2
Model Design Options for Forecasting Systems
Your idea has to be original only in its adaptation to the problem you’re working on.
—Thomas Edison
In its first report (NRC, 2010), the committee outlined the principal attributes of a forecasting system (described in Chapter 1 of this report) and created a concept diagram (see Figure 1.2) for a forecasting system for disruptive technologies. In November 2009, at the 1-day Forecasting Future Disruptive Technologies Workshop convened by the committee, three subgroups of attendees successfully designed potential 1.0 version concepts for forecasting systems that would satisfy the parameters defined in the first report. Workshop participant Stan Vonog submitted a fourth forecasting concept at the end of the day.
Each subgroup was asked to prioritize a list of key design criteria and then to use the results as a basis for building a process diagram for a forecasting system showing the essential steps of how the system works. The key design criteria were these:
-
Openness
-
Persistence
-
Bias mitigation
-
Robust and dynamic structure
-
Anomaly detection
-
Ease of use
-
Strong visualization tools/graphical user interfaces
-
Controlled vocabulary
-
Incentives to participate
-
Reliable data construction and maintenance
Once the process diagrams were developed, the subgroups were asked to estimate a level of effort for technical and human resources. These estimates were discussed by all workshop participants and then incorporated into the model design. This chapter contains detailed descriptions of the four proposed system models:
-
Intelligence Cycle Option
-
Roadmapping Option
-
Crowdsourced Option
-
Storytelling Option
FIRST FORECASTING SYSTEM: INTELLIGENCE CYCLE OPTION
The name Intelligence Cycle Option was given to the system design that uses an option similar to the classic approach used by the intelligence community: hypothesize, task, collect, and analyze. This system starts with a “big question,” which initiates creative hypothesis generation fed by passive data collection (from movies, media, and online databases, for example) and active data gathering (e.g., crowdsourcing, games). The inputs then flow through hypothesis evaluation and testing by experts or participants in the science and technology, financial, and sociopolitical arenas or through mechanisms such as gaming and crowdsourcing. The raw output from these processes is shaped into a narrative1 for stakeholders and then fed back into the hypothesis engine. See Figure 2-1 for the option design from the workshop.
The system design was organized around four functions:
-
The input of a “big question,”
-
Signal identification and hypothesis generation,
-
Hypothesis evaluation and testing, and
-
The authoring of potential future narratives.
The Input of a Question
The forecasting process is initiated with a big-picture question posed by the stakeholder for a particular audience (i.e., Congress, the White House) and communicated to the system director/manager (see Table 2-1 for a detailed description of the different roles). Generating the big-question-based raw hypotheses requires an understanding of the forces that shape the perspective of the customer, and this is a key responsibility of the director/ manager, who is responsible for all interaction with the stakeholders. Throughout the development of the forecast, the stakeholder will receive feedback from the data, collection, hypothesis-generation, and hypothesis-evaluation processes and be able to assign parameters to ensure that the final narrative addresses the original purpose.
Another approach to generating the big question is to leverage outside experts or the crowd to suggest a list of big questions that stakeholders should consider. This list could be reduced through discussions with stakeholders to identify one or two big question(s) that should be addressed using this process. This approach is useful for finding questions that would not normally be generated by people inside a system, and it is a valuable way to avoid closed ignorance.2
Processes
The raw hypothesis based on the stakeholders’ big question is fed into an interconnected enterprise of passive and active data gathering, analysis, and hypothesis generation. The forecasting system’s hypothesis managers add a rough story and idea to the question and send the hypothesis to the passive and active analysis functions. Information is passively collected using software-centric textual and multimedia data mining and statistical analysis to identify applications, technologies, or ideas that have garnered increased interest, that cross subject areas, or cross regional boundaries. Inputs might include themes or ideas from U.S. and foreign movies and literature, electronic discussions of technologies and applications, cultural media, and volume and pricing of key technologies on eBay. Financial input might come from venture capital information sources or from internal agency sources of the government (e.g., the Federal Reserve, the Department of Energy’s Energy Information Administration, the Bureau of Labor Statistics).
Social and political input might come from U.S. and foreign government organizations, academic institu-
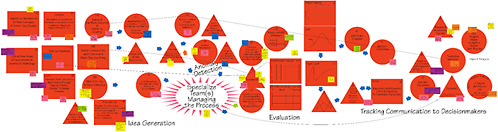
FIGURE 2-1 Intelligence Cycle Option. NOTE: The CD included in the back inside cover of this report has an enlargeable version of this figure, which is also reproduced in the PDF available at http://www.nap.edu/catalog.php?record_id=12834.
TABLE 2-1 Staff Required for the Function of the First Design Concept—Intelligence Cycle Option
Position Title |
Responsibility |
Desired Characteristics |
Number of Staff |
Forecasting system director/manager |
Interact with stakeholders |
Broad-thinking visionary with 10 to 20 years of experience in science and technology ventures or research and development. Prior government contracting experience a bonus. Should be pragmatic and goal-oriented. |
1 |
Hypothesis manager |
Flesh out hypotheses |
Good storytellers with training and experience in science and technology |
3-6 |
Passive data analyst |
Gather and analyze data from primarily online sources |
Strong information technology background with experience in search engine technology and statistics. Knowledge of algorithms for anomaly identification. |
|
Active data analyst |
Research, track, analyze, and synthesize data obtained from public data sources, subject-matter experts, U.S. government and potentially nonclassified data from intelligence community (IC) databases |
Training and experience in several areas of science and technology, military experience a plus. Should be able to recognize plausibility of technologies and applications. |
|
Group analysis facilitator |
Design and conduct live data-gathering activities using public data sources, subject-matter experts, U.S. government and potentially nonclassified data from IC databases |
Experience with groupware, social networking, and online gaming systems. Teaching or sociology background, with interest in science and technology. |
|
Alternate-future narrative producer |
Develop powerful narratives for delivery to final audience |
Experience in traditional written and slideshow presentations plus storyboard development and movie or theater production. Interested and well read in science and technology. |
2-4 |
tions, or other external activities such as gaming or crowdsourcing. Science fiction and futurist input might come from authors and online sources such as longbets.org3 and Technovelgy.com.4 Signals such as a change in money flows, or the sudden increase in purchases of thermo-cyclers indicative of the closing of national laboratories, or even the emergence of a new set of popular literary ideas might be used to develop existing working hypotheses or generate new raw hypotheses. Such a system can be built somewhat rapidly with a limited number of inputs to begin with and then scaled up by increasing the number and variety of information sources being mined as the forecasting system team deems necessary. Information is actively researched, analyzed, and synthesized to be fed into the raw hypothesis by forecasting system active data analysts, who research technologies, applications, and ideas from public data sources, subject-matter experts, the U.S. government, and potentially nonclassified data from intelligence community (IC) databases.
