3
Estimating and Validating Uncertainty
To address the almost unbounded variety of possible uses of uncertainty information in hydrometeorological forecasts (see, e.g., Box 3.1 and Section 2.1), it is essential for NWS to transition to an infrastructure that produces, calibrates, verifies, and archives uncertainty information for all parameters of interest over a wide range of temporal and spatial scales. This chapter focuses on the limitations of current methods for estimating and validating forecast uncertainty and recommends improvements and new approaches. The committee takes the view that these changes are a fundamental first step in transitioning from a deterministic approach to one that enables all users to ultimately harness uncertainty information.
By no means exhaustive, this chapter reviews aspects of the current state of NWS operational probabilistic forecasting, discusses related efforts in the research community, and provides recommendations for improving the production of objective1 uncertainty information. The chapter also discusses subjective approaches to producing uncertainty information that are utilized by human forecasters.
Many groups within NWS generate forecasts and guidance. Those included in this chapter are the Environmental Modeling Center (EMC), the Climate Prediction Center (CPC), the Office of Hydrologic Development (OHD), the Hydrometeorological Prediction Center (HPC), the Weather Forecast Offices (WFOs), the Storm Prediction Center (SPC) and the Space Environment Center (SEC). This sample was chosen because it demonstrates the range of NWS products and also places particular emphasis on the NWS’s numerical “engines,” that is, the centers from which NWS forecast guidance is generated.
The forecasting system components (Table 3.1) of each of the NWS centers covered in this chapter are broadly equivalent in function, but the differences in underlying physical challenges and operational constraints require that each entity be treated differently. The chapter begins with the EMC and discusses the production of global and regional objective probabilistic guidance. It then covers the CPC’s seasonal forecasts that include numerical, statistical, and subjective approaches to probabilistic forecast generation. The multiple space- and time-scale forecasts of the OHD are covered next, and the hydrologist’s unique role as both user and producer of NWS forecast products is highlighted. The subjective generation of forecasts by groups like the WFOs, the HPC, and the SPC is covered, and the chapter ends with a detailed discussion of verification issues. The SEC is presented as an example of an NWS center that makes the quantification and validation of uncertainty central to its operations. The SEC is an example of what can be accomplished within NWS once uncertainty is viewed as being central to the forecasting process (Box 3.2).
3.1
ENVIRONMENTAL MODELING CENTER: GLOBAL AND MESOSCALE GUIDANCE
The EMC2 is one of the National Centers for Environmental Prediction (NCEP3) and is responsible for the nation’s weather data assimilation and numerical weather and climate prediction. The primary weather-related goals of the EMC include the production of global and mesoscale atmospheric analyses through data assimilation, the production of model forecasts through high-resolution control runs and lower-resolution ensemble runs, model development through improved numerics and physics parameterizations,
BOX 3.1 Wide Breadth and Depth of User Needs User needs for hydrometeorological information span a wide variety of parameters and time and spatial scales. For instance, minimum daily temperature is important for citrus farming but has little relevance for water conservation, where seasonal total streamflow is of primary importance. In addition, requirements for uncertainty information are not always well defined among users. Even in the cases where they are well defined, they can vary greatly among users and even for a single user who has multiple objectives. The citrus farmer may require local bias information for a 10-day temperature projection, and at the same time require probability information for a winter-season temperature and precipitation projection. The manager of a large multiobjective reservoir facility may require ensemble inflow forecast information with a seasonal (or longer) time horizon for flood and drought mitigation but with hourly resolution for hydroelectric power production. |
TABLE 3.1 Forecasting System Components
Component |
Description |
Observations |
Observations are the basis of verification and are critical to the data assimilation process. Observations in conjunction with historical forecasts provide a basis for forecast post-processing (e.g., Model Output Statistics, or MOS) and associated uncertainty estimates. |
Data assimilation |
Data assimilation blends observation and model information to provide the initial conditions from which forecast models are launched. Data assimilation can also provide the uncertainty distribution associated with the initial conditions. |
Historical forecast guidance |
An archive of historical model forecasts combined with an associated archive of verifying observations enables useful post-processing of current forecasts. |
Current forecast guidance |
Model forecasts are used by human forecasters as guidance for official NWS forecasts. |
Models |
Models range from first-principle to empirical. Knowledge of the model being used and its limitations helps drive model development and model assessment. |
Model development |
Models are constantly updated and improved, driven by computational and scientific capabilities and, ultimately, the choice of verification measures. |
Ensemble forecasting system |
A collection of initial conditions, and sometimes variations in models and/or model physics, that are propagated forward by a model. The resulting collection of forecasts provides information about forecast uncertainty. Ensemble forecasting systems are developing into the primary means of forecast uncertainty production. |
Forecast post-processing |
Forecast post-processing projects forecasts from model space into observation space. Given a long record of historical forecasts and associated verifying observations it is possible to make the forecasts more valuable for a disparate set of forecast users. Post-processing can be cast in a probabilistic form, naturally providing quantitative uncertainty information. Examples of post-processing include bias correction, MOS, and Gaussian mixture model approaches like Bayesian model averaging (BMA). |
Forecast verification |
Forecast verification is the means by which the quality of forecasts is assessed. Verification provides information to users regarding the quality of the forecasts to aid in their understanding and application of the forecasts in decision making. In addition, verification provides base-level uncertainty information. Verification also drives the development of the entire forecasting system. Choices made in model development, observing system design, data assimilation, etc., are all predicated on a specified set of norms expressed through model verification choices. |
and model verification to assess performance. This section focuses on the global weather modeling component of the Global Climate and Weather Modeling Branch (GMB4) and on the mesoscale weather modeling component of the Mesoscale Modeling Branch (MMB5) of EMC.
3.1.1
Ensemble Forecasting Systems
Ensemble forecasting systems form the heart of EMC’s efforts to provide probabilistic forecast information.6 The aim of ensemble forecasting is to generate a collection of forecasts based on varying initial conditions and model
BOX 3.2 Space Environment Center The Space Environment Center (SEC)a monitors and forecasts Earth’s space environment. It is an example of an NWS center that successfully engages its users, works with users to enhance existing products and develop new products, and conscientiously estimates and includes uncertainty and verification information with its forecasts. The SEC has created a culture and infrastructure that provides quantitative uncertainty information, real-time and historical verification information, and comprehensive product descriptions. Examples of official SEC forecast products that explicitly provide uncertainty information include Geomagnetic Activity Probability forecasts, whole disk flare probabilities, and explicit error bars on graphical and text forecasts of sunspot number. Uncertainty and verification information are explicitly available both for model (or guidance) forecasts and for official forecasts. For example, guidance forecasts from the Costello Geomagnetic Activity Index model include both error bars and an indication of recent model performance by plotting a time series of recent forecasts along with their verifying observations (e.g., Figure 3.1). The SEC has a Web site for communicating the verification statistics of its official (human-produced) forecast products.b On this site the geomagnetic index and short-term warning products are verified using contingency tables (hit rate, false alarm rate, etc.), and the geomagnetic probability forecasts are verified using measures like ranked probability score (and are compared with scores from climatology), reliability, and resolution. ![]() FIGURE 3.1 Model forecast (symbol and bar) and verification (solid line) of the Kp geomagnetic activity index. Note the error bars on the Kp forecast. Historical verification information is available through the associated Web site. SOURCE: SEC Web site, http://www.sec.noaa.gov/index.html. |
The SEC appreciates the importance of providing archives of both observations and forecasts to best serve their users. The observations utilized by the SEC are listed and described on the SEC Data and Products Web site.c In addition, links are provided to the most recent observations and archived measurements. Historical observations are archived by the SEC itself, by the Advance Composition Explorer (ACE) Science Center,d and the National Geophysical Data Center (NGDCe). The SEC Web site provides links to all the relevant archives. A short archive of forecasts and model guidance is available on the SEC Data and Products Web site, and all forecasts are archived at the NGDC. The SEC is a new member of NWS. Prior to January 2005, it was under the National Oceanic and Atmospheric Administration (NOAA) office of Oceanic and Atmospheric Research (OAR). It is a small center with a relatively small number of products and verifying observations. This facilitates interaction among different groups within the SEC and close contact with forecast users. While under OAR the SEC forged strong links with the research community, and its products were directly driven by their user community rather than indirectly through NWS directives. Since joining NWS it has maintained this culture and recognizes the benefits derived from close interaction with the university and user communitiesf The SEC is lightly regulated by NWS directives,g allowing continuity of its OAR culture. Admittedly, the number of variables forecast by and the diversity of user groups served by the SEC are much smaller than for other NWS centers, making it easier to provide uncertainty and verification information and to forge links with the user community. Concomitantly, the SEC is much smaller than other NWS centers, suggesting that their success in engaging the user community is more cultural than resource based. Each year the SEC hosts a “Space Weather Week” meeting that draws internationally from the academic, user, and private communities.h The bulk of the meeting takes place in a single room with approximately 300 participants, leading to strong interactions between the academic, government, private, and user communities. The user community consists of organizations ranging from power companies, airlines, the National Aeronautics and Space Administration (NASA), and private satellite operators. The SEC uses the meeting to identify the needs and concerns of its users and to monitor and influence the efforts of the research community. The SEC values the meeting to such an extent that it has maintained it even while sustaining significant budget cuts. Other examples of links with the extended space weather community are found in SEC’s model development efforts. All the operational models currently utilized by SEC are empirically based; the community does not yet fully understand the relevant physics, and there is not enough of the right type of data to drive physics-based models. SEC’s model development is routed through the Rapid Prototyping Center (RPCi). The aim of the RPC is to “expedite testing and transitioning of new models and data into operational use” and encompasses modeling efforts ranging from simple empirical methods to large-scale numerical modeling. In addition to SEC’s internal efforts, the multiagency Community Coordinated Modeling Center (CCMCj) encompasses over a dozen physics-based research models. Complementing CCMC’s government agency-driven approach is the University-centric Center for Integrated Space Weather Modeling (CISMk). CISM aims to develop physics-based models from the Sun to Earth’s atmosphere. The SEC expects to incorporate physics-based models into its operational suite in the coming years and anticipates making full use of data assimilation and ensemble forecasting approaches to improve the forecast products (Kent Doggett, personal communication). |
specifications that attempts to sample from the uncertainty in both. This collection of forecast states contains information about the uncertainty associated with the forecast. Global ensemble forecasting has been run operationally at NCEP since 1993 (Toth and Kalnay, 1993), and mesoscale ensemble forecasting, also known as short-range ensemble forecasting (SREF), has run operationally since 2001 (Du et al., 2003). Global ensemble forecasts currently consist of 15 ensemble members whereas SREF consists of 21 (McQueen et al., 2005).
