ATTACHMENT 4
DIAGNOSTIC CLASSIFIER—GAINING CONFIDENCE THROUGH VALIDATION
Weida Tong, Ph.D.
National Center for Toxicological Research
U.S. Food and Drug Administration
Jefferson, Arizona
INTRODUCTION
In clinical settings, accurate diagnosis and prognosis relies mainly on histopathology, cytomorphology, or immunophenotyping. Unfortunately, some diseases are hard to classify by current clinical techniques. To overcome the inherent limitations of traditional methods of diagnosis/prognosis, much attention has been recently placed on use of molecular profiles (e.g., gene expression patterns) derived from omics experiments for clinical application, such as DNA microarray and surface-enhanced laser desorption/ionization time-of-flight mass spectrometry (SELDI-TOF MS). It has been expected that recent technological advances in the fields of genomics, proteomics and other omics will offer a unique opportunity for not only improving diagnostic classification, treatment selection and prognostic assessment but also for understanding the molecular basis of health and disease. Classification methods, because of their power to unravel patterns in biologically complex data, have become one of the most important bioinformatics approaches investigated for use with omics data in clinical application. A number of classification methods have been applied to microarray gene expression data as well as other omics data. This presentation discusses the issues and challenges associated with application of classification using supervised learning methods on omics data applied to clinic. Specifically, a novel tree-based consensus method, decision forest (DF), will be discussed, which has been successfully used to develop diagnostic classifiers based on gene
expression patterns, SELDI-TOF MS data, and SNPs (single nucleotide polymorphisms) profiles in a case-control study. While the general procedure to validate a classifier will be discussed, the emphasis is manly placed on assessing prediction confidence and chance correlation, two critical aspects that, unfortunately, have not been extensively discussed in the field.
ISSUES, CHALLENGES, AND RECOMMENDATIONS
Developing classifiers from omics data is difficult because (1) there are many more predictor variables than the sample size (the number of subjects); (2) the sample size is often small with a skewed patient/healthy individual distribution; and (3) the signal/noise ratio in both clinical outcomes (dependent variables) and omics profiles (independent variables) are low.
Most molecular classification approaches reported in the literature have focused on developing and validating a single classifier. Although many successes have been demonstrated, the single classifier approach is inherently susceptible to the data quality and size; as the sample size and/or the signal/noise ratio of a data set decrease, the quality of a single classifier declines rapidly. Another aspect that is unique, or at least very significant, to molecular classification is that redundant information is normally present in an omics profile. The nature of the data reflects biologic phenomena where multiple molecular expression patterns are often equally important as biomarkers in diagnosis/prognosis. Unfortunately, a single classifier tends to optimize a single pattern for classification.
Consensus modeling, that combines multiple classifiers to reach a consensus conclusion, is theoretically less prone to data quality and size and more robust to handle an unbalanced data set. Most importantly, consensus modeling makes full use of the redundant information presented in omics data to explore all possible biomarkers. Thus, consensus modeling offers a unique opportunity in molecular classification.
The critical and implicit assumption in consensus modeling is that multiple classifiers will effectively identify and encode more aspects of the variable relationships than will a single classifier. The corollaries are that combining several identical classifiers produces no gain, and benefits of combining can only be realized if individual classifiers give different results. In other words, benefits of combining are only expected if separate classifiers encode differing aspects of disease-omics pattern associations. More recently, we also found that the information gained
from combining classifiers is valuable in assessing prediction confidence, which is usually difficult to obtain from a single classifier.
DECISION FOREST—A ROBUST CONSENSUS METHOD FOR DIAGNOSTIC CLASSIFICATION
Most consensus modeling relies on resampling approaches that use only a portion of the subjects for constructing the individual classifiers. Since we normally have a relatively small sample size, this approach will weaken individual classifiers’ predictive accuracy, which follows the reduction of the improvement in a combining system gained by the resampling approach.
A preferable consensus approach is to develop multiple classifiers using different sets of omics patterns. This approach takes full advantage of the available sample size as well as the redundant information presented in the data. Accordingly, we have developed the robust DF method (see Figure 4-1).
DF emphasizes the combining of heterogeneous yet comparable trees in order to better capture the association of omics profiles and disease outcomes. The heterogeneity requirement assures that each tree uniquely contributes to the combined prediction; whereas the quality comparability requirement assures that each tree equally contributes to the combined prediction. Since a certain degree of noise is always present in biologic data, optimizing a tree inherently risks over fitting the noise. DF attempts to minimize over fitting by maximizing the difference among individual trees.
There are three benefits associated with DF compared with other similar consensus modeling methods: (1) since the difference in individual trees is maximized, the best ensemble is usually realized by combining only a few trees (i.e., four or five), which consequentially reduces computational expense; (2) since DF is entirely reproducible, the disease-patterns associations are constant in their interpretability for biologic relevance; and (3) since all subjects are included in individual tree development, the information in the original data set is fully appreciated in the combining process.