Active data analysts could also gather potentially valuable information through existing science-and-technology-focused organizations and groups that are designed to inspire the public to think of new ways of creating and adapting technology. Some examples of technology innovation competitions include the following: Imagine
3 |
For more information, see http://www.longbets.org/. Last accessed January 28, 2010. |
4 |
For more information, see http://www.technovelgy.com/. Last accessed January 28, 2010. 5-10 |
Cup, which gathers 300,000 students from around the world (http://imaginecup.com/); the International Genetically Engineered Machine (IGEM) competition, an international undergraduate competition (http://2009.igem.org/Main_Page); and the Discovery Channel and 3M Young Scientist Challenge for U.S. fifth- and eighth-grade school children (http://www.youngscientistchallenge.com).
Brick-and-mortar organizations and online organizations or groups dedicated to promoting access to science and technology resources and fostering innovation (i.e., such as the Tech Museum of Innovation [www.TheTech.org] and Do It Yourself Biology [DIYBio.org]) could provide information on innovative technologies, applications, and ideas as well as provide a pool of physical and online group participants for the forecasting system.
Similarly, intra-organizational challenges, such as military innovation challenges open to all ranks, are enterprises that could be tracked by the forecasting system for innovation data and the identification of current and future innovators. For hypotheses and scenarios that the stakeholder wants to control access to, gaming and crowdsourcing could be used within Department of Defense (DoD) or IC subcommittees (e.g., enlisted military personnel with a high school diploma). The members of this subgroup were strongly intent on avoiding biases held by the highly educated. They wanted recruitment efforts directed at both “experts” and those without college degrees.
The members of the subgroup discussed how the group participation exercises should be managed, how groups could be recruited, and what form the exercises might take. The group analysis facilitators are responsible for selecting participants to provide analysis that reduces the biases noted in the first report (i.e., multinational, multiethnic, and spanning socioeconomic, educational, and expertise levels). It was generally agreed that in order to reduce bias, forecasting system team input would be limited in the passive data collection and group analysis functions. However, as a result, both functions will require a large number and variety of inputs or participants. Suggestions for methods to attract participants ranged widely from a “honey pot” approach that attracts interest from the general population to a “lightning rod” that attracts idea-oriented people rather than filtering them from a larger group. The Technology, Entertainment, and Design (TED) Web site (www.TED.com) offers a good example of what this type of incentive would look like. The TED Web site broadcasts talks from stars in their fields, who are drawn to the opportunity to speak before a prestigious audience. One workshop participant suggested the Long Now Foundation online portal as a model for what he calls “gravity wells,” sites or organizations that draw people by offering the opportunity to network with important names.
When manually selecting group participants, it is easy to meet the requirements for reducing bias and to select individuals who are highly innovative or outside the norm in their field. However, dealing with self-selecting/ enrolling online systems attracting users who might represent a “disruptive” community involves the paradoxical task of eliciting participation from those who are, by definition, outside the norm and who may prove to be difficult to manage in a group exercise or may provide input that has little utility when held up against even the relaxed litmus of low probability but moderate or better plausibility. (See the discussion of desirable disruptive group participants in the subsection below.) Examples of group analysis activities identified by the committee include techcasting, crowdsourcing, alternative reality games (ARGs), sponsored innovation competitions, and round-table discussions (Halal, 2009).
While round-table discussions and innovation competitions are self-explanatory and involve person-to-person interactions, techcasting, crowdsourcing, and ARG can be implemented online. Techcast(ing), as presented to the committee by William E. Halal, is a forecasting methodology described briefly on TechCast, LLC’s, Web page:
[TechCast’s] researchers scan the literature and media, interview authorities, and draw on other sources to identify trends and other background data on roughly 70 emerging technologies. This data is summarized to guide the estimates of 100 technology officers, research scientists and engineers, scholars, and other experts. Results are aggregated to forecast the most likely year each breakthrough will occur, the potential economic demand, and confidence level. We find this method to be very powerful. It can forecast any issue, results are replicable within ±3 years, and the process enhances understanding. (Halal, 2009)
However, the committee noted that the system reports the statistical consensus or data clustered around the center of the bell curve of a normal distribution, which is problematic for two reasons: (1) It favors agreement and ignores potentially important disagreement represented by data at the tails of the curve, and (2) the system employs only highly educated or experienced subject-matter experts, which may adversely affect unconventional or innovative thinking.
The committee discussed the possibility of implementing variations of this method that would employ much more diverse groups to identify technologies and time lines and would report the data in the peak and tails of normal distributions, and non-normal distributions (i.e., bimodal and asymmetrical), all of which is believed to reduce bias in data analysis and to help with the identification of disruptive signals and signposts. Two group activities that were created specifically to decentralize analytical processes and leverage the participation of diverse populations are crowdsourcing and ARGs. It would be incumbent on the forecasting system group analysis facilitator to maintain his or her knowledge of existing and emerging group analysis methods and to evaluate their effectiveness when these methods are used for the forecasting system.
Desirable Disruptive Group Participants
The following are comments by the workshop participants and the committee members regarding desirable disruptive group participants.
-
“One of the things that I’ve noticed about disruptive technologies and people who are involved in it,” observed one participant, “is they’re disruptive, and that’s the nature of who they are.”
-
“If you have too much education, you learn too much about what’s not supposed to be and what you can’t do.”
-
“We want to talk to that 13-year-old who made it in his garage, not the 25-year-old graduate student well on his way to a Ph.D.”
-
“There’s something to be said about naïveté. And that’s the other people that are disruptive, that they don’t know that they can’t do that.”
-
“In the last five years, you’ve trained about five times more post-docs than the system could feasibly use. So you’re getting a bunch of post-docs who are no longer in the academic hierarchy but still love science and would like to stay active in some capacity.”
