes’ control over their own air resources and economic development. Tribal participation in regional organizations, such as the Western Regional Air Partnership, is vital for filling gaps in air quality and emissions data and for effective air quality management. However, lack of resources imposes severe constraints on air quality management activities for many tribes. As indicated in Chapter 7, more federal funding for tribal assistance grants is needed to support tribal self-determination and tribal participation in the national AQM system. |
by EPA. Most SIPs are also continuously evolving to reflect new federal or state requirements, new information, or change in status of NAAQS attainment.2 Thus, at any one time, a state may have a number of new and revised SIPs at various stages of development and federal review.
The requirements for any state’s SIP depend on its air quality, which is determined by the attainment designations assigned to the individual areas3 within that state. Section 107 of the CAA defines three designations for any area’s compliance with NAAQS for criteria pollutants. They are as follows:
-
Nonattainment. Any area that does not meet (or that contributes to ambient air quality in a nearby area that does not meet) a national primary or secondary ambient air quality standard for the pollutant.
-
Attainment. Any area that meets the national primary and secondary ambient air quality standard for the pollutant and does not contribute to the violation of a national primary or secondary ambient air quality standard in a nearby area.
-
Unclassifiable. Any area that cannot be classified on the basis of available information as meeting or not meeting a national primary or secondary ambient air quality standard for the pollutant.
The CAA further classifies O3 nonattainment areas as marginal, moderate, serious, severe, and extreme, and CO nonattainment areas as moderate or serious (see Table 3-1). The procedures used to determine the appropriate designation and classification for an area are described in Box 3-2.
The basic requirements for states in general and for those areas that are in nonattainment of one or more NAAQS are listed in Box 3-3. The requirements for a SIP grow in stringency and complexity as an area’s designation shifts from attainment to nonattainment and, for ozone (O3) and carbon monoxide (CO), to the more acute nonattainment classifications. Because the major efforts in air quality management (AQM) for criteria
TABLE 3-1 Classification of Nonattainment Areas for O3 and CO Mandated in the CAA Amendments of 1990
Area Classification |
Design Value, ppm |
Attainment Date for Primary Standarda |
|
O3 Nonattainment Areas |
|
Marginal |
0.121–0.138b |
Nov. 15, 1993 |
Moderate |
0.138–0.160b |
Nov. 15, 1996 |
Serious |
0.160–0.180b |
Nov. 15, 1999 |
Severe-15c |
0.180–0.190b |
Nov. 15, 2005 |
Severe-17c |
0.190–0.280b |
Nov. 15, 2007 |
Extreme |
0.280 and aboveb |
Nov. 15, 2010 |
|
CO Nonattainment Areas |
|
Moderate (low)d |
9.1–12.7e |
Dec. 31, 1995 |
Moderate (high)d |
12.8–16.4e |
Dec. 31, 1995 |
Serious |
16.5 and abovee |
Dec. 31, 2000 |
aThe primary standard attainment date for O3 is determined from the date of the enactment of the CAA Amendments of 1990. bThe classification scheme for O3 was devised by Congress before EPA promulgated a new 8-hr standard, and, thus, the classification relates to the old 1-hr form of the standard. cThe requirements for severe-15 and severe-17 O3 nonattainment areas are the same except for the attainment dates. dModerate CO nonattainment areas with design values of 12.7 ppm or less have reduced SIP requirements compared with those areas with design values above 12.7 ppm. eThese values for CO refer to a rolling 8-hr average. Abbreviation: ppm, parts per million. |
BOX 3-2 Designation of an area as an attainment, nonattainment, or unclassifiable area is made by the EPA administrator in consultation with the relevant state governors and is based on data gathered from the NAMS/SLAMS air quality monitoring networks (which are discussed in Chapter 6). The designation process engenders two fundamental assumptions: (1) that attainment and maintenance of a NAAQS can be established using data from a limited number of surface air quality monitoring sites, and (2) that geographical areas can be effectively regulated with a single, uniform designation and a common plan for achieving and/or maintaining an acceptable level of air quality. An area’s attainment or nonattainment status with regard to a criteria pollutant is determined by comparing the NAAQS with the area’s “design value”a for the pollutant. The design value is derived from air quality monitoring data gathered by local or state authorities following guidelines specified by EPA. If the design value exceeds the NAAQS, the area is designated as being in nonattainment. For CO and O3, an additional, more detailed classification scheme is used to indicate the severity of the nonattainment (see Table 3-1). Because the statistical form of the standard varies from one pollutant to another, the method used to determine the design value also varies by pollutant. For example, in the case of the old 1-hr O3 standard, the design value is calculated as the fourth highest 1-hr averaged concentration observed at any monitoring site in the area over three consecutive years. The new 8-hr O3 design value, on the other hand, is defined as the 3-year average of the annual fourth highest 8-hr averaged concentration observed each year. The new 24-hr averaged PM2.5 design value is derived from the 3-year average of the 98th percentile measured concentrations in an area. The annual PM2.5 design value is obtained from an average of all measurements made in the area. These various protocols involving multiyear and spatial averages and selecting concentrations below the absolute maximum observed have been chosen to limit the impact of statistical outliers and extreme and anomalous meteorological events. Nevertheless, as discussed elsewhere, the use of design values in the attainment demonstration of the SIP presents important challenges. In addition to providing a mechanism for identifying and designating nonattainment areas, the CAA (in Section 175(a)) also provides a process by which a nonattainment area can be redesignated an attainment area. To be redesignated, an area must file a “maintenance SIP.” In addition to showing that the area is in compliance with the NAAQS on the basis of relevant air quality monitoring data, the maintenance SIP must provide a plan for ensuring that the standard will not be violated in the future. Once such a plan is approved by EPA, the area is generally referred to as a maintenance area. EPA currently lists 62, 59, 30, and 16 maintenance areas for O3, CO, SO2, and PM10 respectively. There are a number of other nonattainment areas that have demonstrated achievement of the standards, but either because they have not completed the process of filing a maintenance SIP or because EPA has not yet accepted it, they have not been redesignated. |
BOX 3-3 A. For all statesa SIPs must be submitted within 3 years of promulgation of new NAAQS and provide for “implementation, maintenance, and enforcement” of the standard. Among other things each SIP must
B. For nonattainment areasb Attainment-demonstration SIPs must be submitted within 3 years of an area being designated a nonattainment area. In addition to the items listed in part A, the SIP must
|
For a nonattainment area to be redesignated an attainment area, a revised SIP must be submitted and approved. This planc must
C. For O3 nonattainment areasd In addition to the items listed in parts A and B, SIPs for marginal and above O3 nonattainment areas must
In addition, SIPs for moderate and above O3 nonattainment areas must
In addition, SIPs for serious and above O3 nonattainment areas must
In addition, SIPs for severe O3 areas must
|
In addition, SIPs for extreme O3 areas must
|
pollutants are currently focused on nonattainment areas, our discussion here will be largely limited to the “attainment-demonstration SIP” submitted by an area after it has been designated a nonattainment area. As indicated in Box 3-3, the CAA requires that an attainment-demonstration SIP be submitted to EPA within 3 years of an area’s being designated a nonattainment area. The agency or agencies responsible for preparing an attainment-demonstration SIP vary from state to state. Often, it is prepared by the relevant state or tribal authority; in other cases, a local or regional government is given primary responsibility.
THE MAIN COMPONENTS OF AN ATTAINMENT-DEMONSTRATION SIP
The key elements of an attainment-demonstration SIP are the following:
-
An emissions inventory.
-
An analysis involving air quality model simulations as well as observational data and related evidence to determine the amount and types of emission reductions needed to bring about compliance by the appropriate date.
-
A description of the emission-control strategies and enforcement measures to be adopted to achieve the required reductions.
Each of those components is described below.
Emission Inventories
The first step in developing an emission-control strategy for a criteria pollutant is to develop an inventory of pollutant emissions that lists all sources of the pollutant or its precursor and the rate at which each source emits the pollutant to the atmosphere. EPA has specified a general procedure for emissions-inventory development that categorizes emissions into four source types: stationary, mobile, biogenic, and geogenic (EPA 2003f). Stationary sources are further divided into major stationary and area sources. Major stationary sources are defined as stationary sources having emissions that exceed a minimum or threshold level, which varies depending on the pollutant. Stationary sources that fall below the threshold level are merged as area sources. The reporting requirements for major stationary sources are more detailed than those for area sources (65 Fed. Reg. 33268 [2000]). Mobile sources include on-road and nonroad vehicles and sources such as lawn and garden, recreational, construction, and marine equipment; aircraft; and locomotives. Biogenic sources include plants, trees, grasses, and agricultural animals and crops, and geogenic sources include gas seeps, soil wind erosion, geysers, and volcanoes. Inventories are generally developed using a combination of the direct measurements and emission models described below (EPA 1998b).
Continuous Emissions Monitoring Systems
The most direct way to determine the rate at which a pollutant is emitted into the atmosphere is through emissions monitoring. However, the large number and varying types of sources that generally exist for a given pollutant make this impractical and only the largest major stationary sources are generally equipped with continuous emissions monitoring (CEM) systems.
As a result of the requirements in the Acid Rain Title of the 1990 CAA Amendments, increasing numbers of electrical utility boilers are now using CEM systems. The data from them are posted quarterly on the internet, providing hourly emissions as well as values averaged quarterly and annually (EPA 2003g). Because of the use of CEM systems, the emission rates from these sources are generally viewed as being among the most accurate of all known rates in the United States.
