1
Introduction
All prediction, including weather, hydrologic, and climate forecasting, is uncertain. Although information about this uncertainty1 is potentially of great value to society, many users neither have access to it nor apply it. Such shortcomings will decrease as methods for estimating uncertainty are improved, as knowledge of the best approaches for communicating uncertainty is enhanced, as the user and forecasting community becomes better informed regarding the advantages of uncertainty information, and as means for disseminating uncertainty information are refined.
This study summarizes the current situation regarding the generation, communication, and use of uncertainty information in weather, seasonal climate, and hydrological forecasts (collectively, hydrometeorological2 forecasts) and makes recommendations for improvements. Specifically, the charge to the committee from the National Weather Service (NWS), which is part of the National Oceanic and Atmospheric Administration (NOAA), is to
-
Provide guidance on how to identify and characterize, and examples of, needs for uncertainty information among various users of forecasts, including the public, emergency managers and other government decision makers, and private-sector entities, both direct users and intermediaries.
-
Identify limitations in current methods for estimating and validating forecast uncertainty, relating these limitations to users’ needs and recommending improvements or new methods and approaches (with the goal of finding ways to better couple methods of estimating uncertainty to users’ needs).
-
Identify sources of misunderstanding in communicating forecast uncertainty, including vulnerabilities dependent on the means of communication used, and to recommend improvements in the ways used to communicate forecast uncertainty.
With respect to the general approach of this study, NWS asked the committee to recognize the diverse roles of participants in the weather and climate enterprise (Enterprise)3 and the diverse needs of forecast users. The Enterprise encompasses all sectors and parties engaged in generating and communicating hydrometeorological forecasts. It includes the public, private, and academic sectors, as well as user groups, such as segments of the media and risk management industry, who add value to products.4
The report addresses the first task in Chapter 2, the second task in Chapter 3, and the third task in Chapter 4. Each of these chapters includes detailed recommendations. In addition, a set of overarching recommendations is presented in Chapter 5. NWS requested that the committee’s recommendations focus primarily on the NWS mission, but it was agreed that this study could also address other components of NOAA or guide other relevant government agencies and sectors within the Enterprise. In cases where a recommendation states that “NWS should …” it is the committee’s
intention that the recommendation also applies to any relevant group or activity within NOAA, such as the Office of Oceanic and Atmospheric Research (OAR). Recognizing the breadth and depth of the task presented to the committee, NWS urged the committee to think in terms of “teaching us how to fish as opposed to giving us a fish.” The committee approached the task accordingly, and explains its approach to each task at the beginning of each chapter. The remainder of this first chapter summarizes the issues that are central to the committee’s charge, most of which are examined in more detail in subsequent chapters.
1.1
THE UNCERTAIN ATMOSPHERE AND HYDROSPHERE
Uncertainty in hydrometeorological predictions, often described in terms of probability (Box 1.1), varies by weather/climate situation, location, and length of forecast. As demonstrated in the seminal work of Lorenz (1963, 1965, 1968, 1969), the origins of this uncertainty include (1) lack of an accurate, complete description of the initial three-dimensional state of the atmosphere; (2) incomplete and inaccurate descriptions of physical processes and other inadequacies in the modeling systems; and (3) the chaotic character of the atmosphere, in which small uncertainties in the starting point of a forecast or in the forecasting system can result in large differences as the prediction unfolds. In addition to the inherent sources of uncertainty in the atmosphere, there are also uncertainties in Earth surface characteristics and fluxes that contribute to the overall uncertainty in hydrometeorological prediction (e.g., Beven, 1989).
Numerical prediction is the basis for most hydrometeorological forecasts beyond several hours. All numerical weather forecasts begin with a three-dimensional description of the atmosphere, known as an initialization. Such initializations have improved during the past several decades as more observing systems have become available and as data assimilation systems, which combine observations with previous forecasts to provide a coherent, three-dimensional description of the atmosphere, have improved. Nevertheless, all observations have errors, data assimilation systems have inadequacies, and even the improved observational networks have substantial gaps on all scales. The result is that the three-dimensional descriptions of the atmosphere, even from large centers such as NWS’s National Centers for Environmental Prediction (NCEP), are inevitably imperfect. Such imperfect initializations lead to errors and therefore uncertainty in the forecasts that increases with forecast projection forward in time.
