6
Research Approaches to Furthering Understanding
Previous chapters have covered the current understanding of radiative forcings, how the forcings have varied over Earth’s history, different ways to quantify forcings, and critical uncertainties involved in predicting future forcings. This review of current understanding has illustrated that significant knowledge of forcings—including knowledge of their sources, magnitudes, variations, and effects on climate—has been achieved over the past decades and that there are still many critical unknowns. In this chapter, the many research approaches for studying forcings are described. These include observations from multiple platforms (e.g., surface observing networks, satellite-based remote sensing instruments), laboratory and process studies, atmospheric reanalysis and data assimilation, tools to relate emissions to atmospheric concentrations, “proxy” observations of past forcings and response, and a variety of climate modeling approaches.
OBSERVATIONS OF RADIATIVE FORCING AND RESPONSE
Robust observations of radiative forcings are critical for improving understanding of these climate drivers, how they varied in the past, and how they might change in the future. Current observational approaches include in situ and surface-based monitoring of greenhouse gases and aerosols; satellite-based observations of atmospheric composition, land cover, and solar variability; and intensive campaigns that utilize aircraft-based observations with in situ and satellite measurements to study processes in detail. Observations of climate response, such as surface temperature or ocean heat content, also provide important information about climate
forcings. Much of the current understanding of radiative forcing and other forcing concepts has been obtained from climate models. To improve this understanding, routine observations of climate forcings will be essential, both as a record of change in the climate system and as a critical constraint for climate models.
Long-Lived Greenhouse Gases
The major long-lived greenhouse gases (carbon dioxide [CO2], methane [CH4], nitrous oxide [N2O], and halocarbons) are all extensively observed by surface networks such as the National Oceanic and Atmospheric Administration (NOAA) Climate Monitoring and Diagnostics Laboratory (CMDL) and the Atmospheric Lifetime Experiment (ALE)/Global Atmospheric Gases Experiment (GAGE). All have sufficiently long lifetimes to be well mixed in the atmosphere. Their spectroscopy is also well established. Radiative forcings can thus be assessed with confidence.
There is, however, a strong impetus to improve the observational system for these gases in order to constrain inverse model analyses of their regional budgets. For example, many analyses have used the large-scale gradients of CO2 measured from the surface networks to constrain the global carbon budget and quantify the terrestrial sink at northern midlatitudes. However, they have not been successful in determining the longitudinal distribution of the carbon sink among the three northern mid-latitude continents. The International Geosphere-Biosphere Programme (IGBP) TransCom activity (http://transcom.colostate.edu/) has provided a forum for standardizing and comparing these inverse model analyses, but model transport errors ultimately limit their ability to exploit the relatively sparse surface air observations in terms of regionally resolved source and sink constraints (Gurney et al., 2002).
Better understanding of terrestrial uptake is critically needed for future projections of CO2 concentrations (IPCC, 2001). An extensive network of CO2 flux measurement towers has been deployed worldwide in recent years and is coordinated through the FLUXNET activity (Baldocchi et al., 2001). It includes in particular the AmeriFlux network in North America (http://public.ornl.gov/ameriflux/). These measurements provide direct observations of the terrestrial component of the carbon budget and also the biogeochemical constraints needed to interpret these observations. However, it has not been clear how to integrate them into large-scale inverse model analyses. The North American Carbon Program (NACP) outlines a strategy for doing so, involving in particular the use of aircraft observations to scale up the tower flux observations and providing a linkage to the global observation network (Wofsy and Harriss, 2002; Denning et al., 2003).
Global mapping of CO2 concentrations from space would greatly im-
prove our ability to constrain carbon sources and sinks in inverse models. It would pave the way for construction of national carbon budgets, providing important input for global environmental agreements aimed at mitigating climate change. The challenge is to deliver a measurement with sufficiently high precision to be useful for inverse modeling. A precision of 0.3 ppmv (parts per million by volume) is thought to be necessary (Pak and Prather, 2001; Rayner and O’Brien, 2001). The Orbiting Carbon Observatory (OCO) satellite instrument, planned for launch in 2007, is expected to provide this precision (Crisp et al., 2004). It will measure CO2 column mixing ratios with kilometer-scale spatial resolution by solar backscatter in the 1.58 μm band, with measurements in additional bands to correct for aerosol and surface pressure effects. Simulations with chemical transport models sampled along the OCO orbit track suggest that the measurements should be of great value for constraining carbon fluxes down to a regional scale (Crisp et al., 2004).
Methane concentrations have increased by a factor of 2.5 since the eighteenth century, but the rate of growth began to slow in the 1980s and was close to zero in 1999-2002 (Dlugokencky et al., 2003). The reason for this slowdown is not clear. Changes in agricultural practices, decreased natural gas production in Russia, and increasing OH concentrations (reducing the lifetime of methane) may all have contributed (Khalil and Shearer, 1993; Dentener et al., 2003; Wang et al., 2004). A number of inverse model studies have been conducted to constrain sources of methane using long-term observations from the NOAA CMDL network (Hein et al., 1997; Houweling et al., 1999; Wang et al., 2004), but they do not yield consistent results. Aircraft observations in continental outflow over the northwest Pacific have been used recently to constrain Eurasian sources of methane (Xiao et al., 2004) and halocarbons (Palmer et al., 2003). Satellite measurements of methane and halocarbons have so far been restricted to the stratosphere. There has been great interest in using solar backscatter measurements to constrain the column mixing ratio of methane (Edwards et al., 1999), but efforts so far have been unsuccessful. Similar to CO2, satellite observations of methane with sufficiently high resolution would increase considerably our ability to constrain regional sources.
Ozone
Ozone has a lifetime ranging from days to months in the troposphere and up to years in the lower stratosphere. Its distribution in the atmosphere is thus highly variable, in contrast to the long-lived greenhouse gases. Vertical profiles from ozonesondes provide at present the best characterization of the global distribution of ozone. Their coverage is extensive in the extratropical Northern Hemisphere but relatively sparse in the tropics and the
Southern Hemisphere. Relatively low sampling frequencies (typically weekly) and calibration issues have made it difficult to use these observations to quantify long-term trends of ozone and its vertical distribution, in both the troposphere and the stratosphere (Logan, 1999). This uncertainty in ozone trends and our ability to describe them in models is the main difficulty in quantifying the radiative forcing of ozone in the past and making projections for the future.
