4
Case Studies
INTRODUCTION
The committee undertook case studies to illustrate the use of the recommended biomarker evaluation framework. Five case studies are presented in this chapter, each highlighting one or more aspects of the framework. The first case study is tumor volume in cancer, which highlights the need for rigorous analytical validation. The second case study is C-reactive protein (CRP), which highlights that data are crucial to ascertaining whether a biomarker can be more than a prognostic factor. The third case study is troponin, which highlights the utility of biomarkers for which sufficient data for use of the biomarker as a surrogate endpoint do not exist. The fourth case study is on low-density lipoprotein (LDL) and high-density lipoprotein (HDL) cholesterol, which highlights how even biomarkers frequently used as surrogate endpoints need to be carefully evaluated prior to each use. Finally, the fifth case study is on beta-carotene, which contains lessons for each step of the qualification framework. In particular, beta-carotene highlights the importance of biomarkers in nutrition-related settings.
Table 4-1 gives a brief summary of the results of the case studies. As can be seen, biomarkers are useful for a variety of purposes. In order for a biomarker to be used as a surrogate endpoint, however, a strong understanding of the causal pathways of the disease process and of an intervention’s intended and unintended effects are usually needed. Achieving such understanding is a daunting challenge, and the committee acknowledges that it is infrequent that this understanding is achieved. The case
TABLE 4-1 Brief Summary of the Results of the Case Studies
Biomarker |
Analytical Validity |
Qualification |
Utilization: Possible Uses |
Utilization: Surrogate Endpoint Use |
Tumor Size |
No |
Numerous trials exist with inconsistent findings on tumor shrinkage and clinical benefit |
Data needed to improve analytical validity of one or more test |
Current data do not support use as a surrogate endpoint |
CRP |
High-sensitivity tests available |
RCTs and observational studies available; limited data on CRP’s biological role in disease progression |
Risk prediction; potential expansion of statin treatment to specific populations |
Current data does not support use as a surrogate endpoint |
Troponin |
Validated tests are available for many uses. More sensitive tests are also being developed |
Extensive data for acute troponin, limited data on chronic troponin; data collection is ongoing |
Safety uses |
Current data does not support use as a surrogate endpoint |
studies chosen are generally ones for which a great deal of data already exist, and in a number of the case studies, the biomarkers have been discussed for several decades. However, the case studies illustrate that even in these situations the lack of sufficient data for surrogate endpoint status for the biomarkers is evident. Readers of these case studies may wonder what lessons can be gained toward prospective evaluation of biomarkers. For a newly discovered biomarker, it is likely that very little data will be available for review in the analytical validation and qualification steps of the evaluation framework. In these situations, the lack of data should be noted. During the utilization step of the framework, then, needs for further data are identified. After these data are collected, the evaluation process can be revisited until the data available support the use for which the biomarker is proposed.
It should be emphasized that these case studies are illustrative. Complete, rigorous, systematic reviews of the evidence base were not conducted by the committee. Each case study first introduces general information about the biomarker itself. Analytical validation, qualification, and utilization analyses are then discussed. Finally, a summary of the lessons learned through each case is given.
Biomarker Discovery and Development
Although many candidate biomarkers have been reported, few have been sufficiently evaluated to justify their use in developing drugs or making treatment decisions. This slow pace has been attributed to the challenges posed by the discovery and development processes. The discovery process is dependent on the technologies available to interrogate complex biochemistry of health and disease, and identifying differences that can be detected consistently in diverse populations (IOM, 2007). Advances in the fields of genomics and proteomics have made it easier to interrogate hundreds or even thousands of potential biomarkers at once, leading to large datasets requiring sophisticated analyses to identify individual biomarkers of interest, or patterns of markers. A recent IOM committee determined that realizing the full potential of biomarker-based tools is dependent on progress in biomarker discovery (IOM, 2007). However, technologies to identify and quantify proteins and metabolites have lagged behind methods to assess nucleic acids because of the diverse biochemical characteristics of the protein and metabolic products of the human genome. Beyond technology platforms, the committee also discussed the need to develop new software packages, algorithms, and statistical and computational models capable of integrating data from multiple inputs, such as proteomic or genomic data from the same samples.
Drug and diagnostic industries, along with academic researchers,
are involved in biomarker discovery activities. In drug development, biomarkers may be used in target validation, or in demonstrating that a potential drug target plays a key role in the disease process; early compound screening, identifying compounds with the most promise for safety and efficacy; pharmacodynamic assays to assess drug activity and select schedule/dose; patient selection; and surrogacy (IOM, 2007). Because therapeutics are generally only effective in a subset of patients, drug and diagnostic industries may develop (or in some cases, codevelop therapeutics and diagnostics) assays to assess which subset of patients would most benefit from a therapeutic. However, once a drug is approved, there is less financial incentive to develop biomarkers to guide treatment decisions because it would likely restrict the number of patients taking the drug.
TUMOR SIZE AS BIOMARKER FOR CANCER CLINICAL ENDPOINTS
Biomarkers play several roles in patient care in the context of cancer, as discussed in the Institute of Medicine’s (IOM’s) Cancer Biomarkers report (IOM, 2007). In patients who do not have a cancer diagnosis, biomarkers can be used for risk stratification, prevention of carcinogenesis in precancerous tissues, and screening for early-stage tumors. Biomarkers aid in making a diagnosis of cancer, classifying a particular patient’s disease, and determining disease prognosis. In the context of a particular treatment, biomarkers are used for treatment stratification (treatment decisions based on patient characteristics), risk management (regarding adverse effects of a therapy), monitoring effectiveness or side effects of a therapy, and post-treatment disease surveillance. One metric used as a biomarker in cancer care, in the absence of or in conjunction with molecular markers, is tumor size measured with anatomic imaging, most meaningfully expressed in terms of tumor volume (Lin et al., 2008; Van Beers and Vilgrain, 2008).
Tumor response rates, defined by a change in tumor bulk, were commonly used for making decisions regarding approval of anticancer drugs in the 1970s, but in the mid-1980s, the Food and Drug Administration (FDA) added a requirement that a clinical survival benefit or quality-of-life benefit should be demonstrated. Because long trials are usually needed to demonstrate significant survival benefit and the demand for new anticancer drugs is always urgent, in 1996 the FDA extended Accelerated Approval under subpart H of the New Drug Application for drugs that are effective against serious or life-threatening diseases as measured by surrogate endpoints to anticancer drugs (HHS, 1996).1 This included
surrogate endpoints such as tumor size as it is represented in composite endpoints such as progression-free survival and time to progression. Accelerated approval is granted with the understanding that confirmatory evidence gathered in postmarket trials will lead to traditional approval of the drug, and a lack of such evidence may result in its removal from the market by the FDA.