Emission Estimation Models
Models for estimating emissions have been developed and made available by EPA for selected sources, particularly area sources for which mea-
surements are difficult to make. Emission models are used for estimating on-road emissions and air emissions from landfills, storage tanks, wastewater-collection and -treatment systems, wind erosion, fugitive dust from roads, material handling, agricultural tilling, and construction and demolition. These models generally estimate Ei(s), the emission rate (in, say, tons per day) of pollutant i from source s, as

(3-1)
where EFi(s) is the emission factor (in units of tons of emissions per unit of activity) and A(s) is the activity level for that specific emission. Depending on the source, various types of activity levels can be chosen—for example, the total amount of fuel used by the source, the amount of product produced or consumed, the population density, or the vehicle miles traveled. In some cases, an emission factor is derived for an uncontrolled source. In this case, the emission rate for a source with controls is corrected to allow for the fractional control of the emissions. The control efficiency (CE) may be further modified by correction factors to take into account the rule effectiveness (RE) and the rule penetration (RP) (EPA 2001b). In this case, Equation 3-1 is modified as follows:

(3-2)
where CE is the fraction of a source category’s emissions that are controlled (for example, controlled by a control device or process change). RE is an adjustment to the CE to account for failures or uncertainties in the performance of the control. RP is the fraction of the source category that is covered by the regulation or is expected to comply.
EPA compiles and periodically updates emission factors for a large number of sources (EPA 2002g). Emission factors are simply averages of all available data of acceptable quality and are generally assumed to be representative of long-term averages for all facilities in the source category (that is, a population average) (EPA 1995). For example, for mobile sources, emission factors are derived from measurements made on a selected set of vehicle types and ages deemed to be representative of the fleet of vehicles in use. In other cases, emission factors may be based on the properties of the fuel used. For example, sulfur emissions from vehicles are often estimated from fuel consumption and fuel sulfur concentration, which is determined by measurements made on a sampling of the fuel in use at the time.
An activity level can be estimated from a wide range of data and measurements. For area sources involving consumer products, it can simply be the population density or other relevant socioeconomic indicator (for example, the amount of a given item sold or consumed). Activity levels for
mobile sources are often derived from estimates of vehicle miles traveled and fuel consumption statistics. Stationary-source activity levels can be based on economic indicators, fuel-usage statistics, and surveys and questionnaires.
Critical Review of Emission Inventories
The emission inventories used in AQM and the methods used to develop them have been reviewed and critiqued extensively by previous investigators and committees (see NRC 1991, 1998b; Placet et al. 2000). Current emission inventories are generally held to have an uncertainty of about a factor of two or more (see NARSTO 2000), although, as discussed later, the uncertainty factor is poorly defined. The consequences of errors in emission inventories can be profound. For example, during the 1970s and 1980s, emission inventories for O3 precursors, volatile organic compounds (VOCs), and nitrogen oxides (NOx) had significant errors, the emissions of VOCs being greatly underestimated and the emissions of NOx from power plants being somewhat overestimated. Collectively, these errors probably contributed to the adoption of less-than-optimal control strategies for ground-level O3 pollution in many regions of the United States (NRC 1991; NARSTO 2000).
A major contributor to the large uncertainties in current emission inventories arises from the use of emission models to derive the inventories (that is, estimating the emissions from a source as the product of an emissions factor and an activity level). The application of an average emissions factor based on the measurements of a small subset of a total population necessarily introduces uncertainty. Such application is especially problematic if the total emissions of a particular type are dominated by statistically anomalous members of the emitting population. For example, in the case of mobile emissions, a disproportionate amount of the emissions arises from a relatively small percentage of high-emitting motor vehicles (NRC 2001c and references therein; Holmes and Cicerone 2002 and references therein). Because it is not well-understood why some of these vehicles are high emitters and others are not, it is extremely difficult to identify a representative sample of motor vehicles that will provide a statistically valid representation of the total population. A related problem arises from the difficulty in accounting for large emission spikes from stationary sources related to so-called upsets, which are not captured in the average emission estimate for each source and which may result in increased overall emissions (TNRCC 2003). Additional uncertainty can arise when the design and operation of the sources that are measured to determine the emission factors are not representative of the design and operation of the entire population of sources. Beyond the uncertainties in estimating emission factors, the
estimation of the activity levels for each source adds more uncertainty, some measures (such as miles traveled or numbers of vehicles in use) being relatively easy to estimate and others (such as industrial emissions tied to economic activity and fugitive dust tied to construction activity) being substantially more difficult to estimate. Further, other sources, such as wildfires, can contribute substantial quantities of air pollutants, but emission estimates are highly uncertain.
Another source of uncertainty in emission inventories arises from the “bottom-up” nature of the process; for example, an inventory is constructed by adding up the emissions from the known sources. With this process, there is no internal mechanism to ensure that important sources have not been overlooked. The importance of overlooked sources was highlighted recently by the measurement of emissions of particles and organics, including chlorinated dioxins and furans, from the burning of household waste in barrels. The research showed that this uncontrolled source of emissions could yield emissions comparable to those of a controlled combustor for a community of 30,000 people (EPA 1998c).
There are at least two steps that could be taken to address the difficulties discussed above and thereby improve the accuracy and utility of emission inventories in AQM. The first step involves the enhanced use of ambient air measurements in conjunction with diagnostic tests, such as source apportionment, to evaluate emission inventories independently (see Figure 3-1). It is often found that the process of reconciling emission estimates from source-oriented models with those from receptor-oriented models helps to identify problematic emissions and activity factors as well as important sources of emissions that had been heretofore neglected (Watson et al. 2002). The second step is the development and routine use of methods to estimate the uncertainty and determine the quality of the inventory data (see Frey et al. 1999). Currently, EPA grades inventory data from A to E based on the quality of the data, but no quantitative measure of uncertainty is maintained. As discussed later, a quantitative estimate of the uncertainty in an emissions inventory could then be used in a propagation of error analysis to advise policy-makers on the range of possible outcomes that could arise from any given emission-control strategy.
Although EPA is responsible for defining how to develop emission inventories, state and local agencies have most of the responsibility for gathering the data. To overcome some of the difficulties that this separation of responsibility generates, the Emission Inventory Improvement Program (EIIP)—a jointly sponsored effort of the State and Territorial Air Pollution Program Administrators and the Association of Local Air Pollution Control Officials (STAPPA-ALAPCO) and EPA—was established in the early 1990s. The goal of EIIP is to provide cost-effective, reliable inventories by improving the quality of emission information and develop-
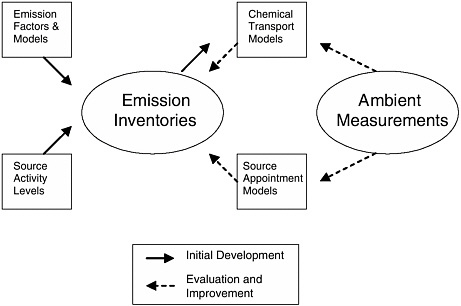
FIGURE 3-1 Emission-inventory development, evaluation, and improvement. Ambient measurements can be used to evaluate and improve emission inventories initially developed using emission factors and activity levels.
ing systems for collecting, calculating, and reporting emission data. EIIP is directed to set up protocols for data collection, transfer, and documentation to overcome some of the problems with data quality. EPA has indicated that documents prepared by EIIP should be used instead of existing federal guidance when appropriate.
Mobile-Source Emission Inventories
The emission inventories for mobile sources are particularly noteworthy. Mobile emissions represent a major source of many pollutants in the United States.4 Moreover, because of the large number of types, ages, and operating modes of mobile sources, their inventories are especially difficult to develop. As a result, uncertainties in mobile-source emissions can result in significant uncertainty in the overall emissions inventory for an area.
Mobile-source types range from lawn and garden equipment to motor vehicles, aircraft, and locomotives. EPA has developed two main computer tools for determining the emissions from mobile sources. The MOBILE
4 |
A detailed discussion of the mobile-source emissions control program in the United States is presented in Chapter 4. Here, the discussion is limited to the emission inventories used to characterize their emissions. |
computer program, in various versions, has been used to compute the emissions from on-road vehicles (cars, trucks, buses, and motorcycles) for over 30 years. The NONROAD program is used for the computation of emissions from other mobile sources.
As in all emission inventories, the accuracy of the estimated on-road and off-road emissions depends on two components: the emission factors and the activity levels. The MOBILE model provides emission factors for on-road vehicles. Local areas have to develop activity levels, such as vehicle miles traveled, to get a final emissions inventory in tons per day. In contrast, the NONROAD model contains both emission factors and activity levels. However, little is known about the accuracy or uncertainty of either component (Bammi and Frey 2001).
The National Research Council (NRC) has published a report on the modeling of mobile-source emissions, the major focus being the MOBILE model (NRC 2000b). A number of problems with MOBILE were identified, including emission factors based on laboratory testing and not actual driving conditions. A number of recommendations for improving that model were made. The NRC report also noted that nonroad (or off-road) sources were becoming an increasingly important part of the inventory (see discussion in Chapter 4) and that, because of a lack of data, the NONROAD model does not accurately estimate the emission inventories or the effect of controls on off-road sources. The report recommended that more attention be given to the NONROAD model.
At the time that the NRC report was issued, EPA was in the process of producing a new version of the MOBILE model, referred to as MOBILE6, which appears to address several of the concerns with previous versions of the model (NRC 2000b). However, a significant shortcoming remaining with MOBILE6 stems from its inadequate treatment of the effects of emission spikes that come from variability in engine loads and the importance that such spikes have in overall emission inventories (Barth et al. 1997; NRC 2000b; Hallmark et al. 2001). With the ultimate goal being to translate vehicle performance into their effects on air quality, a much tighter coupling between vehicle operating characteristics and emissions is being pursued through EPA’s next-generation MOVES model (EPA 2002h). A tighter coupling of emissions to vehicle operations will aid not only in the simulation of regional emissions and air quality but also in the assessment of improvements that are more microscale in nature, such as the effects on emissions from changes in traffic-signal operations and traffic flow.