A global climatology of total ozone columns extending back to 1979 is available from the Total Ozone Mapping Spectrometer (TOMS; see Figure 6-1) and other sensors, and has been used extensively and successfully for trend analyses (WMO, 2003). A similarly long, although sparser, record is available for the vertical distribution of ozone down to the lower stratosphere from the Stratospheric Aerosol and Gas Experiment (SAGE) and
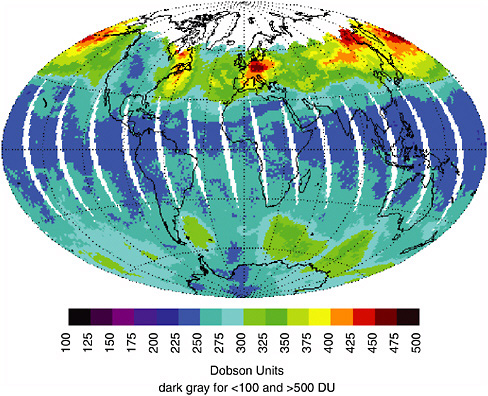
FIGURE 6-1 Total column ozone observed on January 25, 2005, by the Total Ozone Mapping Spectrometer (TOMS) aboard the Earth Probe satellite. SOURCE: NASA Goddard Space Flight Center.
other sensors. Most problematic are the tropopause region and the troposphere, which are of most interest from a radiative forcing standpoint. Despite these limitations, for this short-lived forcing, unlike for other such species, chemical transport models are not needed to evaluate the forcing because of the presence of a reliable, continuous global monitoring network.
The inadequacy of current tropospheric ozone observations for constraining global distributions and trends has spurred the concept of an Integrated Global Atmospheric Chemistry Observation System (IGACO) to integrate and expand the current observational network (Barrie et al., 2004). Satellite observations have to play a key role in mapping the global distribution. There is at present no direct measurement of tropospheric ozone from space. A number of attempts have been made to constrain tropospheric ozone columns from a combination of independent measurements of the total column and the stratospheric contribution, starting from the pioneering work of Fishman et al. (1990), but there are large uncertainties with these products even at equatorial latitudes where they are most robust (Martin et al., 2002). Some attempts have been made to infer tropospheric ozone columns from solar backscatter measurements, but the results so far are only qualitative. The Tropospheric Emission Spectrometer (TES), launched on the Aura satellite in July 2004, will provide the first opportunity for global mapping of tropospheric ozone from space. It will observe infrared emission of ozone in the nadir and in the limb with line-by-line resolution (Beer et al., 2001). Algorithm development studies suggest that it should provide one to two constraints on the vertical profile in the troposphere with sufficient precision to allow global mapping (Clough et al., 1995; Bowman et al., 2002).
Aerosols
Observational approaches to better understand aerosol radiative forcing include closure studies, remote sensing from space-based and other platforms, Lagrangian studies, and surface-based observations, which are described in more detail below. Until recently, models were needed to infer the direct forcing. However, recent field campaigns, including the Indian Ocean Experiment (INDOEX) and the Aerosol Characterization Experiment in Asia (ACE-Asia), have obtained the direct forcing from radiation budget observations at the surface and the top of the atmosphere (TOA; Figure 6-2). Regarding direct radiative forcing by tropospheric aerosols, there are several tests that have been and should continue to be performed between models and existing observations. These include comprehensive comparisons against surface concentration measurements, aerosol optical depth measurements (e.g., AERONET), reflected radiation flux at TOA
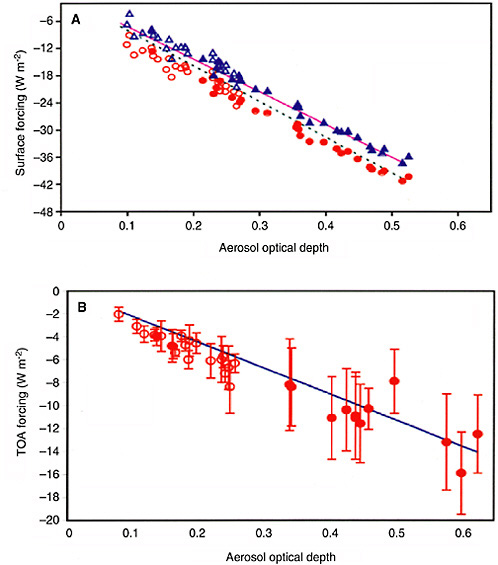
FIGURE 6-2 Direct observations of clear-sky forcing efficiency. The top panel shows the reduction at the surface due to aerosols as a function of the aerosol optical depth, while the bottom panel shows the same at the top of the atmosphere. Surface measurements were obtained with broadband pyranometers and spectral radiometers, while the TOA measurements were obtained from Clouds and the Earth’s Radiant Energy System (CERES) radiation budget instruments on board the Tropical Rainfall Measuring Mission (TRMM) satellite. The values are diurnal mean values for clear skies and the data are for the Arabian Sea. The figure shows that the surface forcing is three times the TOA values, both being negative. The forcing includes both natural and anthropogenic aerosols, and the measured single scattering albedo is about 0.9 (±0.03). SOURCE: Satheesh and Ramanathan (2000).
(e.g., Earth Radiation Budget Experiment [ERBE] clear-sky measurements over oceans), radiation measurements at the surface (e.g., Baseline Surface Radiation Network [BSRN]), and vertical profiles where available. Long-term monitoring is essential to understand interannual variations in forcing by short-lived species. Finally, extracting the indirect effect from observations, particularly those based on regional and global datasets, may require one to deal with the response of cloud systems to the thermodynamic environments that are tied to the polluting particles (Harshvardhan et al., 2002).
Closure Studies
Closure experiments provide constraints on aerosol radiative properties. In a closure experiment, an aerosol property is measured in one or more ways and then calculated from a model based on independently measured data (Quinn and Coffman, 1998). The objective is to evaluate models using a collection of independent observed quantities to provide multiple constraints on the aerosol properties being analyzed. Closure studies of aerosol direct and indirect effects typically use multiple measurements in a single atmospheric column at one moment in time to constrain the radiative forcing. The comparison between the calculated and measured values provides a test for the reliability of the measurements and the model.
Successful closure experiments have been conducted in a number of field campaigns including ACE-1 and ACE-2, the Tropospheric Aerosol Radiative Forcing Observational Experiment (TARFOX), INDOEX, and ACE-Asia. For example, ACE-1 and ACE-2 provided detailed aerosol characterization that showed good agreement between modeled and observed optical depth (Quinn and Coffman, 1998; Collins et al., 2000; Redemann et al., 2000; Russell et al., 2000; Fridlind and Jacobson, 2003; Wang et al., 2003). Closure experiments were conducted using a collection of vertically resolved measurements of aerosol size and composition with simultaneous vertical profiles of spectrally resolved optical depth. Light scattering and one-dimensional radiative transfer calculations were then used to calculate the optical depth profile, and these calculated values were compared with the aerosol size and composition-based calculations of optical depth.
Other closure experiments have provided important constraints on the direct effect of aerosols on radiation. Collins et al. (2000) thus determined that multiple aerosol layers in the atmosphere are significant in scattering light in the troposphere, showing a good correspondence between measured aerosol concentrations and measured scattering. It appears from these and other aircraft-based closure experiments that aerosol forcing is well understood when the column loading and the distribution of aerosol size and composition have been characterized.