For example, we develop a DF classifier on a proteomic data set to distinguish the prostate patients from healthy individuals. The data set consists of 326 samples, of which 167 samples are from the prostate cancer patients and the noncancer group contains 159 samples including both benign prostatic hyperplasia patients and healthy individuals. The
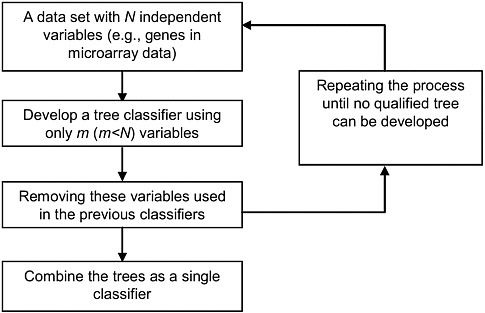
FIGURE 4-1 Overview of decision forest (DF). The individual tree classifiers are developed sequentially, where each tree uses a distinct set of variables. Classification (i.e., prediction) of an unknown subject is based on the mean results of all trees. Source: Tong et al. 2006. Reprinted with permission; copyright 2006, Toxicology Mechanisms and Methods.
classifier contains four trees, each of them having the comparable misclassifications ranging from 12 to 14 (3.7-4.3% error rate). The misclassification is significantly reduced as the number of trees to be combined increases to form a DF classifier (Figure 4-2). The four-tree DF classifier gave 100% classification accuracy.
VALIDATION 1: CROSS-VALIDATION VERSUS EXTERNAL VALIDATION
A classifier's predictive capability is commonly demonstrated using either external validation or cross-validation procedures. Although both procedures share many common features in principle, they are different in both ability and efficiency in assessing a classifier’s overall prediction accuracy, applicability domain, and chance correlation during implementation and execution.
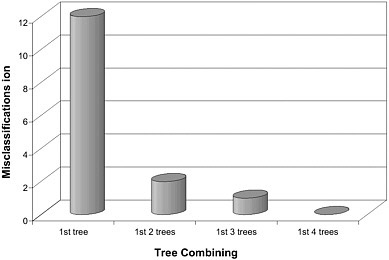
FIGURE 4-2 Plot of misclassifications versus the number of tree classifiers to be combined in DF. Source: Tong et al. 2004.
When sufficient subjects are available, a classifier should be validated by predicting subjects not used in the training set, but whose diagnostic outcomes are known (the test set). This external validation method lacks validity unless the test set is sufficiently large. Using a small number of subjects is inadequate for validation and also possibly wastes valuable data that otherwise could improve the overall quality of a classifier. Unfortunately, there is no consensus on how many subjects should be set aside to provide a valid validation.
A common practice for defining a test set in external validation is to randomly select a portion of subjects from an original population. From this perspective, cross-validation provides a similar validation for a given and fixed set of subjects. The 10-fold cross-validation procedure is commonly used to assess the predictive capability of a classifier. By comparing with external validation, cross-validation could provide a systematic measurement of a classifier’s performance without the loss of subjects set aside for testing.
It is necessary to point out that the cross-validation results vary for each run due to random partitioning of the data set, and thus we recommended repeating the cross-validation process many times (complete or extensive cross-validation). The average result of the multiple cross-validation runs provides an unbiased assessment of a classifier’s predictivity.
It is worthwhile to mention that classifier development and variable selection are integral in DF. Thus, DF avoids the selection bias during cross-validation that thereby provides a realistic assessment of the predictivity of a DF classifier. It is our experience that, unless the sample size is fairly large, given a constant set of subjects (a fixed data set with limited size), the cross-validation is more powerful for DF in measuring a classifier’s performance than external validation with respect to assessing overall prediction accuracy, prediction confidence and chance correlation.
VALIDATION 2: ASSESSING PREDICTION CONFIDENCE
A molecular classifier is a product of a mathematic correlation between dependent and independent variables. The classifier’s ability to predict an unknown sample is directly dependent on the nature of the training set. In other words, predictive accuracy for different subjects varies according to how well the training set represents the given samples. Thus, the concept of “prediction confidence” is viewed for measures of confidence in each prediction when the overall quality of a classifier is acceptable. It is critical to be able to estimate the degree of confidence for each prediction. The ability to quantify confidence greatly enhances the utility of any diagnostic classifier (to determine how best to apply the classifier).
External validation generally provides only overall quality assessment of a classifier with no indication of the confidence in individual prediction. In other words, external validation is of little value for assessing the prediction confidence which, in turn, can be readily available from cross-validation. In DF, the information derived from many runs of 10-fold cross-validation permits assessment of the prediction confidence.