Lathia et al. (2009) recently noted that “between 1992 and 2004, 22 applications for 18 anticancer drug or biologic agents were granted accelerated approval in the United States. These approvals were generally granted on the basis of end points such as overall response rate, time to progression, and disease-free survival. Of the 22 applications that received accelerated approval before January 2004, 6 were converted to regular approvals (i.e., demonstrated an effect on survival/outcome) whereas the remaining 16 were not converted to regular approvals; all these agents remain on the market.”
While the outcome measured in phase III cancer trials is often overall survival, surrogate endpoints play a large role in evaluation of new therapeutic agents in phase II clinical trials (Ratain et al., 1993; Sargent et al., 2009; Scher et al., 2008; Seibert et al., 2007). A primary endpoint commonly reported in phase II trials for cancer therapeutics is response rate, defined in its most primitive form as tumor shrinkage. Unfortunately, phase II results based on tumor shrinkage are not always predictive of outcomes in phase III trials. In the case of agents with low response rates in phase II that go on to show an increase in progression-free survival or overall survival in phase III trials, speculation has been that this result may be due to tumor stabilization rather than tumor shrinkage by these therapeutic agents. This would suggest that although tumor shrinkage is an important variable to monitor, the way response rates are measured in phase II trials is failing to capture all clinically meaningful changes that should be considered in the drug evaluation process (Dhani et al., 2009; Llovet et al., 2008; Stewart, 2008; Weber, 2009).
Tumor size is an inconsistently defined biomarker often used for determining efficacy of cancer therapeutics (Marcus et al., 2009). Validation, qualification, and utilization analyses are complicated by use of multiple imaging platforms (hardware), nonstandardized acquisition and analysis protocols (software), dissimilar contrast agents and targeted imaging agents across trials and institutions, and inconsistent methods for measuring, calculating, and reporting tumor size.
Tumor Size: Analytical Validation
Tumor size measurements reported include tumor diameter, volume, and mass, as measured using anatomic imaging modalities such as mag-
netic resonance imaging (MRI), computed tomography (CT), ultrasound (US), and mammography (Strassburg et al., 2008). Validating use of tumor size as a biomarker is difficult because it is measured and defined in different ways depending on the imaging modality, the type of tumor, and the institution (Tran et al., 2004). Tumor size is sometimes expressed as diameter of the tumor in one or two views. Such values can also be used to approximate tumor volume using a spherical, cuboidal, prolate spheroid, or oblate spheroid model. However, many solid tumors are of irregular shape, and their volume can be best approximated by measuring tumor diameter in three (if possible) orthogonal views and using an elliptoid model to estimate tumor volume. A growing body of literature is advocating for the use of elliptoid modeling of tumor volume as the most meaningful representation of tumor size in terms of its accurate reflection of changes in tumor bulk confirmed by other volumetric measurements, such as water displacement and its correlation to clinical endpoints. However, some widely used standardized response criteria, such as the Response Evaluation Criteria in Solid Tumors (RECIST), employ a sum of the longest dimension recorded of each tumor when attempting to quantify disease burden (Eisenhauer et al., 2009; Gehan and Tefft, 2000).
A newer and more accurate approach to estimating tumor volume involves using two-dimensional tumor contours on sequential imaging slices to calculate volume in three dimensions. This technique can be used with MRI, CT, and positron emission tomography–computed tomography (PET–CT) images. Tumors are outlined on each slice manually or with automatic model-based segmentation and compiled to estimate gross tumor volume. This technique, particularly with implementation of automatic model-based segmentation to reduce interobserver discordance, provides a platform for accurately measuring tumor volume in a way that is reproducible and can be standardized relatively easily (Galanis et al., 2006).
Tumor mass can also be approximated using an estimation of tumor volume. This may be a useful metric in a laboratory setting where such quantities can be confirmed using ex vivo measures, or when tumor size is measured with anatomic imaging and tumor density is measured with functional imaging, such as PET, and the two measures are combined to estimate tumor mass. In cases, however, where mass is extrapolated from volume using an estimate of density, this calculation may introduce another source of error.
To further complicate measurement of tumor size, tumor borders are often poorly demarcated in highly invasive cancers, resulting in ambiguity about diameter length and interobserver discordance. For treatment evaluation the most emphasis should be put on reproducibility and accuracy of serial measurements; in this case reproducibility includes stan-
dardizing data collection across institutions and trials so that meaningful comparisons can be made between populations of patients. The variability in imaging platforms and techniques makes it unlikely that step one of the qualification framework is fulfilled given the current lack of standardization in the field. Adherence to American College of Radiology Appropriateness Criteria regarding appropriate modalities for imaging various types of cancer at different points along disease progression and treatment is one effort that could decrease discrepancies in data collection across trials (Böhm-Vélez et al., 2000; Fishman et al., 2000; Javitt, 2007). Because standardizing the hardware used at individual institutions may be difficult, it may be more feasible to standardize imaging acquisition and analysis protocols within a multicenter trial, and certainly within institutions (Grossi et al., 2004). Finally, some have explored the use of Bayesian analysis techniques to improve the accuracy of conclusions drawn from tumor images and other clinical data (Vokurka et al., 2002; Yang et al., 2003).
Tumor Size: Qualification
Because the growth of local or metastatic cancer cells can lead to the death of the host, it is biologically plausible that shrinkage of the existing tumor or prevention of further growth could serve as indications of biological and clinical benefit. However, many hypotheses exist regarding how cancer causes death in an organism (Lichtenstein, 2005). Some cancers cause death because cancer cells, much like parasites, compete with native tissue for nutrients, so that the organism essentially starves. Tumors frequently interfere with physiologic processes through mass effect, such as compression of vessels and other luminal structures or intracranial compression of brain tissue, or through invasion of normal tissue, which can result in clinical disease and death of the organism. Paraneoplastic syndromes and immune response to neoplastic cells also play a role in the mortality and morbidity of many types of cancer.
Given the contributions of these and other factors, the biological plausibility of using tumor size as a surrogate endpoint for evaluating disease progression and therapeutic efficacy in cancer is not entirely obvious. Smaller tumors tend to grow faster, so major shrinkage of tumor mass does not necessarily translate to prolonged survival (Citron, 2004; Hudis, 2005). Data have shown that tumor size may not correlate with long-term clinical outcome in some cancers, such as in locally advanced breast cancer, where lack of nodal involvement is predictive of disease-free survival and overall survival rates, but tumor size does not affect these rates (Beenken et al., 2003; Berruti et al., 2008). Additionally, real clinical benefit is not always accompanied by measurable reduction in
tumor size, as is the case with cytostatic drugs or agents that reduce the density of cells within a tumor but leave the tumor volume unchanged (Young et al., 1999). Even in the case of treatment with conventional cytotoxic drugs, initial tumor shrinkage is nearly always followed by tumor cell repopulation (Kerbel, 2006).
