E
An In-Depth Look at Study Designs and Methodologies
This appendix provides an in-depth look at study designs and methodologies. It first reviews selected designs (regression discontinuity designs, interrupted time series analysis, observational studies, pre-/posttest designs, and economic cost analysis) commonly used as alternatives to randomized experiments. It concludes with guidance on how to use theory, professional experience, and local wisdom to adapt the evidence gathered to local settings, populations, and times.
COMMON RESEARCH DESIGNS
Reichardt (2006) presents a typology that encompasses the full range of randomized and strong quasi-experimental nonrandomized designs (see Table E-1). This typology is useful because it can substantially broaden the range of design options that can be considered by researchers. Reichardt considers all possible designs that can be created
TABLE E-1 A Typology of Research Designs
Prominent Size-of-Effect Factor |
Assignment to Treatment |
||
Random |
Nonrandom |
||
Explicit Quantitative Ordering |
No Explicit Quantitative Ordering |
||
Recipients |
Randomized recipient design |
Regression discontinuity design |
Nonequivalent recipients design |
Times |
Randomized time design |
Interrupted time series design |
Nonequivalent times design |
Settings |
Randomized setting design |
Discontinuity across settings design |
Nonequivalent settings design |
Outcome variables |
Randomized outcome variable design |
Discontinuity across outcome variables design |
Nonequivalent outcome variables design |
SOURCE: Reichardt, 2006. |
based on the combination of two dimensions: assignment rule and primary dimension for assignment of units.
With respect to assignment rule, units can be assigned (1) according to a randomized allocation scheme, (2) on the basis of a quantitative assignment rule, or (3) according to an unknown assignment rule. Randomization schemes in which each unit has an equal probability of being in a given treatment condition are familiar. A quantitative assignment rule means that there is a fixed rule for assigning units to the intervention on the basis of a quantitative measure, typically of need, merit, or risk. For example, organ transplants are allocated on the basis of a weighted combination of patient waiting time and the quality of the match of the available organ to the patient. Finally, unknown assignment rules commonly apply when units self-select into treatments or researchers give different treatments to preexisting groups (e.g., two communities, two school systems). Unknown assignment rules are presumed to be nonrandom.
With respect to units, participants (people or small clusters of people), times, settings, or outcome measures may serve as the units of analysis. Research in public health and medicine commonly assigns treatments to individual (or small groups of) participants. But other units of assignment are possible and should be entertained in some research contexts. Time can be the unit of assignment, as, for example, in some drug research in which short-acting drugs are introduced and withdrawn, or behavior modification interventions are introduced and withdrawn to study their effects on the behavior of single patients. Settings can be the unit of assignment, as when different community health settings are given different treatments, or different intersections are given different treatments (e.g., photo radar monitoring of speeding in a traffic safety study). Finally, even outcome measures can be assigned to different conditions. In a study of the effectiveness of the Sesame Street program, for example, different sets of commonly used letters (e.g., [a, o, p, s] versus [e, i, r, t]) could be selected for inclusion in the program. The knowledge of the specific letters chosen for inclusion in the program could be compared with the knowledge of the control letters to assess the program’s effectiveness. Once again, each of these types of units could potentially be assigned to treatment conditions using any of the three assignment rules.
Reichardt provides a useful heuristic framework for expanding thinking about strong alternative research designs. When individuals are not the unit of analysis, however, complications may arise in the statistical analysis. These complications are addressable.
In this section, some commonly utilized quasi-experimental designs from Reichardt’s framework are described. First, two designs involving nonrandom, quantitative assignment rules—the regression discontinuity design and the interrupted time series design—are discussed. Next, the observational study (also known as the nonequivalent control group design or nonequivalent recipients design), in which the basis for assignment is unknown, is considered. Finally, the pre-experimental pre–post design, commonly utilized by decision makers, is discussed. For each design,
Campbell’s and Rubin’s perspectives (detailed in Chapter 8) are brought into the discussion as the basis for suggestions for enhancements that may lead to stronger causal inferences.
Regression Discontinuity (RD) Design
Often society prescribes that treatments be given to those with the greatest need, risk, or merit. A quantitative measure is assessed at baseline (or a composite measure is created from a set of baseline measures), and participants scoring above (or below) a threshold score are given the treatment. To cite three examples from the educational arena, access to free lunches is often given to children whose parents have an income below a specified threshold (e.g., the poverty line), whereas children above the poverty line do not receive free lunches. The recognition of dean’s list is awarded only to students who achieve a specified grade point average (e.g., 3.5 or greater). And children who reach their sixth birthday by December 31 are enrolled in first grade the following August, whereas younger children are not. Given assessment of the outcome following the intervention, comparison of the outcomes at the threshold for the intervention and in control groups permits strong causal inferences to be drawn.
To understand the RD design, consider the example of evaluating the effectiveness of school lunch programs on health, which is illustrated in Figure E-1. In the figure, all children with a family income of less than $20,000 qualify for the program, whereas children whose families exceed this threshold do not. The outcome measure (here a measure of health problems, such as number of school absences or school nurse visits) is collected for each child. In modeling the relationship between the known quantitative assignment variable (family income) and the outcome, the treatment effect will be represented by the difference in the levels of the regression lines at the cutpoint. In the basic RD design, treatment assignment is determined entirely by the assignment variable. Proper modeling of the relationship between the assignment variable and the outcome permits a strong inference of a treatment effect if there is a discontinuity at the cutpoint.
Ludwig and Miller (2007) used this design to study some of the educational and health effects of the implementation of the original Head Start program in 1965. When the program was launched, counties were invited to submit applications for Head Start funding. In a special program, the 300 poorest counties in the United States (poverty rates exceeding a threshold of 59.2 percent) received technical assistance in writing the Head Start grant proposal. Because of the technical assistance intervention, a very high proportion (80 percent) of the poorest counties received funding, approximately twice the rate of slightly better-off counties (49.2 percent to 59.2 percent poverty rates) that did not receive this assistance. The original Head Start program included not only its well-known educational program, but also basic health services to children (e.g., nutrition supplements and education, immunization, screening). In addition to positive effects on educational achievement, Ludwig and Miller
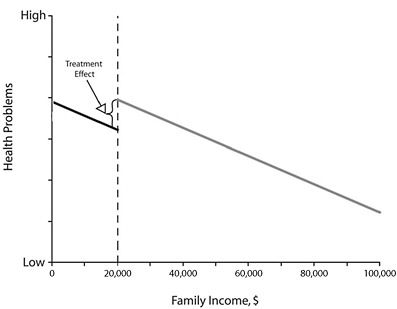
FIGURE E-1 Illustration of the regression discontinuity design using the example of an evaluation of the effect of school lunch programs on children’s health.
NOTE: All children whose family income was below the threshold, here $20,000 (dotted line), received the treatment program (school lunch program); all children whose family income was above the threshold did not receive the program. The difference between the regression lines for the program and no-program groups at the threshold represents the treatment effect.