Integrated Approaches for Obtaining Aerosol Forcing from Observations
Since 1999 there have been several successful efforts to obtain aerosol radiative forcing information from surface, aircraft, and satellite observations (e.g., Figure 6.2). The success of these studies clearly illustrates the need for accurate observations of radiation budget, aerosol optical depth, and cloud fraction and cloud type at the surface (in selected regions) and from space. In situ aerosol chemical data from aircraft have been used to separate anthropogenic from natural forcing. Surface-based aerosol column optical measurements have been combined with Moderate Resolution Imaging Spectroradiometer (MODIS) data for clear skies to obtain aerosol forcing at the top of the atmosphere (Kaufman et al., 2002). These clear-sky forcing values provide an important constraint on the closure approaches described earlier and on climate model simulations of direct aerosol forcing. Integrated approaches have also been very effective in capturing the effect of aerosols in nucleating more cloud drops and in suppressing precipitation efficiency. For example, the spectral dependence of cloud reflectivity measured from space has been used to obtain the effective radius of clouds (e.g., Coakley et al., 1987; Nakajima et al., 2001). Comparisons of the effective radius between pristine and polluted clouds have provided estimates of the global indirect effect, although additional work is needed to improve the accuracy of these estimates. In situ aircraft observations have been used to characterize the dependence of cloud drop number density and effective radius on aerosol number concentration and cloud condensation nuclei (CCN) for low clouds (Taylor and McHaffie, 1994; Gultepe et al., 1996; Pawlowska and Brenguier, 2000; McFarquhar and Heymsfield, 2001) and high clouds (Sherwood, 2002). Satellite data for aerosol optical depth and cloud fraction have been used to infer the semidirect effect (Koren et al., 2004; Kaufman and Fraser, 1997). Major new insights into the role of anthropogenic aerosols in reducing precipitation efficiency have been obtained by combining satellite data for effective cloud drop size, precipitation rate (using microwave radiometer and radar), and aircraft data (Rosenfeld, 1999, 2000).
To exploit the new generation of satellite data for clouds and aerosols (e.g., the National Aeronautics and Space Administration [NASA] A-Train), in situ aerosol-cloud observatories are needed in different regions of the planet (preferably the regions contributing most to anthropogenic aerosol forcing). This combination of satellite and in situ data will enable us to address fundamental issues, including the global distribution of black carbon; regional statistics of aerosol number concentration, composition, CCN, and cloud drop distribution; and global distribution of aerosol forcing at the surface and the TOA.
Lagrangian Studies of the Indirect Effect
The critical issue in field studies addressing the indirect aerosol effect is that simultaneous measurements of the aerosol entering the cloud and of the cloud microphysical characteristics are needed. A Lagrangian sampling strategy is essential. This approach was tried during ACE-2 with limited success due to the complexity of regional boundary layer dynamics that resulted in particularly complex clouds and decoupled mixed layers (Johnson et al., 2000; Sollazzo et al., 2000). Ship tracks have provided an opportunity for Lagrangian sampling and yielded evidence that even hydrophobic organic compounds may be incorporated in cloud droplets (Russell et al., 2000).
Surface-Based Observations
Surface sites and ships provide platforms for long-term continuous measurements. Ground-based experiments have studied the role of cloud-particle interactions through fog events and showed that chemical composition is a key factor in determining cloud droplet activation properties (Noone et al., 1992). Recent studies have shown evidence consistent with activation of organic particles (Facchini et al., 2000; Decesari et al., 2001; Ming and Russell, 2004). Additional long-term datasets at surface sites may provide statistically significant constraints on direct and indirect aerosol effects. To be most useful, these sites should be coordinated with local meteorological and air quality observations and should enforce strict protocols for accuracy and cross-site calibrations.
Land-Use and Land-Cover Change
The mechanisms involved in land-atmosphere interactions are not well understood, let alone represented in climate models. A synergistic approach combining state-of-the-art models, field observations, and satellite imagery will be needed to advance our knowledge. Surface properties such as albedo, fractional vegetation coverage, emissivity, soil type, functional plant type, snow cover, and permafrost are examples of land-surface data that are needed. At the microscale, the use of very-high-resolution large-eddy simulations and micrometeorological observations from towers and low-flying, slow aircraft can elucidate some of the fundamental processes affecting the land-surface radiation balance through its interaction with turbulence and heat and momentum fluxes. Ever-increasing computing power now readily available allows very-high-resolution simulations with large-eddy simulations, including flow inside tree canopies.
At the mesoscale, land-cover heterogeneity triggers atmospheric circu-
lations that enhance the heat and momentum fluxes in the atmospheric boundary layer and seem to increase the production of clouds. These circulations and the resulting cloud types and depths are sensitive to meteorological conditions and also depend on aerosol concentrations and size distribution. Satellite images have been key to identifying these types of clouds (Rabin and Martin, 1995). Yet there is still debate about the frequency of occurrence and intensity of clouds and precipitation resulting from such circulations, and their impact on the radiation balance (Weaver and Avissar, 2001; Doran and Zhong, 2002). Field campaigns at the mesoscale, which could be used to study these processes in more detail, are costly and complicated to perform. An integrated approach is needed involving a combination of satellite, aircraft, and tower observations. At the global scale, satellite imagery and models become even more important because in situ observations from ground stations and soundings are extremely limited. The challenge in modeling land-atmosphere interactions at that scale consists of including physical, chemical, and biological processes that occur at the microscale but propagate to the global scale through teleconnections. For example, global models have shown that the intensification of thunder-storm activity resulting from deforestation in Amazonia can affect precipitation in the U.S. Midwest. To capture these phenomena globally and with more accuracy, it is necessary to represent the global atmosphere at a very high resolution, which remains a challenge, even with the computing power available now. Appropriate parameterizations remain to be developed. Better datasets from more accurate and more frequent satellite observations are essential for the initialization and evaluation of global models.
Satellite MODIS data are promising in this regard because they can be used to globally monitor the land surface and its changes, seasonally and over longer time periods (Figure 6-3). The scientific value of MODIS is discussed in Running et al. (2004), Townshend and Justice (2002), and Schaaf et al. (2002). This instrumentation can be related to the longer-term measurements from the Advanced Very High Resolution Radiometer (AVHRR) and Landsat satellites as a monitor of land-use change and vegetation dynamics across several decades. Other satellite platforms to monitor land cover are reported in Bartalev et al. (2004) and include visible, infrared, and microwave wavelength sampling.
Field campaigns provide a complementary method to advance understanding of land surface processes. Campaigns such as BOREAS (for the boreal forest region of Manitoba and Saskatchewan), FIFE (for a 15 km by 15 km area in east-central Kansas), and others are summarized in Kabat et al. (2004). Such regionally specific programs permit the ground truthing of satellite data and provide higher spatial and temporal resolution.