In the case of many biomarkers or surrogate endpoints, a causal role for the biomarker in the disease pathway is established. LDL, for example, is hypothesized to have a causal role in the atherosclerotic disease process, and while this has not been conclusively proven, LDL is measured as a biomarker of atherosclerotic cardiovascular disease and targeted pharmaceutically. Clearly tumor size is a different brand of surrogate endpoint from most molecular biomarkers in that increasing tumor size is viewed as a result of disease progression, not a causative factor. The exception to this mode of thinking about tumor size is tumors that secrete biologically active factors that promote proliferation via autocrine or paracrine signaling; in this case tumor growth may beget tumor growth while adequate vascular supply exists to support it (Imamoto et al., 1991).
In many studies tumor size is used as an indicator of response rate and for determining time to progression and disease-free survival (Ohara et al., 2002; Ollivier et al., 2007; Pugnale et al., 2003). While the link between tumor size and clinical benefit is less firm than what is traditionally required for associating a biomarker with a particular clinical endpoint (Therasse et al., 2006), use of tumor size as a biomarker in cancer has been rationalized by the serious nature of the disease and a lack of more solidly linked prognostic indicators. It is important to emphasize, however, that this rationalization is not universally accepted (Fleming et al., 2009). As will be discussed in Chapter 5, the in situations where it is deemed reasonable to permit marketing of drugs before clinical outcome evidence is available, it is important that this data be collected and analyzed through postmarket studies. In cancers where tumors shrink predictably in response to efficacious cytotoxic therapy, serial tumor-size measurements can provide insight into whether a new therapeutic agent or technique warrants further study or whether a particular patient or patient population is likely to benefit from that therapy (Henson et al., 2005; Husband et al., 2004; Kamel and Bluemke, 2002; Karrison et al., 2007). For example, in the case of locally advanced breast carcinoma treated with cytotoxic agents, tumor volume calculated using measurements taken with US, mammography, or MRI have been demonstrated to be prognostic and can also aid in selecting an effective treatment regimen (Berruti et al., 2005; Buijs et al., 2007; Cheung et al., 2003; Dose Schwarz et al., 2005; Eng-Wong et al., 2008; Hylton, 2006; Noterdaeme et al., 2009). Similarly, tumor volume is a critical measurement for monitoring and
directing local control of non-small-cell lung cancer (NSCLC) with radiation therapy.
The response rate often reported in phase II trials is based on an incorrect premise that tumor size is analogous or proportional to the number of tumor cells, as described in RECIST (Desar et al., 2009; Park et al., 2003; Tuma, 2006). The Choi Criteria, which were originally developed to assess tumor progression in gastrointestinal stromal tumors (GISTs), incorporate tumor size and density (measured with contrast-enhanced CT) into a metric of tumor progression. The Choi Criteria are a more sensitive measure of responsiveness to a particular therapy and have been demonstrated to more accurately predict overall survival in GIST than reduction in tumor size (Benjamin et al., 2007; Choi, 2005; Choi et al., 2007; Hohenberger and Wardelmann, 2006; Sevinc and Turhal, 2008; Stacchiotti et al., 2009).
The Southwest Oncology Group developed new criteria for evaluation of response in NSCLC that define response to therapy as anything other than progression. Patients who demonstrate a decrease in tumor size or who have stable disease are considered nonprogressive, and in NSCLC this measure of “disease control rate” is more predictive of overall survival than tumor shrinkage. The North Central Cancer Treatment Group and National Surgical Adjuvant Breast and Bowel Project (NSABP) have similarly used nonprogression at a specific time point as a measure of response to therapy that is more predictive of overall survival than tumor shrinkage (Tuma, 2006).
Tumor Size: Utilization
Cancer is a complex collection of diseases, which makes it difficult to make generalizations about how a particular surrogate endpoint should be used in trials for all types of cancer. One caveat to using tumor shrinkage as a surrogate endpoint is that it may not represent clinical benefit in all situations. In the case of GIST, progression usually occurs within the original tumor boundaries. Treatment of these tumors with Gleevec (imatinib) results in decreased cell density within the tumor and prolonged patient survival, but rarely shrinks measurable diameters of existing tumors to a significant degree. In this example Gleevec is thought to have both cytotoxic and cytostatic effects, and GIST cells are replaced by myxoid degeneration following cell death, both reasons why tumor size as a surrogate endpoint correlates poorly with clinical endpoints (Benjamin et al., 2007; Choi, 2005).
Factors to consider for contextual analysis of tumor size as a surrogate endpoint include the following: (1) when in a patient’s treatment this variable is considered, and (2) for what purposes (Cademartiri et al., 2008; Christensen, 2008). In some cancers, tumor size is a useful diagnostic
and prognostic biomarker. For some cancers, though, imaging tumor size does not play a significant role in prognosis at the time of diagnosis. In the context of locally advanced breast cancer, for example, nodal involvement has a greater role in prognostication than tumor size (Beenken et al., 2003). NSABP has established criteria using pathologic complete response, which is defined as no evidence of malignancy on histologic analysis, instead of tumor shrinkage measured with anatomic imaging, to predict long-term prognosis over the course of disease. Obviously pathologic complete response cannot be evaluated for all cancers in all sites at all points along the history of the disease, which is why imaging has such an enormous role in monitoring response to therapy. In some types of breast cancer, for example, monitoring tumor size with imaging is tremendously useful for gauging efficacy of a particular therapy (Berruti et al., 2005, 2008; Buijs et al., 2007; Cheung et al., 2003; Eng-Wong et al., 2008; Hylton, 2006; Nicoletto et al., 2008).
Tumor Size: Lessons Learned
Although tumor shrinkage does not positively correlate with clinical benefit in all situations, the patchy qualification of tumor shrinkage as a surrogate endpoint for cancer trials has been tolerated by regulatory agencies for several reasons. Cancer as a family of diseases continues to result in high mortality and morbidity. Truly novel and efficacious therapeutics are not emerging as rapidly as society demands. Conditional approvals based on tumor size are not always followed by full approvals, but when measured correctly and used in the appropriate context, perhaps in conjunction with other variables like tumor density, tumor size is a useful parameter for detecting clinical benefit (Jensen et al., 2008; Monteil et al., 2009; Specht et al., 2007). Even so, use of tumor size as a surrogate endpoint for regulatory approvals is decreasing and is being replaced by other, better qualified surrogate endpoints. These surrogates, including progression-free survival, also require postmarket studies to connect the interventions to beneficial changes in clinical outcomes.