SOURCE: West et al., 2008. Reprinted with permission. West et al., Alternatives to the randomized controlled trial, American Journal of Public Health, 98(8):1364, Copyright © 2008 by the American Public Health Association.
found results demonstrating lower mortality rates in children aged 5 to 9 from diseases addressed by the program (e.g., measles, anemia, diabetes).
The RD design overcomes several of the objections to the randomized controlled trial (RCT) discussed in this report. When an existing program uses quantitative assignment rules, the RD design permits strong evaluation of the program without the need to create a pool of participants willing to be randomized. Sometimes outcome data may be collected routinely from large samples of individuals in the program. As illustrated by the Ludwig and Miller study, the design can be used when individual participants, neighborhoods, cities, or counties are the unit of assignment. When new programs are implemented, assignment on the basis of need or risk may be more acceptable to communities that may be resistant to RCTs. The use of a clinically meaningful quantitative assignment variable (e.g., risk level) may help overcome ethical or political objections when a promising potential treatment is being evaluated. Given that the design can often be implemented with the full population of interest—a state, community, school, or hospital—it provides direct evidence of population-level effects.
The RD design is viewed as one of the strongest alternatives to the RCT from both Campbell’s (Cook, 2008; Shadish et al., 2002; Trochim, 1984) and Rubin’s (Imbens and Lemiuex, 2008; Rubin, 1977) perspectives. However, it introduces two new challenges to causal inference that do not characterize the RCT. First, it is assumed that the functional form of the relationship between the quantitative assignment variable and the outcome is properly modeled. Early work on the RD design in the behavioral sciences typically assumed that a regression equation representing a linear effect of the assignment score on the outcome plus a treatment effect estimated at the cutoff would be sufficient to characterize the relationship. More recent work in econometrics has emphasized the use of alternative methods to characterize the relationship between the assignment variable and the outcome separately above and below the threshold level. For example, with large sample sizes, nonparametric regression models can be fit separately above and below the threshold to minimize any possibility that the functional form of the relationship is not properly specified. Second, in some RD designs, the quantitative assignment variable does not fully determine treatment assignment. Econometricians make a distinction between “sharp” RD designs, in which the quantitative assignment variable fully determines treatment assignment, and “fuzzy” RD designs, in which a more complex treatment selection model determines assignment. These latter designs introduce considerably more complexity, but new statistical modeling techniques based on the potential outcomes perspective (see Hahn et al., 2001) minimize any bias in the estimate of treatment effects.
From Campbell’s perspective, several design elements can potentially be used to strengthen the basic design. Replication of the original study using a different threshold can help rule out the possibility that some form of nonlinear growth accounts for the results. Masking (blinding) the threshold score from participants, test scorers, and treatment providers, when possible, can minimize the possibility that factors other than the quantitative assignment variable determine treatment. Investigating the effects of the intervention on a nonequivalent dependent variable that is expected to be affected by many of the same factors as the primary outcome variable, but not the treatment, can strengthen the inference. In the case of fuzzy RD designs, sensitivity analyses in which different plausible assumptions are made about alternative functional forms of the relationship and selection models can also be conducted.
Interrupted Time Series (ITS) Analysis
Often policy changes go into effect on a specific date. To illustrate, the Federal Communications Commission (FCC) allowed television broadcasting to be introduced for the first time in several medium-sized cities in the United States in 1951. Bans on indoor smoking have been introduced in numerous cities (and states) on specific dates. If outcome data can be collected or archival data are available at regular fixed intervals (e.g., weekly, monthly), the ITS provides a strong design for causal inference. The logic of the ITS closely parallels that of the RD design except that the threshold
on the time rather than the baseline covariate is the basis for treatment assignment (Reichardt, 2006).
Khuder and colleagues (2007) present a nice illustration of an ITS. An Ohio city instituted a ban on smoking in indoor workplaces and public places in March 2002. All cases of angina, heart failure, atherosclerosis, and acute myocardial infarction in city hospitals were identified from hospital discharge data. Following the introduction of the smoking ban, a significant reduction of heart disease–related hospital admissions was seen.
From a design standpoint, causal inferences from the simple ITS perspective need to be tempered because the basic design fails to address three major threats to the certainty of the causal relationship between an intervention and the observed outcomes (internal validity) (Shadish et al., 2002; West et al., 2000). First, some other confounding event (e.g., introduction of a new heart medication) may occur at about the same time as the introduction of the intervention. Second, some interventions may change the population of participants in the area. For example, some cities have offered college scholarships to all students who graduate from high school. In such cases, in addition to any effect of the program on the achievement of city residents, the introduction of the program may foster immigration of highly education-oriented families to the city, changing the nature of the student population. Third, record-keeping practices may change at about the time of the intervention. For example, new criteria for the diagnosis of angina or myocardial infarction may change the number of heart disease cases even in the absence of any effect of the intervention.
From a statistical standpoint, several potential problems with longitudinal data need to be addressed. Any long-term natural trends (e.g., a general decrease in heart disease cases) or cycles (e.g., more admissions during certain seasons of the year) in the data need to be modeled so their effects can be removed. In addition, time series data typically reflect serial dependence: observations closer in time tend to be more similar than observations further apart in time. These problems need to be statistically modeled to remove their effects, permitting proper estimates of the causal effect of the intervention and its standard error. Time series analysis strategies have been developed to permit researchers to address these issues (e.g., Chatfield, 2004). In addition, as in the RD design, the actual introduction of the intervention may be fuzzy. In the Khuder et al. study, for example, there was evidence that the enforcement and full implementation of the smoking ban required some months after the ban was enacted. In such cases, a function describing the pattern of implementation of the intervention may need to be included in the model (e.g., Hennigan et al., 1982).
In the Campbell tradition, causal inferences drawn from the basic ITS design can be greatly strengthened by the addition of design elements that address threats to validity. Khuder and colleagues included another, similar Ohio city that did not institute a smoking ban (control series), finding no parallel change in heart disease admissions after the March 2002 timepoint when the smoking ban was introduced in the treatment city. They also found that hospital admissions for diagnoses unrelated
to smoking did not change in either city after March 2002 (nonequivalent dependent variable). In some time series applications, a design element known as switching replications can be used, which involves locating another, similar city in which the intervention was introduced at a different timepoint. In their study of the introduction of television and its effects on crime rates, for example, Hennigan and colleagues (1982) located 34 medium-sized cities in which television was introduced in 1951 and 34 cities matched for region and size in which television was introduced in 1954 following the lifting of a freeze on new broadcasting licenses by the FCC. They found a similar effect of the introduction of television on crime rates (e.g., an increase in larceny) beginning in 1951 in the prefreeze cities and in 1954 in the postfreeze cities. In both the Khuder et al. and Hennigan et al. studies, the addition of the design element greatly reduced the likelihood that any threat to the level of certainty of the causal inference (internal validity) could account for the results obtained. As with the RD design, moreover, the ITS design can often be implemented with the full population of interest so that it provides direct evidence of population-level effects.