Another concern raised in a separate General Accounting Office report (GAO 1997) was how inspection and maintenance (I/M) programs are modeled in MOBILE. I/M modeling is an important element because local areas use this part of the model to derive mobile emission reductions arising from the implementation of I/M programs in their local areas. Another
NRC report on I/M programs concluded that such programs provide “much lower benefits” than those estimated by the MOBILE model (NRC 2001c).
Air Quality Modeling
The second step in the development of an attainment-demonstration SIP is to determine the amount of pollutant-emission reductions that will be needed to bring about compliance with the NAAQS. Air quality models generally play the central role in that process. Typically a model is used to simulate one or more of the historical events that contributed to the area’s “design value” (defined in Box 3-2) and, through these simulations, demonstrate that appropriate emission controls will prevent NAAQS violations in the future during similar events.5 Because the model simulations are required to show compliance in the future, they must be run with future projections for a variety of hard-to-predict quantities that affect emissions (for example, socioeconomically driven activity factors, such as vehicle miles traveled and the effectiveness of emission-control technology). The projections and the attainment demonstration based on simulations of the extreme events that contributed to the design value introduce uncertainty into the process beyond that arising from intrinsic uncertainties in the emission inventories and models.
The air quality models used in these analyses are designed to allow policy-makers to link quantitatively pollutant emissions to concentrations of pollutants in the atmosphere. Models of several types have been in development and used for over 30 years and, through a close collaboration between the scientific, engineering, and regulatory communities, have continuously evolved in their capabilities and technical completeness (Russell and Dennis 2000). There are three major classes of air quality models: (1) statistical and empirical models that are based on observed relationships between pollutant concentrations and emission rates with little or no explicit consideration of the underlying physical and chemical processes that determine these relationships; (2) deterministic models that solve mathematical equations that describe the physics and chemistry of air pollutant emissions, formation, transport, and removal; and (3) a hybrid of the former two that, although essentially empirical or statistical in its approach, makes use of physically and chemically based algorithms. An example of the latter
would be a grid-based model that uses inverse methods to derive pollutant emission rates from observed pollutant concentrations. This section briefly reviews and provides a critique of some of the key models commonly used in AQM.
Empirical Rollback Model
The empirical rollback model is the simplest model available for AQM. This model assumes that an air pollutant’s concentration in an airshed is directly proportional to the total emission rate of the pollutant in the airshed. In other words, if Po is the current concentration of a pollutant in an airshed, Eo is the current rate of emission, and El is the hypothetical emission rate after the introduction of emission controls, the new pollutant concentration, Pl, predicted by a rollback model would be

(3-3)
where Pbackground is the so-called background concentration of the pollutant that would be found in the absence of any emissions within the airshed. From Equation 3-3, one can solve for Ea, the emission rate needed for attainment of Ps, the pollutant air quality standard or goal:

(3-4)
Although easy to use, rollback models have distinct limitations. Because of the assumption of linearity between emissions and concentrations, these models are only suitable for primary pollutants or secondary pollutants with relatively simple chemical-production mechanisms. Because rollback models only calculate a single concentration for the pollutant in the airshed, they are unable to simulate or account for spatial and temporal variations in pollutant concentrations. As a result, these models are most useful for pollutants that tend to be uniformly mixed in an airshed. They were used extensively in AQM before the mid-1970s and have since been largely supplanted by more sophisticated models. They nevertheless continue to be used in some applications, most notably in the design of urban strategies to meet the NAAQS for carbon monoxide (CO) (NRC 2002b).
Receptor Models
Receptor models are similar to rollback models in their dependence on observed concentrations and their neglect of chemical and meteorological processes. However, instead of being limited to assuming simple linear relationships between a pollutant concentration and its emission rate, re-
ceptor models can adopt more sophisticated statistical techniques to derive a more complex (and possibly more accurate) description of the relationship. They have been used most successfully and most widely in estimating the relative contributions of various emission sources to measured ambient air particulate matter (PM) composition at a monitoring site. The estimates are made by relating the measured elemental and organic tracer composition of ambient PM to the known elemental composition of the sources of PM in the region. Reviews of these methods can be found in Brook et al. (2003) and the references therein.
Although receptor models do not require emission inventories, some of them (such as chemical mass balance models) require source profiles, which define the detailed elemental or organic composition of all sources. In contrast, statistical factor analysis models do not require source composition data but use variations and covariations among species over time to identify sources. If the source profiles vary among sources in the same category, space, or time, a large measurement project is required to obtain profiles that adequately characterize all the source classifications that are to be used in the model. These models have a long history of applications and have in general provided valuable information for the design of primary PM-control strategies. Recent extensions of these models allow the identification of sources of primary organic PM as well as sources of inorganic particles. Their greatest weakness is the estimation of the contribution of secondary PM. To date, a combination of receptor models has been the most successful approach for PM nonattainment areas.
Emissions-Based Air Quality Models
Emissions-based models for O3 and CO are important tools used today in AQM to evaluate alternative emission-control strategies and estimate the amount of emission reductions required to meet a specific air quality goal or standard. The models use a mathematical representation of the relevant physical and chemical processes and then solve the governing equations (usually numerically) in time and space to determine the relationships between pollutant emissions and pollutant concentrations. Because these models require the input of pollutant emission rates, they are sometimes referred to as emissions-based models. One of the major advantages of these models for AQM is their predictive capability. Because they calculate pollutant concentrations as a function of pollutant emissions, they can be run in a prognostic mode to predict the air quality response to any hypothetical change in pollutant emissions.
Emissions-based air quality models can vary in complexity. The simplest are box models, which simulate the evolution of pollutant concentrations in an idealized well-mixed parcel of air. Dispersion models, such as
ISC3 and CalPUFF (EPA 1995a,b), simulate a plume parcel of air as it advects and mixes with ambient air. The most complex are state-of-the-science three-dimensional (3D) chemical transport models (CTMs), which attempt to recreate the observable distributions of chemical species in time and space. They are called 3D models (also, grid models) because they use a three-dimensional grid to represent space. In the chemical transport model, gases and particles are transported horizontally and vertically by winds and turbulence between adjacent grid cells of the model, and chemical reactions between gases and particles occur at rates governed by reactant concentrations in individual grid cells. These models generally require a massive amount of input data to operate (Russell and Dennis 2000). In addition to the emissions of all pollutants in the model domain, these models require the input of temporally (usually hourly) and spatially resolved information on the relevant meteorological conditions (wind speed and direction, temperature, relative humidity, mixing depth, and solar insolation) (Pielke and Uliasz 1998). They also require the initial concentration fields of all pollutants in the entire domain, plus temporally resolved concentrations at the domain boundaries. Collecting and processing all this input information usually requires many measurements and additional models (meteorological, emissions, and coarser resolution models for the boundary conditions) and is usually the most time-consuming and resource-intensive part of the modeling exercise. A relatively new but important component of some 3D models is the inclusion of algorithms to internally assess the statistical uncertainties in the model output.
The First-Generation 3D CTMs—Urban-Scale Photochemical Grid Models for O3
Ground-level O3 is produced by the oxidation of VOCs in the presence of sunlight and NOx. Control of ground-level O3 pollution in an urban area can be achieved in principle by reducing the emissions of VOCs or NOx locally or in areas upwind of emission sources. However, the formation and transport of O3 in the atmosphere is a complex chemical and physical process, and determining the most effective combination of VOC- and NOx-emission controls to mitigate O3 pollution has proved to be a challenging task (see Box 3-4). It is best accomplished, however, using so-called photochemical models capable of simulating the chemical reactions that lead to the production of O3 in the presence of sunlight.