Tumor size as a surrogate endpoint highlights the many analytical validation issues of imaging biomarkers. Validation standards for imaging biomarkers should vary depending on their intended use as surrogate endpoints; criteria should be more stringent for the purposes of drug registration than for earlier stages of drug development. Emerging molecular and functional imaging technologies will likely provide tools to address some of the deficiencies of anatomic imaging in cancer discussed here (Funaioli et al., 2007; Goldstein et al., 2005; Pantaleo et al., 2008a, 2008b; Schepkin et al., 2006; Ullrich et al., 2008; Wahl et al., 2009). Combined with functional imaging technologies like PET and targeted molecular
agents, and with dynamic imaging technologies like perfusion and diffusion MRI, anatomic imaging in the future may serve as a more reliable surrogate endpoint in clinical trials for cancer (Carrió, 2008; Jennings et al., 2008; Leimgruber et al., 2006; Noterdaeme et al., 2009; Sharma et al., 2009; Stadler and Ratain, 2000; Stegger et al., 2008).
C-REACTIVE PROTEIN
Although cardiovascular disease mortality has fallen over the past century, cardiovascular disease (CVD) remains the leading cause of death in the United States (Mensah and Brown, 2007). The aging of the population, decline in the case-fatality rate of cardiovascular disease, and a relatively stable incidence of cardiovascular disease has also translated to a higher prevalence of cardiovascular disease in the United States (Pearson, 2007). An estimated 80 million American adults have one or more types of CVD, with an estimated 38 million of these cases in individuals 60 years or older (Lloyd-Jones et al., 2009). According to one estimate by the American College of Cardiology, the prevalence of chronic heart conditions will grow approximately 16 percent a decade for the next three decades (Foot et al., 2000). The burden of high prevalence of disease—in terms of morbidity, lost productivity, monetary cost, and increased use of healthcare services—has prioritized the need for improved prevention, risk assessment, and treatment of heart disease.
Traditionally, the prevention of cardiovascular disease has occurred through lowering risk factors associated with the development of CVD (Krumholz and Lee, 2008). The concept of risk factors was formalized by the Framingham Heart Study (FHS), a cohort study initiated in 1948 to assess the development of CVD over a long period of time in individuals who had not yet developed overt symptoms of CVD or suffered a heart attack or stroke (FHS, 2009; Kannel et al., 1961). Studies such as the FHS were able to demonstrate that hyperlipidemia and high blood pressure precede the development of CVD, and they are also associated with a higher risk of disease development. Compelling epidemiological and clinical trial evidence has demonstrated that smoking, hyperlipidemia, high blood pressure, and diabetes mellitus are independent risk factors for CVD, and therefore are considered the “traditional” risk factors for the disease (HHS, 1990; MacMahon et al., 1990; Stamler et al., 1993; Verschuren et al., 1995). Greenland et al. (2003) found that exposure to at least one clinically elevated traditional risk factor ranged from 87 to 100 percent for fatal coronary heart disease (CHD) in three prospective cohort studies while Khot et al. (2003) observed a prevalence of traditional risk factors of 80 to 90 percent of among patients with CHD.
Some have suggested that traditional risk factors (e.g., smoking,
hyperlipidemia, high blood pressure, and diabetes mellitus) do not fully explain cardiovascular risk. In the United States, each year 800,000 individuals will have a myocardial infarction (MI) and 700,000 will experience stroke, yet nearly half of these events occur in individuals without evidence of overt hyperlipidemia (Thom et al., 2006). On a population level, plasma total cholesterol levels poorly discriminate risk for coronary heart disease: 35 percent of CHD occurs among individuals with below-average levels of total cholesterol (Castelli, 1996). Khot et al. (2003) found that around 50 percent of subjects have zero or only one traditional risk factor for cardiovascular disease. In addition, many individuals with multiple risk factors never develop cardiovascular disease; Greenland et al. found that exposure to one or more of the traditional risk factors was highly prevalent among individuals who did not develop clinical CHD (Greenland et al., 2003), suggesting that additional work is needed to identify new risk prediction strategies. New biomarkers for cardiovascular disease are sought to improve risk prediction, to identify potential therapeutic targets, and to provide a more complete understanding of the pathophysiology of disease. Despite some controversy over the utility of new biomarkers in risk prediction (Greenland et al., 2003; Wang et al., 2006; Welsh et al., 2008), new biomarkers continue to be sought in hopes that the disease burden of cardiovascular disease can be mitigated by better identifying and stratifying those individuals at risk and intervening with better therapeutic targets.
As the understanding of cardiovascular disease has evolved to include the impact of inflammation on the progression of disease, inflammatory biomarkers have received substantial attention. Inflammation is believed to contribute to different stages in the pathogenesis of coronary heart disease, including a role in the development and progression of atherosclerosis (reviewed by Casas et al., 2008; Packard and Libby, 2008; Ross, 1993, 1999). A popular hypothesis, supported by both laboratory and clinical data, suggests that LDL modified by oxidation or glycation facilitates an inflammatory response in the artery wall, activating biological cascades that contribute to atherosclerosis initiation, progression, and complication (Packard and Libby, 2008; Ross, 1999). The most extensively studied inflammatory biomarker at the population level is C-reactive protein, but many other inflammatory biomarkers have been identified, including fibrinogen, serum amyloid A, VCAM-1, tumor necrosis factor, interleukin (IL)-1, IL-6, IL-18, and lipoprotein-associated phospholipase A2, among others (Figure 4-1).
CRP is an acute phase, non-specific, systemic marker of inflammation. In normal individuals, CRP levels are low, but the serum concentration of CRP can increase upward of 1,000-fold upon exposure to a strong acute stimulus, such as sepsis or acute myocardial infarction (AMI), and
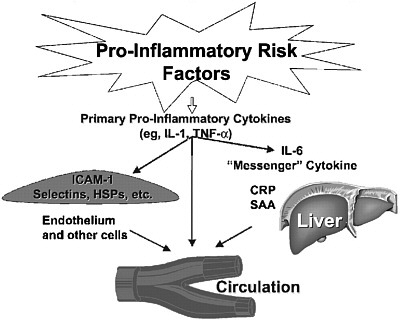
FIGURE 4-1 Inflammatory risk factors.