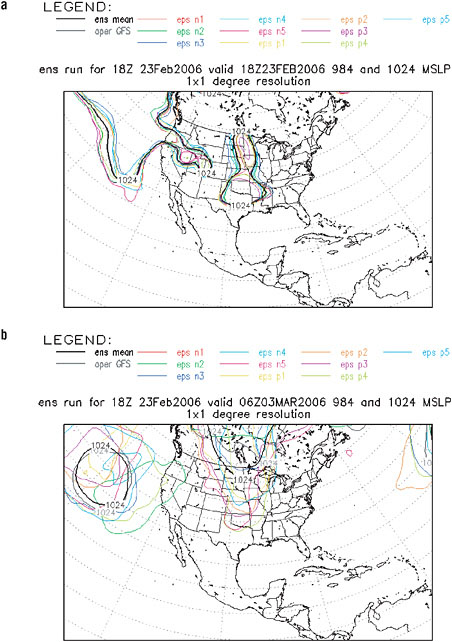
FIGURE 3.2 “Spaghetti” diagrams with contours of the 1024-mb sea-level pressure contour of 11 ensemble members (see legend for a description of the different colors) for (a) the ensemble of initial conditions and (b) after running the model 7.5 days into the future. SOURCE:SOURCE: EMC, http://wwwt.emc.ncep.noaa.gov/gmb/ens/fcsts/ensframe.html.
Many products are derived from the ensemble forecasts. Figure 3.2 shows an example of a GMB ensemble product (the MMB produces a similar product). These so-called spaghetti diagrams plot contours from all ensemble members in a single figure. The degree of difference between the forecast contours provides information about the level of uncertainty associated with the forecast of each parameter (e.g., sea-level pressure). Figure 3.3 shows an example of an MMB product—a meteogram that provides information about five weather parameters at a single location as a function of time.
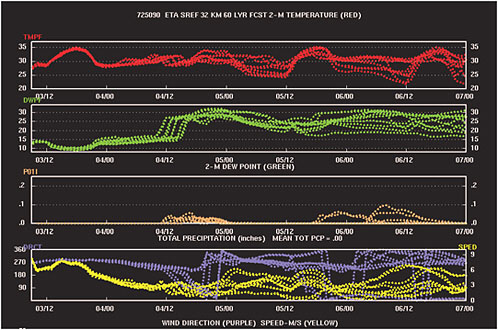
FIGURE 3.3 Example of an experimental SREF ensemble meteogram for Boston. The red curves trace temperature (at 2 meters above the surface) for each ensemble member as forecast time increases, the green curves provide information about dew point temperatures at 2 meters above the surface, the orange curves are precipitation, the purple curves are wind direction, and the yellow curves are wind speed. SOURCE: EMC, http://wwwt.emc.ncep.noaa.gov/mmb/srefmeteograms/sref.html.
This product is experimental and uncalibrated, but provides information about the uncertainty associated with each parameter from the spread of lines within each panel. Both GMB and MMB provide links from their ensemble Web page to many other methods of visualizing and communicating the information contained in their ensemble forecasts.
Mesoscale ensemble systems are also being run in the university environment. For example, a mesoscale ensemble system with 36- and 12-km grid spacing has been run at the University of Washington since 1999. The academic community is studying variants of the ensemble Kalman filter (EnKF) approach (Evensen, 1994), including the University of Washington implementation of a real-time EnKF data assimilation (Box 3.3) and ensemble forecasting system for the Pacific Northwest.7 The National Center for Atmospheric Research (NCAR) hosts a Data Assimilation Research Testbed8 which enables researchers to use state-of-the-science EnKF data assimilation with their model of choice. The testbed also enables observationalists to explore the impact of possible new observations on model performance and allows data assimilation developers to test new ideas in a controlled setting.
Selection of initial conditions for ensemble forecasting is critically important. The GMB and MMB both use a “bred vector” approach to generate initial ensemble members. This approach relies on model dynamics to identify directions that have experienced error growth in the recent past (see Box 3.4). The primary benefits of the breeding methodology are that it provides an estimate of analysis uncertainty (Toth and Kalnay, 1993) and that it is computationally inexpensive. Recognizing the intimate links between data assimilation and the specification of initial ensemble members, the research community has taken a lead in developing ensemble-based approaches to data assimilation and ensemble construction using the EnKF approach (e.g., Torn and Hakim, 2005; Zhang et al., 2006). The GMB has recently implemented an “ensemble transform” ensemble generation technique that utilizes an approximation to the ensemble transform Kalman filter ideas of Wang and Bishop (2005). The EMC is also experimenting with ensemble-based data assimilation through its involvement in THORPEX (Box 3.5),
BOX 3.3 Data Assimilation Numerical Weather Prediction (NWP) is primarily an initial condition problem, and data assimilation (DA) is the process by which initial conditions are produced. DA is the blending of observation information and model information, taking into account their respective uncertainty, to produce an improved estimate of the state called the analysis. DA is also capable of providing uncertainty estimates associated with the analysis, which, in addition to quantifying the uncertainty associated with the initial state estimate, can be used to help construct initial ensembles for ensemble forecasting efforts. Numerous forms of data assimilation exist, and they can be broadly classified as either local in time or distributed in time. Distributed-in-time approaches use trajectories of observations rather than single snapshots. Within each classification one can choose to take a variation approach to solving the problem or a linear algebra, direct-solve approach. Local-in-time techniques include the Kalman Filter, three-dimensional variational assimilation (3d-Var), the various versions of EnKFs, and ensemble-based perturbed observation versions of 3d-Var. Distributed-in-time techniques include the Kalman smoother, four-dimensional variational assimilation (4d-Var), the various versions of ensemble Kalman smoothers, and ensemble-based perturbed observation versions of 4d-Var. In the operational context, the NCEP Spectral Statistical Interpolation (SSI) uses a 3d-Var approach and the European Centre for Medium-range Weather Forecasts (ECMWF) and the Canadian Meteorological Centre (CMC) use 4d-Var. Data assimilation requires estimates of uncertainty associated with short-term (typically 6-hr) model forecasts, and the uncertainty associated with these forecasts is expected to change as a function of the state of the atmosphere. The computational costs associated with the operational DA problem can make estimation of the time-varying forecast uncertainty prohibitively expensive, but the benefits of doing so are likely to be felt both in the quality of the analysis and in the quality of the uncertainty associated with the analysis. This uncertainty can then be used to help in the construction of initial ensemble members, ultimately improving ensemble forecasts. There is thus an intimate link between the DA problem and the ensemble forecasting problem. The ensemble approach to DA is one way to incorporate time-varying uncertainty information. The CMC now runs an experimental EnKF data assimilation system that compares favorably with 4d-Var,a demonstrating that accounting for time-varying uncertainty information is possible in the operational setting. The move by the EMC to the Gridpoint Statistical Interpolation system will, among other things, enable the inclusion of time-varying forecast uncertainty information in the DA process. Work funded by NOAA’s THe Observing system Research and Predictability Experiment (THORPEX) initiativeb is exploring both ensemble-based filtering and hybrid ensemble/3d-Var approaches aimed at the operational DA problem. |
with planned prototype testing and a tentative operational transition date of 2009 if the approaches prove useful.9 Other operational centers use different approaches to ensemble construction (see Box 3.4).
Finding: A number of methods for generating initial ensembles are being explored in the research and operational communities. In addition, ensemble-based data assimilation approaches are proving beneficial, especially at the mesoscale.
Recommendation 3.1: As the GMB and the MMB of the EMC continue to develop their ensemble forecasting systems, they should evaluate the full range of approaches to the generation of initial ensembles and apply the most beneficial approach. The EMC should focus on exploring the utility of ensemble-based data assimilation approaches (and extensions) to couple ensemble generation and data assimilation at both the global and the mesoscale levels.
3.1.2
Accounting for Model Error in Ensemble Forecasting
A limitation of any ensemble construction methodology based on a single model is the difficulty in accounting for model inadequacy. This is related to the problem of ambiguity in Chapter 2—the notion of the uncertainty in the estimate of the uncertainty. One approach that attempts to account for model inadequacy during ensemble forecasting is to use different models and/or different parameterizations in the same model. This approach is theoretically underpinned by an extensive literature on Bayesian statistical approaches to explicitly considering multiple models/parameterizations
BOX 3.4 Ensemble Forecasting and Ensemble Initial Conditions Background The aim of ensemble forecasting is to provide uncertainty information about the future state of the atmosphere. Rather than running models once from a single initial condition, a collection of initial conditions are specified and the model is run forward a number of times. The range of results produced by the collection (ensemble) of forecasts provides information about the confidence in the forecast. There are two important conditions that must be met for the ensemble of forecast values to be interpreted as a random draw from the “correct” forecast probability density function (PDF): (1) the ensemble of initial conditions must be a random draw from the “correct” initial-condition PDF, and (2) the forecast model must be perfect. In practice these conditions are never met, but a probabilistically “incorrect” ensemble forecast is not necessarily useless; with a sensible initial ensemble and appropriate post-processing efforts and verification information, useful information can be extracted from imperfect ensemble forecasts. NCEP Ensembles The GMB utilizes the so-called bred vector approach to ensemble construction (Toth and Kalnay, 1993). Ensemble perturbations are “bred” by recording the evolution of perturbed and unperturbed model integrations. Every breeding period (typically 6 model hours), the vector between a 6-hour high-resolution control forecast and each 6-hour forecast ensemble member is identified. The magnitude of the perturbation vector is reduced and its orientation is rotated via a component-wise comparison between the perturbation vector and an observation “mask” which is meant to reflect the spatial distribution of observations; components in data-rich areas are shrunk by a larger factor than those in data-sparse regions. Data assimilation is performed on the 6-hour high-resolution control forecast, and each of the rescaled and rotated ensemble perturbations is added to the new analysis. The structure of initial-condition uncertainty is defined by the data assimilation scheme. Because the bred-vector approach only crudely accounts for the impact of data assimilation through the use of the observation mask the initial ensemble draws from an incorrect initial distribution. However, utilizing the model dynamics to “breed” perturbations still provides useful information. In addition to using the bred-vector method to generate perturbations in the model initial conditions, the regional ensemble system of the MMB (the SREF) attempts to account for model inadequacy by utilizing a number of different models and a number of different sub-gridscale parameterizations within models. The global ensemble system is also moving toward a multimodel ensemble approach through its involvement with the THORPEX Interactive Grand Global Ensemble (TIGGE), and the North American Ensemble Forecasting System (NAEFS). ECMWF Ensembles The ECMWF has a different ensemble construction philosophy. They utilize a “singular vector” approach to ensemble construction where they attempt to identify the directions with respect to the control initial condition that will experience the most error growth over a specified forecast period. These very special directions are clearly not random draws from an initial condition PDF,a but they are dynamically important directions that will generate a significant amount of forecast ensemble spread and have been shown to provide useful forecast uncertainty information. CMC Ensembles The CMC takes a third approach. They utilize an EnKF for their operational ensemble construction scheme (Houtekamer and Mitchell, 1998). Ensemble filters utilize short-term ensemble forecasts to define the uncertainty associated with the model first guess. The product of the data assimilation update is not a single estimate of the state of the system but rather an ensemble of estimates of the state that describe the expected error in the estimate. The EnKF naturally provides an ensemble of initial conditions that are a random sample from what the data assimilation system believes is the distribution of initial uncertainty. The CMC uses a multimodel approach to the EnKF where different ensemble members have different model configurations. This allows for partial consideration of model inadequacy in the EnKF framework. Model inadequacy in the context of ensemble forecasting is discussed in Section 3.1.2. |
BOX 3.5 THORPEX—A World Weather Research Programa THORPEX is a part of the World Meteorological Organization (WMO) World Weather Research Programme (WWRP). It is an international research and development collaboration among academic institutions, operational forecast centers, and users of forecast products to accelerate improvements in the accuracy of 1-day to 2-week high-impact weather forecasts for the benefit of society, the economy, and the environment, and to effectively communicate these products to end users.b Research topics include global-to-regional influences on the evolution and predictability of weather systems; global observing system design and demonstration; targeting and assimilation of observations; and societal, economic, and environmental benefits of improved forecasts. A major THORPEX goal is the development of a future global interactive, multimodel ensemble forecast system that would generate numerical probabilistic products that are available to all WMO members. These products include weather warnings that can be readily used in decision-support tools. The relevance of THORPEX to this report is primarily through its linkage of weather forecasts, the economy, and society. Social science research is an integral component of the THORPEX science plan.c For example, THORPEX Societal/Economic Applications research will (1) define and identify high-impact weather forecasts, (2) assess the impact of improved forecast systems, (3) develop advanced forecast verification measures, (4) estimate the cost and benefits of improved forecast systems, and (5) contribute to the development of user-specific products. This research is conducted through a collaboration among forecast providers (operational forecast centers and private-sector forecast offices) and forecast users (energy producers and distributors, transportation industries, agriculture producers, emergency management agencies and health care providers). THORPEX also forms part of the motivation for the GMB of the EMC to implement multimodel ensemble forecasting, sophisticated post-processing techniques, and comprehensive archiving of probabilistic output. The goals of TIGGE ared
NOAA is an active participant and has funded a range of THORPEX-related external research on observing systems, data assimilation, predictability, socioeconomic applications, and crosscutting efforts.e |
(e.g., Draper, 1995; Chatfield, 1995). The GMB does not account for this type of uncertainty but is addressing it in part through its participation in TIGGE (Box 3.5) and the NAEFS with Canada and Mexico (which will be extended to include the Japan Meteorological Agency, UK Meteorological Office, and the Navy’s operational forecasting efforts). The NAEFS will facilitate the real-time dissemination of ensemble forecasts from each of the participating countries in a common format. Research is under way among NWS and its international partners to provide coordinated bias-correction, calibration, and verification statistics.