Figure 4-3 gives an example illustrating how prediction accuracy and prediction confidence are related in DF. Prediction accuracy is plotted versus prediction confidence for both DF and decision tree (DT) for a problem using 2,000 runs of 10-fold cross-validation for a molecular classifier to predict prostate cancer from SELDI-TOF MS data (the same data set shown in Figure 4-2). A strong trend of increasing accuracy with increasing confidence is readily apparent for both DF and DT, as is the substantially higher accuracy for DF across almost the entire range of confidence levels. If we define high confidence predictions as those with confidence level >0.4 (Figure 4-3) and low confidence predictions as those with confidence level <0.4, we found that the high confidence prediction accuracy is ~99%, ~20% higher than the low confidence predic-
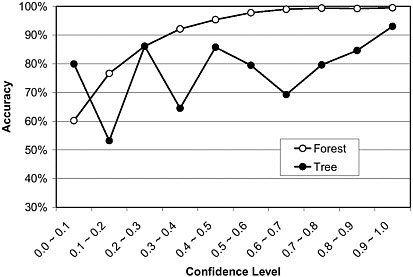
FIGURE 4-3 Prediction accuracy versus confidence level for a DF classifier of a proteomics data based on 2,000 runs of 10-fold cross-validation. The confidence level is defined as |Pi -0.5|/0.5, where Pi is the probability value for sample i. Source: Tong et al. 2004.
tion accuracy (~79%). The results demonstrate that the DF classifier gives a better assessment of prediction confidence than does the single tree classifier.
VALIDATION 3: DETERMINING CHANCE CORRELATION
Testing whether a classifier is, in fact, a chance correlation is highly recommended. Testing becomes increasingly imperative for smaller training sets with increasing numbers of independent variables, noise in biologic data, and unbalanced distribution of patient versus healthy individuals. All of these conditions increase the omnipresent risk of obtaining a chance correlation lacking predictive value.
Chance correlation is difficult to assess using external validation and is best obtained from cross-validation. To assess a degree of chance correlation for a DF classifier, we normally generate many pseudo data sets (e.g., 2,000 pseudo data sets) first using a randomization test, where the subject classification is randomly scrambled. Next, we apply a 10-fold cross-
validation on each of pseudo data sets to generate a null distribution, i.e., the distribution of prediction accuracy from all classifiers developed on all pseudo data sets. The null distribution can then be compared with the distribution of multiple 10-fold cross-validation results derived from the real data set. The degree of chance correlation in prediction can be estimated from the overlap of the two distributions.
Figure 4-4 shows the results of a test for chance correlation of a DF classifier to predict the prostate cancer. The distribution of prediction accuracy of the real data set centers around 95% while the pseudo data sets are near 50%. The real data set has a much narrower distribution compared to the pseudo data sets, indicating that the classifiers generated from the cross-validation procedure for the real data set give consistent and high prediction accuracy. In contrast, as expected, the prediction results for the pseudo data sets varied widely, implying a large variability of signal/noise ratio across these pseudo classifiers. Importantly, there is no overlap between two distributions, indicating that a statistically and biologically relevant DF classifier can be obtained using the real data set.
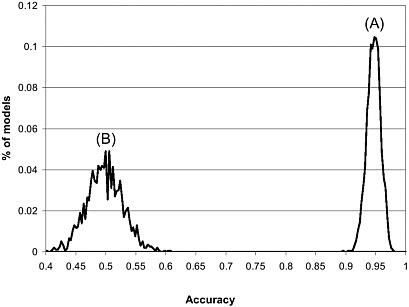
FIGURE 4-4 Prediction distribution in 2,000 runs of 10-fold cross-validation process: (A) real data set and (B) 2,000 pseudo data set generated from a randomization test. Source: Tong et al. 2004.
CONCLUSIONS
Diagnostic classification based on omics data presents challenges for most conventional supervised learning methods. Validation is a vital step towards the practical use of diagnostic classifiers. A classifier should be validated from three perspectives of assessment: (1) overall quality (prediction accuracy, sensitivity, and specificity); (2) prediction confidence; and (3) chance correlation. These can be more readily assessed in a consensus method, such as DF, than in other conventional methods using cross-validation. For DF, we have found that
-
Combining multiple valid tree classifiers that use unique sets of variables into a single decision function produces a higher quality classifier than individual trees.
-
The prediction confidence can be readily calculated.
-
Since the feature selection and classifier development are integrated in DF, cross-validation avoids selection bias and become a more useful means than external validation in assessing a DF classifier’s robustness and quality.
-
Carrying out many runs of cross-validation is computationally inexpensive, which provides an unbiased assessment of a classifier’s predictive capability, prediction confidence and chance correlation and facilitates identification of potential biomarkers.
REFERENCES
Tong, W., Q. Xie, H. Hong, H. Fang, L. Shi, R. Perkins, and E. Petricoin. 2004. Using Decision Forest to classy prostate samples based on SELDI-TOF MS data: Assessing prediction confidence and chance correlation. Environ. Health Perspect. 112(16):1622-1627.
Tong, W., H. Fang, Q. Xie, H. Hong, L. Shi, R. Perkins, U. Scherf, F. Goodsaid, and F. Frueh. 2006. Gaining confidence on molecular classification through consensus modeling and validation. Toxicol. Mech. Method. 16(2-3):59-68.