Observational Studies
The observational study (also known as the nonequivalent control group design) is a quasi-experimental design that is commonly used in applied research on interventions, likely because of its ease of implementation. In this design, a baseline measure and a final outcome measure are collected on all participants. Following the baseline measurement, one group is given the treatment, while the second, comparison group does not receive the treatment. The groups may be preexisting (e.g., schools, communities), or unrelated participants may self-select into the treatment in some manner. For example, Roos and colleagues (1978) used this design to compare the health outcomes of children who received and did not receive tonsillectomies in a province of Canada. The bases on which the selection into the tonsillectomy treatment occurred were unknown and presumed to be nonrandom, possibly depending on such factors as the child’s medical history, the family, the physician, and the region. The challenge of this design is that several threats associated with possible interactions between selection and other threats to level of certainty (internal validity) might be plausible. These threats must be addressed if strong causal inferences are to be drawn.
To illustrate this design, consider an evaluation of a campaign to increase sales of lottery tickets (Reynolds and West, 1987). State lottery tickets are sold primarily in convenience stores and contribute to general state revenue or revenue for targeted programs (e.g., education) in several states. The stores refused randomization, ruling out an RCT. The Arizona lottery commission wished to evaluate the effectiveness of a sales campaign to increase lottery ticket sales in an 8-week-long lottery game. In the “Ask for the Sale” campaign, store clerks were instructed to ask each adult customer during checkout if he or she wished to purchase a lottery ticket. A nearby sign
informed customers that if the sales clerk did not ask them, they would get a lottery ticket for free.
From Campbell’s perspective, four threats to the level of certainty (internal validity) in this example observational study could interact with selection and undermine causal inference regarding the intervention and the observed outcomes (Shadish et al., 2002):
-
Selection × history interaction—Some other event unrelated to the treatment could occur during the lottery game that would affect sales. Control stores could be disproportionately affected by nearby highway construction, for example, resulting in a decrease in customer traffic.
-
Selection × maturation interaction—Sales in treatment stores could be growing at a faster rate than sales in control stores even in the absence of the treatment.
-
Selection × instrumentation interaction—The nature of the measurement of lottery ticket sales in the treatment stores could change during the game in the treatment but not the control group. For example, stores in the treatment group could switch disproportionately from manual reporting of sales to more complete computer recording of sales.
-
Selection × statistical regression interaction—Stores having unusually low sales in the previous lottery game could self-select to be in the treatment group. Sales could return to normal levels even in the absence of the intervention.
Reynolds and West (1987) matched treatment and control stores on sales in the prior game and on ZIP code (a proxy for neighborhood socioeconomic status). As shown in Figure E-2, they implemented the basic observational study design and then added several design features to address possible threats to the certainty of the causal relationship (internal validity). Panel (a) displays the results of the basic design, showing no difference in sales in the prior game (game 10, no program intervention in both stores, i.e., baseline) but greater sales in the treatment stores during the campaign (game 11) compared with matched control stores with no program intervention. Panel (b) displays the results from a set of nonequivalent dependent measures, sales categories that would be expected to be affected by other general factors that affect sales but not by the intervention. The increase in sales of lottery tickets was greater than the increase for other sales categories. Panel (c) displays the results from a short time series of observations in which the sales campaign was implemented in the treatment stores in week 4 of the game. The results show that both the treatment and control stores experienced similar levels of sales each week prior to the intervention, but that the treatment stores sold far more tickets each week following the initiation of the campaign at the beginning of week 5 (i.e., “program started” in Figure E-2). Taken together, the pattern of results presented by the basic design and the additional design elements provided strong support for the effectiveness of the sales campaign.
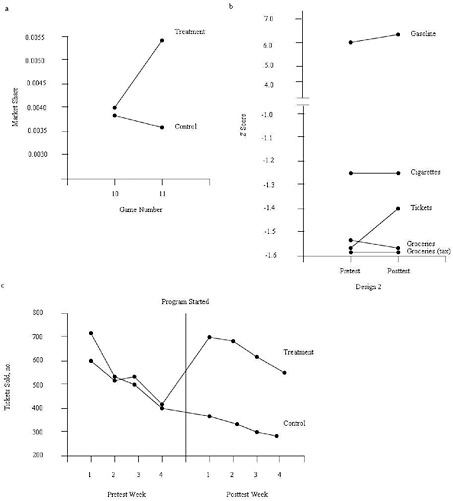
FIGURE E-2 Design elements that strengthen causal inferences in observational studies.
NOTE: (a) Matching. Treatment and control stores were selected from the same chain, were in the same geographic location, and were comparable in sales during the baseline (lottery game 10). Introduction of the treatment at the beginning of lottery game 11 yielded an increase in sales only in the treatment stores. (b) Nonequivalent dependent variables. Within the treatment stores, sales of lottery tickets increased substantially following the introduction of the treatment. Sales of other major categories (gasoline, cigarettes, nontaxable groceries, and taxable groceries) that would be expected to be affected by confounding factors but not the treatment did not show appreciable change. (c) Repeated pre- and posttest measurements. Treatment and control stores’ sales showed comparable trends during the 4 weeks prior to the introduction of the treatment. The level of sales in the treatment and control stores was similar prior to the introduction of the treatment, but differed substantially beginning immediately after the treatment was introduced.
SOURCE: Adapted from Reynolds and West, 1987. Reynolds, K. D., and S. G. West, Evaluation Review 11:691-714, Copyright © 1987 by SAGE Publications. Reprinted by permission of SAGE Publications.
Rubin’s (2006) perspective takes an alternative approach, attempting to create high-quality matches between treatment and control cases on all variables measured at baseline. Two recent developments in the technology of matching procedures have greatly improved the quality of matches that can be achieved. Historically, the number of variables on which high-quality matches could be achieved was limited because of the difficulty of finding cases that matched on several different variables. Rosenbaum and Rubin (1983) developed the idea of matching on the propensity score—the probability that the case would be in the treatment group based on measured background variables. They proved mathematically that if close matches between cases in the treatment and control groups could be achieved on the propensity score, the two groups would also be closely balanced on all baseline variables from which the propensity score was constructed. Propensity scores are typically created on the basis of a logistic regression equation in which the probability of being in the treatment group based on the measured covariates is estimated (see Schafer and Kang, 2008, for a discussion of available techniques). The distributions of each separate baseline covariate in the treatment and control groups can be compared to ensure that close matches have been achieved. Second, new optimal matching algorithms for pairing cases in the two groups maximize the comparability of the groups’ propensity scores (Rosenbaum, 2002). The central challenge for these modern matching procedures is ensuring that all important covariates related to both the treatment group and the outcome have been included in the construction of the propensity score. Otherwise stated, have “identical” units been created by the matching procedures?