Once established, the forecasting system team may identify groups that might be considered unconventional but which, if accessed, could provide the system with valuable input (e.g., the Do It Yourself Biology community [DIYBio.org]).
As data are received and analyzed, the hypothesis managers collate the results and use them to create multiple hypotheses based on the initial raw hypothesis. The data and working hypotheses are provided to stakeholder(s), who can refine their original questions and raw hypotheses and feed them back into the system. One or more additional rounds of analysis of data and raw hypotheses to acquire more information of interest could occur, perhaps to reduce a suspected bias in a data set. For example, one focus of the additional analysis could be the posing of stakeholder questions to participants in different cultures to identify new trends, outliers, enthusiasts, or the convergence of multiple fields or money flows on a specific technology or idea, or to track the enhancers and inhibitors of technology diffusion rates. Alternatively, hypotheses might undergo another level of processing such as a technical evaluation, a prioritization of the scenarios with the greatest impact, or a policy evaluation step led by decision makers who actually allocate funds and affect policy (see Box 2-1 for dialogue from the workshop on crowdsourcing).
Hypothesis Evaluation and Testing
The quantitative analysis of hypotheses by human and software systems can use the same methods as the overall forecasting process—initiating with a question and then identifying signals and generating hypotheses; however, the scope would be pared down to focus on specific hypotheses. The goal of this process would be to analyze rather than to develop the hypotheses. As data are gathered, it is important to identify the signposts for following the progression of a potential disruption and to choose metrics that could be used to predict the timing of a disruption.
A standing committee of experts or dedicated outsourced group of analysts would coordinate the quantitative
evaluation of the hypotheses taking into account technology innovation, application innovation, and precursor events. The evaluations would also include the impact of the nationality, expertise level, and socioeconomic class of the hypothesized actors. This group would apply science, technology, science fiction, financial, social, and political filters to identify signals and signposts. Input for science and technology of the future might come from the generation most likely to create it—graduate or postdoctoral students—or the national laboratories, for example.
The outputs of the hypothesis evaluation and testing function are hypotheses with accompanying evaluations, which serve a dual purpose: to generate the final potential future narratives and to contribute further to the improvement of the hypothesis generation function.
Authoring of Potential Future Narratives
Narrative writers provide the final future narratives to the stakeholders (also known as customers) and/or the users, taking into account the quantitative narrative analysis in a ranking-type scheme, which will address normal and outlier data and give voice to both the consensus and the unorthodox opinions. The narrative writers could take into account science and technology, social, political, and financial rumors as well as science fiction and fantasy as they address plausibility, inhibitors and accelerators of progress, and convergence or divergence of applications. It is important to keep in mind that the stakeholders or customers (who pose the original questions to the system) and the audience (who might be asked to act on the results of a forecast) may be the same entity; or, the audience may be a separate entity that will receive a briefing from the stakeholder or the forecasting group for information and/or decision support. This process will also provide feedback to the hypothesis generation and hypothesis evaluation functions as well as identifying new big-picture questions for the stakeholders.
The format of the narratives needs to be very compelling or even provocative to have value and to motivate decision makers to take action before the actual event, attract participants to the system, and effectively communicate the data and analysis. Multimedia and video storytelling might make the most effective presentations of the scenarios. Scenarios could be presented as movie shorts or put on the Internet or turned into an ARG or simulation that people can play for several days. The predictive value of the narratives could be tracked using portfolio assessment tools to provide the organization and future stakeholders with a metric to reference for future big questions.
Forecasting System Attributes
The committee maintained, from the earliest meetings that it held during the course of this study through the workshop, that a persistent forecasting system must be quantitative, self-learning, and inclusive of the improbable technologies, imaginative applications of technologies, and the unconventional ideas and beliefs that spawn them. As discussed previously, software systems can apply statistical analysis and identify outliers in normal and non-normal data distributions. A further software solution to anomaly detection could be thought of as a continuous exceptions-analysis machine that is fed key words or phrases and returns the outliers, as opposed to Google’s algorithms that search for commonalities. Such automated approaches would certainly help to identify both the expected signals and signposts, which would be highly probable and plausible, as well as identify the anomalous signals and signposts, which would be plausible but of moderate to low probability. The manual application of anomaly detection and evaluation will be critical to the building and early analysis of working hypotheses and the evaluation of the final hypotheses, prior to narrative production. One workshop participant postulated that this is simply a matter of “cultivating a proper sense of the weird.” It was suggested that analysts leverage the Internet by choosing a network and pulling in group conversations, or monitoring existing conversations and asking questions to extract unusual threads. This skill set will need to be sought in forecasting system team members from the beginning and actively nurtured within the organization.
The self-learning aspect of the system is ensured by the design of the system, an interconnected group of functions that feed forward, sideways, and backward on the flowchart to allow for revision and refinement throughout the hypothesis generation and evaluation processes. Two other system elements are also critical to the learning system. The first is system scope—concurrent projects for one or more stakeholders will enhance the data collec-
BOX 2-1 Methods and Benefits of Crowdsourcing Following are quotations on methods and benefits of crowdsourcing from participants in the Forecasting Future Disruptive Technologies Workshop held November 5, 2009, in San Francisco.
“So in terms of what was being said about something provocative that evokes a response, it’s almost like the tool has got to have some mechanism to launch these little missiles out that engender that kind of bang, and develop it so it has a cadence over time so you’re constantly testing, bouncing an idea, getting that echo of a response. Because the response isn’t necessarily going to come at you immediately, sometimes it takes months. But it will come if you have the right content and message. “From the standpoint of communication and messaging and how you got the responses back, typically they’re latent. They’re there … just beneath the surface. And when you put something out there, people respond to it because it’s already on their mind, just right beneath their skin. It’s been bothering them for a while and then, boom, that was the trigger that got it out…. But those are trigger points on the edges that you want to be able to look at and say okay, who reacted to that and why?” |
tion, data/hypothesis analysis, and hypothesis generation functions of the forecasting system; the second is temporality—the continuous passive monitoring of previously identified signals and the periodic review of previous hypotheses and narratives as the system ages over time will enhance current and future projects.
Although not formally presented due to the myriad forms that it could take, the system will incorporate a quantitative ranking or scoring system that will be consistent throughout the forecasting system’s functional areas and will provide a numerical assignment of values to factors, such as probability and plausibility, for technologies, applications, ideas, hypotheses, and narrative outlines.