On the mesoscale, the MMB ensemble system uses multiple models and varied physics configurations.10 In such multimodel ensemble forecasting efforts it is important to account for differences in (1) methods of numerically solving the governing dynamics and (2) physical parameterizations. Within the 21 NWS SREF ensemble members there is some
diversity in model physics, with particular emphasis given to varying convective parameterizations. A number of major sources of uncertainty still need to be explored, including variations in surface properties and uncertainties in the boundary-layer parameterizations.
An alternative approach to generating ensemble initial conditions that takes information about model differences into account is the use of initial conditions (and boundary conditions in the case of mesoscale models) from a variety of operational centers. Grimit and Mass (2002) demonstrated the utility of this approach in the mesoscale modeling context and Richardson (2001) explored it in the global modeling context. Richardson found that for their chosen means of verification, a significant portion of the value of multimodel ensemble forecasts was recovered by using a single model to integrate the initial conditions from the collection of available models. Model error can also be accounted for stochastically. Such an approach is in operational use at ECMWF and is being explored by EMC.
Finding: There is a range of approaches to help account for model inadequacy in ensemble forecasting. So far, NCEP has explored varying physics parameterizations, multimodel initial conditions, and stochastic methods.
Recommendation 3.2: The NCEP should complete a comprehensive evaluation to determine the value of multiple dynamical cores and models, in comparison to other methods, as sources of useful diversity in the ensemble simulations.
3.1.3
Model Development
The MMB SREF approach uses an ensemble system with 32-km grid spacing and the output available on 40-km grids. Such a resolution is inadequate to resolve most important mesoscale features, such as orographic precipitation, diurnal circulations, and convection, and greatly lags the resolution used in deterministic mesoscale prediction models (generally 12 km or less). An inability to resolve these key mesoscale features will limit the utility of the ensemble forecasting system. Although post-processing (Section 3.1.5) can be applied to the SREF system in an effort to downscale model forecasts to higher resolution, comparisons between the University of Washington SREF system (run at 12-km resolution) and MOS have shown that even without post-processing the SREF system was superior in predicting the probability of precipitation (E. P. Grimit, personal communication). In addition, Stensrud and Yussouf (2003) demonstrated the value of an SREF system in comparison to nested grid model MOS in a NOAA pilot project on temperature and air quality forecasting in New England. Generically, SREF systems have the ability to be adaptive to flow-dependent errors to a greater degree than MOS, as MOS depends on a long training period (typically 2 years). SREF systems can easily accommodate rapidly developing forecasting systems.
Moving the NCEP SREF system to higher spatial resolution will require substantial computer resources, but the near-perfect parallelization of ensemble prediction makes possible highly efficient use of the large number of processors available to NCEP operations.
Finding: The spatial resolution of the MMB SREF ensemble system is too coarse to resolve important mesoscale features (see also Chapter 5, recommendation 4). Moving to a finer resolution is computationally more expensive, but necessary to simulate key mesoscale features.
Recommendation 3.3: The NCEP should (a) reprioritize or acquire additional computing resources so that the SREF system can be run at greater resolution or (b) rethink current resource use by applying smaller domains for the ensemble system or by releasing time on the deterministic runs by using smaller nested domains.
3.1.4
Archiving Observations and Forecasts
To provide maximum value, a forecasting system must make available all the information necessary for interested parties to post-process and verify ensemble forecasts of variables and/or diagnostics. Required information includes an archive of historical analyses (initial conditions), historical forecasts, and all the associated verifying observations. Historical model information is available in four forms: archived analyses, reanalyses, archived forecasts, and reforecasts. The archived analyses and forecasts reflect the state of the forecast system at the time of their generation; data assimilation methodologies, observations, and models change with time. The reanalyses and reforecasts attempt to apply the current state of the science retroactively. Archived analyses, reanalyses, archived forecasts, and a wide range of observations with records of various lengths are archived and searchable at the National Climatic Data Center (NCDC11). Within NCDC is the NOAA National Operational Model Archive and Distribution System (NOMADS12), which provides links to an archive of global and regional model output and forecast-relevant observations in consistent and documented formats.
NOMADS provides the observation and restart files necessary to run the operational data assimilation system (SSI). NWS does not produce a reforecast product, but reforecasts are available from the NOAA Earth System Research Laboratory (ESRL13). The model used is crude by current standards, but the long history of ensemble forecasts permits calibration that renders long-lead probabilistic fore-
casts superior to those produced operationally. The reforecast product has numerous applications (Hamill et al., 2006) and is most useful when the model used to produce the reforecast dataset is the same model that is used for the production of operational guidance.
Finding: An easily accessible observation and forecast archive is a crucial part of all post-processing or verification of forecasts (see also Chapter 5, recommendation 6).
Recommendation 3.4: The NOAA NOMADS should be maintained and extended to include (a) long-term archives of the global and regional ensemble forecasting systems at their native resolution, and (b) reforecast datasets to facilitate post-processing.
Finding: Reforecast data provide the information needed to post-process forecasts in the context of many different applications (e.g., MOS, hydrology, seasonal forecasts). NWS provides only limited reforecast information for some models and time periods. In addition, post-processing systems need to change each time the numerical model changes. To facilitate adaptation of applications and understanding of forecast performance, reforecast information is needed for all models and lead times whenever a significant change is made to an operational model.
Recommendation 3.5: NCEP, in collaboration with appropriate NOAA offices, should identify the length of reforecast products necessary for time scales and forecasts of interest and produce a reforecast product each time significant changes are made to a modeling/ forecasting system.
3.1.5
Post-processing
It is difficult to overemphasize the importance of post-processing for the production of useful forecast guidance information. The aim of post-processing (Box 3.6) is to project model predictions into elements and variables that are meaningful for the real world, including variables not provided by the modeling system. Common examples of post-processing include bias correction, downscaling, and interpolation to an observation station. Post-processing methods specifically for ensemble forecasts also exist.