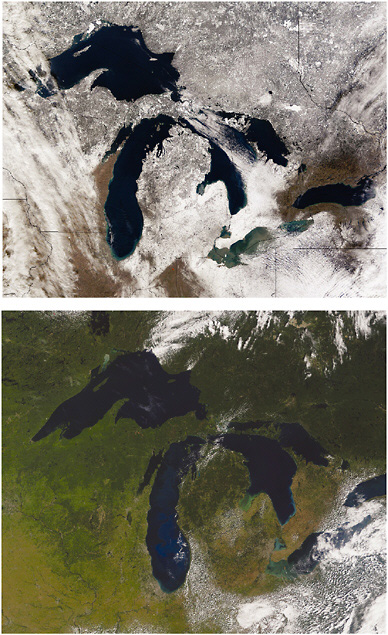
FIGURE 6-3 Observations of the land cover in the Great Lakes region of the United States and Canada, obtained by the Moderate Resolution Imaging Spectroradiometer (MODIS) instrument aboard the Terra satellite. The top image was acquired on December 26, 2003, and the bottom image was acquired on September 16, 2002. SOURCE: NASA.
Solar
Space-based solar monitoring over the past 25 years covers more than two solar activity cycles and has established, unequivocally, the variability of the Sun’s brightness at all wavelengths. Irradiance observations during the indefinite future are essential to quantify solar radiative forcing. Observations over only 2.5 cycles are insufficient to characterize the extremes of solar cycle irradiance variability or to detect speculated longer-term irradiance changes.
The extant record of total solar irradiance is compiled from observations made by half a dozen individual radiometers, cross-calibrated to account for individual absolute uncertainties and instabilities. Measuring solar irradiance with sufficient accuracy and repeatability to record true variability is a challenging radiometric task. The measurements must be made from absolute, electrically self-calibrating radiometers on space-based platforms. Significant drifts in instrument sensitivity can arise from changes in the space environment (solar exposure, thermal drifts, spacecraft pointing, power instabilities) and in optical and electrical components. These instabilities must be carefully removed from the radiometer signals.
Future observations of solar radiative forcing must address these challenges. Continuous, overlapping observations by multiple space-based radiometers are required. The overlap must be sufficiently long (of order one year or more) to provide the radiometric cross-calibration able to sustain the required long-term measurement accuracy. The cross-calibration must account for both the overall absolute level (typically traceable to uncertainties in the area of the primary aperture) and differences in temporal behavior (arising from instrumental drifts from many sources, but especially from solar exposure). The reliance of solar forcing observations on overlapping measurements will be alleviated only when absolute uncertainties are reduced by more than an order of magnitude relative to existing capability. Only then will benchmark measurements traceable to absolute standards be possible. Current expectations are that a new generation of phase-sensitive detection radiometers, the first of which is currently flying on the Solar Radiation and Climate Experiment (SORCE) mission, can achieve absolute accuracies of 0.01 percent. But this will have to be demonstrated by careful measurement validation and interpretation since the most recent SORCE measurements differ by 4 W m−2 (0.3 percent) from the historical database. This significant discrepancy motivates detailed scrutiny of all past and current radiometric observations so as to better identify and quantify sources of uncertainty in future measurements.
Future measurements of solar radiative forcing require not only measurements of total (spectrally integrated) irradiance but also simultaneous and self-consistent characterization of the spectrum of irradiance variabil-
ity, which is strongly wavelength dependent. This is necessary because the multiple processes involved in solar radiative forcing are strongly wavelength dependent. SORCE observations capable of monitoring solar spectral irradiance from 0.2 to 2 μm have now begun. This continues the database of ultraviolet (UV) irradiance acquired by the Upper Atmosphere Research Satellite (UARS) since mid-1991 and commences a new, high-precision database of spectrally resolved irradiance observations, 25 years after the initiation of the total solar irradiance record.
The immediate challenge is to secure observations of both total and spectral irradiance that can connect the current measurements from SORCE (launched in 2002) with the eventual operational monitoring by the National Polar-orbiting Operational Environmental Satellite System (NPOESS). Current plans identify only total solar irradiance measurements during the intervening period. Once NPOESS has commenced observing solar radiative forcing, the challenge will be to obtain the necessary overlap of successive instruments. Current plans for the operation of the NPOESS spacecraft do not specify the needed (or any) overlap so that the long-term record may be jeopardized. A further issue is the probable lack of multiple observations of solar irradiance. Much has been learned in the past 25 years from comparison of independent radiometers at different stages of instrumental aging (and solar exposure). Multiple observations also provide crucial insurance against losing the entire long-term forcing record
Ocean Heat Content
Measurement of the ocean heat content provides an integrated method to monitor the radiative imbalance (Piexoto and Oort, 1992). This is because the ocean is the dominant heat storage location in the climate system (Levitus et al., 2000, 2001). The Argo network of ocean floats and satellite observations of ocean altimetry have been used to estimate trends in ocean heat content (Levitus et al., 2000, 2001; Willis et al., 2003). There is a need to better assess the frequency and the spatial coverage of ocean heat changes that are required to accurately determine the radiative imbalance on the order of tenths of a watt per square meter (e.g., Levitus et al., 2000, 2001). In addition, the data have to be evaluated in the context of radiative imbalance since 1995.
One possible approach for improving knowledge of past changes in oceanic heat content is to use ocean general circulation models (GCMs) to interpolate missing subsurface information in past decades from the increasingly sparse ocean measurements available back in time. Such ocean reanalysis projects are currently in their infancy (e.g., Carton et al., 2000). Another approach involves the use of sea level measurements to infer past changes in oceanic heat content. Such measurements are available both
from modern satellite altimetry measurements, such as TOPEX/Poseidon data, that span roughly the past decade and sparser but longer-term tide gauge networks (Nerem and Mitchum, 2001). In order to infer ocean heat content changes from sea level estimates, however, one must make potentially restrictive assumptions regarding the thermal contributions to ocean heat content changes versus contributions from changes in continental run-off and glacial melting.
Metrology
Radiative forcings on climate have some overarching characteristics. They occur on intermediate to long timescales (longer than annual) that exceed the typical duration of the programs and systems that monitor them, and they involve the absorption, scattering, emission, and redistribution of electromagnetic radiation in the form of photons with a wide range of wavelengths and fluxes.
Because climate timescales are long, observations of radiative forcings and effects must be planned and maintained for the indefinite future. To secure real long-term changes and trends, the relevant geophysical quantities must be determined with a level of uncertainty (accuracy) that is significantly smaller than the expected changes. Thus far, the approach to achieve this relies on overlapping successive measurements to cross-calibrate their absolute uncertainties, which typically exceed the expected change. For example, total solar irradiance varies by about 0.1 percent from the minimum to the maximum of the 11-year solar cycle, but individual radiometers have absolute uncertainties of only 0.2 percent. A composite irradiance record is thus possible only by cross-calibrating individual radiometers (Fröhlich and Lean, 2002), taking into account as well the effect of sensitivity drifts and environmental (e.g., space platform) influences on their long-term repeatabilities (also called long-term precision or relative accuracy). This situation is equally true for the tropospheric temperature record constructed from observations made by Microwave Sounding Units (NRC, 2000). Questions remaining about the reliability of these long-term composite records undermine the certainty with which the parameters are known.