Forms of the Forecasting System
The workshop participants and the committee members discussed what forms the system organization might take and how it could be funded. The most immediately identified form is for an existing governmental or nongov-
|
ernmental organization to implement the forecasting system as a proprietary organization with proprietary software, which recruits and manages external participant groups solely for data and hypothesis evaluation.
A second proposed form would establish the forecasting system as a paid membership organization, open to individuals, private companies, nonprofit organizations, and governmental organizations with an interest in using a persistent forecasting system or becoming contributing members of the forecasting system’s analysis and evaluation functions. In such a system, the previously defined stakeholders/customers, analysts, and audiences can be considered “users” of the system, and some users can have multiple roles. Given the high degree of flexibility and interactivity in such an open forecasting system, it would be important for the director/manager to give guidance continually on the various functions of the staff and virtual team in asking the right questions and finding the best sources and resources to create useful answers.
A third proposed form would essentially operate a proprietary and an open forecasting system simultaneously
to serve both a proprietary community and the open-access community: that is, a proprietary secure DoD persistent forecasting system and a nonprofit open-access persistent forecasting community.
SECOND FORECASTING SYSTEM: ROADMAPPING OPTION
The second proposed system model—the Roadmapping Option—focuses on developing roadmaps from the present to predicted future scenarios based on collected data, including observations from communities of interest. The key to this model is the generation of signposts that can be monitored as the roadmap progresses. The elements of the system are these:
-
Idea generation;
-
Techniques for mapping, processing, and evaluating inputs; and
-
Communication to decision makers.
This design starts with the selection of existing communities that are experimenting to solve problems. The subgroup decided that for the 1.0 version, a limited number of communities of interest should be monitored in order to gain an understanding of their activities. These communities of interest should have a lot of activity and resources—both human and capital—behind them. See Figure 2-2 for the actual design work from the workshop.
Ideas collected from these communities and using other traditional data-gathering techniques are used to develop future scenarios to be explored. The collected ideas then need to be filtered and spun into narratives. The predictions or hypotheses generated from the narratives are then correlated with current events, mapped to current trends and models, and explored by different communities to test their validity. Refined hypotheses or narratives are analyzed to determine the impacts of and paths to their realization. Experts backcast by predicting, based on a forecasted future event, a roadmap of how that event might occur, including the signposts and signals that indicate progress. The forecasting system then searches for signposts and signals that correlate with scenarios. As data accumulate and correspond to the narratives, some emerge as more relevant than others. Ultimately, the signals, signs, scenarios, and their impacts are reported to decision makers. Because this reporting needs to be useful and understandable from a personal perspective, it should emphasize the impacts that each scenario will have on individual lives. All of the final output is used to create new input and ideas for the system.
Generating Ideas
In this system, the initiating process consists of picking communities of interest, watching what members are doing, and generating ideas about future developments based on those activities. For the forecasting system, the existence of a community of interest is needed to make an idea plausible. The team actively observes the selected communities to gather four categories of data: topics about which there is deep uncertainty (the unknown unknown), topics that are known to exist but about which not much is known (the known unknown), topics that are well understood (the known known), and topics that represent overlooked knowledge (the unknown knowns; see Table 2-2).
In the category of the unknown unknown, data-gathering activities might focus on technology experimentation and themes from science fiction to generate theories and test their viability. In the category of known knowns, looking for the rapid adoption of well-understood technologies in new emerging markets or the application of reverse innovation could indicate a disruption is about to take place (Bhan, 2010; Govindarajan, 2009). In the category of the known unknown, interest-based communities are the key. The team continuously identifies unknown topics and casts a net for more information on those topics, seeking individuals and communities that hold the missing knowledge. The more open and broad the reach of the system, the more robust will be its ability to catch needed information. Part of the challenge in this task is reaching constrained communities in remote areas. For example, one group member suggested that cellular telephone technology might be used to access isolated communities that do not have access to the World Wide Web for surveys and data collection.
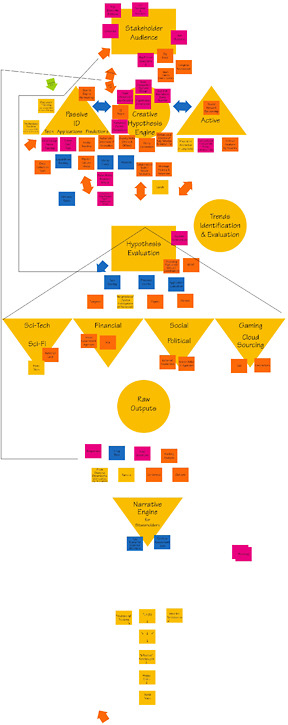
FIGURE 2-2 Roadmapping Option. NOTE: The CD included in the back inside cover of this report has an enlargeable version of this figure, which is also reproduced in the PDF available at http://www.nap.edu/catalog.php?record_id=12834.
TABLE 2-2 Categories of Information for Data Gathering for the Roadmapping Option
Unknown |
unknown knowns (overlooked knowledge) |
unknown unknowns (deep uncertainty) |
Known |
known knowns (mastered futures) |
known unknowns (research priorities) |
|
Known |
Unknown |
The inputs that come back are refined and qualified, shaped or formed by the team. At this point the team’s most important role begins: that of quantifying data and ensuring that fundamental principles are observed and that assumptions have not changed. Validated pieces of information make up clues that contribute to the whole, which grows and adjusts as data are added. Traditional surveys of communities are one way to quantify the data. In the process of harvesting, data mining, and brainstorming, there is also an iterative process of experimentation in the selected communities to realize the team’s hypothetical visions and test whether or not they meet the communities’ needs. If they do, the scenario building continues. If they do not, the scenario might be adjusted or rethought or matched to address different community issues.