Wu and colleagues (2008a,b; see also West and Thoemmes, 2010) used these procedures in a study of the effect of retention in first grade on children’s subsequent math and reading achievement. Based on input from subject matter experts, they measured 72 variables at baseline believed to possibly be related to retention, achievement, or both. Propensity scores were constructed using logistic regression. From 784 children who were below the median at school entrance, 97 pairs that were closely matched on the propensity scores were constructed using optimal matching procedures. Figure E-3 shows that the distribution of the retained and promoted groups on propensity scores became closely balanced following the use of the optimal matching procedures. The use of propensity scores helps rule out the possibility that preexisting differences (selection bias) between the groups could account for the results obtained.
The central challenge of the propensity score procedure is ensuring that the groups have been closely balanced on all important covariates. For example, matching on a few demographic variables is unlikely to represent fully all of the important baseline differences between the groups (Rubin, 2006). Checks on the balance for each individual covariate can be performed, and propensity scores can be reestimated or specific controls for unbalanced covariates included in the statistical models. Finally, researchers can conduct sensitivity analyses to explore how much the results would change if important covariates were omitted from the propensity score model.
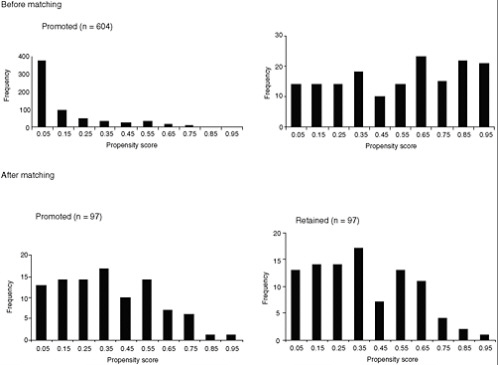
FIGURE E-3 The distributions of propensity score before and after matching in a study of the effects of retention in first grade on children’s subsequent math and reading achievement.
NOTE: The scale of the y-axis (frequency) differs between the two top panels (before matching), but is identical for the two bottom panels (after matching). SD = standard deviation.
SOURCE: Wu et al., 2008b.
Pre-/Posttest Designs
Sometimes researchers believe that designs involving a pretest (baseline), a treatment, and a posttest (outcome) measure are sufficient to evaluate the effects of interventions. Such designs are attractive because of their ease of implementation, but they are far weaker in terms of causal inference than experimental and quasi-experimental designs. Campbell and Stanley (1966) present these designs in detail, considering them in a separate, weaker class of “pre-experimental” designs. The following threats to the level of certainty (internal validity) characterize the pretest–posttest design
-
History—Some other event unrelated to the treatment occurs between the pretest and posttest that could cause the observed effect in the absence of a treatment effect.
-
Maturation—Maturational growth or decline in individuals (or secular trends in larger units) could cause the observed effect in the absence of a treatment effect.
-
Instrumentation—A change in the measuring instrument could lead to increases or decreases in the level of the outcome variable in the absence of a treatment effect. Sometimes these changes can be quite subtle, as when a well-validated measurement instrument may measure different constructs in children of different ages (e.g., standardized math tests).
-
Testing—Simply the taking of a test or knowledge of the specific dimensions of one’s behavior that are being monitored could lead to increases or decreases in scores in the absence of a treatment effect.
-
Statistical regression—Participants could be selected for treatment that is extreme relative to the mean of their group or is initiated at particular crisis periods in their lives (e.g., a period of binge eating during the holidays). Unreliability in measurement or the passage of the crisis with time could lead to scores that are closer to the mean upon retesting in the absence of a treatment effect. Again, these effects can be quite subtle (see Campbell and Kenny, 1999, for a review).
Any pretest–posttest design may be subject to plausible versions of one or more of these threats to its level of certainty (internal validity) depending on the specific research context, making it very difficult to conclude that a causal effect of the treatment has occurred. Once again, following Campbell’s tradition, the strategy of adding design elements to address specific plausible threats can help provide more confidence that the treatment rather than other confounding factors has had the desired effect. For example, replicating the pretest–posttest design at different times with different cohorts of individuals can help rule out history effects, while taking several pretest measures to estimate the maturation trend in the absence of treatment can help rule out history. Box 8-4 in Chapter 8 identifies many of the design elements that can be employed; Shadish and Cook (1999) and Shadish and colleagues (2002) present fuller discussions. The design element approach does not enable the certainty about causal inference provided by the RCT, but it can often greatly improve the evidence base on which decision makers make choices about implementing interventions.
Economic Cost Analysis
Studies that assess the economic costs of obesity can differ in terms of their breadth and perspective. Differences in breadth will be reflected in choices of the population(s) covered (e.g., defined by age, gender, race/ethnicity, socioeconomic status), the range of diseases considered, and the types of costs to include. These decisions will be driven by the perspective taken in the study, as well as by available data.
One key distinction is in the methodological approach employed. In conducting economic cost studies, researchers choose between a “prevalence-based” approach and an “incidence-based” approach (Lightwood et al., 2000). The prevalence based approach (also referred to as an “annual cost” or “cross-sectional” approach) is
used to estimate the costs associated with a given problem at a point in time (e.g., a given year), reflecting not just the costs resulting from the condition of interest at that point in time, but also the costs resulting from cumulative past health status variables that manifest themselves at that point in time. Given the lags between poor diets, physical inactivity, obesity, and resulting diseases, cost estimates based on the prevalence approach reflect historical behavior patterns and cannot be used to predict the short-term impact of interventions aimed at reducing obesity and its consequences. Nevertheless, this is the most common approach used in economic cost studies given its relatively simpler methodology and the availability of necessary data.
The incidence-based approach (also referred to as a “lifetime cost” or “longitudinal” approach) aims to estimate the additional costs expected to result from a given condition in a specific population over their lifetimes. When applied to health care costs resulting from obesity, this approach balances the additional health care costs an obese individual faces at a point in time against the health care cost savings that accrue as a result of the shorter lifetime of an obese individual. These estimates can be useful for decision makers, who can estimate the change in costs of obesity that results from policy and other interventions aimed at changing the behaviors that result in obesity. However, the estimates produced by the incidence-based approach can be quite sensitive to assumptions about future costs, changes in health care delivery and technology, and the way future costs are discounted. An additional challenge relates to adequately controlling for the variety of other determinants of costs, that is, trying to estimate costs for a nonobese person so that the true excess costs resulting from obesity can be determined.