A secure long-term database of radiative forcings and effects requires that the accuracies of the geophysical parameters ultimately be tied to irrefutable absolute standards that are tested and validated in perpetuity for uncertainty and repeatability. Also essential is a requirement that total forcing be documented along with the forcing due to individual components. Anderson et al. (2003a) point out that the uncertainty in net forcing is much greater than the forcing due to an individual forcing agent. Benchmark measurements of radiative forcings and climate parameters are needed
immediately to provide records of absolute values for all time of a number of carefully selected observables that define climate forcings and climate responses. Since radiative forcings and climate responses are highly wavelength dependent, high spectral resolution is needed to isolate the spectral signatures of the relevant processes and components. This produces additional challenges since accuracy and calibration difficulty increases as spectral resolution increases (stray light, instrument profile function, wavelength calibration, signal to noise, matching to available standards). Key parameters for which benchmark measurements are crucial include among others sea level altimetry, solar irradiance, global positioning system (GPS) index of refraction, ozone and CO2 concentrations, and spectrally resolved, absolute radiance to space.
Establishing and validating the accuracy and precision of a geophysical quantity involves tracking instrument calibrations from the laboratory to deployment and throughout mission lifetimes to reduce systematic errors on orbit. This requires considerable additional effort and commitment to an experimental strategy designed to reveal systematic errors and drifts through independent cross checks, open inspection, and continuous interrogation. It involves simultaneous observations of related and similar quantities using both similar and differing radiometric techniques. Regular calibrations are needed, for example, using the Sun, Moon, known land scenes, or on-orbit sources or detectors. Since the forcings and responses that determine any one particular climate state involve a distribution about a mean, the ensemble must be properly characterized and quantified so that changes in the mean can be identified reliably. Ultimately, the specification of the forcings and responses must be integrated to test climate forecast models.
Achieving radiometric accuracy and traceability requires new programs and techniques to advance the current state of metrology and transfer these advances to the determination of radiative climate forcings and effects. This has motivated an alliance of NPOESS and the National Institute of Standards and Technology (NIST), but there is a need to accord this a high priority with sufficient time and funds. It is unlikely that many of the climate variables measured by NPOESS will be directly traceable to an absolute standard. A recent NIST workshop (Ohring et al., 2004) to address this challenge notes that “measuring the small changes associated with long-term global climate change from space is a daunting task. Satellite instruments must be capable of observing atmospheric temperature trends as small as 0.1°C per decade, ozone changes of as little as 1 percent per decade and variations in the Sun’s output as tiny as 0.1 percent per decade.” NIST is developing new facilities to meet the metrology challenges of future climate-related observations. Of particular relevance is the Spectral Irradiance and Radiance Calibrations with Uniform Sources (SIRCUS),
which has the ability to characterize and calibrate radiometric instruments with spectrally pure sources of differing flux levels over a wide range of wavelengths (ultraviolet to infrared), with detectors tied to cryogenic cavity radiometers (absolute accuracy 0.001 percent for power measurement) and apertures measured at NIST.
LABORATORY AND PROCESS STUDIES FOR AEROSOLS
Key uncertainties in the composition and properties of aerosol particles and their role in clouds require measurements and models under controlled conditions. Laboratory and process studies are needed to resolve these questions by four types of measurements. First, measurement of the composition and thermodynamic properties of organic and inorganic components, together with development of intelligent parameterizations of these properties, is necessary to describe the properties of individual particles. Second, measurements of spectrally resolved imaginary refractive indices are needed to determine absorbing properties. The third type of measurement characterizes the morphology and reactivity of particle surfaces. Finally, surface tension and wettability of organic particles must be measured in order to predict cloud droplet activation properties.
The thermodynamic properties of organic and some mineral components are not well understood. Most particle types show a strong hysteresis effect between the relative humidity for deliquescence (conversion from solid to liquid) and the relative humidity for efflorescence (conversion from liquid to solid). This hysteresis effect is not well characterized in the laboratory or in the field, yet it plays a critical role in particle optical properties. The partitioning of semivolatile organic compounds between the gas and the aerosol phase also needs to be better determined as a function of temperature and aerosol phase composition.
The optical properties of organic compounds present in aerosols are poorly known. Much of the existing information is limited to the UV and driven by the needs of the polymer industry. Very little information exists for spectrally resolved imaginary refractive indices in the visible spectrum. Because these measurements are relatively routine but very time-consuming, there has been little interest in the research community in collecting the required database of optical properties.
The third type of measurement is the reactivity of ambient particles, given their shapes and structures. Such reactivity may include probabilities of inorganic and organic reactions that affect particle lifetime, distribution, and optical behavior. Porosity and surface area strongly determine the rates and yields of heterogeneous chemical reactions, yet very little is known about these characteristics for ambient particles.
A fourth property of chemical mixtures that is not well understood for
pure or mixed organic or inorganic particles is their surface structure and wetting behavior. Surface tension plays an important role in the indirect effect of aerosol particles, potentially providing an important determining factor for the particles to activate. Organic compounds in particles may significantly alter the efficiency with which particles can serve as cloud condensation nuclei (Facchini et al., 2000; Feingold and Chuang, 2002; Ming and Russell, 2004). The transformation timescale from hydrophobic to hydrophilic states is a seriously uncertain parameter in current models.
ATMOSPHERIC REANALYSIS AND DATA ASSIMILATION
Atmospheric reanalysis involves using models to interpolate observations in order to construct physically consistent estimates of atmospheric structure and dynamics. The National Centers for Environmental Prediction (NCEP) Reanalysis (Kalnay et al., 1996) and the European Centre for Medium-Range Weather Forecasts (ECMWF) Reanalysis (ERA-40) (Bengtsson et al., 2004) are two global analyses that extend across several decades and will continue into the future. Reanalyses can be used to assess the change over time of selected space- and time-integrated climate metrics, such as the 1000-500 mb thickness, the 200 mb heights, tropopause height, and the 200 mb winds (Chase et al., 2000b; Pielke et al., 2001; Santer et al., 2003b).
It remains difficult, however, to estimate reliable, small-amplitude trends from reanalyses (Bengtsson et al., 2004), owing mainly to temporal variations in input data quantity and quality. Given these heterogeneities in reanalyses, it is essential to determine the magnitude of trends that must occur before they can be determined to be statistically significant (Chase et al., 2000b). The use of metrics that integrate atmospheric structure and dynamics represents another effective procedure to utilize reanalyses for trend assessments in that the effect of heterogeneities in the data record may be reduced. Examples include the thickness between pressure surfaces, tropopause height, or the vertical wind shear across the troposphere. The first two provide vertically integrated measures of the warming of the troposphere in response to radiative heating. The third provides an integrated measure of the horizontal gradient in tropospheric mean temperature.
Future reanalyses should strive for as homogeneous a dataset as possible to monitor temporal and spatial changes in tropospheric heat content. This information would be valuable in relating to observed temporal and spatial changes in ocean heat content. For example, can the atmospheric reanalyses help explain the observed focusing of ocean warming in the midlatitudes of the Southern Hemisphere, and will this continue into the future? Accurate reanalyses can also address the question of whether the
difference between surface and tropospheric temperature trends is real or a product of inconsistencies in monitoring.