Since the 1970s, when the first NAAQS for O3 was promulgated, photochemical models have evolved considerably in their scientific complexity and completeness as well as their numerical sophistication. Simple rollback and box models were used initially to develop O3 mitigation strategies; by 1973, EPA had committed its research efforts to supporting the
BOX 3-4 Since the first O3 NAAQS were set in response to the 1970 CAA Amendments, significant progress in O3 reduction has been made in some areas of the United States. These areas include Los Angeles, New York, and Chicago, where the average daily maximum 1-hr O3 concentration decreased by about 15% over the decade 1986–1996. Despite almost three decades of massive and costly efforts to bring the O3 pollution under control, however, the lack of O3 abatement progress in a number of areas of the country has been discouraging and perplexing (NRC 1991). The complex O3-formation process, which involves the interaction of NOx, VOCs, and dynamic atmospheric processes, has probably contributed to the difficulties encountered in abating O3 pollution in the United States. Reduction of O3 concentrations requires control of either NOx or VOC emissions or a combination of both. For the first two decades of O3 pollution mitigation in the United States, VOCs were the primary targets for emission reductions. The initial decision to pursue VOC controls was based on results from federal smog chamber experiments and data collected at monitoring sites in several U.S. cities, as well as the recognition that VOC controls would reduce the concentrations of eye irritants. In 1971, EPA issued the so-called Appendix J curve (Figure 3-2) for use by state and local agencies in developing SIPs (Fed. Reg. 36 [1971]). The curve (essentially from a modified rollback model) was derived from observations in six U.S. cities. On the basis of the maximum O3 concentrations observed at these cities and their estimated VOC emissions, the curve purported to indicate the percentage of VOC emission reduction required to reach attainment in an urban area as a function of the peak concentration of photochemical oxidants observed in that area. In the late 1970s, a more sophisticated method involving the use of a photochemical box model was developed by EPA. The empirical kinetic modeling approach (EKMA) (Dimitriades 1977) used the improved chemical mechanisms that were under intense development in the late 1970s and early 1980s (Atkinson and Lloyd 1984) to simulate the production of O3 within an idealized air parcel as it advected over an urban area with VOC and NOx emissions. Figure 3-3 illustrates the typical output from EKMA—the so-called Haagen-Smit or EKMA diagram, where lines of constant peak O3 concentrations (called O3 isopleths) are plotted as a function of VOC and NOx emissions. Inspection of Figure 3-3 reveals distinct regions of VOC and NOx sensitivity. For VOC-to-NOx ratios less than about 4:1, the atmosphere is VOC limited, the most expeditious path to O3 control is VOC reduction, and a reduction in NOx could increase O3. However, for VOC-to-NOx ratios greater than about 15:1, NOx controls provide the best way to reduce O3. EKMA plots, as shown in Figure 3-3, captured the major features and complexities of the NOx/VOC/O3 system. However, to effectively apply the information to an O3 abatement strategy for a given nonattainment area, one must be able to accurately characterize where on the diagram an area is situated in terms of the VOC:NOx emissions ratio. Based on the available emissions inventories in the late 1970s and early 1980s, it appeared that most urban areas were near or above the ridge of the diagram, suggesting that VOC controls were the most effective path to attainment. Results using more sophisticated models, such as the UAM (urban airshed model), based on essentially the same emission inventories, generally |
supported the conclusion reached by the applications of the EKMA plots—that VOC emission controls provided the best path to attainment of the O3 NAAQS. As a result of these analyses, the SIPs submitted in response to the 1970 and 1977 CAA Amendments focused on reducing VOCs to reduce ambient O3, and from the early 1970s to the early 1990s, EPA and Congress promoted VOC control as the principal path to attaining the O3 standard.a However, by the mid-1980s, it became clear that few areas would actually meet the December 31, 1987, attainment deadline for the O3 standard required by the 1977 CAA Amendments; by 1990 some 100 areas were still classified as nonattainment for O3. At the same time, there was growing appreciation for the importance of NOx emission reductions. In the mid-1980s California began to pursue NOx controls. Reports from both the OTA (1989) and the NRC (1991) identified NOx control as an essential new direction for reducing O3 pollution. Although it has taken some time to implement this change, the late 1990s saw an increase in efforts to control NOx, including much more stringent NOx controls on heavy-duty diesel vehicles and the NOx SIP call for the eastern United States. A number of factors led to the slow pace of implementing substantial NOx emission controls to mitigate O3 pollution. Both the J curve and EKMA model had deficiencies, but the major reason was the incomplete and often erroneous emission inventories used. It was not until the late 1980s, with the analysis of data gathered from various field experiments carried out in Southern California and the Southeast United States, that two deficiencies were identified in these inventories: a significant underestimate of VOC emissions from mobile sources (Pierson et al. 1990), and a failure to account for biogenic VOC emissions (Chameides et al. 1988). As these deficiencies were addressed, it became apparent that a greater emphasis on NOx controls was needed (NRC 1991). In retrospect, it is possible that more progress in mitigating O3 pollution in the United States might have been achieved had there been a greater reliance on analysis of field data and the use of observation-based methods (Roth et al. 1993) to complement the more traditional emissions-based modeling approaches during the first two or three decades of O3 mitigation efforts. |
development of an urban-scale 3D chemical transport (grid-based) model—the urban airshed model (UAM). At the same time, other urban-scale 3D models were developed and used by the scientific community (Reynolds et al. 1973). In the 1980s, the use of 3D models spread to other major metropolitan areas, and the 1990 CAA Amendments specifically called for
the use of such models for all O3 nonattainment areas classified as serious and above.
Today, the scientific and engineering community has developed more than 20 3D CTMs for O3 in the United States and Europe, in addition to the UAM (see Russell and Dennis [2000] for a detailed review). These models continue to grow and develop as new scientific information becomes available and as regulatory needs dictate. For example, O3 pollution was initially viewed as an urban problem, and the initial versions of the UAM were restricted to simulating individual urban areas. Today, it is recognized that O3 pollution extends over multistate airsheds, and the current version of the UAM and many other contemporary 3D photochemical models used to simulate O3 pollution are designed to treat this larger spatial domain.
Modern Multipollutant, Multiscale CTMs
Even as the UAM and other urban-scale CTMs were being developed and refined in the 1980s, the lessons learned from the application of these models were being applied to other types of CTMs. One of the most important of these efforts was the National Acid Precipitation Assessment Program’s development of a regional (or multistate airshed) CTM able to simulate acidic deposition in the eastern United States and Canada (NAPAP 1991b). The resulting model, the regional acid deposition model (RADM), included state-of-the-science representations of the physical and chemical processes leading to the formation and deposition of acidic species over multistate geographic areas (Chang et al. 1987). The model (together with similar tools, such as the ADOM model developed for Canada) provided important insights into the source-receptor relationships in the acid deposition problem in the United States and Canada. Although these models ultimately played a relatively minor role in the development of the Acid Rain Program enacted in the CAA Amendments of 1990,6 they represented a major step in the development of CTMs—namely, the expansion of the algorithms used in urban-scale CTMs for O3 into a more comprehensive regional-scale model able to simulate processes related to the formation, transport, and deposition of PM as well as gaseous pollutants.
Just as the development of urban-scale CTMs for O3 aided in the development of regional acid deposition models, such as RADM, the development of RADM provided the basis for significant advances in CTMs used for O3 pollution. Most present-day O3 models have regional rather than urban-scale coverage. Many have multiscale and nesting capabilities so that they can simulate a relatively large multistate area but also focus on
6 |
A more-detailed discussion of the Acid Rain Program is presented in Chapter 5. |
one or more localities of interest (for example, an urban center). Many of these models also have more advanced treatments of clouds and depositional processes, which can have a significant effect on O3 and related gaseous pollutants.
The proposed fine particulate (PM2.5)7 NAAQS and the need for models to carry out the related SIP attainment demonstrations will present an opportunity to build further upon the experience gained from modeling both O3 and acid deposition. Fine particulate matter comes not only from direct emissions but also from atmospheric reactions that transform primary pollutants such as sulfur dioxide (SO2), NOx, ammonia, and VOCs into particulate sulfate, nitrate, carbonaceous material, and ammonium. Some of the processes that transform gases into PM take place in clouds and are therefore closely tied to the processes that lead to acidic precipitation. As a result, models for PM2.5 require a conjoining of processes relevant to the formation of ground-level O3 and to the formation of acid deposition. Because of this complexity, most models capable of simulating PM can also be used to address other air pollutant problems (O3, acidity, and visibility reduction), and for that reason, they are also referred to as multipollutant or unified air quality models. A prime example of such a model is EPA’s new Models-3, which is available to both the scientific and regulatory communities in various forms. Seigneur (2001) has published a review of the strengths and weaknesses of these tools.
Observation-Based Model for O3
One significant problem with emissions-based air quality models for O3 is their dependence on uncertain VOC and NOx emission inventories (see Box 3-4). In recognition of that limitation, additional diagnostic tools have been developed to complement the air quality models. These tools, sometimes referred to as “observation-based methods,” use ambient air quality measurements rather than emission inventories to determine the relative effectiveness of VOC and NOx emission reductions on O3 pollution mitigation. The advantage of these methods is that they are not affected by uncertainties in emission inventories. On the other hand, they have two distinct disadvantages: (1) they require high-quality data on the ambient air concentrations of relevant chemical constituents, and much of those data are not gathered in routine monitoring networks; and (2) they are diagnostic and not prognostic (predictive). The latter limitation means that observation-based models can usually be used to estimate the types but not the amounts of emission reductions needed to meet a specific air quality
goal or standard (NARSTO 2000). For that reason, observation-based models provide a useful adjunct to emissions-based models in an attainment demonstration but are not a substitution for them.
Air Quality Models: Lessons Learned
Scientists have had over three decades of experience in developing and applying a number of models for air quality management, and in that time, a number of insights into what is working well and what is not working well have been gained.
What Is Working Well
-
The dynamic partnership existing between the technical and regulatory communities promotes continuous improvement and advancement in air quality modeling and management. On the one hand, the evolving needs of the regulatory community promoted and catalyzed the scientific and engineering communities to develop increasingly sophisticated air quality models; on the other hand, insights gained from advanced models have prompted members of the regulatory community to rethink their approaches to air quality management.
What Is Not Working Well
-
As a general rule, models should be subjected to comprehensive performance evaluations using detailed data sets from the atmosphere before using them in regulatory applications (Roth 1999; Seigneur et al. 2000). Unfortunately, because of the lack of adequate atmospheric data (such as those on pollutant concentrations), that rule is all too often overlooked by the regulatory community.8 The consequences of this practice can be and, in some instances, have been the promulgation of less than optimal or even ineffective control strategies (see Box 3-4).
-
Model results are sometimes used inappropriately by regulators. One example is the practice in attainment demonstrations of using a model to predict pollutant concentrations years or even decades into the future without recognizing that input parameters, which are based on estimates of future socioeconomic factors, for the simulation are highly uncertain.
-
Substantial delays occurred in incorporating new scientific insights from models into policy design. One example is the reliance on VOC
-
controls for O3 pollution long after science suggested that this strategy was not effective.
-
The ability to quantify uncertainty in the predictions of state-of-the-science chemical transport models remains inadequate, although improvements have been made.
-
In a number of areas of the country, there have been inadequate resources (financial and personnel) for the correct application of these tools to local and regional problems.