Finally, decisions about what costs to include under either approach will have a significant impact on the resulting estimates (Lightwood et al., 2000). There are several key distinctions here, with choices related to the perspective from which one is assessing costs. Economists, for example, distinguish between internal and external costs, with this distinction reflecting who ultimately bears the costs. Internal costs are those that are borne by the individual who engages in a given behavior or has a given condition; for obese individuals, these include the out-of-pocket costs and health insurance premiums they pay for health care, as well as the lower wages they receive if these wages reflect their lower productivity. External costs are those that are borne by individuals as a result of others’ behaviors; for example, monies spent on public health insurance programs that cover the costs of treating diseases caused by obesity are shared by all taxpayers, including those at healthy weights. Many argue that only external costs should be considered when making decisions about whether policy interventions are warranted. However, to the extent that other market failures exist (e.g., information failures reflecting a poor understanding of the link between sugar-sweetened beverage consumption and weight that can be further distorted by effective marketing of such beverages or individuals’ time-inconsistent preferences that result in short-term gratification at the cost of long-term harm), it may be appropriate to include some portion of internal costs.
A second key distinction relates to a focus on the “gross” versus the “net” costs of a given behavior. The prevalence-based approach produces an estimate of gross costs—those that result from the consequences of obesity in a given year, for example. In contrast, the incidence-based approach produces an estimate of net costs, reflecting the trade-offs between higher average annual costs for an obese individual and the extra costs that result from a nonobese individual’s living longer. When one is comparing gross and net costs, a particularly controversial issue relates to what has come to be known as the “death benefit,” that is, whether the “savings” that result from lower pension and social security payments that an obese individual who dies prematurely will not collect should be included in the cost accounting.
MATCHING, MAPPING, POOLING, AND PATCHING
Despite best efforts to amass available evidence, decision makers grappling with an emergent problem such as obesity will face inevitable decisions that must be made and actions that must be taken in the relative absence of evidence. This section offers useful methods for combining evidence with theory, expert opinion, experience, and local wisdom about local traditions and probable responses to proposed actions in a process that has been described as “matching, mapping, pooling, and patching” (Green and Glasgow, 2006; Green and Kreuter, 2005, pp. 197-203).
Matching
Matching refers to aligning the source of evidence with the targets of an intervention. The evidence from different disciplines of science and research is distributed according to the level (individual, family, organization, or community) at which it was generated and matched to the level(s) of the proposed intervention and its intended impact (McLeroy et al., 1988; Richard et al., 1996; Sallis et al., 2008). Evidence from psychology and medicine generally focuses on the individual, while evidence from sociology and public health generally applies to groups, organizations, and populations. The ecological thinking and recognition of social determinants of health implicit in this approach have seen a renaissance in public health in recent decades (Berkman and Kawachi, 2000; Best et al., 2003; French et al., 2001; Richard et al., 1996; Sallis et al., 2008). The usual representation of the ecological model in public health and health promotion is a set of concentric circles or ovals, as illustrated in Chapter 1 (see Figure 1-5) (e.g., Booth et al., 2001; Green and Kreuter, 2005, p. 130; IOM, 2007; National Committee on Vital and Health Statistics, 2002). For example, an ecological approach to planning a comprehensive childhood obesity control intervention would involve matching evidence of an intervention’s effectiveness with each ecological level to which the evidence might apply:
-
evidence from studies of individual change in diet and physical activity, particularly from clinical studies and evaluations of obesity control programs directed at individuals and small groups;
-
evidence from studies of family, community, and cultural groups regarding group or population changes, and evaluations of change in response to mass communication or organizational or environmental changes; and
-
evidence from policy studies, public health evaluations, and epidemiological studies—for example, the impact of school lunch programs on food consumption patterns, or the impact of policies, executive decisions, and regulatory enforcement of codes for construction of sidewalks or hiking or bike paths on community physical activity patterns.
The preponderance of evidence, especially that judged worthy of inclusion in systematic reviews for evidence-based practice guidelines, tends to be derived from studies of the impact of interventions on individuals. Yet there is widespread agreement that further progress in controlling obesity will require policy changes, organizational changes, changes in the built environment, and changes in social norms, all of which require interventions and measurement of change at levels beyond the individual.
An example of an ecological model that illustrates the matching process is the Multilevel Approach to Community Health (MATCH) model of B. G. Simons-Morton and colleagues (1995) (see Figure E-4). The MATCH model suggests the alignment of evidence with each of the four levels shown by the vertical arrangement of boxes in the figure. It grew out of a conceptualization of intervention research (Parcel, 1987; D. G. Simons-Morton et al., 1988a), and was applied in a series of Intervention Handbooks published by the Centers for Disease Control and Prevention (CDC), including one on promoting physical activity among adults (D. G. Simons-Morton et al., 1988b), one on a series of reviews on health-related physical fitness in childhood (B. G. Simons-Morton et al., 1988a), and one on implementing organizational changes to promote healthful diet and physical activity in schools (B. G. Simons-Morton et al., 1988b). Other ecological models have been suggested for planning and evaluating overall programs (e.g., Green and Kreuter, 2005; Richard et al., 1996) or components of programs such as mass communications (Abroms and Maibach, 2008), and for integrating knowledge for community partnering (Best et al., 2003). The MATCH model is a first step in blending evidence from various sources for the design of a comprehensive intervention that will address the various levels at which evidence is needed and for which adaptations and innovations will be necessary when the evidence is lacking at specific levels.
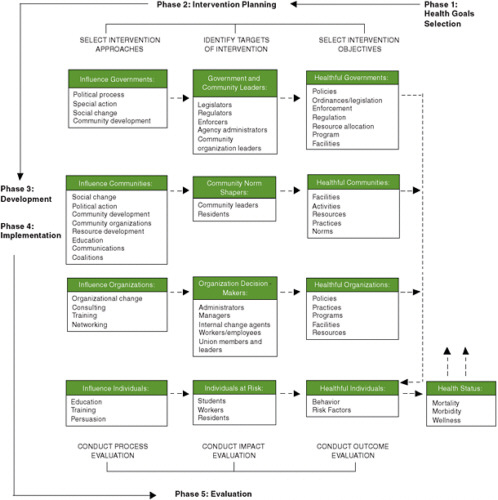
FIGURE E-4 The Multilevel Approach to Community Health (MATCH) model used to align the source of evidence with the targets of an intervention, plan and evaluate programs, and integrate knowledge for community partnering.
SOURCE: B. G. Simons-Morton et al., 1995. Reprinted by permission of Waveland Press, Inc. from B. G. Simons-Morton, W. H. Greene, and N. H. Gottlieb, Introduction to health education and promotion, 2nd ed. Long Grove, IL: Waveland Press, Inc., 1995, All rights reserved.
Mapping
Mapping refers to tracing the causal chains or mechanisms of change inferred when evidence is matched with levels of intervention and change. The evidence will be incomplete at each ecological level with respect to the local or state circumstances in which a decision and action must be taken, but theory can (and will, formally or informally, consciously or unconsciously) be brought to bear. People naturally impose their own assumptions about causes and needed actions, and these constitute, in the crudest sense, theories of the problem and the solution. A formal process of mapping objectives, evidence, and formal theories of behavior and social change to the design of coherent, practical interventions includes guidelines for how to assess evidence and theories (Bartholomew et al., 2006). For example, Schaalma and Kok (2006) describe their development of an HIV prevention program using theory to guide their selection of determinants of behavior and the environment (e.g., knowledge, risk perceptions, attitudes, social influences, self-efficacy) relevant to the health problem (e.g., safe sex). Using this process of mapping the objectives of the intervention, existing evidence (both qualitative and quantitative), and theory, the authors were able to identify theoretical methods of behavior change (e.g., social cognitive theory) and translate them into practical strategies (e.g., video-guided role playing). The mapping of theory helps the decision maker fill gaps in the evidence and consider how the evidence from a distant source and a particular population may or may not apply to the local setting, circumstances, and population.