RELATING CONCENTRATIONS OF GREENHOUSE GASES AND AEROSOLS TO SOURCES
An important step in understanding human and natural impacts on climate is relating what is known about sources of greenhouse gases and aerosols to their observed abundances in the atmosphere. Understanding this link is especially challenging for those atmospheric species that are produced in the atmosphere by chemical reactions of precursor species, have short atmospheric lifetimes, or have a multitude of sources. Two modeling tools—chemical transport models (CTMs) and inverse models—have been developed to assist scientists in relating sources to atmospheric concentrations.
Chemical Transport Model Analyses
Aerosols and ozone have short atmospheric lifetimes and hence inhomogeneous atmospheric distributions. Radiative forcing calculations for these species require global three-dimensional characterization of their concentration fields, the evolution of these concentration fields with time, and correlations with other radiative forcing agents such as clouds and water vapor. This is generally done with CTMs that solve the continuity equation for the species of interest using information on sources, transport, chemical processes, and deposition. CTM simulations provide the basis for the current Intergovernmental Panel on Climate Change (IPCC, 2001) estimates of the radiative forcings from aerosols and tropospheric ozone. They need to be improved in the future by assimilating high-density chemical observations from satellites, using algorithms similar to those presently implemented for meteorological data assimilation. This is already done routinely for stratospheric ozone (Stajner et al., 2001) and should be extended to satellite observations of tropospheric ozone and its precursors (including nitrogen dioxide [NO2], formaldehyde [HCHO], and carbon monoxide [CO]), aerosol optical depths, and aerosol size distributions (Figure 6-4). Eventually, chemical data assimilation and the associated CTM calculations should be done within GCMs and coupled with meteorological data assimilation. This approach will have the advantage of better accounting for correlations with clouds and water vapor. It will also resolve the synoptic-scale coupling of the radiative effects and the meteorological response, as well as coupling interactions between aerosol and cloud processes (Koch et al., 1999; Mickley et al., 1999).
Several elements of stratospheric forcings from changes in ozone and
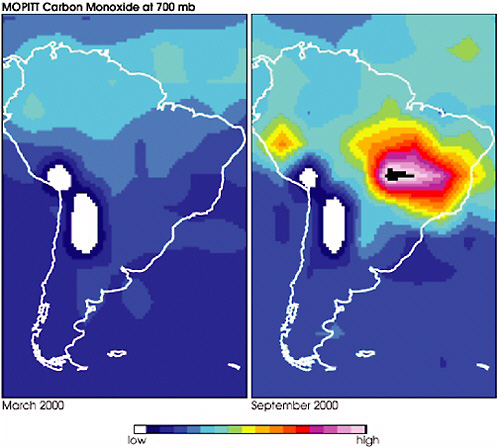
FIGURE 6-4 Carbon monoxide at 700 mb altitude over South America, as observed by the Measurements Of Pollution In The Troposphere (MOPITT) sensor flying aboard NASA’s Terra spacecraft, and assimilated into an atmospheric chemical transport model using wind vectors provided by the National Center for Environmental Prediction (NCEP). Data for producing the image on the left were acquired on March 3, 2000, when fairly low levels of CO were observed, and for the image on the right on September 7, 2000, when a large carbon monoxide plume is seen over Brazil. The generally higher carbon monoxide levels in September are attributed to South American fire emissions and the transport of carbon monoxide across the Atlantic Ocean from Southern Africa fires. SOURCE: NASA Goddard Space Flight Center.
volcanic aerosols are now very well simulated. In the case of stratospheric ozone, the resulting stratospheric cooling is an integral component of the forcing, and the simulated temperature changes match reasonably well with observations (Ramaswamy and Schwarzkopf, 2002; Schwarzkopf and Ramaswamy, 2002; Shine et al., 2003). In the case of volcanic aerosols, models have performed useful comparison exercises (e.g., Pollack et al., 1993). The 1991 Mt. Pinatubo eruption has provided a number of tests against which model simulations can be verified. Stratospheric warming observed after Pinatubo is well simulated by models that employ the detailed spatial-temporal evolution of the particles and incorporate them in a multiwavelength radiative transfer code within a reasonable GCM (Ramaswamy et al., 2004). Indeed, the warming resulting from this eruption, the radiative flux comparisons with satellite observations, the cooling of the troposphere, the change in precipitable water, and the winter warming in northern high latitudes are all at least qualitatively well simulated, attesting to a degree of confidence in the working of climate models (Ramachandran et al., 2000; Ramaswamy et al., 2004; Soden et al., 2002; Stenchikov et al., 2002).
Global CTM simulations of stratospheric and tropospheric ozone are now fairly mature (IPCC, 2001). However, great difficulties remain in the simulation of transport across the tropopause, where ozone has its largest radiative effect. Most CTMs have excessive cross-tropopause transport of air (Tan et al., 2004), at least in part because of noise in the vertical winds induced by the meteorological data assimilation process. In addition, CTMs tend to greatly underestimate the observed trend of tropospheric ozone over the past century (Mickley et al., 2001; Shindell and Faluvegi, 2002) and over the past decades (Fusco and Logan, 2003), suggesting some fundamental difficulty in relating tropospheric ozone concentrations to their sources. Addressing this issue will require focused studies of regional-scale budgets of ozone and its precursors, as well as improved understanding of the natural sources of tropospheric ozone precursors including fires, lightning, and vegetation.
Global CTM studies of aerosols are still in their infancy. Sources of radiatively important aerosol types including organic carbon, elemental carbon, dust, and sea salt are highly uncertain and crudely parameterized. There are relatively good constraints on emissions of sulfur gases, but oxidation to form sulfate aerosols takes place principally in clouds and is thus strongly tied to the simulation of the hydrological cycle (which is highly uncertain). Loss of aerosols occurs mainly by wet deposition, which is subgrid scale for global models and thus has to be parameterized. Better coupling of aerosols with the hydrological cycle is needed; joint data assimilation of aerosol, cloud, and precipitation properties should be pursued in the future. However, assimilation techniques also have fundamental limita-
tions (e.g., lack of knowledge on subgrid scales, inadequate diagnoses of vertical velocities, possible inconsistency between reality and assimilation model physics) that could have a significant impact, especially on the concentrations of short-lived species.
Almost all global CTM studies of aerosols so far have been mass-only simulations that do not resolve the aerosol size distribution, mixing across components, or phase. This is evidently problematic for radiative forcing calculations and, in particular, prevents simulations of the indirect effect except through loose empirical relationships between cloud droplet number concentrations and preexisting aerosol mass concentrations (Boucher and Lohmann, 1995). There is a major computational problem because accounting for aerosol microphysics and allowing for an ensemble of aerosol mixing states rapidly increases the number of prognostic model variables. It appears unlikely that this problem will be solved over the next decade by simple increases in computing resources. Innovative algorithms for simulating aerosol microphysics are needed, such as the method of moments (McGraw, 1997) or new sectional approaches (Adams et al., 2003). Better understanding is also needed of the fundamental processes driving aerosol microphysics, particularly nucleation.