Beyond the specific concerns listed above, there are two broader concerns—model uncertainty and over-reliance on models for O3 SIPs—and one important opportunity—multipollutant models—that bear more detailed discussion.
Model Uncertainty
An assessment of the uncertainties in model output has not been a routine component of the information used by regulators. Unfortunately, the uncertainty in model predictions depends on a variety of factors, including the type of prediction (such as variables and averaging time), the application, the uncertainties associated with the model’s input and internal parameters, and the model itself. As a result, a universal, comprehensive statement cannot be made about the uncertainties of any model simulation (see Oreskes et al. 1994). Literature estimates for individual components of an air quality model—emissions, chemistry, transport, vertical exchange, deposition—typically indicate uncertainties of 15–30%, but when the supporting data sets are weak, the uncertainties can be significantly higher. A consideration of all factors reveals that model uncertainty can be both significant and poorly characterized (NARSTO 2000). It follows, therefore, that relying solely on the output of an air quality model to resolve emission-control issues or to demonstrate attainment of an air quality standard or objective is problematic.
Despite these limitations, air quality models remain the only tools available for quantitatively simulating or estimating future outcomes. Although challenging to use, air quality models are essential to the current AQM system. Their use can be optimized by collecting and analyzing appropriate data sets, carefully assessing model sensitivity and uncertainty, and avoiding inappropriate applications.
Over-Reliance on Models for O3 SIPs
As discussed in more detail later in this chapter, SIPs developed for O3 nonattainment areas have relied heavily on so-called attainment demon-
strations, in which an air quality model is used to determine the amount of emission reductions needed to reach attainment by a specified date. In general, these attainment demonstrations have tended to be overly optimistic, and O3 concentrations in these areas have tended to exceed the values projected by the models (API 1989).
An Opportunity: Emerging Multipollutant Models
The growing development and use of multipollutant models suggests that a multipollutant approach to AQM is now viable. The current approach in the United States tends to ignore the interrelationships among pollutants. For the most part, planning and regulatory efforts have occurred without serious consideration of the linkages among pollutants and the commonality of their sources (the one exception being particles and visibility). At the very least, the effort to solve each air quality problem in isolation from the rest has probably resulted in missed opportunities to address different pollutants simultaneously. Future multipollutant modeling efforts should be enhanced to support strategies for simultaneous reduction of multiple pollutants.
Using the Weight-of-Evidence Approach in the Attainment Demonstration
In recognition of the uncertainties inherent in the use of the air quality models, EPA now encourages states to perform complementary analyses using available air quality, emission, and meteorological data along with outputs from alternative receptor- and observation-based models before arriving at a final target for the emission reductions that will be needed to meet attainment. These complimentary analyses can be used to assess the reasonableness of the results obtained from the air quality model simulations or as a weight-of-evidence determination that a given amount of emission reductions will be adequate to meet the NAAQS attainment standard.
The inclusion of a weight-of-evidence analysis in attainment-demonstration SIPs should be a positive development from a scientific and technical point of view. It implicitly acknowledges the limitations of air quality model simulations and allows planners to use information and insights from existing data and other analytical procedures to develop a more comprehensive and conceptual understanding of the relationship between pollutant emissions and the concentration of a criteria pollutant. In principle, such an understanding can make it possible for SIP developers to arrive at a more robust estimate of the emission reductions that will be needed to reach attainment. However, for this approach to work, the weight-of-
evidence analysis must be applied in an unbiased manner and not simply to justify lower emission reductions than those indicated by air quality model simulations. The introduction of the weight-of-evidence approach in the 1996 guidance document for preparing new SIPs for the 1-hr O3 standard appeared to have invited such a biased application, and bias in using the weight-of-evidence approach has been alleged in legal challenges to SIPs for a number of states. More recent guidance documents for SIPs for the 8-hr O3 standard (EPA 1999e) and for PM2.5 and regional haze (EPA 2001c) appear to have a more balanced approach. Time will tell how states will respond to this guidance.
Finally, the inclusion of the weight-of-evidence analysis does not eliminate a major problem in the current SIP process. This problem—an overemphasis on the emissions target obtained in the attainment demonstration—is discussed in more detail later in this chapter.
Emission-Control Strategy Development in an Attainment-Demonstration SIP
When the total amount of emission reductions required to reach attainment of the NAAQS has been determined though modeling and weight-of-evidence analysis, air quality planners must devise a strategy of emission controls and enforcement to bring about these reductions by the required date. In principle, these reductions can be derived from measures designed to change sociological and behavioral factors that influence pollutant emissions as well as from technological changes that directly affect emissions. In practice, however, AQM in the United States has emphasized technological solutions (see Box 3-5).
The emission-control strategy in an attainment-demonstration SIP is typically developed in stages, as the emission reductions from federal measures, mandatory local measures, additional local measures, and possible regional multistate measures are assessed consecutively.
Federal Measures
The CAA empowers EPA to impose nationwide emission-control measures on selected industries (for example, motor vehicle manufacturing facilities). When developing a SIP, state and local agencies can include the emission reductions anticipated from these measures. The specific amount of emission-reduction credit an area is allowed from each federal measure is determined from EPA-supplied guidelines and models. For most states, emission-reduction credits from federal control measures have represented a major fraction of the emission reductions in their respective SIPs (see Box 3-6); thus, the existence of federally mandated emission-control measures
BOX 3-5 One of the challenges facing the SIP process and the attainment of NAAQS has been the inability of air quality management in the United States to rely on social and behavioral measures in addition to technological innovations to improve air quality. The 1970 CAA Amendments were passed at a time of great environmental activism. In addition to technological requirements, the amendments contained provisions to obtain emission reductions from actions to change behavior, such as reducing vehicle miles traveled or changing land-use planning. Early efforts to implement these measures (for example, through increased tolls, parking freezes, and other measures to discourage travel) were met with such intense political resistance that EPA was expressly prohibited in the 1977 CAA amendments from imposing the more stringent measures on any area. The 1977 CAA Amendments did include, however, the requirement that transportation-control measures be enacted in certain nonattainment areas. In the 1990 CAA Amendments, Congress added the employee commute options requirement. Even these less stringent measures have met with resistance from those who do not believe that implementation of the CAA should result in changes in life style. In 1995, Congress repealed the employee commuter requirements and placed limitations on the use of enhanced I/M requirements. As a result, most emission reductions continue to come from technological changes. In some instances, the inability of air quality managers to affect social and behavioral patterns has significantly degraded the impact of the technological changes that were imposed to lower pollutant emissions (see, for example, the discussion on mobile emissions in Chapter 4). |
have eased the burden of state and local authorities in developing attainment SIPs.
Mandatory Local Measures
Once an area has accounted for emission reductions based on federal measures, the area must then plan and account for local emission-control measures that are specifically mandated by the CAA. The extent of these mandatory measures depends on the pollutant and the classification of the area. As detailed in Box 3-3, the measures range from the imposition in all nonattainment areas of reasonably available control technology (RACT) on large stationary sources, such as electricity-generating and industrial combustion facilities, to the promulgation of the reformulated gasoline program in severe and extreme O3 nonattainment areas. As in the case of the federal measures, the credits for emission reductions based on each mandatory measure are determined by EPA-supplied guidelines and models. The emission reductions allowed by EPA for some of these measures (for
BOX 3-6 When an area is found to be in nonattainment with a NAAQS, the relevant state (and/or local) agency must submit an attainment-demonstration SIP to EPA. Among other things, the SIP must contain a list of emission-control measures that, when implemented, will bring the area into compliance. The control measures may include those promulgated at the national level by the federal government as well as those promulgated at the state and local level. To illustrate how the mix of federal, state, and local emission programs have been adopted, the O3 attainment-demonstration SIPs from four nonattainment areas are examined here:
Although some of these areas have submitted SIP revisions more recently, the ones chosen were those with the most comprehensive discussion of emission-reduction strategies since passage of the 1990 CAA Amendments and are thus most appropriate for our discussion here. Tables 3-2 and 3-3 list the federal, state, and local emission-control measures and anticipated emission reductions for the two O3 precursors, VOCs and NOx, for each of the SIPs. The identification of a reduction as a federal, state, or local measure in the SIPs is determined by the states themselves and is therefore not consistent among the states. For example, stringent motor vehicle standards initially implemented in California and then later extended to other states in a federal regulation are counted as a state measure in California and a federal measure elsewhere. In addition, most states claim I/M programs as a state initiative rather than a federal control, even though the CAA Amendments of 1990 require areas in moderate O3 nonattainment to have basic I/M programs and areas in serious and above O3 nonattainment to have enhanced I/M programs. The data in the tables suggest that federal programs often play a major role in the emission reductions assumed in SIPs. Federal measures are responsible for the majority of VOC emission reductions in all but one of the examples. California is the exception in that it claims that nearly all of its emission reductions result from state and local initiatives. In the case of NOx emission controls, the federal measures result in essentially no reductions in Southern California and an almost 30% decrease in Wisconsin. In the Philadelphia-Trenton-Wilmington area, multistate measures, specifically the NOx SIP call, account for about 30% of the anticipated NOx emission reduction. |