Computer forecast models also possess other sources of error that result in degraded prediction. Some errors result from inadequacies in the model descriptions of the physics of the atmosphere, such as radiation, cloud physics, and surface drag. Other errors result from lack of sufficient horizontal and vertical resolution, the scales at which the numerical simulation can accurately forecast the evolving atmosphere and hydrosphere. Because computer resources are finite, there are always small scales that are not properly described. Additional errors accrue due to approximations in numerics, how the forecast models are integrated into the future. Still other errors arise because of fundamental uncertainties in boundary fluxes, especially surface fluxes such as latent and sensible heat fluxes, and surface properties. The results of model inadequacies are often apparent early in forecasts and generally increase in time, resulting in increasing uncertainty as the forecasts progress.
In summary, the chaotic character of the atmosphere, coupled with inevitable inadequacies in observation quality and data assimilation, model physics and numerics, boundary conditions, and model resolution, result in forecasts that always contain uncertainties that generally increase with forecast lead time and vary by the type of weather situation and location. Uncertainty is thus a fundamental characteristic of hydrometeorological prediction, and no forecast is complete without a description of its uncertainty.
1.2
THE EVOLUTION OF HYDROMETEOROLOGICAL UNCERTAINTY PREDICTION
Early forecasters, faced with large gaps in their nascent science, understood the uncertain nature of the hydrometeorological prediction process and were comfortable with expressing uncertainties in their forecasts. Cleveland Abbe (Figure 1.2), who organized the first American forecast group as part of the U.S. Army Signal Corps, did not use the term “forecast” for his first prediction in 1871, but rather employed the term “probabilities,” resulting in him being known as “Old Probabilities” or “Old Probs” (Box 1.2). A few years later, the term “indications” was substituted for probabilities and by 1889 the term “forecasts” received official sanction (Murphy, 1977).
As meteorology evolved during the late 19th and early 20th centuries into a more exact science based on explicit physical laws, the weather forecasting community increasingly presented deterministic5 predictions, with the uncertainties eventually succumbing to improving knowledge, technology, and observations (Murphy, 1977). The advent of numerical weather prediction around 1950 and its early successes strengthened this deterministic viewpoint. Forecast skill6 rapidly improved in the 1950s and 1960s as faster computers allowed higher spatial resolution and increasing sophistication in the numerical prediction models. But also during this period, the research of Lorenz (1963, 1965, 1968) and others demonstrated that forecast skill was inherently limited in a chaotic atmosphere in which small initialization
BOX 1.1 Interpretation of Probability This report has many references to the notion of probability. While often treated as a synonym for uncertainty, it is better described as one of many ways in which uncertainty can be expressed. The meaning of probability is an active area of debate. In spite of differences in its interpretation, probability thrives as a useful measure of uncertainty because the same calculus of probability—the ways in which probability distributions can be manipulated—applies to all of the definitions. De Elia and Laprise (2005a,b) describe different interpretations of probability and their implications in the context of hydrometeorological forecasting. These are the frequentist, subjective, and propensity interpretations of probability. In the frequentist interpretation, probability is defined as the limit, as the number of trials becomes arbitrarily large, of the number of times an event occurs divided by the number of opportunities for the event to occur. In frequentist theory, probability theory is legitimately applied only to phenomena for which such limiting frequencies are expected to exist. Frequentists use observed empirical frequencies from finite sequences to estimate the inherently unknowable limiting frequency. Such estimates are expected to become more accurate with larger numbers of trials. For example, consider the gambler who only trusts that a die is honest after counting the number of times the number two is rolled and dividing by the total number of rolls of the die. Another example is given by observational climatologies: the probability of exceeding a certain temperature threshold on a certain day can be obtained by counting the number of times that threshold was exceeded over the observational record for that day and dividing by the number of years of the observational record. In both cases, the probability calculated from past events is projected into the future and assumed to be valid. A strength of the frequentist approach is that it is unambiguous; two people analyzing the same dataset would produce the same estimates of probability values, but critics would claim that probabilities estimated in this way confuse the definition of probability with the measurement of probability. Another shortcoming of the frequentist approach