NOTE: CRP = C-reactive protein; HSP = heat-shock protein; ICAM-1 = intercellular adhesion molecule 1; IL-1 = interleukin 1; IL-6 = interleukin 6; SAA = serum amyloid A; TNF-α = tumor necrosis factor α.
SOURCES: Pearson et al. (2003). Reprinted with permission, Copyright 2003 by the American Heart Association. See also Libby and Ridker (1999).
can fall again when the stimulus is removed (Casas et al., 2008; Paffen and DeMaat, 2006). In the early 1990s, it was observed that individuals with active coronary syndromes and individuals with AMI who were tested prior to the acute-phase response to infarction were shown to have higher levels of CRP. The observation of the role of inflammation in cardiovascular disease and studies that revealed CRP predicted future coronary events began the current interest of CRP and cardiovascular disease (Pepys, 2005; Ridker et al., 1997).
CRP: Analytical Validation
CRP tests were first developed to measure acute phase responses of CRP, with detection limits around 2 to 10 mg/L. However, the newer assays were subsequently developed to measure CRP in the non-acute phase ranges, and are referred to as high sensitivity-CRP (hs-CRP) assays. These newer tests are the basis for measuring higher levels of CRP in the normal range associated with cardiovascular risk prediction, and com-
monly use CRP cutoffs of less than 1 mg/L, 1–3 mg/L, and greater than 3 mg/L to indicate low, average, and high cardiovascular risk.
CRP is easily measured via a number of standardized commercial hs-CRP assays, typically with detection limits of less than 0.3 mg/L and assay imprecision of less than 10 percent at low CRP concentrations (Roberts, 2004). In their 2003 scientific statement, the American Heart Association (AHA) and the Centers for Disease Control and Prevention (CDC) indicated that the hs-CRP assay was the best inflammatory assay candidate (Table 4-2) (Pearson et al., 2003). At the same time, the CDC published the first set of guidelines related to standardization of immunoassays for measurement of CRP (Kimberly et al., 2003). Sources of analytic variation for hs-CRP assays include laboratory methodology, reference material, precision, and calibration, among others (Ledue and Rifai, 2003). However, hs-CRP assay standardization efforts have continued (Kimberly et al., 2009), and the hs-CRP assay is currently considered standardized.
Specimen collection variables and physiologic characteristics are known to impact CRP measurement, but most research has indicated that CRP is a robust analyte that has negligible diurnal variation, does not depend on food intake, and has a long half-life (19 hours). Fresh, stored, and frozen plasma provide similar CRP measurement results (Ledue and Rifai, 2003). Physiologic characteristics, including race, ethnicity, age, sex, seasonality, biological variation, and lifestyle factors, have variable impact on CRP concentration. Some evidence suggests that men and women who are not receiving hormone replacement therapy have comparable CRP distributions (Ledue and Rifai, 2003), but other studies suggest that different gender subgroups have either lower or higher CRP concentrations. For instance, Japanese women may have slightly lower CRP concentrations (Yamada et al., 2001), while those of black females tend to be significantly higher (Albert et al., 2004). Likewise, research has also indicated that CRP concentrations vary by race and ethnicity, but there are limited data to evaluate the clinical relevance of these differences. Lifestyle factors that impact CRP levels include exercise, smoking, measures of adiposity, alcohol, anti-inflammatory drugs, and estrogen replacement therapy.
Despite these sources for variation in CRP concentration and measurement, CRP has proved to be a clinically useful measurement because it is an independent predictor of cardiovascular risk (Ridker, 2007), and there are widely available standardized, relatively low-cost hs-CRP assays that can be subjected to a number of collection variables, making CRP relatively easy for clinical use.
TABLE 4-2 Assays of Inflammatory Markers for Potential Clinical Usea
Analyte |
Stability |
Assay Availability |
World Health Organization Standards Available?b |
Interassay Precision |
Soluble adhesion molecules (e.g., E-selectin, P-selectin, intracellular adhesion molecule-1, vascular cell adhesion molecule-1) |
Unstable (unless frozen) |
Limited |
No |
CV<15% |
Cytokines (e.g., interleukin-1β, -6, -8, and -10 and tumor necrosis factor-α) |
Unstable (unless frozen) |
Few |
Yes (Gaines Das and Poole, 1993) |
CV<15% |
Acute-phase reactants Fibrinogen |
Unstablec (unless frozen) |
Many |
Yesd (Whitton et al., 2000) |
CV<8% |
SAA |
Stable |
One |
Yes (Poole et al., 1998) |
CV<9% |
hs-CRP |
Stable |
Many |
Yes (Whicher, 1998) |
CV<10% |
WBC count |
Stable |
Many |
Yes |
CV<3% |
NOTES: CRP = C-reactive protein; CV = coefficient of variation; SAA = serum amyloid A; WBC = white blood cell. a Courtesy of William Roberts, M.D., Ph.D. b Information on specific standards is available at the following World Health Organization website: http://www.who.int.biologicals. c In correctly anticoagulated blood, stable for at least 12 hours on ice or several hours at room temperature. d World Health Organization standard available only for mass assay, not for functional assays most commonly in use. SOURCE: Pearson et al. (2003). Reprinted with permission, Copyright 2003 by the American Heart Association. |
CRP: Qualification
Inflammatory biomarkers for cardiovascular disease are newly emerging, and have less evidence supporting their use than traditional biomarkers, such as LDL cholesterol or blood pressure. CRP is the most extensively studied inflammatory biomarker, but evidence gaps prevent full understanding of the biomarker. Although research indicates that CRP is an independent predictor of cardiovascular risk, it is not known whether CRP plays a causal role in cardiovascular disease, which creates uncertainty about its use as a potential therapeutic target and surrogate endpoint.
Although inflammation is clearly involved in the development of atherosclerosis, researchers have not definitively ascertained whether CRP plays an active role in the disease process. The role of CRP in human physiology is not fully understood (Nilsson, 2005). Because no CRP deficiency or structural polymorphism has been reported, nor have therapeutic interventions specifically inhibited human CRP in vivo, the effects of absence, inhibition, and lack of function of CRP have yet to be tested (Casas et al., 2008). Moreover, it is uncertain whether CRP is a bystander in the cardiovascular disease process or whether it plays a causal role in the pathophysiology of disease.
CRP has been shown to have prothrombotic and proinflammatory properties (Bisoendial et al., 2005; Pasceri et al., 2000), including the perpetuation and amplification of inflammation and the immune response (reviewed by Calabro et al., 2009). There are some concerns that studies demonstrating proinflammatory and prothrombotic effects of CRP have been confounded by contamination (Packard and Libby, 2008; Pepys, 2005; Taylor et al., 2005; Van den Berg and Taylor, 2005). However, other studies have demonstrated that contaminant-free CRP preparations have direct atherogenic effects (Singh et al., 2005; Yaron et al., 2006).