NWS does little post-processing of its ensemble forecasts to provide reliable (calibrated) and sharp probability distributions (see also Chapter 5, recommendation 5). NWS’s Meteorological Development Laboratory (MDL) provides post-processed, operational guidance products through their MOS14 (Box 3.7), with several of them providing probabilistic guidance. MOS finds relationships between numerical forecasts and verifying observations and is applied to output
BOX 3.6 Post-processing Post-processing of model output has been a part of the forecasting process at least as long as the MOS (Box 3.7) approach has been used to produce forecast guidance. Post-processing has become a critical component of the interpretation and use of predictions based on ensemble forecasts. Post-processing makes it possible for meaningful and calibrated probabilistic forecasts to be derived from deterministic forecasts or from ensembles. Post-processing of models projects forecasts from model space into observation space and produces improved forecasts of the weather element of interest. Post-processing has a role across a spectrum of applications, including the calibration of ensemble predictions of specific prognostic variables; the interpretation of sets of upper-air prognostic variables in terms of a surface weather variable; and the combination of ensemble-based distribution functions. Post-processing can be cast in a probabilistic form and thus naturally provides quantitative uncertainty information. Examples of post-processing methods include bias corrections based on regression, MOS interpretation of upper air prognostic variables, and Gaussian mixture model approaches like BMA (Raftery et al., 2005) to combine probability distributions. Verification is an important aspect of post-processing, since the choice of post-processing depends on how the forecast is optimized (see Section 3.4). Post-processing is a necessary step toward producing final guidance forecasts based on model forecast output. |
from both global and mesoscale models. MOS can often remove a significant portion of the long-term average bias of model predictions and can provide some information on local or regime effects not properly considered by the model. The MOS flagship products are minimum and maximum temperature along with probability of precipitation (PoP; e.g., Figure 3.4), but it is also applied to variables like wind speed and direction, severe weather probabilities, sky cover and ceiling information, conditional visibility probabilities, and probability of precipitation type. Online verification statistics are available for maximum temperature, minimum temperature, and PoP as a function of geographical region, forecast lead time, and numerical model.15
The probabilistic MOS products are based on deterministic forecasts. An experimental ensemble MOS product exists, but because climatology is included as a MOS predictor all ensemble MOS forecasts tend to converge to the same answer for longer forecasts. The implied uncertainty decreases rather than increases at the longest lead times. There are
BOX 3.7 Model Output Statistics MOS is a technique used to objectively interpret numerical model output and produce site-specific guidance.a MOS was developed in the late 1960s as the original form of post-processing (Box 3.6) and was designed to improve forecasts of surface weather variables based on the output from deterministic NWP models. To develop the MOS equations, statistical methods are used to relate predictors (i.e., NWP output variables) to observations of a weather element. Typically, MOS equations are based on multiple linear regression techniques (Neter et al., 1996). Each MOS equation is relevant for a single predictand, region, model, projection, and season, and the equations are applied at individual locations to produce NWS guidance forecasts. Single regression equations can be used directly to produce probability forecasts for binary events. For probability forecasts of elements with multiple categories (e.g., precipitation amount), the multiple probabilities are typically derived using a procedure called “Regression Estimation of Event Probabilities” in which the predictand space is subdivided and separate regression equations are developed and applied to each subcategory. This procedure approximates a forecast of a probability distribution. More information about these methods and processes can be found in Wilks (2006). PoP is the most recognizable official probabilistic forecast produced by MOS, but MOS probability forecasts are produced for a number of other variables (e.g., precipitation amount, ceiling) and these probabilities are used to identify the most likely category, which is subsequently provided in the official forecasts. These underlying probabilities are available graphically at the MOS Web site. Currently, MDL is developing gridded MOS forecasts that can be used by forecasters as guidance for preparing forecasts for the National Digital Forecast Database. |
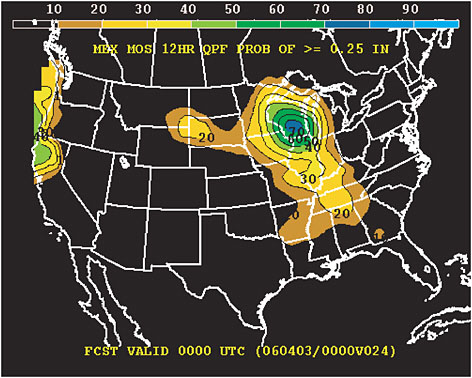
FIGURE 3.4 Example of probabilistic forecast of precipitation amount based on MOS applied to the Global Forecasting System model output. SOURCE: NWS.
other means of ensemble post-processing. For example, the ESRL reforecasting product can produce ensemble analogs, whereas the BMA approach of Raftery et al. (2005) attempts to fit and combine Gaussian distributions to output from multimodel ensemble forecasts. The EMOS procedure of Gneiting et al. (2005) also combines multimodel ensemble forecast information but with the additional constraint that the post-processed PDF be a Gaussian.
NWS is considering a National Digital Guidance Database (NDGD) to support the National Digital Forecast Database (NDFD; Zoltan Toth, personal communication). In much the same way as the NDFD can be interrogated by users to provide deterministic forecast information, the NDGD could be interrogated to provide probabilistic forecast information. NWS intends to provide a database of raw and post-processed guidance for this purpose. The plans for the initial post-processed product are to provide gridded probability thresholds for near-surface weather variables produced from bias-corrected NAEFS ensembles. The EMC also plans to implement a post-processing procedure for the ensemble spread. Ultimately, the NDGD would include information from both the global and the mesoscale modeling systems.
Finding: A database such as the NDGD would provide a wealth of information for the NWS forecaster and academic researcher and provide a basis for significant added value by the private sector, catalyzing economic activity. In addition, there is a strong complementarity between NDGD and NOMADS.
Recommendation 3.6: Efforts on the proposed NDGD should be accelerated and coordinated with those on the NOAA NOMADS (recommendation 3.4).
3.1.6
The Enterprise as a Resource
Scientists in GMB and MMB are aware of the issues raised in this chapter and are eager to continue their development of the forecast guidance system in an increasingly probabilistic manner. Indeed, there are several internal documents that make similar recommendations.16,17 NWS scientists are making strides with their ensemble guidance products and are performing internal research in multimodel ensemble forecasting and the probabilistic post-processing of ensemble guidance.
Funding and personnel are always limiting factors, but a huge resource exists in the form of the Enterprise external to NWS, particularly the academic community. There are examples of successful academia partnerships at NWS, including at GMB and MMB, but wider-reaching, more formalized partnerships are needed.
Without frequent and close interaction with NCEP, it is difficult for academics to appreciate the constraints of an operational setting. For example, outside researchers cannot anticipate the potential difficulty of implementing a seemingly trivial change because they do not have a working knowledge of the relevant software systems and how they interact. In addition, after implementing each new idea a series of experiments need to be run to assess the impact of the change. These experiments are expensive, and there are far more ideas than available computer time.
Beyond the partnership of NCEP with external research entities, and given the great variety of potential users and uses, it will be necessary to involve users in designing the form and content of forecast products and intermediaries in developing useful products and decision-support systems. The interaction of forecasters and emergency managers, for instance, could establish norms of communication, providing forecasters with the opportunity to understand better the objectives of emergency management and their temporal and spatial determinants and providing emergency managers with information on what is feasible in terms of forecast uncertainty descriptors from operational forecast systems.
The transition of NWS (and the entire Enterprise) to uncertainty-centric prediction will require advances in forecasting and communication technologies that demand the close cooperation of the research, operational, and user communities. An approach to fostering such cooperation is through testbeds. Testbeds provide laboratories for evaluating new prediction and dissemination approaches in a pseudo-operational setting before they are used on a national scale, bringing expertise and experience outside of national centers to bear on key problems. Testbeds have been shown to be an effective approach to promoting closer cooperation between academic, government, and private sectors of the Enterprise, while enhancing communication with users.
Testbeds have been developed in a variety of forms. In California, a Hydrometeorological Testbed has brought together NOAA, universities, and state and local agencies to study heavy precipitation and flooding along the mountainous western states. Another testbed in northern California has brought together hydrologic forecast and water resources management agencies with researchers from academic and nonacademic institutions to assess the utility of operational weather and climate information for operational reservoir management in large reservoir facilities. In the Pacific Northwest, the Northwest Consortium—a group that includes state, federal, and local agencies, as well as local universities and private firms—has promoted the development and real-time evaluation of new mesoscale forecasting approaches. On a national scale, the Developmental Testbed Center (DTC) combines the efforts of several federal agencies and NCAR to evaluate and support advances in the WRF modeling system, and the joint NOAA-Navy-NASA Hurricane
Testbed aims to advance the transfer of new research and technology into operational hurricane forecasting systems by serving as a conduit between the operational, academic, and research communities.
Considering the profound changes in practices that a shift to probabilistic prediction and communication will entail, the entrainment of the larger community made possible by the testbed approach will enable rapid advancement. For example, regional testbeds can evaluate methods of ensemble generation and post-processing, as well as new approaches for communicating uncertainty information. An example of the potential of this approach is the Northwest Consortium’s mesoscale ensemble system. The information from this ensemble system is displayed on a variety of Web sites that experiment with intuitive approaches to display and communication.18 Other testbeds could focus on providing uncertainty information for specific forecasting challenges (e.g., hurricanes, severe convection, flooding). A national uncertainty testbed could also be established within the DTC to bring together a broad community to evaluate generally applicable approaches to ensemble generation, post-processing, verification, communication, and application development. In short, testbeds offer a powerful extension and multiplier of the efforts at major national modeling centers such as NCEP and the Fleet Numerical Meteorology and Oceanography Center.
Finding: The United States suffers from a separation between operational numerical weather prediction and the supporting research community (see, for example, NRC, 2000). This separation increases the challenge of communicating operationally relevant scientific problems to academia and providing resources and motivation for NWS scientists to work more closely with their academic colleagues. In addition, for a paradigm shift from deterministic to probabilistic forecasting to be useful for a variety of users, a means of participation of such users and intermediaries in the forecast product design and communication phases is critical. Potential approaches for strengthening the links between the operational and research communities range from development and support of testbeds to development of lists of operational goals and research needs19 to ensuring that supporting and descriptive documentation on NWS Web sites is up to date.
Recommendation 3.7: NWS should work toward a culture and systems that encourage interactions among all components of the Enterprise and should use testbeds as a means of bringing together diverse groups from different disciplines and operational sectors. With the help of external users and researchers, NWS centers and research groups should construct and disseminate a prioritized list of operational goals and associated research questions. These lists should be dynamic, providing mechanisms by which NWS can elicit feedback from the research and user communities and the research and user communities can support and drive the direction of NWS. Potential solutions to these research questions could then be explored in testbeds.
3.2
CLIMATE PREDICTION CENTER
The charge of the CPC20 is to monitor and forecast short-term climate fluctuations. In addition, CPC provides information on the effects climate patterns can have on the nation. This section focuses only on CPC’s monthly and seasonal outlooks and the approach used to estimate the uncertainty associated with these forecasts. CPC also provides a variety of other forecasts, including 6- to 10- and 8- to 14-day outlooks, tropical cyclone outlooks, and weekly hazard assessments that are not considered here.
3.2.1
Scientific Basis and Forecast Methodology
The primary basis for skill in monthly and seasonal climate predictions lies in the ability to forecast sea surface temperatures, especially those associated with El Niño conditions, along with linkages of the El Niño-Southern Oscillation (ENSO) state and, less importantly, the state of other climate system oscillations to global seasonal temperature and precipitation anomalies. Additional capability is associated with the use of information about land surface conditions (e.g., soil moisture). The CPC approach to seasonal forecasting involves the combination of information from a variety of statistical and analog models with the output of the recently implemented Climate Forecast System (CFS) numerical weather prediction model, which was developed and is run operationally by the NCEP Global Climate and Weather Modeling Branch. Information from these different sources is subjectively combined by CPC forecasters to create the operational outlooks (Saha et al., 2006). These outlooks are probabilistic and thus provide direct information about the uncertainty associated with the forecasts.
The CFS is a fully coupled ocean-land-atmosphere dynamical model, with initial conditions obtained from the Global Ocean Data Assimilation System. The model is well described and evaluated by Saha et al. (2006). An ensemble mean based on 40 members is used in the seasonal forecasting process. To facilitate optimal use of the CFS, NCEP has created many years of reforecasts (i.e., applications of the CFS to historical observations) for seasonal projections. The reforecast data provide useful information for understanding the performance characteristics of the CFS and for correcting
18 |
For example, http://www.probcast.com. |
19 |
See, for example, the CPC list at http://www.cpc.ncep.noaa.gov/products/ctb/Laver.ppt, and the research plans associated with the WMO THORPEX program. |
20 |
model biases, and they provide opportunities for a wealth of forecasting experiments. Reforecast data are available for the seasonal forecasts but not for shorter lead times due to the large ocean data requirements. Recommendation 3.5 is addressed at this limitation.