is that it is not well suited for short records or extreme events (unless there is a large enough observational record). The notion of conditional probabilities (e.g., probability of temperatures in excess of 90ºF in the second week of May in El Niño years) effectively reduces the observational record, and indicates that there are many “types” of 90-degree days, each of which might have different probabilities. This is often called the reference class problem. The subjective interpretation of probability is an expression of the degree of belief that an event is going to occur. While useful for expressing uncertainty, the production of subjective estimates of probability is difficult, or impossible, to quantify. If a forecaster issues a subjective probability of a 30 percent chance of rain, how was 30 percent chosen over 25 or 35 percent? Subjective probability has been found to be a useful means of expressing uncertainty in a wide variety of applications, so regardless of issues associated with the production of subjective probabilities there are well-established methodologies for their assessment. A particular benefit of the subjective interpretation of probability is its ability to assign probability values to extreme events. An advocate of the propensity interpretation of probability would argue that the true probability density function (PDF; Figure 1.1) is available for any event, and forecasters and ensemble forecasting systems are striving to produce estimates of that PDF. In the context of hydrometeorological prediction, the argument would be that physical laws place constraints on the states that the weather, climate, and hydrosphere can realize, and a ![]() FIGURE 1.1 Example of a PDF (curve) that describes how the probability of some event varies as a function of some variable. In this case, the x-axis represents temperature at a location, and the y-axis the associated probability density. The probability of a particular range of temperatures is obtained by computing the area under the curve (the integral) for that range of temperatures. For example, the filled area indicates the probability of a temperature exceeding 76ºF. SOURCE: Committee on Estimating and Communicating Uncertainty in Weather and Climate Forecasts. |
complete knowledge of those laws would ultimately enable correct probability forecasts. This is analogous to the concept of attractors in nonlinear dynamical systems theory where the attracting set reflects an underlying PDF that describes the probability of finding the system state in a particular region of state space. A practical argument against the propensity interpretation in hydrometeorological forecasting is that the “true” probability is conditioned on the exact initial state of the atmosphere, ocean, land surface, sun, and all associated physical processes. It is effectively a function of the state of the universe and is therefore unknowable. A related argument would be that, in the same way that initial condition uncertainty renders the future deterministic state of the system unknowable, an imperfect knowledge or representation of the model that governs the hydrometeorological system renders the future PDF of the system unknowable, even if the initial PDF is correct. Effectively estimating and communicating uncertainty in hydrometeorological forecasts is not dependent on which of these interpretations of probability is used, and it is likely that all three can be applied to provide users with valuable information. For example, ensemble forecasting can be motivated by propensity ideas where scientists strive to make improvements to models and forecasting system components that drive objectively produced, ensemble-based forecast PDFs closer and closer to “true” PDFs. A measure of the quality of the ensemble forecasts is given by their comparison to frequentist climatological PDFs, and human forecasters use these objective PDFs as guidance for their own subjective estimates of probability. Users can also utilize the different forms as well as combinations of uncertainty information to aid in their own decision making. |
or model errors would inevitably grow large. To deal with forecast uncertainty, Epstein (1969) suggested stochastic-dynamic forecasting, in which forecast errors are explicitly considered during model integration, but computer processing power was not sufficient to support this method at that time. Another approach, ensemble prediction, was proposed by Leith (1974), who suggested that prediction centers run a collection (ensemble) of forecasts, each starting from a different initial state. The variations in the resulting forecasts could be used to estimate the uncertainty of the prediction or produce probabilistic guidance. But even the ensemble approach was not tractable at that time due to limited computer resources. The 1970s and 1980s brought rapid improve
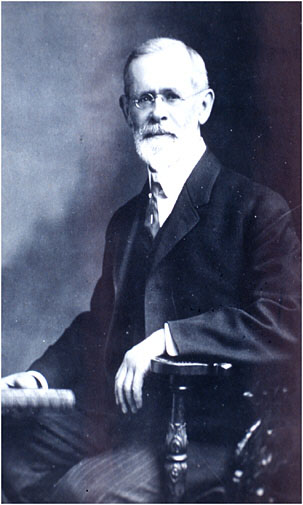
FIGURE 1.2 Professor Cleveland Abbe, who issued the first official “Weather Synopsis and Probabilities” on February 19, 1871. SOURCE: NOAA Photo Library, http://www.photolib.noaa.gov/people/images/big/pers0074.jpg.
ment in deterministic forecasts due to satellite observations, better numerical models, and rapidly increasing model resolution, further enhancing the dominance of a single-solution, deterministic approach to forecasting.