CRP can bind selectively to LDL and very low-density lipoprotein (VLDL), suggesting that CRP could potentially be involved in atherosclerosis (de Beer et al., 1982; Pepys et al., 1985; Zhang et al., 1999), and experimental studies showed that CRP avidly binds to modified LDL, which accumulates in atherosclerotic plaques (Bhakdi et al., 1999). Based on these observations, models were developed to test the effects of CRP on cardiovascular outcomes in animal systems. Paul and colleagues (2004) demonstrated that human CRP transgenic apolipoprotein E-knockout mice had larger aortic atherosclerotic lesions than control mice. However, with this same model, Hirschfield and colleagues did not detect any proatherogenic or proinflammatory effects of transgenic expression of human CRP (2003, 2005). Pepys et al. (2006) developed a small-molecule inhibitor of CRP and demonstrated that administration of the inhibitor to rats undergoing AMI abrogated increase in infarct size and cardiac dys-
function produced by injection of human CRP. However, it is important to note the limitations of these preclinical models: rats and mice have extremely low levels of native CRP and “[i]ntroduction of human CRP into animals, wherein the protein is interacting with xenogenic molecules, cells, physiological and pathological processes, cannot be assumed to be a robust test for functions of human CRP in humans” (Casas et al., 2008).
The JUPITER trial (Justification for the Use of Statins in Prevention: An Intervention Trial Evaluating Rosuvastatin) found that in individuals with CRP concentrations of 2.0 mg per liter or higher but LDL levels of less than 130 mg per deciliter,2 statin treatment significantly lowered the rate of first major cardiovascular events as compared to placebo (Ridker et al., 2008a). In a later analysis of the data, Ridker et al. (2009) found that JUPITER participants who achieved both LDL cholesterol level of less than 1.8 mmol/liter and CRP less than 1 mg/liter had a recorded 79 percent reduction in vascular event rates, while participants who achieved both LDL cholesterol level of less than 1.8 mmol/liter and CRP of less than 2 mg/liter had recorded a 62 percent reduction in vascular event rates. LDL cholesterol and CRP reductions were only weakly correlated with each other in this analysis. Although the JUPITER trial did not show that lowering CRP levels reduced cardiovascular risk, the trial does indicate that those patients with LDL levels of less than 130 mg per deciliter and CRP levels of greater than 2 mg per liter are at higher absolute risk and that rosuvastatin therapy resulted in a significant benefit in lowering cardiovascular events. Previous statin trials, including CARE (Cholesterol and Recurrent Events) and PRINCE (Pravastatin Inflammation/CRP Evaluation), also found that high CRP levels are significantly lowered with pravastatin therapy (Albert et al., 2001; Ridker et al., 1998). In the PRINCE study, no significant association was observed between baseline CRP and baseline LDL levels, end-of-study CRP and end-of-study LDL levels, or change in CRP and change in LDL levels over time; in linear regression analyses, only pravastatin therapy and baseline CRP levels were significant predictors of CRP reduction (Albert et al., 2001).
Some of the limitations of the JUPITER trial have been discussed in the literature, especially in relation to understanding the biological role of CRP. Hlatky (2008) noted that JUPITER trial entry criteria (apparently healthy men and women with LDL cholesterol of less than 130 mg per deciliter and CRP concentrations of 2.0 mg per liter or higher) provided only limited and indirect information about the biological role of CRP. The JUPITER trial did not compare subjects with CRP measurements of greater than 2.0 mg per liter with subjects having CRP measurements of
less than 2.0 mg per liter, nor did the trial compare the use of other markers of cardiovascular risk. Additionally, the trial did not evaluate whether individuals with CRP levels of less than 2.0 mg per liter would benefit from rosuvastatin treatment (Hlatky, 2008). However, the Air Force/Texas Coronary Atherosclerosis Prevention Study (AFCAPS/Tex-CAPS) found that lovastatin was ineffective among participants with a ratio of total to HDL cholesterol and a CRP level that were both lower than the median (Rikder et al., 2001). Ridker and colleagues (2009) acknowledge that it has not been determined the extent to which anti-inflammatory properties of statins affect clinical outcomes and whether these effects are independent of LDL cholesterol but suggest that it remains an intense area of research. Chan and colleagues (2009) note that the JUPITER trial provides “no results showing that C-reactive protein is an independent predictor of the relative or absolute benefit of therapy, since the treatment effects seen with rosuvastatin could have been mediated by reductions in low-density lipoprotein cholesterol.”
Additional studies suggest CRP is not involved in the disease process, including Mendelian randomization studies. Genetic data provide an opportunity to assess the causality of biomarkers for disease. For a biomarker that has a causal role, the expected random distribution in a population of a polymorphism that determines high or low biomarker concentrations would be skewed in individuals depending on their disease status. Data from so-called “Mendelian randomization” studies are accumulating for several biomarkers such as CRP. Indeed, Zacho et al. (2008) found that although polymorphisms in the CRP gene are associated with markedly higher CRP levels, genetic polymorphisms in the CRP gene were not associated with an higher risk of ischemic vascular disease. Arriving at a similar conclusion, Elliot et al. (2009) conducted a larger genome-wide association study that found a lack of concordance between CRP genotypes and effect on coronary heart disease risk. While these studies do not discount the role of inflammation in cardiovascular disease pathogenesis, they cast doubt on the causal role of elevated CRP levels in cardiovascular disease (Elliott et al., 2009; Nordestgaard, 2009; Shah et al., 2009), prompting some to suggest that CRP-targeted drug development efforts should be abandoned (Kolata, 2009). However, others argue that Mendelian randomization findings may be limited by alternative explanations for epigenetic phenomena (Ogbuanu et al., 2009) and low predictive ability for genes related to biomarkers.