The statistical and analog methods that are used to create the CPC forecasts include canonical correlation analysis, ENSO composites, Optimal Climate Normals, Constructed Analog on Soil moisture, and Screening Multiple Linear Regression. The output of these guidance forecasts, used in creating the final outlooks, is also presented on the CPC’s Web page.21 The CPC has begun to experiment with objective approaches for combining the various pieces of information (i.e., results of the CFS and statistical/analog approaches) to create the final forecasts, rather than using the heuristic, subjective approach that has been the operational standard. Relatively large improvements in forecasting performance have been obtained even with the simple objective approach applied so far. Not only are the forecasts more skillful, but the areas with forecasts that differ from climatology are larger. These positive initial efforts toward objective combination of the different forms of information are based on the historical performance of the forecast methods. More sophisticated approaches are under development.
Finding: The CPC is making great strides in improving information to guide development of their monthly and seasonal forecasts (e.g., development and implementation of the CFS), but there is room for further improvement through post-processing and use of the full CFS ensemble.
Recommendation 3.8: The CPC should investigate methods to use the full distribution of the CFS ensemble members (e.g., through a post-processing step) rather than relying solely on the ensemble mean or median. In addition, the center should make use of reforecast datasets and historical forecast performance information for developing the monthly and seasonal probabilities.
Finding: The CPC has had success with its initial efforts to use objective approaches to combine its various sources of forecast information using simple weighting schemes. More sophisticated methods (e.g., expert systems, statistical models) for combining the forecast components are likely to lead to further improvements in forecast performance.
Recommendation 3.9: The CPC should develop more effective objective methods for combining forecast components to improve forecast performance.
3.2.2
Performance of NCEP/CPC Monthly and Seasonal Predictions
Limited information regarding forecast skill is provided on the CPC Web pages. This information is primarily in the form of time-series plots of the Heidke skill score (HSS)22 accumulated across all continental U.S. climate districts. These plots indicate that the forecasts have limited overall skill (e.g., an average HSS of around 0.10 to 0.20 for temperature forecasts and 0.0 to 0.10 for precipitation forecasts, which suggests that the forecasts are 0 to 20 percent better than random forecasts). However, the scores exhibit significant year-to-year variability (i.e., sometimes the skill is strongly positive and at other times it is strongly negative). Results presented to the committee by Robert Livezey (September 2005) and Ed O’Lenic (August 2005) indicate that the skill also varies greatly across regions and seasons and that skill is minimal at longer lead times for most locations. The best performance occurs when there is strong forcing (e.g., from an El Niño). When this forcing does not exist, the skill of the outlooks may be about the same as a climatological forecast. The precipitation outlooks, in particular, have very little skill in non-ENSO years. Recommendation 6 in Chapter 5 is addressed at the issues raised in this and related sections.
Finding: In many cases the CPC’s monthly and seasonal forecasts have little skill, especially at long lead times. Other forecasting groups, such as the International Research Institute, only provide forecasts out to 6 months due to the limited—or negative—skill for longer projections.
Recommendation 3.10: The CPC should examine whether it is appropriate to distribute forecasts with little skill and whether projections should be limited to shorter time lengths. Information about prediction skill should be more readily available to users.
3.2.3
Research on Seasonal Prediction
Research on seasonal prediction includes improvements in land surface observations, development of improved land surface models, and improved methods for incorporating the land surface information in the prediction models. In addition, the use of multimodel ensembles for seasonal prediction and interpretation of the ensemble output are areas of current research. There is a strong research community focused on the climate forecasting problem. For example, seasonal prediction is being studied at a variety of research laboratories and educational institutions, including the Center for Ocean-Land-Atmosphere Studies, NCAR, and the Geophysical
21 |
http://www.cpc.ncep.noaa.gov/products/predictions/90day/tools/briefing/. |
22 |
The HSS measures the skill of the CFS in assigning the correct precipitation or temperature categories, relative to how well the categories could be assigned by chance. See Box 3.9. |
Fluid Dynamics Laboratory, among other groups, as well as at many universities.
NCEP recently established a Climate Test Bed (CTB), associated with the CPC, to accelerate the transfer of new technology into operational practice.23 The CTB provides a natural link between the seasonal prediction research community and the operational center and facilitates the ability for researchers to develop and test tools, models, and methodologies in an operational context. Given adequate resources, this setting should make it easier and more straightforward for the CPC to quickly incorporate new research results into operational practice and will thus have the potential to greatly improve CPC’s forecasts and services.
Finding: The CTB provides an opportunity for the CPC to efficiently incorporate new knowledge and technologies into their forecasting processes.
Recommendation 3.11: The NWS and the NCEP should fully support the CTB to engage the Enterprise, particularly the research community, in operational problems and develop meaningful approaches that enhance and improve operational predictions.
3.3
OFFICE OF HYDROLOGIC DEVELOPMENT
The vision statement from the NWS OHD (NWS, 2003) expands the NWS operational hydrology role and responsibilities from the long-time focus on producing river and stream forecasts to such fields as drought prediction, soil moisture estimation and prediction, precipitation frequency, and pollutant dispersion. Primary customers for this information are the forecasters of the NWS River Forecast Centers (RFCs), WFOs, and National Centers. In addition, the OHD vision states that development and tools are produced to inform the appropriate elements of federal, state, and local government, industry, and the public to help manage water resources. There are many ways that NWS’s RFCs use meteorological information (forecasts and data) to produce deterministic and ensemble hydrology forecasts. The procedures and products vary significantly from center to center, and in several cases forecaster subjective judgment is involved in product generation. The following discussion presents several types of operational hydrologic forecast products, emphasizing the associated sources of uncertainty. The discussion then highlights recent activities within OHD that target the production of probabilistic and ensemble hydrologic forecasts and suggests improvements.
3.3.1
Operational Hydrology as a User of NCEP and Weather Forecast Office Products and a Producer of Streamflow Forecasts
The OHD, the Hydrology Laboratory, and 13 regional RFCs provide hydrologic forecast services for the nation. Such forecast services require upstream input (e.g., precipitation forecasts) produced by NCEP, and, thus, the hydrologic service offices are direct users of input products generated by other parts of NWS. In addition, the NWS hydrologic services offices and centers provide advanced hydrologic forecast products for the public, flood risk managers, and other users. Thus, the NWS hydrologic service has a dual role as both a user and a provider of NWS forecasts.
3.3.1.1
Operational Hydrology Products
River and streamflow forecasts are the main operational hydrology products. Their development under the current operational forecast system in most RFCs is based on water volume accounting over hydrologic catchments using a variety of numerical and conceptual models that include some type of channel routing procedure. Most of these models preserve mass in the snow/soil/channel system of the land surface drainage and parameterize internal and output flow versus storage relationships with a set of parameters whose values require estimation from historical input (precipitation and temperature) and output (flow discharge) data (e.g., Burnash, 1995; Fread et al., 1995; Larson et al., 1995; Anderson, 1996). Short-term flow forecasts out to several days with 6-hourly resolution and ensemble streamflow forecasts out to several months with 6-hourly or daily resolution are routinely produced by RFCs around the country. In addition, very-short-term flash-flood guidance estimates are produced for the development of flash-flood warnings on small scales (counties) out to 6 hours with hourly resolution (e.g., Sweeney, 1992; Reed et al., 2002).
The short-term streamflow forecasts are generated in a deterministic way using the RFC models and best estimates of mean areal model forcing (Figures 3.5 and 3.6). In some cases (e.g., forks of the American River in California) state estimators based on Kalman filtering are used with streamflow observations to update the model states (e.g., water stored in various soil zones and water stored in channel reaches) and to generate estimates of variance in short-term forecasts (e.g., Seo et al., 2003). An outline of the types of observations relevant to the hydrological prediction problem and their uncertainty is given in Box 3.8.
A variety of long-term forecasts is produced routinely for water managers to provide them with guidance in water supply decisions. Two common approaches are regression on the basis of observed hydrologic states and seasonal temperature and precipitation forecasts, and ensemble streamflow prediction (ESP). Seasonal water supply outlooks are produced for several western states on the basis of regres-
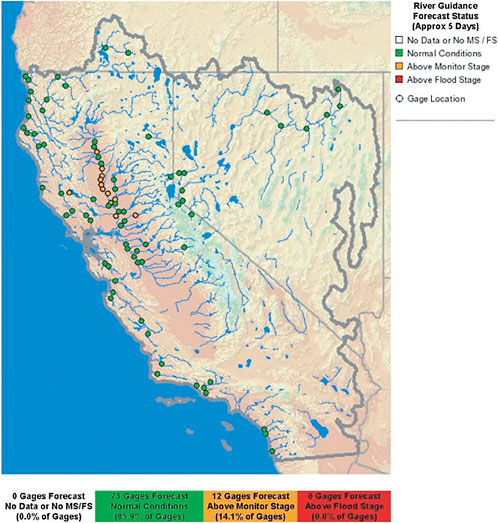
FIGURE 3.5 Example map with official river forecast points for the California Nevada River Forecast Center (CNRFC). The colors indicate the severity of river conditions and are updated in real time. SOURCE: CNRFC, http://www.cnrfc.noaa.gov.
sion equations as collaborative efforts of NWS with other agencies (e.g., National Resources Conservation Service and Bureau of Reclamation). These typically involve water supply volume regressions on several variables including snow pack information and seasonal forecasts of temperature and precipitation. The regressions provide a deterministic water supply volume (representing the 50 percent exceedance forecast). Uncertainty is produced by assuming a normal error distribution with parameters estimated from historical data. The resultant range of expected errors is adjusted to avoid negative values. Lack of representation of the skewed streamflows by this approach generates significant questions of reliability for the generated exceedance quantiles.24
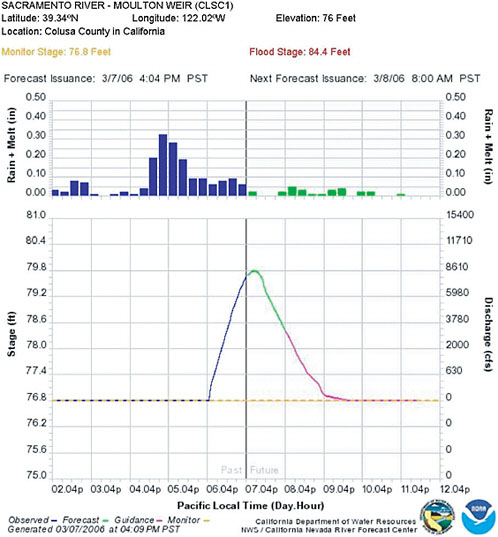
FIGURE 3.6 Example deterministic stream stage forecast from the CNRFC map of Figure 3.5. SOURCE: California Department of Water Resources/NWS California Nevada River Forecast Center.