The first operational probabilistic forecasts in the United States were produced in 1965. These forecasts, for the probability of precipitation (PoP), were produced by human weather forecasters and thus were subjective predictions. The first objective probabilistic forecasts were produced as part of the Model Output Statistics (MOS) system that began in 1969. MOS made use of the historical performance of deterministic predictions to provide probabilistic predictions for parameters such as precipitation, precipitation type, and
BOX 1.2 Early History of Forecast Uncertainty Cleveland Abbe (“Old Probabilities”), who led the establishment of a weather forecasting division within the U.S. Army Signal Corps, produced the first known communication of a weather probability to users and the public. On May 7, 1869, Abbe proposed to the Cincinnati Chamber of Commerce that they “inaugurate such a system, by publishing in the daily papers, a weather bulletin, which shall give the probable state of the weather and river for Cincinnati and vicinity one or two days in advance.” Cleveland Abbe released the first public weather forecast on September 1, 1869. With a sense of history, he wrote to his father: “I have started that which the country will not willingly let die.” Following the signing by President Ulysses S. Grant of an authorization to establish a system of weather observations and warnings of approaching storms, on February 19, 1871, Abbe issued the first “official” public Weather Synopsis and Probabilities. An early example reports “Synopsis for past twenty-four hours; the barometric pressure had diminished in the southern and Gulf states this morning; it has remained nearly stationary on the Lakes. A decided diminution has appeared unannounced in Missouri accompanied with a rapid rise in the thermometer which is felt as far east as Cincinnati; the barometer in Missouri is about four-tenths of an inch lower than on Erie and on the Gulf. Fresh north and west winds are prevailing in the north; southerly winds in the south. Probabilities [emphasis added]; it is probable that the low pressure in Missouri will make itself felt decidedly tomorrow with northerly winds and clouds on the Lakes, and brisk southerly winds on the Gulf.” W. E. Cooke, a government meteorologist in Australia in the early part of the 20th century, was one of the first forecasters to advocate a numerical form for uncertainty information in weather forecasts. Cooke developed a numerical scale for presenting forecaster confidence that would “indicate, approximately, the weight or degree of probability which the forecaster himself attaches to that particular prediction” (Cooke, 1906). C. Hallenbeck of the U.S. Weather Bureau was the first to suggest the possibility of probabilistic forecasts of precipitation using a true numeric probability scale (Hallenbeck, 1920). Others in this period also experimented with objective methods for producing probability forecasts, such as through the use of scatterplots showing relationships between previous forecasts and observations (an earlier form of forecast verification). A. Ångström, who focused most of his career on studies of atmospheric radiation, also considered uncertainty in weather forecasting in papers published in 1919 and 1922. In particular Ångström identified sources of uncertainty in weather forecasts (e.g., incomplete observations) and advocated the combination of statistical methods and physics in producing forecasts. Angstrom was apparently the first to suggest the economic value of probabilistic forecasting, long before others made this connection (Liljas and Murphy, 1994). In 1944, G. Brier summarized the rationale for providing forecast uncertainty information to decision makers rather than implicitly making decisions for them by providing deterministic forecasts. This rationale was expounded by other researchers (e.g., Thompson, 1962) and proven analytically by Murphy (1977). |
thunderstorms. MOS predictions for most parameters (e.g., wind and temperature) have only provided single-value deterministic forecasts, even though the method is capable of providing uncertainty information.