Although the biological role of CRP in cardiovascular disease remains uncertain, CRP has been shown to be an independent predictor of cardiovascular risk; at least 30 population cohorts (Shah and deLemos, 2009) have found that higher levels of CRP in the normal range are associated with higher risks for future coronary events, includ-
ing MI, ischemic stroke, peripheral vascular disease, and vascular death (Calabro et al., 2009; Musunuru et al., 2008; Ridker, 2007). In addition to individual studies, four meta-analyses have been conducted to assess CRP’s independent predictive ability for future cardiovascular disease (Buckley et al., 2009; Danesh et al., 1998, 2000, 2004). Danesh et al. (2004) found that CRP is a relatively moderate predictor of coronary heart disease in comparison with traditional risk factors (such as higher LDL levels and cigarette smoking). The most recent meta-analysis (Buckley et al., 2009) concluded that strong evidence indicates CRP is independently associated with CHD events, and that moderate, consistent evidence suggests that adding CRP to risk prediction models improves risk stratification for those individuals initially at intermediate risk. The purpose of this meta-analysis was to assist the U.S. Preventive Services Task Force in determining whether CRP assessment should be incorporated into guidelines for cardiovascular risk assessment. Specifically, the authors focused on the potential benefit of adding CRP to models to improve risk stratification for those among intermediate risk because it would enable better tailoring of treatment decisions based on reclassification into either higher or lower risk categories. The meta-analysis found moderate evidence for adding CRP to prediction models for those at intermediate disease risk.
In spite of being an independent predictor of risk, some question the clinical utility CRP holds over the traditional risk factors (Folsom et al., 2006; Wang et al., 2006). The low incremental value that CRP and other new biomarkers have over traditional risk factors has been largely attributed to minimal impact on the area under the receiver operator curve, or c-statistic. However, others argue that this is an incorrect usage of the c-statistic, suggesting instead that reclassification into clinically relevant risk strata is a better way to assess prospectively the clinical impact of models (Cook, 2007, 2008; Cook et al., 2006). For example, adding CRP and family history to prediction models using traditional risk factors reclassifies 30 percent of individuals at intermediate risk into higher or lower levels of cardiovascular risk (Cook, 2008). The new risk strata were found to be better calibrated by comparing the predicted probabilities with the observed proportions within the reclassified categories.
CRP: Utilization
The third step of the committee’s qualification framework is a contextual analysis of the available evidence about a biomarker with regard to the specific proposed use of the biomarker. As discussed in Chapter 2 of this report, biomarkers have many uses in both clinical care and drug development, including for risk stratification, prevention, screening,
diagnosis, prognosis, patient selection, and pharmacodynamics (see Table 2-1). Potential uses of CRP include risk prediction, prevention, drug development activities, and as a surrogate endpoint for drug or health claim approval. Each of these circumstances requires differing levels of evidence.
For clinical use in risk prediction, several factors would need to be considered. First, the prognostic value would be tantamount; elevations in CRP levels would need to be definitively linked with increased risk for cardiovascular events. Other factors that could play an important role in the qualification of a biomarker for risk prediction would include the strength of the biomarker risk prediction capabilities compared to other biomarkers that predict risk (especially those indicating inflammation). Use of CRP for risk prediction could also depend on the incremental clinical value of including the biomarker test within the other methods of assessing risk. For example, the addition of CRP and parental history significantly improves global cardiovascular risk prediction with the Reynolds Risk Score for Men (Cook and Ridker, 2009; Ridker et al., 2008b), but other evaluations question the utility of emerging biomarkers in risk prediction (Folsom et al., 2006; Wang et al., 2006). The complexity of cardiovascular disease, including the nature of competing risks within the general population, may favor the use of CRP in risk prediction because inflammation may be an important risk factor for different subpopulations, such as non-HDL cholesterol measurement is for those with familial hypercholesterolemia. A 2003 a joint scientific statement from AHA and CDC recommended against routine use of CRP in risk assessment for primary prevention of CHD, but supported its use in persons with a 10-year CHD risk of 10 to 20 percent (or those at intermediate risk of developing CHD disease), although the benefits of this strategy were unclear (Pearson et al., 2003). Other meta-analyses, such as Buckley et al. (2009), found moderate evidence for adding CRP to prediction models for those at intermediate disease risk.
A further context of use may be primary prevention, such as the expansion of statin treatment based on observed drops in CRP and cardiovascular event rates. As noted in Chapter 3, use of an intervention meant for primary prevention has an extremely low tolerance for risk. However, within this minimal tolerance for risk reduction of a very common serious chronic disease, more risk may be tolerated than for an intervention intended to prevent a less common or less serious disease. In the JUPITER trial, the study participants would not have received statin therapy under current treatment guidelines. Some argue that the results of this study suggested a potential role for expansion of statin therapy to 6.5 million adults with normal LDL and high CRP (Michos and Blumenthal, 2009). However, others argue that expanding treatment may require results from
more than one study, and take into account the cost effectiveness of the expansion as well as unintended risks of treatment. For example, based on the JUPITER data, those treated had significantly higher glycated hemoglobin levels and incidence of diabetes (Hlatky, 2008; Ridker et al., 2008).3
In drug development, there is a continuum of uses from discovery on one end to surrogate endpoints on the other end. A determination of the general category of use for which the biomarker is intended is necessary to consider the biomarker’s utilization. For use in early drug development, lower levels of evidence are required. For example, qualification will most likely depend on a low level of biological plausibility—that interventions based on CRP levels make some mechanistic sense. At the other end of the spectrum is use as a surrogate endpoint. CRP is not currently utilized as a surrogate endpoint in drug or health claim approval. In spite of CRP’s utility in cardiovascular risk prediction, its normal function and role in cardiovascular disease remains uncertain. The lack of understanding of CRP’s biological role in human physiology has elicited controversy over assertions of CRP’s causal role in cardiovascular disease. More research is needed to clarify determinants of CRP variation and utility in diverse populations. Although several interventions are known to lower CRP, it is unclear whether the effects of different interventions on CRP are consistently correlated with clinical outcomes. Based on these findings, in the utilization step of the evaluation framework, CRP would not currently qualify for the context of use as a surrogate endpoint, but it may be used in risk prediction in certain populations.
CRP: Lessons Learned
The CRP case study illustrates the importance of evidence accumulation to support different biomarker uses. Current research indicates that CRP may have utility in risk prediction, especially for those at intermediate risk of cardiovascular disease. For use in primary prevention, aside from the JUPITER trial, there is limited information to assess the benefit of intervening with statin therapy in individuals with high CRP levels, but normal LDL cholesterol levels. Although there are indications that reductions in CRP may contribute to clinical benefits, it is unclear whether CRP participates causally in the disease pathway. Multiple interventions
are known to affect CRP levels, including statins, fibrates, exercise, and weight loss, but the benefits of these different interventions on clinical outcomes are still under evaluation. It is not clear whether these all confer valuable benefits on clinical outcomes, and that these benefits are all similar. Likewise, CRP variation in diverse populations and its predictive capacity in diverse racial and ethnic populations require further research. Incomplete understanding of CRP’s normal function or its role in the disease process prevents the use of CRP as a surrogate endpoint at this time. Therefore, while CRP may be a useful biomarker for risk prediction, more evidence needs to be accumulated to establish further uses of CRP, both within clinical practice and regulatory decision making.