TABLE 3-2 Federal, State, and Local VOC Emission-Reduction Measures in Four Illustrative SIPsa
|
Houston-Galveston, TX |
South Coast, CA |
Southeast Wisconsin |
Philadelphia, PA; Trenton, NJ; Wilmington, DE |
Attainment date |
2007 |
2010 |
2007 |
1999 |
1990 base year inventory |
945 |
1,517 |
407 |
616 (grown to 625 in 1999) |
Inventory for attainment |
694 |
323 |
222 |
431 |
Reductions needed |
251b |
1,194 |
185 |
164 |
Reductions from: |
|
|
|
|
Existing state and local programs |
— |
463 (39%) |
— |
— |
Existing federal programs |
133 (81%) |
0 (0%) |
110 (57%) |
40 (23%) |
New local measures |
2 (1%) |
453 (38%) |
0 |
18 (10%) |
New state measures |
30 (18%) |
231 (19%) |
12–17 |
59–77 |
|
|
|
(7%)c |
(39%)c |
Mobile |
12 |
114 |
6 |
— |
Enhanced I/M |
18 |
26 |
4 |
59 |
Stage-2 vapor recovery |
— |
— |
2 |
0–18d |
Pesticides |
— |
2 |
— |
— |
Consumer products |
— |
89 |
— |
— |
Industrial controls |
— |
— |
0–5e |
— |
New federal measures |
0 (0%) |
47 (4%) |
67–72 |
40–58 |
|
|
|
(36%)c |
(28%)c |
Off-road vehicles |
— |
43 |
— |
— |
RACT |
— |
— |
67–72e |
10 |
On-board vapor recovery |
— |
— |
— |
0–18d |
Other |
— |
4 |
— |
30 |
Total |
165b |
1,194 |
194c |
175c |
a VOC emissions and emission reductions in tons per day (tpd, Texas and California) and tons per summer day (tpsd, Wisconsin and Pennsylvania). b The VOC emission-reduction measures described in the Houston-Galveston SIP (that is, 165 tpd) are less than the state claims ar e needed for attainment (that is, 251 tpd). c Mid-point of the range was used in calculations. d Documentation is unclear about contribution of Stage 2 and on-board vapor recovery systems. e Documentation is unclear about contribution of state measures and federal RACT. |
TABLE 3-3 Federal, Multistate, State, and Local NOx Emission-Reduction Measures in Four Illustrative SIPsa
|
Houston-Galveston, TX |
South Coast, CA |
Southeast Wisconsin |
Philadelphia, PA; Trenton, NJ; Wilmington, DE |
Attainment date |
2007 |
2010 |
2007 |
1999 |
1990 base year inventory |
1,236 |
1,361 |
397 |
439 |
Uncontrolled inventory for attainment year |
1,375 |
|
|
455 |
Inventory for attainment |
414 |
553 |
297 |
373 |
Reductions needed |
961b |
808 |
100 |
82 |
Reductions from: |
|
|
|
|
Existing state and local programs |
0 (0%) |
429 (53%) |
0 (0%) |
0 (0%) |
Existing federal programs |
209 (23%) |
0 (0%) |
27 (26%) |
17 (21%) |
New local measures |
24 (3%) |
43 (5%) |
0 (0%) |
0 (0%) |
New state measures |
674 (74%) |
227 (28%) |
75 (74%) |
32 (39%) |
Point source |
586 |
— |
53 |
— |
Enhanced I/M |
36 |
31 |
17 |
32 |
Mobile (other) |
35 |
196 |
5 |
— |
Other |
17 |
— |
— |
— |
New multistate measures |
0 (0%) |
0 (0%) |
0 (0%) |
27 (33%) |
NOx SIP call |
— |
— |
— |
27 |
New federal measures |
4 (<1%) |
109 (14%) |
0 |
6 (7%) |
Off-road vehicles |
— |
87 |
— |
— |
Other |
4 |
22 |
— |
6 |
Total |
911b |
808 |
102 |
82 |
aNOx emissions and emission reductions in tons per day (tpd, Texas and California) and tons per summer day (tpsd, Wisconsin and Pen nsylvania). bThe NOx emission reduction measures described in the Houston-Galveston SIP (that is, 911 tpd) are less than the state claims are needed for attainment (that is, 961 tpd). |
example, I/M and oxygenated fuels) have been criticized as being unrealistically large (NSTC 1997; NRC 2001c, 1996).
Additional Local Measures
As envisioned in the CAA, if the emission reductions projected by federal measures, regional measures (see discussion in next section), and local mandatory measures are not sufficient to bring about compliance, the air quality planners must identify additional local emission controls to make up the shortfall. The only specific requirements on these additional emission reductions are that they be sufficient to meet the areawide target reduction indicated by the attainment demonstration and that the measures are verifiable and enforceable. They can involve deeper emission reductions from sources already regulated via federal or mandatory local measures and emission controls on previously unregulated sources, such as lawnmowers, small industrial and consumer products, open burning, and construction equipment. Although EPA cannot consider costs in setting NAAQS, states and local authorities can and do consider costs and cost-effectiveness when identifying the mix of local emission controls to be included in the SIP. Inclusion of economic incentive programs, such as cap-and-trade programs discussed in Chapter 6, and consideration of environmental justice, as discussed in Chapter 2, are also emerging as important drivers in the process.
Multistate Regional Measures
At the time of the initial passage of the CAA, it was thought that nonattainment within a given area was largely caused by emissions within that area and could be mitigated by local emission controls. It was reasonable, therefore, to place the final responsibility for devising a plan to mitigate this pollution on local and state authorities. By the end of the 1980s, however, it had become apparent that some air quality problems had a larger, multistate component and that a substantial contribution to an area’s pollution could arise from upwind emission sources. In response, Congress included provisions in the 1990 CAA Amendments for implementing multistate air pollution mitigation strategies through the creation of regional planning organizations (RPOs). Although these RPOs cannot be vested with regulatory authority, in principle, their strategies can be implemented and legally mandated by EPA’s issuance of an appropriate multistate rule and by the voluntary inclusion of the measures in the SIPs prepared by the states. As a result, a number of statutory as well as ad hoc RPOs have emerged. In the eastern half of the United States, where regional O3 pollution has been an important concern, organizations such as the Ozone Trans-
port Commission (OTC) and the ad hoc Ozone Transport Assessment Group (OTAG) were established. In the West, where degrading visibility in Class 1 areas has been a growing problem, the Grand Canyon Visibility Transport Commission and Western Regional Air Partnership (WRAP) was established. A brief overview of those three efforts and their relationship to two major regional rules promulgated by EPA (the NOx SIP call and the regional haze rule) is provided below.
The O3 Transport Region and O3 Transport Assessment Group
In recognizing that O3 and its precursors can be transported over large distances and that O3 nonattainment areas, especially in the eastern United States, can be affected significantly by upwind sources, the 1990 CAA Amendments created the Ozone Transport Region (OTR) to help states in the northeastern United States develop a coordinated strategy. The amendments also authorized EPA to create other O3 transport regions.
The states from Virginia to Maine and the District of Columbia make up the OTR. Their representatives and the EPA offices constitute the OTC, which Congress created to formulate solutions to regional O3 pollution. OTC recommends a variety of VOC- and NOx-reduction measures, which the state governments then make enforceable by incorporating them into their respective SIPs. The major initiatives adopted thus far by OTC to address regional O3 pollution include the California low-emissions vehicle (LEV) program and NOx budget trading program for electric utilities and large industrial boilers. Phase I of the program included the installation of RACT, which was required in the 1990 CAA Amendments of all OTR states. Phases II and III committed OTR states to further regional NOx emission reductions in 1999 and 2003 via an integrated interstate emissions-trading program.9
OTAG was established in 1995 by EPA and the 31 states east of the Mississippi River. The major impetus for establishing OTAG was the inability of O3 nonattainment areas in the eastern United States to submit complete SIPs by the 1994 deadline mandated in the 1990 CAA Amendments. The incompleteness of the SIPs arose from the inability of state and local regulators to develop viable plans to reach attainment on the basis of local emission contributions alone. Therefore, OTAG was formed to assess the role of more distant sources in causing O3 nonattainment in localized, largely urban areas and to develop strategies for mitigating their contributions.
In contrast to the OTC formation, the affected states played a major role in initiating OTAG. In addition to EPA and the relevant state agencies,
9 |
This program is discussed in more detail in Chapter 5. |
affected industrial and environmental groups, the academic research community, and Canadian representatives participated in the deliberations. The work and deliberations carried out by OTAG produced a general consensus recommendation to EPA for a new regulatory initiative to reduce NOx emissions throughout the region. In response, EPA proposed a NOx SIP call that would cap NOx emissions in 22 states and the District of Columbia. The caps were imposed on states on the basis of their estimated impact on regional O3 concentrations and not on the basis of their own attainment status with regard to the O3 NAAQS. Following a series of court challenges by midwestern states and industry groups to some of the details of EPA’s proposed cap, the NOx SIP call rule was adopted with some changes and will become effective in 2004. The lengthy regulatory and legal challenge process substantially delayed the implementation of the regional NOx SIP call program and the associated air quality benefits. The OTAG process will have taken 9 years from its initiation to deliver its initial NOx reductions via the NOx SIP call. Under the OTC NOx budget program, which relied on more explicit authority in the 1990 CAA Amendments, the initial NOx reductions were achieved in only 5 years.
OTC and OTAG have provided effective mechanisms for facilitating coordination among states faced with air quality problems that have a significant multistate or regional component. However, the actual emission-reduction strategies developed by those groups have yet to be fully implemented; therefore, it is too early to determine whether these strategies will bring about the desired O3 reductions.
EPA’s Regional Haze Rule
Section 169(a) of the 1990 CAA Amendments required EPA to establish regulations to ensure reasonable progress in improving visibility in 156 national parks and wilderness areas (Class I areas) in the United States. In response, EPA issued a regional haze rule in 1999. The rule sets specific visibility improvement targets for the nation (described in Chapter 2) and then requires all states to develop plans to achieve “reasonable progress” toward those goals.