Inverse Models
The standard way for specifying emission inventories in CTMs uses “bottom-up” approaches in which knowledge of the underlying processes, and of the associated emission factors, is parameterized and extrapolated on the basis of globally available socioeconomic or environmental information. The bottom-up approach provides the fundamental tool for ascribing sources to specific emission processes and for making future projections. However, there are often large uncertainties in the emission factors and their extrapolation. One can attempt to reduce this uncertainty with “top-down” constraints on emissions that combine information on observed atmospheric concentrations with CTM-derived relationships between concentrations and sources. Formal inverse models combine these bottom-up and top-down approaches by seeking an optimum solution for the emissions that best accommodates the a priori constraints from bottom-up inventories and information from observations (Kasibhatla et al., 2002).
Global observations from long-term surface-based networks (e.g., NOAA CMDL and ALE/GAGE networks) have been used extensively in inverse model studies of sources for CO2 (e.g., Peylin et al., 2002), CO (e.g. Kasibhatla et al., 2002; Petron et al., 2002), methane (Wang et al., 2004), and halocarbons (Mahowald et al., 1997). Inverse model studies for CO2 have played a key role in quantifying the terrestrial sink of CO2 at northern midlatitudes. Observations from aircraft campaigns and from satellites are
presently increasing the scope and possibilities of inverse methods (Arellano et al., 2004; Palmer et al., 2003; Heald et al., 2004). Variational data assimilation methods are now being developed to improve the detail in the characterization of sources enabled by large observational datasets (e.g., Kaminski et al., 2002). Future inverse model studies should make use of available observations of aerosol surface concentrations and optical depths, as well as the information contained in the observed correlations between species concentrations, for example, between CO2 and CO (Suntharalingam et al., 2004) or methane and ethane (Xiao et al., 2004). These correlations can improve the top-down constraints on the sources and also reduce the errors associated with CTM transport.
CLIMATE FORCING AND RESPONSE OVER EARTH’S HISTORY
A comprehensive database of radiative forcings and effects exists primarily for the past 25 years because many of the relevant observations require space-based observations. Present in this epoch are two major volcanic eruptions (El Chichon and Mt. Pinatubo), a few significant El Niños (1983, 1997), and two solar irradiance cycles. The reconstruction of much longer-term records of forcings and effects is crucial for a broader perspective.
Empirical analyses of correlations between adopted radiative forcing histories and climate reconstructions provide exploratory but limited insights into the relative roles of radiative forcings of climate change in the recent past (e.g., Lean et al., 1995; Mann et al., 1998; Waple et al., 2002). Correlations of various proxies of climate change and radiative forcings during the Holocene suggest the influences of solar variability and orbital motions on a range of climate phenomena including drought (Hodell et al., 2001), rainfall (Neff et al., 2001), and North Atlantic winds and surface hydrography (Bond et al., 2001). Other studies characterize the evolution of variability modes as sources of historical climate change, including the Arctic Oscillation (Noren et al., 2002) and the El Niño/Southern Oscillation (ENSO; Moy et al., 2002). Another type of forcing response investigation is the effect of ice sheet changes during the last glacial maximum (e.g., Manabe and Broccoli, 1985).
Detailed physical insight into the role of past natural radiative forcing requires that documented climate reconstructions be compared with model simulations driven by the actual geophysical forcings. However, some current limitations hamper our ability to draw precise conclusions from such comparisons, even in the recent past. Moderate differences exist, for example, between various alternative reconstructions of past hemispheric temperature trends (e.g., Folland et al., 2001; Jones et al., 2001; Mann et al., 2003; see Jones and Mann, 2004, for a comparison of multiple reconstruc-
tions). A reduction of uncertainties in these reconstructions, along with a resolution of differences among competing estimates, is essential to improve knowledge of the precise history of large-scale mean temperature changes in past centuries, and hence of radiative forcing effects. Such a resolution is likely to come from the availability of increased high-quality proxy reconstructions in key regions, particularly in the data-sparse regions of the tropical oceans and Southern Hemisphere. Improved specification of physical differences and limitations of various temperature proxies (tree rings versus boreholes versus corals) is also needed.
There is a broadly consistent view between different climate models and empirical proxy-based reconstructions of hemispheric mean surface temperature changes in past centuries. The models indicate that greenhouse gases explain the observed 0.6°C global surface warming in the past three decades and that some combination of solar and volcanic forcings is likely responsible for temperature fluctuations of a few tenths of a degree Celsius in the preindustrial period (IPCC, 2001). Model and observational studies suggest that land-cover change may account for some of the surface temperature variation over land (e.g., Kalnay and Cai, 2003; Marshall et al., 2003).
However, there are also significant differences among the model simulations. These differences arise from a number of sources (see Jones and Mann, 2004), including (1) differences in the sensitivities of the models to radiative forcing, which vary by as much as a factor of two; (2) differences in the reconstructed radiative forcings used to drive the model simulations; and (3) differences in the way that radiative forcing estimates are represented in the model. For example, in the case of volcanic aerosols, some models impose a fixed annual mean TOA radiative forcing simply by changing the solar constant (Gonzalez-Rouco et al., 2003), while others (e.g., Shindell et al., 2003, 2004) specify the forcing on a seasonally, latitudinally, and vertically resolved basis. It is clear that improved estimates of past radiative forcing changes and a more organized community-wide effort to perform a controlled set of simulations using common forcing estimates could help to resolve these differences.
Spatial patterns of climate change are difficult to compare between models and observations. The dearth of proxy data over large parts of the oceans in past centuries restricts the spatial detail available in current proxy-based reconstructions (Jones and Mann, 2004). Moreover, at regional spatial scales, the role of internal, unforced variability in the climate (which is intrinsically irreproducible by a forced simulation) is likely to be greater, and observed variations may be dominated by influences from large-scale modes of atmospheric circulation such as the North Atlantic Oscillation (NAO) and ENSO. Although there has been some success in reproducing past reconstructed changes in model simulations, including an NAO-like
response to radiative forcing changes, experiments employing fully coupled land-ocean-atmosphere models to study regional past climate change are just now under way. It is likely that details of stratospheric dynamics and chemistry, ocean circulation, vegetation and soil dynamics, and mechanisms of land-ocean-atmosphere coupling are all important in describing past regional-scale changes in climate. A particular challenge is to quantify the role of radiative forcings (versus other mechanisms) in effecting coherent climate change in widely separated geographical regions, as is evident in paleoclimate proxies on multiple and often abrupt timescales (Rial et al., 2004).
CLIMATE MODELS
Applications of climate models include developing better understanding of processes and predicting future conditions. Compared to simulating the weather, climate modeling faces the challenges of longer timescales, ranging from years to centuries and longer. Climate modeling also requires the accurate simulation of each important component of the climate system, including the atmosphere, oceans, land surface, and continental ice fields, as well as realistic estimates of external forcings (i.e., solar, volcanoes). Physical, biological, and chemical processes taking place in each of these components interact with each other across the spectrum of space and timescales. In simulating future climate, models must take into account how humans will affect emissions of greenhouse gases and aerosols as well as modify land use and land cover. Because future human activities are inherently uncertain, model projections of future climate are typically computed for multiple scenarios of future emissions.