The types of signals collected will vary by community. In countries, signals can be found in political rhetoric. In companies, the system might look for a corporate culture expressed in slogans, information on how the people in the workforce treat one another, what is important to them, what they value, how they speak. Semantic analysis and natural language processing technologies are being developed to assist in the evaluation of text and speech, and they could be used to help identify interesting problems that might merit an extra expenditure of resources. These emerging technologies are currently being used by commercial companies to analyze language patterns in order to obtain insights into the ways that people and communities communicate and operate. Blogs can be searched for certain themes that people are asking about or problems that are being addressed. The system team studies conditions that allow innovators to arise—root causes and conditions that allow innovators to be successful or that spur communities to act. There might be thresholds at which something becomes unacceptable and spurs action. Monitoring to detect anomalies would be based on defined thresholds. An estimate of a threshold is a judgment that could be dynamically changing. These thresholds would be very dependent on culture. Another spur to action is people seeing something that works in solving some part of their issues and innovating from there. The team observes communities to understand their issues and what they are working on. What are the problem spaces and the opportunity spaces that naturally incentivize innovation?
The system team could employ classic techniques such as brainstorming, Web crawling, and market surveys as well as classic methods with a twist. For example, the workshop group discussed using prediction markets, not for predictions, but to evaluate and analyze signposts—when a signpost might hit, or the probability of a signpost becoming real. Information toward predictions can be extracted from and correlated with data from games, tweets, news, blogs, paper abstracts, articles, book summaries, and comments on the rest. The correlating of data results in an evaluation of the words, a list of words, and a ranking of relevance to track.
The team can also actively solicit or generate ideas with activities such as a worldwide online contest with
weekly winners. The audience might be Hollywood, Bollywood, Sand Hill Road,5 or the intelligence community. The team might sponsor gaming, in which participants play in virtual worlds based on proposed scenarios. To brainstorm ideas, the team might go to specific communities—random communities, expert communities, or enthusiast communities involving a wide range of volunteers—to brainstorm by means of e-mail technology to get and seed succinct ideas. The system team integrates the ideas and other data into multiple narratives.
Most of the techniques for collecting data can be done in parallel or simultaneously. Both human and automated systems can perform the calculation and extract the signals and signposts for a potential future.
The system can automatically data mine the Internet for new concepts and terms; search tag clouds; harvest social networks; and look at summaries, such as those found on Amazon, to extract classes and themes from books, television, movies, and games. There are specific models of technical evolution that should be correlated against the ideas being generated and the signals that are observed. The searches will look for technology as well as human conditions and intersecting plots. The system’s comparative engine, for example, surveys different media automatically and notes changes.
It will require human work to do the creative envisioning. A technique for extending the breadth and reach of the ideas being collected is to take an observed trend and to extend it to an irrational extreme or to assume the opposite: that the trend (for example, the concentration of population into urban areas) reverses. Then take that trend in the opposite direction, to the opposite extreme. This technique is similar to the “what if” exercise that inspires science fiction, and filtering ideas from the corpus of science fiction can contribute to generating ideas. The purpose is to generate the outliers, the scenarios on the fringe.
Another possible technique for identifying extremes is to ask the communities of interest two simple questions: What is the thing that worries you the most? and What is the thing that you would really like to see happen? The survey responses would also identify underlying differences in values and worldview. If segmented by region, the metadata would capture and allow an understanding of regional biases—cultural, economic, technological, and so on. There might be differentiation between signals from a government leader and an ordinary citizen, but the vision could come from anyone. When Mahatma Gandhi rose to prominence in India, it changed the country and the world. It is useful to segment all the data and track demographic change.
From any point of entry, the system team can ask what the best-case scenario vision is and what the worst nightmare is. Once a future vision or narrative of interest is identified, the team or experts build maps that go to that place. From the roadmaps, signals and signposts, like road signs along the way, are identified. This is the map of the potential future against which the system matches data signals from its tracking and monitoring. At the workshop, Committee Chair Gilman G. Louie observed, “Mapping is important because you want to be able to touch each one of those [narrative] lines and whatever your impulse is—whether you’re following experimentation in communities or science fiction writing and possibly literature or funded research or venture capital, it should be addressing forecast signals and signposts.”
One way of developing a roadmap is to take an alternative future and let the experts draw a map of how to get there. They might start with a roadmap that assumes no “miracles” but simply extrapolates from current trends, from what is known. Layered on top of that could be a look at what happens if there is a miracle. How would that event disrupt the pathway to get to that alternative future? Would it speed it up? Block it? Take it down another path? In the end, the roadmap has many roads and signals and signposts on the map. The roads or paths themselves do not need to be analyzed. What is important is the signals that indicate which road is being taken. The analysis of potential scenarios and a breakdown into signs and signals are critical to this forecast system. Signals are so important that they should be rewarded. Any input that delivers a useful signal should be encouraged.
Evaluation Techniques
The techniques for idea generation—automated as well as active—can generate numerous ideas from high-speed processes. The group raised the issue of whether the evaluative mechanisms, which might be labor-intensive,
could scale up to the speed of idea generation. Also discussed was the issue of processing and evaluating inputs, including weak signals, so as to filter data that are not relevant, identify significance, and find even the weak relevant signals without the system’s getting overwhelmed.
Roadmapping is key. If the roadmap to a prediction is structured correctly, there are conditions, thresholds, signals, and signposts that have to be met to proceed toward a particular future. Thus the news or current articles can be mined for all the data possible from the areas that would satisfy the roadmap conditions.
A workshop participant pointed out, “On the machine side, we have lots of techniques for turning the crank and generating signposts. Once we know what we’re looking for—what the search terms are that we’re interested in—then we can find technology trends and measure things of interest like the energy density of batteries.6 You can measure that in several different ways, in many dimensions. You generally need measures of interest, but there are ways to get signposts without measures of interest. The signpost is a recognizable potential future event that also has a recommended action. If it’s not actionable, it’s just a signal, an indicator, or some place on a measure of interest. With signposts, you can then synthesize what we could call the roadmap with lots of roads.” It is a two-dimensional path. The signposts can indicate the potential future. The analysis of the potential futures refines the signposts. The analysis of the signposts can prioritize the paths or roads to the future.
Another technique offered by one participant involved ranking the relevance of paths or roads to the future as a way to offer priorities without eliminating outliers too quickly. He suggested asking both experts and generalists to assign relevance to each roadmap using a five-point scale. Each rater would also rank the projected level of impact of a technology and his or her level of expertise and level of certainty about the ranking. That ranking can then be used to tally a weighted vote.