Too often, the published literature in a discipline will provide little if any detail about the theory on which an intervention was based, sometimes because the study was not designed to test a theory, sometimes out of reluctance or inability to be explicit about the theory, and sometimes because editors of health science journals prefer to devote space to methodological detail and data analysis rather than theory. Greenhalgh and colleagues (2007, p. 861) suggest shifting “the balance in what we define as quality from an exclusive focus on empirical method (the extent to which authors have adhered to the accepted rules of controlled trials) to one that embraces theory (the extent to which a theoretical mechanism was explicitly defined and tested).”
Although theory may not be the interest of the end users of evidence, they will, as noted, have their own assumptions in the form of tacit theories. This step will benefit from their consultation with those who have experience with the problem and understand the scientific literature and its formally tested theories of causation and change.
Pooling
Much of the published evidence in obesity-related research is epidemiological or observational, linking the intermediate causes or risk factors with obesity-related health outcomes. Therefore, the range of values of moderating variables (demographic, socio-
economic, and other social factors that modify the observed relationships) in models that predict outcomes is limited. The experimental evidence is even more scarce and limited in generalizability. Because of these limitations of the evidence-based practice literature, decision makers must turn to practice-based evidence and ways of pooling evidence from various extant or emerging programs and practices.
Pooling refers to consultation with decision makers who have dealt with the problem of obesity in a similar population or setting. After matching and mapping the evidence and theories, local decision makers will still be uncertain about how well the evidence applies to each of the mediators (i.e., mechanisms or intermediate steps) and moderators (i.e., conditions that make an association stronger or weaker) in their logic model for local action. At this point, they should turn to the opinions of experts and experienced practitioners in their or similar settings (e.g., Banwell et al., 2005; D’Onofrio, 2001). Methods exist for pooling these opinions and analyzing them in various systematic and formal or unsystematic and informal ways. For example, Banwell and colleagues (2005) used an adapted Delphi technique (the Delphi Method, described in Chapter 6) to obtain views of obesity, dietary, and physical activity experts about social trends that have contributed to an obesogenic environment in Australia. Through this semistructured process, they were able to identify trends in expert opinion, as well as rank the trends to help inform public policy.
Practice-based evidence is that which comes primarily from practice settings, in real time, and from typical practitioners, as distinct from evidence from more academically controlled settings, with highly trained and supervised practitioners conducting interventions under strict protocols. Such tacit evidence, often unpublished, draws on the experience of those who have grappled with the problem and/or the intervention in situations more typical of those in which the evidence would be applied elsewhere. Even when evidence from experimental studies is available, decision makers often ask, understandably, whether it applies to their context—in their practice or policy setting, circumstances, and population (Bowen and Zwi, 2005; Dobbins et al., 2007; Dobrow et al., 2004, 2006; Green, 2008). They want to weigh what the experimental evidence shows, with its strong level of certainty of the causal relationship between the intervention and the observed outcomes (internal validity), against what the experience of their own and similar practices and practitioners has been, with its possibly stronger generalizability (external validity).
Finally, the use of pooling in weighing and supplementing evidence becomes an important negotiating process among organizations cooperating in community-level and other broad collaborative programs and policies. Each participant in such collaborations will weigh different types of evidence differently, and each will have an idiosyncratic view of its own experience and what it says about the problem and the proposed solutions (Best et al., 2003). This recognition of complexities in the evidence and multiplicities of experience has led to a growing interest in systems theory or systems thinking (Green, 2006) (see Chapter 4).
Patching
Engaging people from the community affected by the problem of obesity or its determinants allows for the inclusion of local wisdom from the outset in the adaptation of evidence-based practices and the creation of new ideas to be tested. As with any federal or state health program that must depend on local and state initiative and implementation, the process of rolling out a policy or taking a program to scale requires the engagement and participation of practitioners and populations at the front lines (Ottoson et al., 2009). Success will depend on continuous adaptation to their perception of needs; their understanding of and access to local resources; their willingness, skill, and confidence to implement the recommended intervention; and the reinforcement they will get from doing so (Ottoson and Green, 1987). Many of the methods for patching together evidence-based practices, theory-based programs, and practice-based experiences into a viable effort at the state or local level are contained in manuals and guidelines such as CDC’s Planned Approach to Community Health (PATCH; Kreuter, 1992) and Racial and Ethnic Approaches to Community Health, (REACH; CDC, 2009b); community-based participatory research (CBPR; Cargo and Mercer, 2008; Horowitz et al., 2009; Minkler and Wallerstein, 2008); the National Cancer Institute (NCI) and Substance Abuse and Mental Health Services Administration’s (SAMHSA) Cancer Control PLANET (NCI and SAMHSA, 2009); and other web resources that need to be made more interactive and responsive as the evidence changes (e.g., http://www.cdc.gov/nutrition/professionals/researchtopractice/index.html [CDC, 2009a]).
An obesity-related example of engaging community members in the planning, delivery, and evaluation of interventions is the Shape Up Somerville environmental change intervention, designed to prevent obesity in culturally diverse, high-risk early elementary school children in Somerville, Massachusetts. One outcome of this initiative was a decrease in BMI z-scores among children at high risk for obesity in grades 1-3, a result of an intervention that aimed to bring participants’ energy equation into balance by modifying the school, home, and community environments to increase both physical activity options and the availability of healthful foods (Economos et al., 2007). Community members and groups engaged in the intervention included children, parents, teachers, school food service providers, city departments, policy makers, health care providers, restaurants, before- and after-school programs, and the media. Another strategy of Shape Up Somerville was to improve school food service, which led to changes that enhanced the nutrient profiles of and attitudes toward school meals. The engagement of students, parents, teachers, school leaders, and food service personnel was an integral part of the process (Goldberg et al., 2009). There is also a growing literature on how to combine systematic reviews of quantitative and qualitative evidence with realist reviews of theoretical assumptions and with the practical experience of those who must make the final decisions on local action (Ogilvie et al., 2005). For example, Mays and colleagues (2005, p. 7) offer:
-
“a description of the main stages in a systematic review of evidence from research and nonresearch sources designed to inform decision making by policy makers and managers;
-
an indication of the range of evidence that could potentially be incorporated into such reviews;
-
pragmatic guidance on the main methodological issues…, given the early stage of development of methods of such reviews, and with a particular focus on the synthesis of qualitative and quantitative evidence;
-
an introduction to some of the approaches available to synthesize these different forms of evidence; and
-
an indication of the types of review questions particular approaches to synthesis are best able to address.”