Longer-term ensemble flow forecasts are also based on the ESP technique, which utilizes the RFC hydrologic models and historical 6-hourly mean areal precipitation and temperature time series (e.g., Smith et al., 1991). For a given date of forecast preparation time and for a given initial condition of model states, the ESP procedure feeds into the models historical time series of concurrent observed mean areal precipitation and temperature from all the previous historical years, extending to the duration of the maximum forecast lead time (a few months; Figure 3.7). For the river location of interest, the generated output flow time series forms the flow forecast ensemble, which is used to compute the likelihood of flood or drought occurrence and various other flow statistics.
BOX 3.8 Operational Hydrology Observations Onsite Data Onsite data are obtained from precipitation gauges of various kinds (weighing or tipping bucket, heated or not heated, shielded or not shielded), surface meteorological stations, soil moisture data, and stream stage or discharge stations. In all cases there is measurement error with uncertainty characteristics (a bias and random component) that must be considered during hydrologic model calibration (Finnerty et al., 1997). Not only is individual sensor error important (e.g., snowfall in windy situations), but interpolation error is also important for the production of catchment mean areal quantities for use by the hydrologic models. For stream discharge estimates, the conversion of commonly measured stage to discharge through the rating curve contributes to further observation uncertainty. Quantifying the latter uncertainty is particularly important for forecast systems that assimilate discharge measurements to correct model states. Remotely Sensed Data Weather radar systems are routinely used in conjunction with precipitation gauges for producing gridded precipitation products for operational hydrology applications. Satellite platforms are also routinely used for precipitation, snow cover extent, and land surface characterization. Although the information provided by these remote sensors is invaluable due to its spatially distributed nature over large regions, the data is not free from errors. Ground clutter, anomalous propagation, incomplete sampling, and nonstationary and inhomogeneous reflectivity versus rainfall relationships, to name a few, are sources of radar precipitation errors (Anagnostou and Krajewski, 1999). Indirect measurements of precipitation and land surface properties from multispectral geostationary satellite platform sensors are also responsible for significant errors in the estimates that are derived from the raw satellite data (Kuligowski, 2002). Challenges The main challenge for operational hydrology measurements remains the development of reliable and unbiased estimates of precipitation from a variety of remotely sensed and onsite data for hydrologic model calibration and for real-time flow forecasting. Measures of observational uncertainty in the final product are necessary (e.g., Jordan et al., 2003). A second challenge is to develop methodologies and procedures suitable for implementation in the operational environment that allow hydrologic models that have been calibrated using data (mainly precipitation) from a given set of sensors (e.g., in situ gauges) to be used for real-time flow forecasting using data from a different set of sensors (e.g., satellite and radar). Although bias removal of both datasets guarantees similar performance to first order, simulation and prediction of extremes requires similarity in higher moments of the data distributions. This challenge is particularly pressing after the deployment of the WSR-88D radars in the United States given the desirability to use radar data to feed operational flood prediction models with spatially distributed precipitation information. |
Flash-flood guidance is the volume of rainfall of a given duration over a given small catchment that is sufficient to cause minor flooding at the draining stream outlet. It is computed on the basis of geomorphological and statistical relationships and utilizes soil water deficits computed by the RFC operational hydrologic models (Carpenter et al., 1999; Georgakakos, 2006). Flash-flood guidance estimates may be compared to nowcasts and very-short-term precipitation forecasts of high spatial resolution over the catchment of interest to determine the likelihood of imminent flash-flood occurrence (Figure 3.8). Current implementation of these guidance estimates and their operational use is deterministic.
3.3.1.2
Types of Input Observations and Forecasts Used by Operational Hydrology
The production of streamflow forecasts in most RFCs requires 6-hourly areally averaged precipitation and temperature input as well as monthly or daily mean areal potential evapotranspiration input over hydrologic catchments (see Box 3.8 for a discussion of typical observations and their uncertainties). Although the specific procedures for producing these mean areal quantities vary among different RFCs, NCEP forecasts are used as input to numerical and conceptual procedures for the development of local and catchment-specific precipitation and temperature estimates (e.g., Charba, 1998; Maloney, 2002). Monthly and daily potential evapotranspiration estimates are produced on a climatological basis or daily using formulas that utilize observed or forecast surface weather variables (e.g., Farnsworth et al., 1982). For the production of ensemble streamflow forecasts, observations of mean areal precipitation and temperature, as well as monthly or daily estimated potential evapotranspiration are used in the ESP technique described earlier. Flash-flood guidance estimates require mainly operational estimates of soil water deficit produced
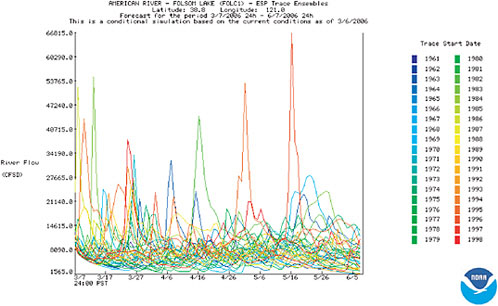
FIGURE 3.7 Example of ensemble streamflow prediction for Folsom Lake inflows in California produced by CNRFC. Precipitation data from historical years 1961 through 1998 are used to create this product. SOURCE: CNRFC, http://www.cnrfc.noaa.gov/ahps.php.
by the operational hydrologic models, and the production of flash-flood warnings on the basis of flash-flood guidance estimates requires spatially resolved mean areal or gridded precipitation nowcasts or very short forecasts (e.g., Warner et al., 2000; Yates et al., 2000).
3.3.1.3
Sources of Uncertainty in Input, Model Structure and Parameters, and Observations
Uncertainty in operational streamflow and river forecasts is due to errors in (a) the input time series or fields of surface precipitation, temperature, and potential evapotranspiration; (b) the operational hydrologic model structure and parameters; and (c) the observations of flow discharge, both for cases when state estimators use them to update model states and when such observations are used to calibrate the models (e.g., Kitanidis and Bras, 1980; NOAA, 1999, Duan et al., 2001). Errors in the input may be in (a) operational forecasts used in short-term flow forecasting, and (b) observations used in the ESP technique to produce longer-term ensemble flow forecasts. A primary source of uncertainty in short-term hydrologic forecasting is the use of quantitative precipitation forecasts (QPFs) for generating model mean areal precipitation input (Olson et al., 1995; Sokol, 2003). For elevations, latitudes, and seasons for which snowmelt contributes significantly to surface and subsurface runoff, surface temperature forecasts also contribute significant uncertainty to short-term river and streamflow forecasts (Hart et al., 2004; Taylor and Leslie, 2005).
3.3.1.4
Challenges
The NWS operational hydrology short-term forecast products carry uncertainty that is to a large degree due to forecasts of precipitation and temperature that serve as hydrologic model input and which are generated by objective or in some cases subjective procedures applied to the operational NCEP model forecasts. These procedures aim to remove biases in the forecast input to allow use of the operational hydrologic models, calibrated with observed historical data, in the production of unbiased operational streamflow forecasts. The challenges are to (1) develop quantitative models to describe the process of hydrologic model input development from NCEP products when a mix of objective and subjective (forecaster) methods are used (Murphy and Ye, 1990; Baars and Mass, 2005), and (2) characterize uncertainty in the hydrologic model input on the basis of the estimated NCEP model uncertainty under the various scenarios of QPF and surface temperature generation (Krzysztofowicz et al., 1993; Simpson et al., 2004).
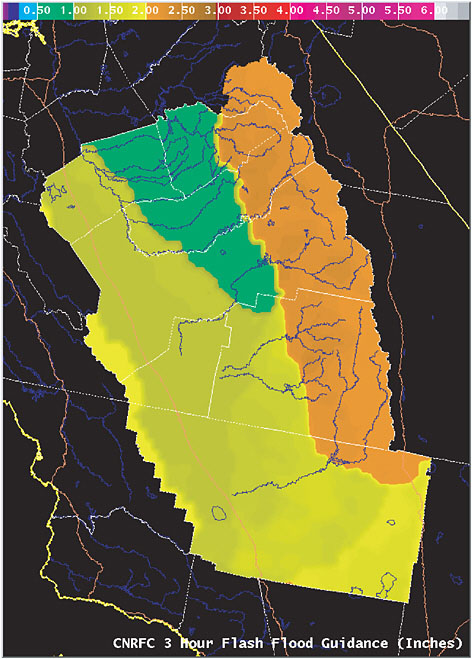
FIGURE 3.8 Areal flash-flood guidance (inches) of 3-hour duration for the San Joaquin Valley/Hanford, California. SOURCE: CNRFC.
The production of longer-term (out to a season or longer) streamflow forecasts is done on the basis of historical observed time series using the ESP technique. The challenge in this case is to develop objective procedures to use NCEP short-term and seasonal surface precipitation and temperature forecasts in conjunction with the ESP-type forecasts for longer-term ensemble flow predictions of higher skill and reliability (e.g., Georgakakos and Krzysztofowicz, 2001).
Prerequisites to successfully addressing the aforementioned challenges are the development of (1) adequate historical-forecast databases at NCEP to allow bias removal of the NCEP forecasts, and (2) automated ingest procedures
to allow direct use of NCEP products into objective hydrologic procedures designed to produce appropriate model input for operational hydrologic models.
Currently, there is a one-way coupling between meteorological and hydrologic forecasts in the operational environment. Another challenge is therefore to develop feedback procedures so that hydrologic model states, such as soil moisture and snow cover, may be used to better condition the meteorological models that provide short-term QPF and surface temperature forecasts (Cheng and Cotton, 2004).
Finding: The transition from deterministic to probabilistic/ ensemble hydrologic forecasting over broad time and small spatial scales will require a number of steps. Availability of data is an important step. In addition to the availability of reforecast products by NCEP as discussed earlier (see recommendation 3.5), it will be necessary to improve operational hydrology databases in the short term with respect to their range of time and space scales (e.g., temporal resolutions of hours for several decades and spatial resolution of a few tens of square kilometers for continental regions) and their content (e.g., provide measures of uncertainty as part of the database for the estimates of precipitation that are obtained by merging radar and rain gauge data).
Recommendation 3.12: The OHD should implement operational hydrology databases that span a large range of scales in space and time. The contribution of remotely sensed and onsite data and the associated error measures to the production of such databases should be delineated.