By the early 1990s, ensemble forecasting became a practical approach to objective estimation of weather forecast uncertainty. Faster computers allowed the initiation of global ensemble prediction at major operational prediction centers such as NCEP, the European Centre for Medium-Range Weather Forecasts, and the Canadian Meteorological Centre. During the past decade the size and sophistication of the global ensemble systems have grown considerably, with medium-range, global ensemble prediction becoming an integral tool for many forecasters. Also during this period, NCEP constructed a higher resolution, short-range ensemble forecast system. High-resolution ensemble systems have also been tested at several universities and government laboratories (Grimit and Mass, 2002; Colle et al., 2003a,b).
NWS began issuing seasonal (30- and 90-day) temperature and precipitation forecasts each month in the late 1950s. These forecasts were developed using historical analogs. They identified areas with a greater chance of experiencing above- and below-average conditions. In the mid-1990s, NWS’s Climate Prediction Center (CPC) began issuing overlapping temperature and precipitation “outlooks” for each month up to a year ahead. Several different methods (e.g., dynamical models, analogs, cannonical correlations with El Niño/Southern Oscillation) are used to determine the probability of experiencing above-, below-, and near-average conditions. CPC continues to adjust the outlook product and has developed additional products thought to be useful to weather-sensitive decision makers.
The generation of uncertainty measures for hydrologic forecasts has also long been recognized as an important component in weather-sensitive decision making (in particular, for those associated with water resources). An

FIGURE 1.3 An example (for Seattle, Washington) of a forecast produced by the IFPS, NWS’s digital forecasting system, which provides deterministic predictions for temperature and other variables out seven days. SOURCE: NWS Seattle Forecast Office Web site.
ensemble-based technique was developed and operationalized during the late 1970s and early 1980s. This technique produced an ensemble of likely future flows on the basis of historical records of precipitation and temperature and current estimates of soil moisture and snow pack (Day, 1985). This approach only accounted for uncertainty in the meteorological input to hydrological models. Since the mid-1970s, hydrologists have used formal estimation theory to account for input, model, and observation errors to produce short-term flow forecast means and variances, and at the same time to assimilate observations of streamflow by the operational models for short-term forecasts (Kitanidis and Bras, 1980).
1.3
COMMUNICATION OF UNCERTAINTY INFORMATION
Hydrometeorological prediction is inherently uncertain and information about such uncertainty should be helpful to users in their decisions. Although some products contain uncertainty information (e.g., in those produced by the private sector for specialized users, and by the NWS in its Area Forecast Discussion and Probability of Precipitation predictions), relatively little headway has been made in supplying actionable uncertainty information to most of the user community.
As an example, the NWS meteorological preparation and communication system (the Interactive Forecast Preparation System, or IFPS) is inherently deterministic. Forecasters prepare graphical descriptions of the state of the atmosphere out as far as seven days into the future, with no uncertainty information or probabilities except for precipitation. The output from the IFPS system (known as the National Digital Forecast Database) is used to provide site-specific forecasts out seven days in graphical and text formats that are single-valued for nearly all parameters (see Figure 1.3).
NWS is not alone in providing such single-valued forecasts, both in the short and extended (3- to 10-day) ranges. Many private-sector firms follow a similar deterministic approach, and their forecasts are provided to the public through new and traditional media outlets. For example, local television news broadcasts, in which the weather is often the lead story, compete to provide single-valued projections as far out as two weeks (Figure 1.4) even though uncertainties typically increase with time and are large beyond a few days. Such single-valued forecasts are a mainstay of the international media and many users have adjusted their decision processes to accommodate these simplified predictions. Even though such deterministic forecasts are without scientific basis and suggest a level of forecasting skill that does not
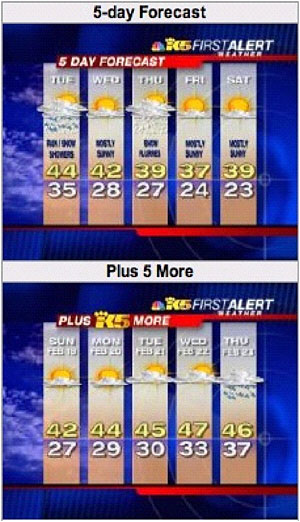
FIGURE 1.4 A 10-day deterministic forecast from a local television station. The forecast implies certainty in long-range predictions. The length of the forecast is dictated by the station’s channel number (an initial five-day deterministic forecast, “Plus 5 More”), as a promotional tactic. SOURCE: KING5-TV, http://www.king5.com/weather/.