Communication to Decision Makers
The strong roadmaps and scenarios that emerge from this process form the basis for a forecast that is eventually reported to a decision maker. The report would include the signals, signposts, scenarios, and impact of the forecast. As much as possible, the impact of the scenario must be analyzed in the context of human issues in everyday life to make it relevant, understandable, and accessible. The presentation could use dashboards, maps, diagrams, or storyboards to illustrate a “day in the life” of the forecasted future. To make it even more compelling, the story could be in the context of a game, a movie, or short work of fiction. A benefit to creating a story for presentation is that this facilitates the use of an individual’s point of view, particular to where that person lives in the world and how a technology is uniquely going to affect the person. The objective of this style of presentation is to move decision makers to take action.
THIRD FORECASTING SYSTEM: CROWDSOURCED OPTION
The third workshop subgroup’s proposed forecasting system—the Crowdsourced Option—is organized by input, analytical approaches, and outputs, with a clear focus on creating clear, actionable outputs in the form of reports. It is called the Crowdsourced Option because of its use of open participation from “the crowd” (either the general public or targeted populations) to gather forecasting inputs. These inputs are analyzed in multiple ways, employing a combination of crowdsourcing techniques and expert analysis. The final analysis is done by the members or delegates of an expert forecasting committee. If this endeavor were to be conceptualized as a business, the expert forecasting committee would be the founding board. That committee, or its delegates, would respond to a specific query from a stakeholder or sponsor. It would then be the responsibility of the expert forecasting committee to produce regular, systematic reports. Reports could also be made on interesting signals, events, or technologies that were independent of a customer query. Figure 2-3 illustrates the subgroup’s model design.
The public face of this system would be “Disruptipedia,” an online portal where data, information, live questions and responses, signs, signposts, forecasts, scenarios, and narratives would be displayed. To attract contribu-
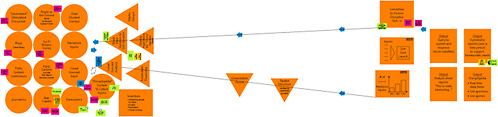
FIGURE 2-3 Crowdsourced Option. NOTE: The CD included in the back inside cover of this report has an enlargeable version of this figure, which is also reproduced in the PDF available at http://www.nap.edu/catalog.php?record_id=12834.
tions from smart, observant, knowledgeable people, incentives could include real or virtual currency or the attention of influential people. One idea was to include on the expert forecasting committee notable figures by whom contributors might want to be heard—Steve Jobs, Apple cofounder and CEO; filmmaker Steven Spielberg; or actress Uma Thurman, for example. The input could have an alternate use that would be beneficial to creative people—as a source for movie script ideas, for example. Such an arrangement could therefore be mutually beneficial to the expert forecasting committee members and to participants. Disruptipedia would serve as a living repository for the gathered information so that consistent data, information, and language could be accessed through the decades as the system grew. It would be both the constitution and the archive of this method. While such a site might help others exploit disruptive ideas and create disruption, the publishing of these ideas could likewise help the public better prepare for potential shocks caused from disruptive activities.
Inputs
Just as anything that goes into the production of a vehicle is an input, any information contributed to Disruptipedia would be an input. Inputs to the system start with narratives. Narratives can be inputs, outputs, or something between. The process is circular—narratives both feed and answer questions and act in data gathering.
To get input from people not in the mainstream, those who might be more likely to be thinking outside the conventions, the system might want to have personnel talking with and listening to people in remote areas. Forecasters should seek to understand the cultural and political context of remote populations to comprehend better the potentially globally disruptive effects of modern technologies introduced to these areas. Historical examples include cellular telephones, AK-47 assault rifles, shoulder-launched antiaircraft and antitank missiles, computers with Internet access, and improvised explosive devices (IEDs). See Table 2-3 for suggestions on how to collect additional data. It might be advantageous to have people on the ground in remote places talking with local residents, who might not have access to computers. People on the ground in remote places could be part of data-gathering staff as information came in from the field through stories being captured by journalists or the blogs of travelers or stories from workers for nongovernmental organizations.
Processes
There is a role for both human and machine processing in the Crowdsourcing Option. The qualitative processing would rely on human judgment, but there could be some automated computation and quantitative number crunching feeding into the human processing. Like any manufacturing process, it is possible to put in place the concepts outlined for the third forecasting method without equipment, information technology (IT), or systems. There are also methods to implement this system with the maximum amount of infrastructure. The degree to which automation, IT systems, and other infrastructure would be used to execute this method would be dictated by the founding team and the resources that could be secured. It is the committee’s belief that the resources needed are minimal.
There are also automated tools that are useful for collecting information and doing first-level processing on it. For example, a Web crawler could be developed to crawl all known terrorist Web sites, extract all the first- and second-level data from the sites, apply statistical machine translation, and perform topic classification or classification according to key words that are constantly being identified. These tools might not be extremely accurate at the end of all of those automated steps, because the technology for translating between languages and text categorization is still emerging, but signals would continue to emerge. Especially if a technology is emerging as a disruptive technology, the signals will persist and the crawling persists. So over time, the automated processing should find significant trends, signposts, or indicators—in markets, among technologies, concepts in conversation—to track.
Inputs could be broken down into smaller questions, an internal task for the operating group that supports the expert forecasting committee. A “hypothesis engine” could develop hypotheses for narratives. Some of the inputs could undergo automated processing, including those inputs that are quantitative in nature or which can be passed through text analysis or other systematic analytical tools. The data collected could be analyzed by expert groups, undergo crowdsourced analysis, or feed discussion by Delphic groups. Ultimately, the inputs are analyzed by the
TABLE 2-3 Inputs and Data-Collection Methods for the Crowdsourcing Option
Source |
Data-Collection Methods, Tools, Human Resources |
Blogs |
Analysts |
Travel logs |
Web crawlers |
Public content conferences |
Analysts |
|
Text search |
Public content journals |
Analysts |
Technical papers |
Technical team |
Internet content |
Web or markets |
Science-fiction writers survey |
Analysts |
|
Events |
|
Literature search |
Government-stimulated discussion |
Responses to requests |
|
Survey data |
Crowdsourced input |
Prize: trip to Technology, Entertainment, and Design (TED) Conference |
Graduate student survey and dissertations |
Three paragraphs on what the writer thinks is the “coolest” technology in his or her field |
Web crawlers |
Intelligent Web spiders to search differences |
|
Purchase |
Web scraping |
Partner with company Eighty Legs to outsource Web scraping |
Forecasters/forecaster shepherding |
People who pull from Disruptipedia concepts for envisioning the future like artists |
Disruptipedia system to collect inputs |
Chaos kiosks |
|
Partnership with the Smithsonian Institution |
|
X-Prize |
|
Netflix automated processing model |
|
Insurance companies’ databases |
|
TED as partner |
|
Public outreach |
expert forecasting committee. These analysts attend conferences, workshops, or laboratories, listening to company presentations and constantly gathering information, then debating and discussing it in order to accomplish the following:
-
Identify discontinuities;
-
Engage decision makers;
-
Interface with the output and users to learn about the quality of data inputs, methodologies used for analysis, and ways to improve the forecasting effort; and
-
Study how to continually refine the inputs and improve the methodologies of analysis.