3.3.2
Operational Hydrologic Forecasts with Uncertainty Measures
3.3.2.1
Ensemble Prediction Methods
The Advanced Hydrologic Prediction Service (AHPS; McEnery et al., 2005) is perhaps the most important NWS national project that pertains to the development of explicit uncertainty measures for streamflow prediction. Short- to long-term forecasts by AHPS are addressed within a probabilistic context. Enhancements of the ESP system are planned to allow the use of weather and climate forecasts as input to the ESP system, and post-processing of the ensemble streamflow forecasts is advocated to adjust for hydrologic model prediction bias. In addition, AHPS advocates the development of suitable validation methods for ensemble forecasts. This requires historical databases of consistent forcing for long enough periods to allow assessment of performance not only in the mean but also for flooding and drought extremes.
Real-time modifications of hydrologic model input or model states are a common mode of operations associated with a deterministic approach to hydrologic forecasting. A fully probabilistic system such as AHPS, which includes validation and model-input bias adjustment, will enable the elimination of this type of real-time modification so that the model state probability distribution evolves without discontinuities and remains always a function of the model elements. The probability distribution of the model state is the new state of the fully probabilistic system, and any changes (including forecaster changes) in real time must result in temporal evolution consistent with probability theory and Bayes theorem (such as when assimilation of real-time discharge measurements is accomplished with extended Kalman filtering).
The evolution of AHPS represents a positive development toward the shift from deterministic to probabilistic forecasting in operational hydrology. As is often the case when first steps are taken to infuse science into operational methods and techniques, the details of how to go about doing this are matters of scientific debate. Such debate could be promoted by the NWS’s AHPS team by encouraging participation of the academic and research community in workshops targeting specific methods of uncertainty analysis and probabilistic prediction. In addition, and specifically for (1) designing the new AHPS products to be useful for users and (2) developing appropriate performance measures for validation of probabilistic and ensemble products, the NWS could encourage contributions to AHPS design from potential product users and analysts who are concerned with decision making under uncertainty. Demonstration testbeds with the participation of forecasters and managers addressing concerns of specific hydrologic forecast users (e.g., reservoir managers, irrigation districts) are a feasible and direct way to accomplish this goal (see also recommendation 3.7).
Finding: Developing and testing alternatives to AHPS components (e.g., downscaling of surface meteorological forcing for hydrological models, manner of incorporating model structure and parameter uncertainty in the ensemble streamflow predictions, assimilation of observations) will require participation from across the Enterprise. In the short term, workshops organized by NWS and with wide participation would be necessary to implement this process.
Recommendation 3.13: The OHD should organize workshops with participation from all sectors of the Enterprise to design alternatives to the AHPS ensemble prediction system components and develop plans for intercomparisons through retrospective studies, demonstration with operational data, and validation, and for participation in testbed demonstration experiments.
3.3.2.2
Limitations of Theory and Input Forecast Requirements for Successful Application
As operational hydrology transitions from deterministic to probabilistic/ensemble short-term hydrologic forecasting
it also transitions from larger to smaller spatial scales (down to the scales of flash-flood prediction). The latter transition makes estimation of the uncertainty in the hydrologic forecasts even more important as there is higher hydrologic simulation uncertainty at smaller scales (Smith et al., 2004). This, for instance, generates the immediate requirement for converting flash-flood guidance systems from deterministic to probabilistic systems. Successful application of ensemble streamflow predictions depends not only on the reliability of the forecasts but also on the manner with which these forecasts are made available (e.g., probability estimates versus an ensemble streamflow time series), communicated, and used by the decision maker or decision-support system (Georgakakos and Krzysztofowicz, 2001).
Ensemble streamflow forecast reliability depends critically on (1) the reliability of the meteorological model ensemble forecasts that are fed into the hydrologic model, (2) the manner with which short-term ensemble weather predictions are blended with longer-term (e.g., seasonal or longer) ensemble climate predictions to create seamless ensemble forcing for the hydrologic models, and (3) the development of reliable methods for model error contribution to the streamflow ensemble forecasts. As the operational hydrologic models transition from spatially lumped to spatially distributed formulations, these critical reliability prerequisites become increasingly difficult to meet with present-day observing networks and meteorological model resolution (e.g., Carpenter and Georgakakos, 2004; Ntelekos et al., 2006).
The issue of blending (point 2, above) is particularly challenging. This issue can be correctly resolved only if the same meteorological model structure and ensemble prediction methodology is used in short-term weather forecasts and long-term climate predictions, and the full range of products becomes available routinely by NCEP from both weather and climate model output.
Finding: Blending of short-term predictions with longer-term predictions to force hydrologic models is particularly difficult. This is an area in which hydrologists, as weather and climate forecast users, can provide significant input to meteorologists.
Recommendation 3.14: The OHD should develop methods for seamlessly blending short-term (weather) with longer-term (climate) ensemble predictions of meteorological forcing within the operational ensemble streamflow prediction system. This will require NCEP model output downscaling and bias adjustment, and real-time data availability.
3.4
SUBJECTIVELY CREATING UNCERTAINTY INFORMATION
Objective uncertainty information in the form of ensemble forecasts and statistical post-processing is made available as guidance for forecasters, but the majority of official probabilistic forecasts are human generated. This section covers some of NWS’s subjectively generated official uncertainty forecasts. One such approach and product was already discussed in the context of the CPC. Here, the discussion is extended to include the WFO25 and to briefly consider forecasts produced by the HPC,26 the SPC,27 and the Aviation Weather Center (AWC).28
Although objective uncertainty information is provided by the EMC ensemble systems and through MOS based on deterministic model predictions, human forecasters within NWS play a very important role in producing uncertainty information. Since 1965, NWS forecasters have produced subjective predictions of probability of precipitation for cities across the country. Such subjective uncertainty information is based on forecaster knowledge and experience informed by model output, observations, and statistical guidance such as MOS and local prediction aids. Several experimental studies have demonstrated that forecasters are able to produce reliable and accurate probability forecasts of a number of additional elements, such as temperature, humidity, tornadoes, winds (e.g., Murphy and Winkler, 1982; Brown and Murphy, 1987). However, NWS forecasters have not produced such forecasts operationally.
NWS staff also communicate the uncertainty in their forecasts through a variety of text discussion products. At the local level, each forecast office produces Area Forecast Discussions several times a day that analyze the weather situation and provide insights into the forecaster’s analysis, including his or her relative certainty regarding the forecast. At some NWS offices, short-range area forecast discussions are also created. Both the short- and longer-term forecast discussions play a critical role in providing the user community with a measure of forecast confidence. For most parameters, there is no other means of providing such uncertainty information. An additional way for forecasters to indicate a significant probability for severe weather events is through watches and advisories. Neither have specific probabilities associated with them, but the wording used may indicate levels of confidence.
The primary tool used by NWS forecasters to produce and distribute their forecasts is the Interactive Forecast Preparation System (IFPS). IFPS is the outcome of a recent paradigm shift in forecast preparation whereby NWS forecasters create graphic renditions of the weather out to 7 days that are dis-
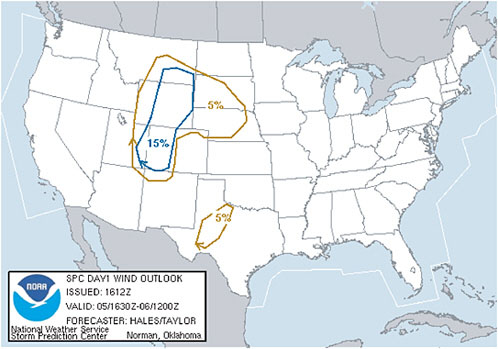
FIGURE 3.9 Probabilistic outlook for damaging winds. SOURCE: Storm Prediction Center.
tributed digitally as well as automatically translated into text. The digital forecast grids, known as the NDFD, represent an entirely deterministic, single-valued approach to prediction with the exception of 6- and 12-hour PoP. It is also possible for forecasters to pair probabilities of precipitation with major weather elements (e.g., thunderstorms, fog, and snow) to provide probabilities of such weather conditions.
On a national level, the HPC produces a variety of forecast discussions that communicate uncertainty, including a Short Range Public Forecast Discussion, an Extended Forecast Discussion, a Quantitative Precipitation Forecast Discussion, an Excessive Rainfall Discussion, a Heavy Snow Discussion, and others. They also produce graphical probabilistic surface low tracks, and the national PoP products as well as several winter weather products29 including snowfall probability and freezing rain probability.30
Forecasters at the SPC produce probabilistic 1- and 2-day outlooks of severe weather, including specific probabilistic forecasts for the occurrence of hail, tornadoes, and damaging winds (Figure 3.9). These outlooks are produced spatially, with specific definitions of the event being forecasted (i.e., the occurrence of the phenomenon within 25 nautical miles of any point within a contour) that are tied closely to the verification of the forecasts. In similarity with the subjective forecasts produced by WFO and HPC forecasters, the probabilistic outlooks are based on forecaster experience and knowledge, as well as the model guidance and other observations and tools. Watches and warnings issued by the SPC also may provide some information about the likelihood of severe weather, through wording of the discussions, but they do not explicitly include uncertainty information.
At the AWC forecasters produce 6-hour outlooks and short-term warning areas for phenomena affecting aviation safety and efficiency, including icing, turbulence, ceiling and visibility, and convection.31 Most of these products include wording that indicates likely changes in the situation with time through the outlook period but they do not indicate the actual likelihood of occurrence of the particular phenomenon. An exception to this is the Collaborative Convective Forecast Product, which shows areas of likely convective activity at projections of 2, 4, and 6 hours. This product
indicates both the expected areal coverage of convection and the forecaster’s confidence that convection will occur in the region identified. Although these parameters are not direct estimates of the probability of convection, they do provide an indication of the uncertainty associated with the occurrence and characteristics of future convective activity.
Although the production of subjective probabilities by NWS (and throughout the Enterprise) is limited, the importance of the human forecaster in the production of forecasts for end users cannot be underestimated. The availability of more and better probabilistic guidance products will lead to the production of improved and more extensive probabilistic forecasts by human forecasters.
3.5
VERIFICATION
All forecasts must be verified. Regardless of whether the forecast was produced by a numerical model, a post-processing procedure, or a human forecaster, verification provides information to users about the performance and uncertainty associated with the forecasts (to guide their use of the forecasts and as input to decision-support systems; see Chapter 2) and ultimately drives the development of a forecasting system. Verification also fosters intellectual honesty about forecasting capabilities: essentially, verification information provides base-level uncertainty information for any measured weather element. A number of types of verification approaches are needed to evaluate the quality of probabilistic and ensemble forecasting systems (Box 3.9), facilitate development of improved forecasting systems, and meet the specific needs of users.