exist, media presentation of these forecasts has multiple drivers in addition to the science.7
Problems with the dominance of deterministic forecasting information are compounded by the poor communication of the limited uncertainty information that does exist. For example, PoP is communicated through icons that are unclear and sometimes inconsistent with their supporting text (e.g., Figure 1.5). Furthermore, a portion of the public is
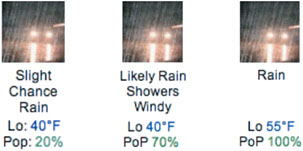
FIGURE 1.5 Inconsistent use of NWS icons used to communicate PoP. Note that the same symbol is used for probabilities ranging from 20 to 100 percent. SOURCE: NWS Web sites.
uncertain regarding the interpretation of precipitation probabilities (see Section 2.2.2.1). Does a 50 percent probability mean half the area will get wet, or it will rain for half of the day, or there is a 50 percent chance it will rain at any single location within the forecast area during some period (the correct choice)?
One of the few attempts at communicating uncertainty for an important parameter other than precipitation has been the recent introduction of the “cone of uncertainty” diagram used for hurricane track prediction, with the width of the path representing the uncertainty in the track forecast (Figure 1.6). However, the existence of a central line in some of these forecast products, indicating the most probable path, may detract from the effectiveness of the graphic, because many users think that the forecast has failed whenever the observed track deviates from the central line. Such a situation occurred in 2004 when Hurricane Charley, for which the central line crossed the coast near Tampa, Florida, made landfall to the south at Punta Gorda, Florida, which was approximately 40 miles east of the centerline track. Both locations were within the forecast track and were under a hurricane warning. Underlying these and other communication problems is that the Enterprise has conducted little formal research on how to effectively communicate uncertainty information to its users.
1.4
REASONS FOR SUPPLYING UNCERTAINTY INFORMATION
The American Meteorological Society’s “Statement on Enhancing Weather Information with Probability Forecasts” (AMS, 2002) noted that the current deterministic approach to weather prediction and communication has resulted in “much of the informational content of meteorological data, models, techniques and forecaster thought processes not being conveyed to the users of weather forecasts. Making and disseminating forecasts in probabilistic terms would
7 |
See Chapter 4. |
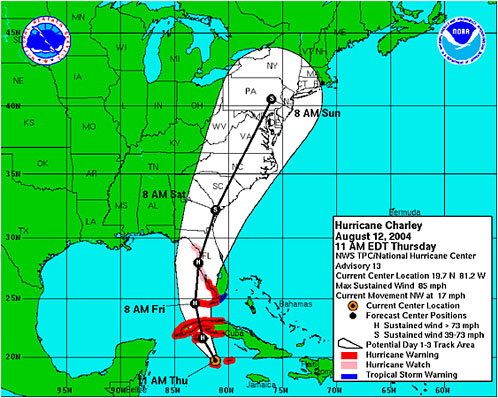
FIGURE 1.6 The three-day forecast of the track of Hurricane Charley about 28 hours prior to landfall near Punta Gorda, Florida. The cone of uncertainty is represented by the white shading, with the most probable track indicated by the black line. This product consistently showed that the ultimate landfall area was within the forecast cone of uncertainty. SOURCE: National Hurricane Center.
correct a major portion of this shortcoming.” By providing mainly single-valued categorical information, the hydrometeorological prediction community denies its users much of the value of the information it produces—information that could impart economic benefits and lead to greater safety and convenience for the nation.