A notable aspect of the regional haze rule is that even states that do not have visibility-degraded Class I areas are required to submit SIPs to reduce the sources of pollution from the states that contribute to visibility degradation elsewhere. Thus, like the NOx SIP call, EPA’s regional haze rule represents a program that attempts to address a regional air pollution problem by making all contributors to the problem contribute to the solution even if they do not suffer significantly from the consequences of this pollution.
Although the rule requires all states to participate, it does not impose specific intra- or interstate emission controls or limits. Instead, all but nine
western states are required to develop long-term strategies for achieving the visibility improvement goals set by EPA and to submit these strategies in the form of a regional haze SIP to EPA for approval and review. The nine western states exempted from this procedure are Arizona, California, Colorado, Idaho, Nevada, New Mexico, Oregon, Utah, and Wyoming. As described below, these states, whose pollution has been deemed to contribute most significantly to visibility degradation in the Grand Canyon National Park, are treated in a separate section of the regional haze rule that allows them to implement the strategies recommended by the Grand Canyon Visibility Transport Commission (GCVTC).
The regional haze rule gives states the option of developing their own implementation plans but encourages them to work collaboratively with other states by forming RPOs. Today there are five regional planning organizations addressing regional haze: WRAP is working to implement the recommendations of the GCVTC (see below), the Central States Regional Air Partnership (CENRAP), the Midwest Regional Planning Organization (Midwest RPO), the Mid-Atlantic/Northeast Visibility Union (MANE-VU), and the Visibility Improvement State and Tribal Association of the Southeast (VISTAS).
Problems have arisen despite the authority of EPA to promulgate multistate regulations to address visibility degradation. For example, when developing their long-term implementation strategies, states are required by the regional haze rule to identify all major sources to which the statute’s best available retrofit technology (BART) requirement can be applied. However, the Court of Appeals for the District of Columbia Circuit has set aside this aspect of the rule on the grounds that it impermissibly constrains the states’ authority to make individualized BART determinations (American Corn Growers Ass’n v. EPA, 291 F.3d 1 [D.C. Cir. 2002]). To help avoid such problems in the future, a recommendation is made in Chapter 7 to enhance EPA’s responsibility for multistate air pollution problems.
Grand Canyon Visibility Transport Commission and the Western Regional Air Partnership
Along with the OTC, the 1990 CAA Amendments also instructed EPA to form GCVTC to deal with fine-particle haze that impaired visual air quality in the Grand Canyon National Park (42 U.S. Code § 7492(f)). The purpose of GCVTC, which EPA officially established in 1991, was to develop consensus recommendations on measures to protect visual resources in the national parks and wilderness areas of the Colorado plateau. Voting members of GCVTC included representatives from eight western states, four tribes, the Columbia River Inter-Tribal Fish Commission, and five federal agencies. Over a 5-year period, GCVTC and its committees per-
formed scientific, technical, and economic assessments of existing and projected visibility in the region and obtained public input on air quality management alternatives. GCVTC reported its final recommendations to EPA in 1996. The regional haze rule that EPA issued in 1999 included separate provisions and deadlines for the western states and tribes to pursue GCVTC’s recommendations (40 CFR § 51.309).
WRAP was formed in 1997 as the successor to GCVTC. Eleven western tribes and nine states are listed as WRAP members (WRAP 2003). In September 2000, WRAP submitted an annex to the 1996 GCVTC report, proposing measures to implement GCVTC recommendations and meet the requirements of the 1999 regional haze rule (WRAP 2000). The annex addresses the period through 2018 and features a shrinking emissions cap-and-trade program for SO2 emissions in the GCVTC region. WRAP expects the emissions cap to be met through voluntary measures; thus, the trading program serves as a “backstop” in case the voluntary measures are not sufficient. EPA proposed to approve the annex on May 6, 2002 (67 Fed. Reg. 30418 [2002]).
Institutional Accountability in the SIP Process
The original 1970 CAA Amendments required SIPs to demonstrate that the primary NAAQS would be achieved in every area within a state by 1977 at the latest. When most urban areas of the country failed to meet that deadline for one or more pollutants, Congress amended the statute in 1977 to require states to demonstrate that attainment of NAAQS would be achieved in every area by 1987 at the latest. In addition, the 1977 Amendments provided for sanctions that EPA could impose upon states that failed to submit adequate plans and for states that did not attain the standards by the deadlines. In especially difficult cases, EPA could take action by writing a federal implementation plan (FIP). Possible sanctions included the withdrawal of federal highway funds for all but the most critical safety-related highway projects in the area and a ban on construction of new major stationary emission sources in the area. In the mid-1980s, EPA instituted sanctions on a few areas that failed to meet the 1983 deadline for PM and SO2, but the agency refrained from administering sanctions in areas in states that had submitted plans for attaining the O3 standards by the 1987 deadline plans that later proved to be inadequate. In addition, EPA rarely wrote FIPs for states that had submitted SIPs that did not appear adequate to achieve NAAQS attainment.
In response to the failure to meet the 1980s deadlines, the 1990 CAA Amendments specified attainment deadlines that depended on how far an area was from reaching attainment in 1990 (see Table 3-1) and a new “bump-up” provision for dealing with areas that failed to meet their attain-
ment deadlines. In this provision, the EPA administrator is directed to reclassify any O3 nonattainment area that is ranked below severe that fails to attain the NAAQS by its attainment date to “the higher of (i) the next higher classification for the area, or (ii) the classification applicable to the area’s design value.” Since the SIP requirements for O3 nonattainment areas increase dramatically as the classification increases, the automatic bump-up provision provides a sanction of sorts for areas that fail to reach attainment by the required dates. However, EPA has only rarely complied with its statutory obligation to make timely findings that areas have not achieved attainment by the statutory deadlines and thereby bump up their nonattainment status. In some cases, EPA has applied a “downwind extension policy” under which areas that did not achieve attainment by the relevant deadlines because of emissions or transport of emissions from other areas would not automatically be bumped up. Nevertheless, one district court ordered EPA to make the required nonattainment finding in a timely fashion, and three courts of appeals found the downwind extension policy to be invalid.10
In addition to the bump-up provision, the 1990 CAA Amendments provide other more tangible penalties and sanctions for states that fail to submit a SIP or submit an inadequate SIP. These include the authority for EPA to write a FIP as well as to impose two types of sanctions: (1) cutting off federal highway funds for the area, and (2) limiting the construction or modification of major new sources in the area by requiring two-for-one offsets from other sources of the same pollutant in the same or (in some cases) in adjacent areas (42 USC § 7509(a), (b)). A new aspect of the sanctions provision in the 1990 CAA Amendments is the requirement for automatic imposition of sanctions on deficient areas. According to the statute, once an area has been found by EPA to be deficient because of a failure to submit an adequate SIP, that area has 18 months to correct the deficiency. At the end of this 18-month “sanctions clock,” EPA must impose at least one of the two sanctions on the area and keep the sanctions in effect until the deficiency has been corrected.
At the time of the writing of this report, EPA has had to impose one or more of the sanctions mandated in the CAA Amendments of 1990 in 28 instances. An additional 39 areas under the 18-month sanctions clock are facing the imposition of sanctions in the near future unless they can correct the relevant deficiency or deficiencies. For the most part, when sanctions have gone into effect, states have acted rapidly to have them removed, and as a result, they have tended to remain in effect for relatively brief periods
(in many cases, sanctions were lifted within weeks or even days of being imposed). In virtually all cases where highway sanctions were imposed, they did not result in an actual loss of highway funds, because no highway projects were being proposed in the areas during the brief periods that the sanctions were in effect. (The two exceptions were East Helena, MT, and Iron County, MO.) The rapidity with which states and areas acted to remove sanctions that had been imposed by EPA might indicate that the requirement for mandatory sanctions in the 1990 Amendments did in fact provide a positive impetus for compliance with the CAA and the SIP requirements. However, it is beyond the scope of this report to determine whether that was the case. The imposition of sanctions appeared to have little or no direct economic impact on the affected areas and states.
THE EFFECTIVENESS OF THE SIP PROCESS
Two basic metrics can be used to assess the effectiveness of the SIP process. The less stringent metric is based on an assessment of whether implementation of SIPs has resulted in a general decrease in pollutant emissions and concentrations and, more specifically, in a decrease in criteria pollutant concentrations in nonattainment areas. As discussed in more detail in Chapter 6, data from the nation’s air quality monitoring networks suggest that the SIP process has resulted in considerable progress in improving air quality on the basis of this metric.