An example process might look like the intelligence community submitting a question to the expert forecasting committee: “Is there any chance in the next 10 to 15 years that somebody’s going to develop a really low-cost way of getting satellites up—cheap and fast?” The query would be broken down into subquestions and fed through the input sources, with hypotheses developed for how this might happen. The query might be logged onto a kind of dashboard that would consistently and persistently survey the data. It might produce something that indicated the number of mentions of particular types of inputs from specific sectors. Low-cost launch might be a function of propulsion, fuels, or guidance systems, which would be tracked. After inputs are received, likely scenarios or hypotheses for the story would be expertly developed as the response to the question and delivered back to the customer. In the process of developing the information, interest confluences or technologies that are moving rapidly would be identified for tracking.
Outputs
The subgroup defined four types of products that would be outputs of the system:
-
A response to a query to the system;
-
Analyst-driven reports about topics of further interest, such as “A Global Outlook on Bio-Augmentation,” or “The State of Disruptive Technologies in Sub-Saharan Africa”;
-
Periodic reporting out and/or systematic reports over a time period to inform the sponsor or stakeholder; shorter quarterly and longer annual reports that review the status of the system and its outputs; and
-
The real-time data feeds, live questions, and live queries that would be part of the Disruptipedia display. User-facing output could be anything that the user found interesting—ideas, raw data, or narratives.
Structure
The committee believes that, as illustrated by the collaborative encyclopedia Wikipedia, the more open a public interface is, the higher the probability of broad contribution and the higher the value of the knowledge that can emerge from it. Although the use of Disruptipedia as an interface for use by a small group would be feasible, increasing openness would create improvements in the data gathered. Some uses of the information might need to be classified, and some information, due to the original source of the information, might need to be kept private or marked proprietary. To accommodate such different needs and interests, it might be useful to have separate, parallel processes. Crowdsourced analysis would be open to the public. The government may need a classified process. Access to this system would broaden the government’s reach and vision into potential disruptive technologies. The capacity to analyze the forecasting results could be broadened by mashing up the analysis community with the modeling and simulation community and cross-training them on some of the new tools areas and methodologies. There might also be a need for a separate process for things that may have federal or legal implications that are not for general public consumption.
The initiation of this forecasting system calls for an independent organization to oversee the implementation and execution of the model. To return to the manufacturing metaphor used to drive the focus here on outputs, it can also be applied to the nature in which this concept is seeded. The model for this endeavor should probably be a corporate approach, a company with an advisory board. At its outset, the endeavor would need to be flexible, focused on keeping the consumers of its reports content, and committed to the long term. A start-up manufacturing organization needs its own plant; it is too different from current operations and would be orphaned in a large existing shop floor (a division of a bureaucracy or existing organization), and it is also too different from the laboratory where the early processes were outlined (the committee that has contemplated these challenges).
Resources
The subgroup brainstormed different estimates of the resources that this system might require. If the government invested $10 million a year for a decade and the result was to diversify risk across a number of different technology sections, then it would be worthwhile. On the opposite side of the scale, if the government invested $1 million over 10 years, that would only pay the salary for one person, and it would be necessary to leverage that funding with some other kind of funds generated by the value of the system itself.
FOURTH FORECASTING SYSTEM: STORYTELLING OPTION
The fourth option proposed for a system model—called the Storytelling Option—was drawn up by workshop attendee Stan Vonog, inspired by his subgroup’s decision to prioritize narrative. The system, drawn from the world of entertainment, is a novel organizational option used by Walt Disney in 1943.
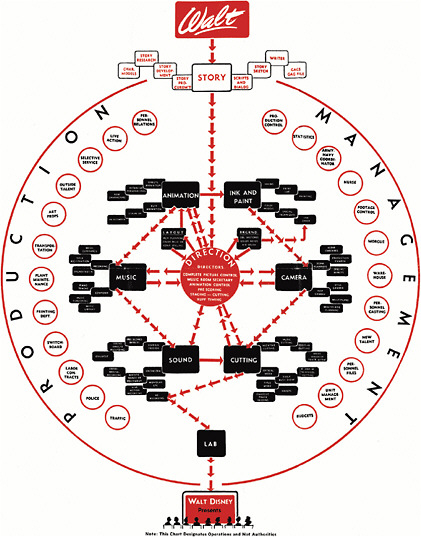
FIGURE 2-4 The Walt Disney Studios functional organizational chart of story realization from 1943. SOURCE: Walt Disney. © Disney Enterprises, Inc.
Five years after its founding and during the height of World War II, Walt Disney Studios released a functional organizational chart based on the storyboard process—bringing a story idea through production to the screen. Notably, Disney’s model incorporates no hierarchy that breaks operating divisions into separate “silos.” The chart also lacks a chain of command and authority. Instead, all staff positions serve to support a common work flow. The chart is well adapted to an idea-based process flow (see Figure 2-4).
This design option was inspired by these points in the discussion:
-
Start with the story: it is critical. Scientific measurements, calculations, and numbers are less important than an emotional appeal in the initial stages.
-
Fictional stories (e.g., the Star Trek television series, the movie Minority Report) have captured or perhaps even inspired a future to come.7
-
“The best way to predict the future is to invent it” (Kay, 1989).
-
When envisioning the future, innovators look to their desires and imagination. Forecasters should do the same.
-
Writers of fiction are professional story generators who can make useful participants in the forecasting process.