There is an extensive literature documenting the potential socioeconomic value of uncertainty information over traditional deterministic forecasts (e.g., Katz and Murphy, 1997). Many of these studies consider simplified forecast-related decisions with a certain cost for protection and a specified loss if the event occurs without mitigation. For example, Murphy (1977) demonstrated that for such predictions reliable probabilistic forecasts always had a higher economic value than categorical forecasts or those based on climate averages, and that even unreliable uncertainty predictions reduce costs over categorical forecasts for most cost/loss ratios.8 Other studies have examined specific users’ decisions in greater detail. In aviation, for example, Keith (2003) demonstrated that the use of probabilistic predictions of visibility and cloud base afforded considerable economic benefit over the usual deterministic Terminal Aerodrome Forecasts. A retrospective study of management strategies for large reservoirs in California (WSAT, 2000) showed that using uncertainty information from a seasonal ensemble resulted in a 9.5 percent increase in annual hydroelectric revenue with a 40 percent reduction in wasteful spillage compared to using deterministic predictions.
The failure to provide forecast uncertainty information can contribute to damage and loss of life. For example, Pielke (1999) discussed how the lack of uncertainty information
in NWS forecasts dealing with the potential rise of the Red River in 1997 contributed to insufficient preparation by the population and public agencies. This catastrophic flooding event, which caused over a billion dollars of damage, was well within the typical error of the forecast river level. But such uncertainty information was not provided to the public or even other federal agencies.
With the availability of uncertainty information, users— each with their own sensitivity to costs and losses and with varying thresholds for taking protective action—could better decide for themselves whether to take action and the appropriate level of response to hydrometeorological situations. For example, current NWS wind predictions provide limited uncertainty information; consequently, for users who experience damage at 30 mph or more, a single-valued prediction of 25 mph or even a range of say 23 to 27 mph might not prompt any protective action. A probabilistic wind prediction, however, would likely indicate a modest probability that wind speeds could exceed 30 mph. Thus, the user with a low cost/loss ratio (low cost to protect or very expensive loss) might choose to use resources for protection in such a circumstance, whereas users with a high cost/loss ratio would likely do the opposite. In this way, probabilistic information can improve use of resources and enhance protection of life and property. More complex situations arise in the case of water resources facilities that serve many users and multiple objectives (e.g., water supply, ecosystem health, hydroelectric power production, flood control). In such cases, more elaborate decision-support systems may be necessary to guide decisions that result in substantially increased benefits for all stakeholders (Loucks, 1989).
In addition to socioeconomic value and scientific validity, a third reason for provision of uncertainty information is to retain user confidence. Forecasting a single atmospheric or hydrologic evolution when considerable uncertainty exists, without effectively communicating that uncertainty, inevitably undermines user confidence since there will always be significant and unavoidable forecast errors. If users knew that a range of occurrences was possible, the credibility of the forecasting community could be maintained since a probabilistic prediction system may have indicated a significant probability for the actual occurrence.
Finally, there is also an ethical dimension to the lack of uncertainty information in most hydrometeorological predictions. The Enterprise is providing many users with deterministic forecasts for a week and beyond, implying a level of forecast accuracy and skill that does not exist. Providing such single-value forecasts at any time range is deceptive and incompatible with the well-known state of the science, which acknowledges the inherent uncertainty in prediction. By comparison, in the medical arena probabilistic prognoses are commonplace as are the probabilities of cure with various therapies. As noted in recommendation 8 of the Fair Weather report (NRC, 2003a), NWS, as “the organization responsible for setting the scientific standard for operational meteorology” should “adopt and improve probabilistic methods for communicating uncertainties in the data and forecasts where such methods are accepted as scientifically valid.”
1.5
THE NEED FOR AN ENTERPRISE-WIDE RESPONSE
The shift of hydrometeorological prediction to a scientifically valid approach that fully considers, communicates, validates, and appropriately applies forecast uncertainty will demand the cooperation of the entire Enterprise. For NWS, this cooperation occurs in the context of NOAA’s partnership policy, which guides the agency in interactions with others in the Enterprise (Box 1.3).