These alternatives to evidence-based practice of the most literal and rigorous scientific variety suggest some advantages and complementarities of a model of practice-based evidence that produces locally adapted and prospectively tested evidence. Users of this and other guidance for linking research to the decisions they must make in their own settings will need to trade off some degree of rigor for more reality in the setting, conditions of practice, and free-living populations observed.
REFERENCES
Abroms, L. C., and E. W. Maibach. 2008. The effectiveness of mass communication to change public behavior. Annual Review of Public Health 29:219-234.
Banwell, C., S. Hinde, J. Dixon, and B. Sibthorpe. 2005. Reflections on expert consensus: A case study of the social trends contributing to obesity. European Journal of Public Health 15(6):564-568.
Bartholomew, L. K., G. S. Parcel, G. Kok, and N. H. Gotleib. 2006. Planning health promotion programs: An intervention mapping approach. San Francisco, CA: Jossey-Bass Wiley.
Berkman, L. F., and I. Kawachi, eds. 2000. Social epidemiology. New York: Oxford University Press.
Best, A., D. Stokols, L. W. Green, S. Leischow, B. Holmes, and K. Buchholz. 2003. An integrative framework for community partnering to translate theory into effective health promotion strategy. American Journal of Health Promotion 18(2):168-176.
Booth, S. L., J. F. Sallis, C. Ritenbaugh, J. O. Hill, L. L. Birch, L. D. Frank, K. Glanz, D. A. Himmelgreen, M. Mudd, B. M. Popkin, K. A. Rickard, S. St Jeor, and N. P. Hays. 2001. Environmental and societal factors affect food choice and physical activity: Rationale, influences, and leverage points. Nutrition Reviews 59(3, Supplement 2):S21-S39.
Bowen, S., and A. B. Zwi. 2005. Pathways to “evidence-informed” policy and practice: A framework for action. PLoS Medicine 2(7):600-605.
Campbell, D. T., and D. A. Kenny. 1999. A primer on regression artifacts. New York: Guilford.
Campbell, D. T., and J. C. Stanley. 1966. Experimental and quasi-experimental designs for research. Chicago, IL: Rand McNally.
Cargo, M., and S. L. Mercer. 2008. The value and challenges of participatory research: Strengthening its practice. Annual Review of Public Health 29:325-350.
CDC (Centers for Disease Control and Prevention). 2009a. Nutrition resources for health professionals. http://www.cdc.gov/nutrition/professionals/researchtopractice/index.html (accessed January 4, 2010).
CDC. 2009b. Racial and ethnic approaches to community health. http://www.cdc.gov/reach/index.htm (accessed January 4, 2010).
Chatfield, C. 2004. The analysis of time series: An introduction. 6th ed. Boca Raton, FL: Chapman & Hall/CRC Press.
Cook, T. D. 2008. “Waiting for life to arrive”: A history of the regression-discontinuity design in psychology, statistics and economics. Journal of Econometrics 142(2):636-654.
Dobbins, M., S. Jack, H. Thomas, and A. Kothari. 2007. Public health decision-makers’ informational needs and preferences for receiving research evidence. Worldviews on Evidence-Based Nursing 4(3):156-163.
Dobrow, M. J., V. Goel, and R. E. G. Upshur. 2004. Evidence-based health policy: Context and utilisation. Social Science and Medicine 58(1):207-217.
Dobrow, M. J., V. Goel, L. Lemieux-Charles, and N. A. Black. 2006. The impact of context on evidence utilization: A framework for expert groups developing health policy recommendations. Social Science and Medicine 63(7):1811-1824.
D’Onofrio, C. N. 2001. Pooling information about prior interventions: A new program planning tool. In Handbook of program development for health behavior research and practice, edited by S. Sussman. Thousand Oaks, CA: Sage Publications. Pp. 158-203.
Economos, C. D., R. R. Hyatt, J. P. Goldberg, A. Must, E. N. Naumova, J. J. Collins, and M. E. Nelson. 2007. A community intervention reduces BMI z-score in children: Shape Up Somerville first year results. Obesity 15(5):1325-1336.
French, S. A., M. Story, and R. W. Jeffery. 2001. Environmental influences on eating and physical activity. Annual Review of Public Health 22:309-335.
Goldberg, J. P., J. J. Collins, S. C. Folta, M. J. McLarney, C. Kozower, J. Kuder, V. Clark, and C. D. Economos. 2009. Retooling food service for early elementary school students in Somerville, Massachusetts: The Shape Up Somerville experience. Preventing Chronic Disease 6(3).
Green, L. W. 2006. Public health asks of systems science: To advance our evidence-based practice, can you help us get more practice-based evidence? American Journal of Public Health 96(3):406-409.
Green, L. W. 2008. Making research relevant: If it is an evidence-based practice, where’s the practice-based evidence? Family Practice 25 (Supplement 1):i20-i24.
Green, L. W., and R. E. Glasgow. 2006. Evaluating the relevance, generalization, and applicability of research: Issues in external validation and translation methodology. Evaluation & the Health Professions 29(1):126-153.
Green, L. W., and M. W. Kreuter. 2005. Health program planning: An educational and ecological approach. 4th ed. New York: McGraw-Hill Higher Education.
Greenhalgh, T., E. Kristjansson, and V. Robinson. 2007. Realist review to understand the efficacy of school feeding programmes. British Medical Journal 335(7626):858-861.
Hahn, J., P. Todd, and W. Van Der Klaauw. 2001. Identification and estimation of treatment effects with a regression-discontinuity design. Econometrica 69(1):201-209.
Hennigan, K. M., M. L. Del Rosario, L. Heath, T. D. Cook, J. D. Wharton, and B. J. Calder. 1982. Impact of the introduction of television on crime in the United States: Empirical findings and theoretical implications. Journal of Personality and Social Psychology 42(3):461-477.
Horowitz, C. R., M. Robinson, and S. Seifer. 2009. Community-based participatory research from the margin to the mainstream: Are researchers prepared? Circulation 119(19):2633-2642.
Imbens, G. W., and T. Lemieux. 2008. Regression discontinuity designs: A guide to practice. Journal of Econometrics 142(2):615-635.
IOM (Institute of Medicine). 2007. Progress in preventing childhood obesity: How do we measure up? Edited by J. Koplan, C. T. Liverman, V. I. Kraak, and S. L. Wisham. Washington, DC: The National Academies Press.
Khuder, S. A., S. Milz, T. Jordan, J. Price, K. Silvestri, and P. Butler. 2007. The impact of a smoking ban on hospital admissions for coronary heart disease. Preventive Medicine 45(1):3-8.
Kreuter, M. W. 1992. PATCH: Its origin, basic concepts, and links to contemporary public health policy. Journal of Health Education 23(3):135-139.