The model shown in Figure 2-5 focuses on the development of narratives from broad themes or big questions. Using this as a central theme, a small set of potential scenarios is created that identify possible contexts for exploration. For example, from the broad theme of the future of medicine, one scenario might include widespread genetic profiling to screen and prevent disease and how this could lead to social challenges and create unanticipated consequences, while another would depict a world in which human augmentation could enhance health and physical and cognitive abilities.
Data relevant to those potential scenarios are then collected using both human- and machine-based methods. Next, the data undergo critical analysis by teams of scientific, technical, and political and economic experts who identify trends and form viable hypotheses, all of which are reported back to a story director. These hypotheses are applied to the initial scenarios to create output in the form of complete narratives that can be used in reports, demonstrations, and entertainment media. The model allows for persistence: themes that warrant further exploration can be extracted from the narratives and redeveloped repeatedly using the same process.
By beginning with scenarios and emphasizing narrative development, this model is intended to produce stories that include a compelling human element. In addition to providing emotional appeal, stories can be used to contextualize the everyday use of technologies that may not be in existence yet. For example, many of the technologies found in the television series Star Trek were “everyday” in this fictional universe decades before they appeared in real life. Captain Kirk’s communicator allowed him to call for help from a planet surface to a starship in planetary orbit. A phaser beam weapon could be used in space to disable another vehicle or in the hand to disable an attacker. To be believable, these technological solutions had to convincingly address a human need. While this human element is a recognized necessity in successful entertainment media, it should be considered equally essential for eliciting action from stakeholders and policy makers who might otherwise have a lukewarm reaction to a potential threat that seemed too unlikely or abstract.
EVALUATION OF MODELS AND THE ACTIVITY
The committee evaluated the various models produced during the workshop exercises. From this activity, it was concluded that there is no one right way to build a next-generation forecasting system for disruptive technologies; each model has strengths and weaknesses. Table 2-4 paraphrases several workshop participants’ assessments of the first three models. See Appendixes D and E for workshop transcripts.
Observation. There is more than one way to build a forecasting system; each model has different strengths and weaknesses.
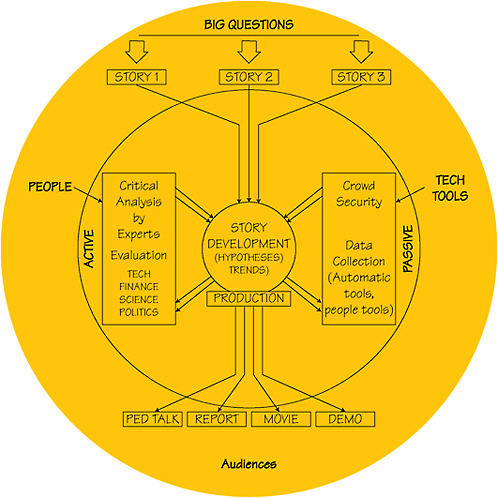
FIGURE 2-5 Storytelling Option. NOTE: The CD included in the back inside cover of this report has an enlargeable version of this figure, which is also reproduced in the PDF available at http://www.nap.edu/catalog.php?record_id=12834.
Recommendation 2-1. The 1.0 version of a forecasting system should employ the extensive passive and active data-gathering techniques employed in the Intelligence Cycle Option, using the data to develop roadmaps of potential futures with signals and signposts derived from data inputs (as seen in the Roadmapping Option). The end product of the system should include constant output and objective-driven output as described in the Crowd-sourced Option.
TABLE 2-4 Workshop Participants’ Assessments of the Three System Design Options.
Participant |
Option 1: Intelligence Cycle Option |
Option 2: Roadmapping Option |
Option 3: Crowdsourced Option |
A |
Perfect if you already have an organization following a structured approach such as the intelligence cycle. |
A lot of assumptions made on data-processing technology that would be available. Good job on how the signposts were set up. |
Web 2.0-influenced. Very open-facing, crowd-engaging way of doing analysis, inspiration-led way of doing analysis. How do you make it into a script? Organization concerns are very real for this. If you want global participation, there is a trust issue, so we must get the organization right the first time. |
B |
|
|
Would add this output array onto Option 1’s, but keep Option 1’s hypothesis engine and analysis. |
C |
|
|
We were clear on the inputs and outputs. Other groups did better on the middle part. |
D |
Inputs particularly well formulated. I would recommend theirs. |
Good on changing narrative, how to do signposts. |
Would like to add the hypothesis engine from Option 1 to this model. Particularly good way of thinking about outputs. Put these four output types with Option 1’s inputs. |
Others |
Followed the intelligence cycle to a T. Learned something from that. Important attribute of that model is that it can be molded to fit existing organizations. |
More challenges. Lots of assumptions about what the technology could do—crawling, scraping. Did a very good job thinking about how science is generated. Turn actionable thing into trap. Requires computer automation. Google approach to solve problem: Scrape sites, gather information, use some human analysis, but emphasize automated analysis. Take some accepted technology model from IGS, mass adoptions. |
Lots of writing. Lots on inputs, going through process that produces very open engagement to do analysis. The circle is an inspiration-led way of how to do analysis and put pieces together to turn a big idea into a script or narrative, but believable. Useful way to look at it. Useful to have outside people come in. Good validation. Good ideas. Organization is the challenge. Requires a global organization. If it is not done right, it is not going to get the insights one is looking for because there will not be the right participation and continual participation. |
REFERENCES
Bhan, Nita. 2010. Emerging markets as a source of disruptive innovation: 5 case studies. Core & Design magazine and Resource. February 3. Available at http://www.core77.com/blog/business/emerging_markets_as_a_source_of_disruptive_innovation_5_case_studies_15843.asp#more. Last accessed March 1, 2010.
Govindarajan, Vijay. 2009. The case for “reverse innovation” now. Business Week. October 26. Available at http://www.businessweek.com/innovate/content/oct2009/id20091026_724658.htm. Last accessed March 1, 2010.
Halal, William E. 2009. Forecasting the technology revolution. Presentation to the Committee on Forecasting Future Disruptive Technologies by the President of TechCast, LLC, August 3.
Kay, Alan. 1989. The best way to predict the future is to invent it. Stanford Engineering 1 (1, Autumn): 1-6.
Krauss, Lawrence M. 1995. The Physics of Star Trek. Harper Perennial.
NRC (National Research Council). 2010. Persistent Forecasting of Disruptive Technologies. Washington, D.C.: The National Academies Press.