Treating uncertainty as a fundamental characteristic of hydrometeorological predictions will require new links and feedbacks among the various sectors of the Enterprise. The academic, public, and private sectors will need to cooperate in estimating and studying uncertainty, understanding user needs and capabilities, generating new products that effectively communicate uncertainty, and developing new types of public outreach and educational and training programs to promote appropriate interpretation and use of these new forecasts. In addition, uncertainty-explicit forecasts will likely foster the emergence of new intermediaries from academia and the private sector to develop decision-support systems
BOX 1.3 NOAA Partnership Policy NOAA’s Policy on Partnerships in the Provision of Environmental Information (updated January 2006) states that “[t]he nation benefits from government information disseminated both by Federal agencies and by diverse nonfederal parties, including commercial and not-for-profit entities. NOAA recognizes cooperation, not competition, with private sector and academic and research entities best serves the public interest and best meets the varied needs of specific individuals, organizations, and economic entities. NOAA will take advantage of existing capabilities and services of commercial and academic sectors to support efficient performance of NOAA’s mission and avoid duplication and competition in areas not related to the NOAA mission. NOAA will give due consideration to these abilities and consider the effects of its decisions on the activities of these entities, in accordance with its responsibilities as an agency of the U.S. Government, to serve the public interest and advance the nation’s environmental information enterprise as a whole.” |
to enhance the utility of such forecasts. Such intermediaries would encourage new links and feedbacks between forecast producers and forecast users within the Enterprise. Last, the introduction of new uncertainty-explicit forecasts will generate the need for continuing validation, producing feedbacks from the forecast users to the forecast producers that may affect the manner of product generation and form of communication.
Although cooperation among sectors of the Enterprise would require some additional effort from all participants, several benefits are envisioned for each sector. NWS, through cooperation with academia and the research sector, gains access to state-of-the-science models, methods, and approaches, whereas through cooperation with the private sector and users it enhances the utility of forecast products, gains an understanding of the economic or market-driven forces of effective communication of uncertainty information, and receives feedback on forecast validation and user needs. The academic and research sectors gain access to NWS data and model resources for advancing research goals, receive feedback from NWS and the private sector for improving educational objectives, and develop new interdisciplinary areas of inquiry at the interface of physical, social, and behavioral sciences. For the private sector, close cooperation would facilitate the transfer of academic research to societal use and would ensure educator/researcher knowledge of the requirements of the workplace. Furthermore, the private sector gains access to validated operational predictions and data that may be used to generate value-added products tailored to user needs.
1.6
EDUCATION AS A CORNERSTONE OF THE TRANSITION TO A PROBABILISTIC VIEWPOINT
If the Enterprise is to make a successful transition from a predominantly deterministic mode of forecast generation and communication to one in which uncertainty information is an integral part of all products, substantial education and retraining of both users and providers of hydrometeorological predictions will be required. The university community will need to support changes in courses and curricula to ensure its graduates possess necessary knowledge of forecast uncertainty, methods for its generation, and the potential value of uncertainty information for meeting societal needs. NWS forecasters will need to master the underlying ideas of ensemble prediction and forecast uncertainty and will require retraining to deal with the new probabilistic forecast communication systems of the future. To facilitate such a transition NWS will need to establish new training materials, drawing on outside expertise in areas including the social sciences to create new tools such as educational modules for the Cooperative Program for Operational Meteorology, Education and Training dealing with forecast uncertainty. Similarly, the private sector will need to retrain its forecast personnel, both in the production and communication of uncertainty information. Reaching out to expertise beyond the traditional hydrometeorological community will increase the probabilities of success. Finally, the Enterprise will need to facilitate substantial outreach to the public and other users to acquaint them with the limitations of traditional single-value predictions and the considerable value of uncertainty information.
1.7
THE UNCERTAINTY IMPERATIVE
There is a confluence of compelling reasons for the Enterprise to transition to a new paradigm for hydrometeorological prediction, one in which uncertainty information is considered an integral and essential component of all forecasts. Prediction is inherently uncertain, and only by having access to actionable uncertainty information can users consider and apply the complete information required to make the best decision for their needs and situation. Fortunately, the demand for a transition to uncertainty communication is concurrent with an increasing ability to generate reliable uncertainty guidance.
The remainder of this report examines the psychological elements underlying use of uncertainty information (Chapter 2), reviews the strengths and weaknesses of the current operational systems for producing and verifying such forecasts (Chapter 3), and then evaluates current modes for communicating uncertainty information (Chapter 4). Based on this analysis, a series of overarching recommendations is presented in the final chapter. Actions in response to these recommendations will enable the transition to a new era in hydrometeorological prediction.