Lightwood, J. D., D. Collins, H. Lapsley, and T. E. Novotny. 2000. Estimating costs of tobacco use. In Tobacco control in developing countries, edited by P. Jha and F. J. Chaloupka. Oxford: Oxford University Press. Pp. 63-103.
Ludwig, J., and D. L. Miller. 2007. Does Head Start improve children’s life chances? Evidence from a regression discontinuity design. Quarterly Journal of Economics 122(1):159-208.
Mays, N., C. Pope, and J. Popay. 2005. Systematically reviewing qualitative and quantitative evidence to inform management and policy-making in the health field. Journal of Health Services Research and Policy 10(Supplement 1):6-20.
McLeroy, K. R., D. Bibeau, A. Steckler, and K. Glanz. 1988. An ecological perspective on health promotion programs. Health Education Quarterly 15(4):351-377.
Minkler, M., and N. Wallerstein, eds. 2008. Community-based participatory research for health: From process to outcomes. 2nd ed. San Francisco: Jossey-Bass.
National Committee on Vital and Health Statistics. 2002. Shaping a vision of health statistics for the 21st century. Hyattsville, MD: National Center for Health Statistics.
NCI and SAMHSA (National Cancer Institute and the Substance Abuse and Mental Health Services Administration). 2009. Research-tested intervention programs. http://rtips.cancer. gov/rtips/index.do (accessed January 4, 2010).
Ogilvie, D., M. Egan, V. Hamilton, and M. Petticrew. 2005. Systematic reviews of health effects of social interventions: 2. Best available evidence: How low should you go? Journal of Epidemiology and Community Health 59(10):886-892.
Ottoson, J. M., and L. W. Green. 1987. Reconciling concept and context: Theory of implementation. Advances in health education and promotion 2:353-382.
Ottoson, J. M., L. W. Green, W. L. Beery, S. K. Senter, C. L. Cahill, D. C. Pearson, H. P. Greenwald, R. Hamre, and L. Leviton. 2009. Policy-contribution assessment and field-building analysis of the Robert Wood Johnson Foundation’s Active Living Research Program. American Journal of Preventive Medicine 36(2, Supplement):S34-S43.
Parcel, G. S. 1987. Smoking control among women: A CDC community intervention handbook. Atlanta, GA: Centers for Disease Control, Center for Health Promotion and Education, Division of Health Education.
Reichardt, C. S. 2006. The principle of parallelism in the design of studies to estimate treatment effects. Psychological Methods 11(1):1-18.
Reynolds, K. D., and S. G. West. 1987. A multiplist strategy for strengthening nonequivalent control group designs. Evaluation Review 11(6):691-714.
Richard, L., L. Potvin, N. Kishchuk, H. Prlic, and L. W. Green. 1996. Assessment of the integration of the ecological approach in health promotion programs. American Journal of Health Promotion 10(4):318-328.
Roos, Jr., L. L., N. P. Roos, and P. D. Henteleff. 1978. Assessing the impact of tonsillectomies. Medical Care 16(6):502-518.
Rosenbaum, P. R. 2002. Observational studies. 2nd ed. New York: Springer.
Rosenbaum, P. R., and D. B. Rubin. 1983. The central role of the propensity score in observational studies for causal effects. Biometrika 70(1):41-55.
Rubin, D. B. 1977. Assignment to treatment group on the basis of a covariate. Journal of Educational Statistics 2(1):1-26.
Rubin, D. B. 2006. Matched sampling for causal effects. New York: Cambridge.
Sallis, J. F., N. Owen, and E. B. Fisher. 2008. Ecological models of health behavior. In Health behavior and health education: theory, research, and practice. 4th ed, edited by K. Glanz, B. K. Rimer and K. Viswanath. San Francisco, CA: Jossey-Bass.
Schaalma, H., and G. Kok. 2006. A school HIV-prevention program in the Netherlands. In Planning health promotion programs: An intervention mapping approach, edited by L. K. Bartholomew, G. S. Parcel, G. Kok, and N. H. Gottleib. San Francisco, CA: Jossey-Bass. Pp. 511-544.
Schafer, J. L., and J. Kang. 2008. Average causal effects from nonrandomized studies: A practical guide and simulated example. Psychological Methods 13(4):279-313.
Shadish, W. R., and T. D. Cook. 1999. Design rules: More steps toward a complete theory of quasi-experimentation. Statistical Science 14:294-300.
Shadish, W. R., T. D. Cook, and D. T. Campbell. 2002. Experimental and quasi-experimental designs for generalized causal inference. Boston: Houghton Mifflin.
Simons-Morton, B. G., G. S. Parcel, N. M. O’Hara, S. N. Blair, and R. R. Pate. 1988a. Health-related physical fitness in childhood: Status and recommendations. Annual Review of Public Health 9:403-425.
Simons-Morton, B. G., G. S. Parcel, and N. M. O’Hara. 1988b. Implementing organizational changes to promote healthful diet and physical activity at school. Health Education Quarterly 15(1):115-130.
Simons-Morton, B. G., W. H. Green, and N. H. Gottleib. 1995. Introduction to health education and health promotion. 2nd ed. Prospect Heights, IL: Waveland Press.
Simons-Morton, D. G., B. G. Simons-Morton, G. S. Parcel, and J. F. Bunker. 1988a. Influencing personal and environmental conditions for community health: A multilevel intervention model. Family and Community Health 11(2):25-35.
Simons-Morton, D. G., S. G. Brink, G. S. Parcel, K. Tiernan, C. M. Harvey, and J. M. Longoria. 1988b. Promoting physical actvity among adults: A CDC community intervention handbook. Atlanta, GA: Centers for Disease Control.
Trochim, W. 1984. Research design for program evaluation: The regression-discontinuity approach. Newbury Park, CA: Sage.
West, S. G., and F. Thoemmes. 2010. Campbell’s and Rubin’s perspectives on causal inference. Psychological Methods 15(1):18-37.
West, S., J. Biesanz, and S. Pitts. 2000. Causal inference and generalization in field settings: Experimental and quasi-experimental designs. In Handbook of research methods in social and personality psychology, edited by H. Reis and C. Judd. Cambridge: University Press. Pp. 40-84.
West, S., J. Duan, W. Pequegnat, P. Gaist, D. Des Jarlais, D. Holtgrave, J. Szapocznik, M. Fishbein, B. Rapkin, M. Clatts, and P. Mullen. 2008. Alternatives to the randomized controlled trial. American Journal of Public Health 98(8):1359-1366.
Wu, W., S. G. West, and J. N. Hughes. 2008a. Effect of retention in first grade on children’s achievement trajectories over 4 years: A piecewise growth analysis using propensity score matching. Journal of Educational Psychology 100(4):727-740.
Wu, W., S. G. West, and J. N. Hughes. 2008b. Short-term effects of grade retention on the growth rate of Woodcock-Johnson III broad math and reading scores. Journal of School Psychology 46(1):85-105.