Forecast verification is the process of evaluating the quality of forecasts. Verification includes the measurement of various attributes of forecasting performance, including such quantities as accuracy, skill, and reliability (Box 3.9). The availability of appropriate information about the quality of forecasts and forecasting systems is important for many purposes and for a variety of types of users. In particular, forecasters and forecast developers need information about forecast quality to help improve forecasts; program managers need verification information to monitor forecasting performance; and end users need verification information to make optimal use of forecasts. Although forecast quality, as measured through verification processes, is related to forecast value, quality and value are not the same thing— improvements in forecast quality may not always result in increases in value (Murphy, 1993). In general, a different set of methodologies, involving users and decision-making or econometric models, is required to estimate forecast value.
Nevertheless, forecast verification approaches can (and should) be designed to provide information about forecast quality that is relevant in the context of how users interpret and use forecasts. Two examples of ways verification information can be made more relevant are (1) the provision of verification information for weather conditions that are
BOX 3.9 Verification Measures and Approaches for Probability Forecasts Forecast verification involves the comparison of forecasts and observations using various approaches to evaluate the quality of the forecasts. The selection of an appropriate verification approach depends on the type of forecast as well as the purpose for the verification. For example, forecasts of rain/no rain require different verification approaches than forecasts of temperature; end users of forecasts require different information than managers. During the past half-century, specific methods and measures (e.g., the Brier score) have been developed to evaluate probabilistic forecasts. More recently, verification method development has begun to focus on the evaluation of forecasts of probability distributions, such as can be obtained from ensemble forecasts. Development of these approaches remains an area of active research. Examples of measures that can be applied for the evaluation of probabilistic and ensemble forecasts include the decomposed Brier score, the Ranked Probability Score (Wilks, 2006), the Continuous Ranked Probability Score (e.g., Hersbach, 2000), the ignorance score (Roulston and Smith, 2002), Relative Entropy, the Minimum Spanning Tree (Wilks, 2004), the Rank Histogram (Hamill, 2001; Talagrand et al., 1997), and the Relative Operating Characteristic (Mason, 1982; Jolliffe and Stephenson, 2003). Attributes of probability forecasts that must be considered in verification include accuracy, reliability (also called calibration), resolution, sharpness, discrimination, and skill. Similar attributes could be defined for forecasts of probability distributions. The two attributes calibration and sharpness are sometimes relied on to provide an overall assessment of the quality of probabilistic forecasts. Calibration measures whether, over a large set of events, individual probability values are equivalent to the relative frequency of occurrence of the event. For example, for a large subset of PoP forecasts in which the probability forecast is 0.25, precipitation would occur 25 percent of the time if the forecasts are calibrated. Sharpness represents the variability of the forecasts. A completely unsharp set of forecasts (e.g., based on the climatological probability) would have no variability (i.e., only one probability value would always be used). A completely sharp set of forecasts would use only the probability values 0 and 1. In general, sharp forecasts have a U-shaped frequency distribution, with the highest frequencies of use at the lower and upper ends—near 0 and 1. Discrimination measures the ability of the probability forecasts to correctly categorize the observed occurrence/nonoccurrence of the event. Skill measures how well a forecast performs relative to a naïve standard of comparison, such as climatology or persistence. Many of these attributes, approaches, and measures are explained on the Web site of the WMO WWRP/Working Group on Numerical Experimentation Joint Working Group on Verificationa and by Jolliffe and Stephenson (2003) and Wilks (2006). |
meaningful to users (e.g., for surface variables as opposed to 500-mb heights32) and (2) the use of diagnostic approaches as opposed to the summary scores that are commonly presented on NWS Web sites (e.g., components of the Brier score versus the overall Brier score). With respect to the first example, GMB assesses its ensemble products primarily (but not exclusively) against analyzed 500-mb heights. This approach naturally results in an ensemble system that shows steady improvement in its ability to probabilistically assess the height of the analyzed 500-mb surface. If one is interested in forecasting the 500-mb analysis then there is nothing wrong with this choice of assessment, but this goal may not be the most relevant for most users, and NWS is making implicit value judgments about what variables are important.
With regard to the second example, diagnostic verification approaches generally provide much broader information about forecast performance than can be obtained from a more traditional verification approach that focuses on single verification measures. The diagnostic approach considers verification from a “distributions oriented” statistical perspective. A basic principle of the diagnostic verification approach is that a variety of verification statistics is needed to provide meaningful information about the quality of any type of weather forecast and to meet the needs of the variety of users and purposes for verification. Clearly, no single measure can adequately represent all aspects of forecast performance for all users. Nevertheless, single measures are commonly presented for many variables (e.g., CPC monthly and seasonal outlooks).
The diagnostic verification approach is characterized by its incorporation of graphical methods, the stratification of verification results into meaningful subsets, and the use of relevant standards of comparison. Graphical approaches are an important component of diagnostic verification and can provide much more information about the distributions of errors than can ever be provided in single summary measures (e.g., Wilks, 2006). In addition, new research on spatial diagnostic verification approaches has led to new methods that provide more user-centric verification of spatial forecasts of variables such as precipitation. With respect to stratification, forecasts are grouped into meaningful subsets so that performance characteristics are not an artifact of combining forecasts too broadly—for example, across climatological zones or time periods. Finally, because verification is essentially a “relative” process, verification results are most meaningful when presented in comparison to the performance of a naïve “standard” (e.g., climatology or persistence).
NWS Instruction 10-160133 provides guidance on verification requirements for NWS offices and centers. This directive defines the verification measures that are required for public and fire forecasts, severe weather watches and warnings, marine forecasts, hydrologic forecasts, aviation forecasts, tropical cyclone forecasts, and climate forecasts. In general, the verification analyses that are publicly provided by NWS for many forecasts (e.g., CPC seasonal forecasts) rely on single summary measures of performance. Exceptions to this approach include the extensive diagnostic verification information provided by the SEC34 and some other centers and offices (e.g., MDL35). Thus, these verification approaches generally do not meet the need for diagnostic or user-focused verification.
Two other aspects of verification that are typically ignored are the nonindependence of nearby grid points and the uncertainty associated with the verification measures themselves. With regard to the first issue, ensemble (and other model) forecast verification efforts tend to treat model gridpoints independently.36 The implication is that the NWS goal is to produce unbiased, reliable probabilistic forecasts of individual weather elements at individual locations. This is a worthwhile effort, and with further development it would allow users to evaluate the probabilistic skill of global and mesoscale PDFs for different variables, locations, and time periods. But covarying uncertainty information can also be important to users. For example, if a hydrologist is concerned about flooding, the covariance of rainfall amounts becomes extremely important. There may be a chance of significant rainfall on opposite ends of a drainage basin, but does high rainfall on one end imply low rainfall on the other, does high rainfall on one end imply high rainfall on the other, or are the two truly independent? It is possible to imagine a forecasting system that is good for individual locations but poor when multiple locations are considered. An emphasis on improving the former (i.e., ignoring the covariances) likely requires a much different development path than an emphasis on improving the latter.
Comparisons of models and forecasting systems often are made using verification information based on simple scalar scores. In some cases these comparisons lead to choices among model or forecast characteristics and parameterizations. Thus the choice of verification measures and approaches is critical. To compound the difficulty in making such comparisons, verification measures and statistics themselves are uncertain. This uncertainty arises from several sources—observational error, sampling variability, and so on—but is almost always ignored in verification studies. To provide meaningful comparisons of probabilistic forecasting systems, this variability is explicitly considered through the use of statistical confidence intervals or hypothesis tests.
32 |
The geographic distribution of the height of the 500-mb-pressure surface above Earth’s surface. |
33 |
|
34 |
|
35 |
|
36 |
See, for example, http://www.emc.ncep.noaa.gov/gmb/ens/verif.html, http://www.emc.ncep.noaa.gov/mmb/SREF/VERIFICATION_32km/new_html/system_48km_30day.html, and http://bma.apl.washington.edu/verify.jsp. |
Diagnostic verification information can be considered base-level forecast uncertainty information. For example, a straightforward way for NWS to provide forecast uncertainty information is to augment existing forecast products with the error bars implied by historical verification statistics. This approach has been used effectively by the National Hurricane Center37 (Figure 1.6). The radius of the uncertainty circle at each lead is the average historical track error over the observational record. This product has (1) provided useful information to the public and the Enterprise, (2) stimulated research to provide improved uncertainty information, and (3) generated debate about what information the public and emergency managers require. A more sophisticated approach that utilizes ensemble information is given by the “dressing” technique described by Roulston and Smith (2003).
Finally, many of the issues considered in Chapter 4 regarding the communication of uncertainty information are relevant for the communication of verification information. Thus, careful consideration is needed for the way this information is presented to users. In addition to the verification measures and approaches considered here, it also is important that the components that went into the verification be made readily available to all forecast users (see Chapter 5, recommendation 6) to allow specialist users to perform their own post-processing and verification.
Finding: Verification drives forecast system development and affects the use of forecast information. By focusing on providing meaningful information to users about forecast quality and by being more explicit about its choice of verification measures for the forecast development process, NWS will enable open Enterprise debate about the choice of verification measures and the implied NWS role and values. Such debate will allow user interests to directly influence the development of NWS forecasting systems. Application of a broad set of diagnostic approaches, including new approaches developed through verification research, and incorporation of statistical standards (e.g., stratification into meaningful subsets, use of confidence intervals, comparison to a naïve standard) will allow the provision of information that is needed by a broad spectrum of users.
Recommendation 3.15: NWS should expand its verification systems for ensemble and other forecasts and make more explicit its choice of verification measures and rationale for those choices. Diagnostic and new verification approaches should be employed, and the verification should incorporate statistical standards such as stratification into homogeneous subgroups and estimation of uncertainty in verification measures. Verification information should be kept up to date and be easily accessible through the Web.
SUMMARY
In spite of the variety of time and space scales, the differences in quality of numerical models, the range of different forcings, and the assortment of phenomena under consideration, four themes emerge relating to estimation and validation of uncertainty of weather, climate, and hydrologic forecasts within NWS.
-
There is a need for the production of guidance databases that include raw and post-processed probabilistic information that can be interrogated by all users of hydrometeorological information, including NWS forecasters, the private sector, and members of the public. There is also a strong need for the construction and maintenance of databases of historical forecasts and the associated observations for the purpose of post-processing and verification.
-
Before such a database can be usefully constructed, improvements are needed in post-processing efforts for the production of objective probabilistic guidance for all parts of NWS.
-
An increased emphasis on verification is needed across all parts of NWS. A wide range of verification measures that are appropriately applied with a valid statistical basis are necessary to properly assess forecasts and provide meaningful information to users. In addition, diagnostic verification information provides a simple approach for adding uncertainty information to forecasts. Because the choice of verification drives forecast system development, verification measures should be carefully chosen.
-
The Enterprise, and in particular the academic community, is a vast resource that is underutilized by NWS. Testbeds are one way in which productive links can be forged among NWS, the academic and private-sector communities, and the users they serve, but only if sufficient emphasis is given and NWS buys into the testbed concept.