Historical data have been used extensively to evaluate climate models. The Atmospheric Model Intercomparison Project (AMIP) is an excellent example of model validation (Gates et al., 1998) based on archived atmosphere and sea surface data. Such model evaluations need to be extended to encompass the spectrum of important climate forcing effects on such societally important quantities as water resources, agricultural and natural vegetation growth, and air pollution. Can skillful forecasts of changes in these quantities be made as a function of radiative and other climate forcings? These issues are regional in scale, such that validation of model process simulation and forecast skill must be completed at these subglobal scales.
A particular challenge for global climate models is modeling forced climate change over the last few decades. This is the time period with the greatest change in well-mixed greenhouse gases as well as the most complete observational datasets. Some studies have found discrepancies be-
tween the surface and tropospheric surface temperature changes in simulations and observations (Chase et al., 2004), which could be attributed to deficiencies in either models or observations, or a combination (NRC, 2001; Christy and Norris, 2004; Mears et al., 2003; Vinnokov and Grody, 2003, Pielke and Chase, 2004; Fu et al., 2004). Other studies, however, find good agreement between observations and the model-predicted spatial and vertical fingerprints of radiatively forced climate change in recent decades (Allen et al., 2000; Stott et al., 2000; Wigley et al., 2000; Barnett et al., 2001; Santer et al., 2000, 2003a,b; Karoly et al., 2003). Additional evaluations of the ability of models to reproduce regional and global climate in recent decades—including tropospheric temperature, ocean heat content, and other climate variables in addition to surface temperature—should be a major priority for further quantifying model predictive skill. Models should also be encouraged to incorporate forward radiance calculations as model diagnostics to compare with observed radiances.
In order to narrow down the uncertainties associated with radiative forcing effects on climate, models have to be improved in many aspects. Of particular importance is improving the representation of cloud processes, the coupling between the atmosphere and the land surface and ocean, the impacts of regional variability in diabatic heating, and the simulation of regional-scale climate.
Clouds and Microphysics
Uncertainties in relating aerosol to cloud droplet populations seriously limits our ability to quantify the indirect aerosol effects. To treat cloud droplet formation accurately, the aerosol number concentration, its chemical composition, and the vertical velocity on the cloud scale need to be known. Abdul-Razzak and Ghan (2000) developed a parameterization based on the Köhler theory that can describe cloud droplet formation for a multimodal aerosol. This approach has been extended by Nenes and Seinfeld (2003) to include kinetic effects, that is, considering that the largest aerosols do not have time to grow to their equilibrium size. To apply one of these parameterizations, the updraft velocity relevant for cloud formation needs to be known. Some climate models apply a Gaussian distribution or use the turbulent kinetic energy as a surrogate for updraft velocity (Ghan et al., 1997; Lohmann et al., 1999). Others avoid this issue completely and use empirical relationships between aerosol mass and cloud droplet number concentration instead (Menon et al., 2002a). This method is limited because of the scarce observational database. At present, the relationship can only be derived between cloud droplet number and sulfate aerosols, sea salt, and organic carbon; no concurrent data for dust or black carbon and
cloud droplet number are available yet. Therefore, and because of their universality, the physically based approaches described formerly should be used in future studies of aerosol-cloud interactions.
Since the first IPCC assessment, great improvements have been made in the description of cloud microphysics for large-scale clouds. Whereas early studies diagnosed cloud amount based on relative humidity, most models now predict cloud condensate in large-scale clouds. The degree of sophistication varies from just predicting the sum of cloud water and ice (Rasch and Kristjánsson, 1998) to predicting cloud water, cloud ice, snow, and rain as separate species (Fowler et al., 1996). Because the aerosol indirect effect is based on the change in cloud droplet number concentration, some models predict cloud droplet number concentrations using one of the above-described physically based aerosol activation schemes as a source term for cloud droplets (Ghan et al., 1997; Lohmann et al., 1999). There is currently a great discrepancy in models between the sophisticated treatment of cloud microphysics in large-scale clouds and their very rudimentary treatment in convective clouds. Furthermore, there is a mismatch between aerosol activation and cloud formation in most climate models because cloud formation relies on a saturation adjustment scheme whereas aerosol activation relies on a subgrid-scale vertical velocity. Part of this problem will be solved within the next decade when climate models can be run at higher spatial resolution and with smaller time steps.
Including Land Surface Models
Changes in land use pose a nonnegligible climate forcing as well. Climate models are just beginning to include detailed land surface models that are coupled to the simulation of the atmosphere. Also, carbon-cycle feedbacks have been shown to be very important in predicting climate change over the next century (e.g., Schimel et al., 2001; Jones et al., 2003). One important question is whether the terrestrial carbon cycle becomes a net source of carbon dioxide during the next century. To address this issue, vegetation-meteorology-biogeochemical cycle interactions need to be included in climate models.
Diabatic Forcing Heterogeneity
A variety of heterogeneous diabatic forcings have been shown to alter the climate both in the region where this forcing occurs and at large distances through teleconnections. These forcings include land-cover change and vegetation dynamics, soil moisture, ocean color, and aerosols (e.g., Chung and Ramanathan, 2003; Shell et al., 2003; Claussen et al., 2004). On the regional scale, there is general agreement on the importance of these
regional forcings on climate as summarized by Kabat et al. (2004). However, despite the plausible scientific basis as to why teleconnections should be expected and the analog to ENSO events, the global teleconnections associated with these regional forcings are not as widely accepted. The argument against the robustness of the long-range connectivity involves possible oversensitivity of the climate models that have been used in the studies and the statistical significance of the results.
To address these comments, climate models with appropriate sensitivity and resolution should be used to perform experiments with observed regional anomalies of diabatic forcing, as well as with realistic perturbation simulations (such as between natural and current landscapes). The results should be tested statistically to assess the robustness of any differences. Van den Hurk et al. (2003), for example, conducted three ensembles of five runs each: the control ensemble used constant global leaf area index (LAI) values; the second ensemble used seasonally varying LAI fields; and a third ensemble used the same seasonally varying LAI fields but with a noise term added. This methodology should be adopted for each of the regional diabatic forcings. Sufficient computer resources are required for these computationally expensive integrations.
Simulating Regional Climate
A summary of the current state of regional climate modeling is reported in Kabat et al. (2004). A major new direction is the dynamic coupling between the regional atmosphere and land surface and between the atmosphere and oceans (e.g., Eastman et al., 2001a,b). Coupled atmosphere-sea ice simulations are also being performed. The incorporation of atmospheric chemistry, including aerosol effects, also needs to be included in this dynamic coupling. Matsui et al. (2004), for example, show the sensitivity of the aerosol effects on cloud and precipitation processes due to environmental thermodynamic structure. These modeling tools will permit the investigation of the role of regional radiative forcing in altering regional climate as well as high-spatial-resolution estimates of the ability of regional climate change and variability to teleconnect to other regions and globally.