The more stringent metric is based on whether implementation of federal, regional, and local programs through the SIP process has resulted in the attainment of the NAAQS for criteria pollutants. On that basis, the effectiveness of the SIP process is less apparent. For example, in Table 3-4, where the number of nonattainment areas in the United States in 1992 and 2002 are listed for each of the criteria pollutants, significant progress is clearly shown. The number of nonattainment areas for CO, lead (Pb), SO2, and nitrogen dioxide (NO2) have decreased substantially. On the other hand, with the exception of NO2, nonattainment areas for each of these pollutants still exist. In the case of O3, there has also been a substantial decrease in the number of nonattainment areas. However, most of the areas that have been redesignated as being in attainment had a moderate or marginal classification. None of the serious O3 nonattainment areas, which were scheduled to reach attainment in 1999 in the 1990 CAA Amendments, have been redesignated. Similarly, all the areas classified as severe and extreme remain on the nonattainment list; however, they are not scheduled to reach attainment until 2005 and 2010, respectively. Thus, the effectiveness of the current SIP process in addressing serious and above O3 nonattainment has yet to be established. Of even greater concern is the lack of progress in alleviating nonattainment of the PM10 NAAQS, especially given
TABLE 3-4 Classifications and Number of Nonattainment Areas in 1992 Remaining in Nonattainment as of February 6, 2003
Pollutant |
Classification (ppm) |
1992 |
2003 |
CO |
Serious (≥ 16.5) |
7 |
6 |
|
Moderate (12.7 to 16.4) |
4 |
1 |
|
Moderate (9.1 to 12.7) |
32 |
4 |
|
Not classifieda |
33 |
2 |
|
TOTAL |
76 |
13 |
Pb |
— |
13 |
3 |
NO2 |
— |
1 |
0 |
O3 |
Extreme (≥ 0.280) |
1 |
1 |
|
Severe-17 (0.190 to 0.280)b |
5 |
5 |
|
Severe-15 (0.180 to 0.190)c |
5 |
5 |
|
Serious (0.160 to 0.180) |
14 |
15 |
|
Moderate (0.138 to 0.160) |
32 |
8 |
|
Marginal (0.121 to 0.138) |
44 |
21 |
|
Section185Ad |
11 |
3 |
|
Othere |
2 |
1 |
|
TOTAL |
137 |
73 |
PM10f |
Serious |
8 |
8 |
|
Moderate |
78 |
58 |
|
TOTAL |
86 |
66 |
SO2 |
Primaryg |
48 |
21 |
|
Secondaryh |
6 |
6 |
a“Not classified” is an area designated a CO nonattainment area as of the date of enactment of the CAA Amendments of 1990, which did not have sufficient data to determine if it is meeting or is not meeting the CO standard. b“Severe-17” nonattainment areas have 17 years to attain standards. c“Severe-15” nonattainment areas have 15 years to attain standards. d“Section 185A” of the CAA (previously called transitional) is an area that has designated an O3 nonattainment area as of the date of enactment of the CAA Amendments of 1990 and that has not violated the national primary ambient air quality standard for O3 for the 36-month period beginning January 1, 1987, and ending December 31, 1989. eThis category includes areas that violate the O3 standard and have a design value of less than 0.121 ppm. That occurs when the exceedance is higher than the O3 standard exceedance rate of 1.0 per year, even though the estimated design value is less than the standard. fThis is not an official list of nonattainment areas. See the Code of Federal Regulations (40 CFR Part 81) and pertinent Federal Register notices for legal lists and boundaries (http://www.epa.gov/oar/oaqps/greenbk/pfrnrpt1.html). For an area designated as being in nonattainment of the NAAQS for PM10, Section 188 of the CAA outlines the process for classification of that area and establishes that area’s attainment date. At the time of designation, all PM10 nonattainment areas are initially classified as moderate by operation of law. A moderate area can subsequently be reclassified as serious either before the applicable moderate area attainment date, if EPA determines the area cannot “practicably” attain the PM10 NAAQS by this attainment date, or after the passage of the applicable moderate area attainment date, if EPA determines the area has failed to attain the standards. g“Primary” standard based on health-related effects. h“Secondary” standard based on welfare-related effects. Abbreviation: ppm, parts per million. SOURCE: EPA 2003h. |
the likelihood that the promulgation of the new PM2.5 NAAQS will give rise to a large number of PM nonattainment areas.
Critical Discussion of the SIP Process
The SIP process is an important and essential component of the nation’s AQM system. It allows state and local agencies to take into account emission controls adopted at the federal and multistate levels and then choose a suitable suite of additional local emission-control measures to reach attainment. On balance, this process should provide an appropriate division of responsibility. It can also provide the basis for a constructive partnership between the federal and state governments that steadily improves air quality on the local, multistate, and national levels. Air quality monitoring data confirm that such improvements have occurred in the past two decades (see Chapter 6). Nevertheless, important adjustments to the SIP process are needed if the difficult challenges ahead are to be effectively addressed. Some of the major concerns are discussed below. Recommendations for addressing these concerns are presented in Chapter 7.
An Overly Bureaucratic Process
The SIP process now mandates extensive amounts of local, state, and federal agency time and resources in a legalistic, and often frustrating, proposal and review process, which focuses primarily on compliance with intermediate process steps (see Box 3-7). This process probably discourages innovation and experimentation at the state and local levels; overtaxes the limited financial and human resources available to the nation’s AQM system at the state, local, and federal levels; and draws attention and resources away from the more germane issue of ensuring progress toward the goal of meeting the NAAQS.
Overemphasis on Attainment Demonstrations
An attainment-demonstration SIP is statutorily required to demonstrate through air quality model simulations and other weight-of-evidence analyses that the relevant nonattainment area will reach attainment by a certain date as a result of the specific pollution-control measures proposed in the SIP. Such an exercise provides policy-makers with critical information: estimates of the magnitude of emission reductions that will be needed to reach attainment and assessment of the efficacy of various options that could be adopted. That information is essential to the development of an implementation plan and should be retained in the AQM plan.
BOX 3-7
|
However, the use of the attainment demonstration as a one-time robust prediction of how air quality in a given area will evolve over a multiple-year to a decadal time scale does not take into account the significant modeling, socioeconomic, and control-technology uncertainties implicit in such a process, and thus improperly applies the scientific and technical tools used in the demonstration (see, for example, NARSTO 2000). Moreover, the attainment demonstration can provide a false sense of assurance, which can discourage a review of the underlying assumptions of the plan until attainment has not been achieved after the prescribed time, such as 5 years or more. Finally, although mid-course reviews of SIPs do occur and although it should be possible to amend a SIP as new information and updated modeling simulations become available, in practice this task is very difficult, because the CAA requires that any such changes be subjected to the full and complex review and approval process used for the original SIP. In Chapter 7, the committee recommends implementation of a more iterative process, which retains the attainment demonstration but places greater emphasis on tracking and measuring progress and performance.
Single-Pollutant Focus of SIPs
Air pollutants occur in complex mixtures, and yet SIPs are constrained to address only individual criteria pollutants. As a result, the entire, relatively cumbersome SIP process must be undertaken for a pollutant such as O3 and then again for PM in a separate process and on a different timetable, despite the fact that the exposures are simultaneous, the sources are often the same, and the two pollutants share many common chemical precursors. One result of this separation is that facilities and other emitters of air pollution may be faced with multiple requirements over time to deal with similar pollutants. This process is made more problematic by the inability to consider key HAPs at the same time unless they are VOCs and therefore also precursors of the other pollutants. Some of the pollutants that states have sought to control in the past had limited sources and could be addressed individually (for example, Pb and CO). However, the major air pollution challenges today, which involve multiple emissions from common mobile and stationary sources, can be more effectively addressed using a multipollutant approach. Such an approach can simultaneously seek reductions of pollutants posing the most significant risks. It can also focus on achieving the most cost-effective mix of emission reductions of key pollutants from any one source rather than asking that source to separately address reductions of different pollutants at different times in response to different SIPs.
Barriers to Addressing Multistate Airshed Pollution
Over the past two decades, the AQM system has had to grapple increasingly with air pollution phenomena that extend over multistate airsheds—phenomena whose effective control requires coordination across state boundaries and participation by states that may contribute to serious air quality problems but not experience them. For example, for acid rain, Congress chose to prescribe a national rule; for regional haze, Congress attempted to provide EPA with the authority to develop multistate strategies; and for O3, OTC (mandated by the CAA) and OTAG (developed by EPA and the states) were formed to develop suitable multistate strategies.
The current form of the CAA does not provide EPA and states a clear mandate and procedure for regularly analyzing and identifying when and how to implement multistate efforts. With the exception of the OTC, the multistate regulatory approach for O3 and regional haze has been linked to the traditional SIP, requiring that a set of multistate controls be identified first and then incorporated voluntarily into the SIPs of all the states in the given region. This indirect and controversial process has been time consuming and fraught with legal uncertainties. The SIPs of the future will require a more effective mechanism for identifying and linking multistate control strategies with local measures. Of particular importance will be the need for mechanisms to induce states upwind of emission sources to take actions that have little direct benefits for them but that are needed for successful attainment of the NAAQS in states downwind of the sources.
SUMMARY
Strengths of the SIP Process
-
The SIP process provides a reasonable mechanism for state and local agencies to take into account emission controls adopted at the federal and multistate levels and then to choose a suitable suite of additional local emission-control measures to attain the NAAQS.
-
The existence of federally mandated emission-control measures has eased the burden of state and local authorities in developing attainment SIPS.
-
The requirement for emission inventories in SIPs has facilitated the development of a uniform methodology for quantifying pollutant emissions in the United States.
-
The requirement for modeling analysis in SIPs has promoted the development of increasingly sophisticated air quality models that link pollutant emissions to pollutant concentrations in the atmosphere.
-
The SIP process has resulted in a general decrease in criteria pollutant concentrations in the United States and, in some areas, has resulted in NAAQS attainment.
-
The sequencing of attainment dates for O3, based on nonattainment classification, provides a more reasonable and flexible timetable for state and local agencies to come to address this persistent air pollution problem.
Limitations of the SIP Process11
-
Implementation of federal, regional, and local control measures through the SIP process has not resulted in attainment for O3 and PM in many areas in the United States.
-
The SIP process has become overly bureaucratic and draws attention and resources away from the more germane issues of tracking progress and assessing performance.
-
The attainment-demonstration SIP places too much emphasis on uncertain emissions-based modeling simulations of future air pollution episodes.
-
SIPs must be developed individually for each criteria pollutant, making it difficult for states and local agencies to consider potentially more cost-effective and more protective multipollutant strategies.
-
The SIP process lacks sufficient mechanisms and governmental infrastructure for addressing multistate airshed aspects of air pollution.
11 |
Recommendations are provided in Chapter 7. |