TROPONIN
Acute myocardial infarction is diagnosed through use of biomarkers, and cardiac troponin (cTn) is the biomarker that is best able to fulfill this task. Troponin is a protein involved in the function of cardiac and skeletal muscle function. Cardiac and skeletal troponins are proteins with three subunits; cardiac troponin contains cardiac troponin C (cTnC), cardiac troponin I (cTnI), and cardiac troponin T (cTnT). Several of the cTn subunits found in cardiac tissue are easily differentiated from the skeletal forms of the protein. Of the three subunits, cTnC is isomorphic with its skeletal counterpart, and so it is not used in cTn assays. Both cTnI and cTnT are distinguished through cTn-specific amino acid sequences near the N-terminus in the case of cTnI and both the N- and C-termini of cTnT. cTn assays utilize these unique characteristics through use of sequence-specific antibodies (Babuin and Jaffe, 2005). Though cTnT and cTnI, both subunits of cardiac troponin, are quantitatively different, from the clinical perspective, they are equivalent with the exception of renal failure.
The use of cTn for the assessment of myocardial infarction in suspected acute coronary syndrome (ACS) patients is ubiquitous and guideline driven. cTn is released only from heart tissue, and therefore elevations of cTn indicate recent myocardial damage. Nevertheless, elevated levels of cTn do not imply a cause of myocardial injury nor are the levels automatically suggestive of an acute coronary event (Wu and Jaffe, 2008). In addition to patients with ACS and MI, there are clinically important groups for whom measurements of cTn can aid in diagnosis and management. Its use in chronic settings is newer, and relies on developing high-sensitivity assays. However, there are already examples of the use of this testing with contemporary assays to assess drug safety and toxicity; these examples have not only proven diagnostically important, but also have assisted in exploration of interventions to address some toxic drug effects (Cardinale et al., 2000, 2002, 2006; Sandri et al., 2003).
Troponin: Analytical Validation
Cardiac troponin is the preferred biomarker to diagnose myocardial infarction and is accepted as the standard biomarker for this use by the American College of Cardiology, European Society of Cardiology, World Heart Federation, and AHA (Thygesen et al., 2007). These entities have defined an elevated cTn as being above the 99th percentile of a healthy population (Morrow et al., 2007; Thygesen et al., 2007). This percentile range is currently determined for each assay (Apple et al., 2007; Morrow et al., 2007; Panteghini et al., 2001). These groups also mandate interpretation of a rising or falling pattern. Calculating this for most assays relies almost entirely on analytic variation (the accuracy and precision of the test used) because contemporary assays are not sensitive enough to detect smaller changes in troponin levels. While troponin levels are different in different patient populations based on a variety of biological factors such as age, posture, and more, biological variation is generally not considered when troponin levels are measured, due to inadequate sensitivity of the assays. In addition, the reference populations for these measurements are variable and determined from convenience samples composed of various ages, and include individuals without cardiovascular disease, but with high concentrations of cTn for other reasons (Apple, 2009). Such individuals often have an adverse prognosis over time, so it is difficult to distinguish between an age-related increase in values and a subtle comorbidity such as silent myocardial damage. The prognostic data argue for the latter (Daniels et al., 2008a; Zethelius et al., 2006).
Various assays, including improved assays with higher analytical sensitivity, are becoming available for the measurement of cTnI and cTnT. In general, cTnI or cTnT are captured by specific antibodies onto surfaces or particles, tagged with the same specific antibodies from free solution, and then tagged with fluorescently labeled antibodies or another detection molecule. The extent to which new assays can decrease non-specific binding, increase binding or detection efficiency, or increase the lifetime or stability of the reagents is a major determinant of improved quality of the assays. Different assays, however, are not standardized because each uses a different set of antibody configurations to detect cTn in blood (Apple et al., 2007). Thus, the different assays recognize different epitopes and thus may measure different fragments or modified forms of the biomarker. In addition, all assays are calibrated differently (Apple, 1999; Katrukha et al., 1998). Furthermore, false positives related to fibrin (Roberts et al., 1997), and heterophilic and crossreacting human antimouse antibodies (Kaplan and Levinson, 1999) are of particular concern (Jaffe et al., 2000), although such problems are relatively infrequent. A new generation of cTn assays that are more precise at low concentrations (Apple, 2009) are being developed. The comparability of these assays with older assays
is being discussed by the FDA with a focus on ensuring accuracy at the 99th percentile and minimizing false-negative and false-positive findings (Apple, 2009). Some have proposed an assay-to-assay scorecard comparison and evaluated various assays and determined their acceptance designation based on imprecision at the 99th percentile.
Although some research assays have a low degree of imprecision at the 99th percentile, some of them may not be able to manifest high precision at many of the lower, more normal, values. For example, consider Patient Z, a 50-year-old man with a history of hypertension, hyperlipidemia, and mild diabetes. He had a mildly positive stress test in the past, but is otherwise asymptomatic. He started on a new antidiabetic agent 5 years ago. At that time, his ultra-sensitive cTn level was 3 pg/ml. It was 4 pg/ml 4 years ago, 7 pg/ml last year, and is now 9 pg/ml. First, most contemporary assays cannot measure these low concentrations. For all of the ultra-high-sensitivity assays, with one exception, these values are within the 99th-percentile reference population. However, it is unclear whether the change in these values exceeds analytical imprecision and biological variability until the third value (7 pg/ml last year) for some assays. In addition, if Patient Z has developed a new comorbidity such as heart failure, such a change might be expected.
Latini and colleagues (2007) suggest that troponin values on the high end of the normal range, at least in association with disease, has adverse prognostic significance for heart failure patients (Latini et al., 2007). Although these preliminary data suggest these assays have enormous potential for detecting disease at a very early stage, much of the necessary data validating such an approach is not yet in the public domain. Moving forward with standardized assays required establishing common 99th-percentile values using a healthy reference population that could provide standardized material for all assays (Apple, 2009). Although assays to measure cTn have not yet shown complete analytical validation, this case study will presume that several of the assays have adequate sensitivity, specificity, precision, and reproducibility, and the biomarker will be advanced to qualification.
Troponin: Qualification
Although measurable levels of cTn are indicative of cardiac injury, they are not “synonymous with an ischemic mechanism of injury” (Jaffe et al., 2000). The Dallas Heart Study demonstrated that it is not normal or healthy for individuals to have detectable cTnT levels. cTnT elevation was associated with congestive heart failure, left ventricular hypertrophy, diabetes mellitus, and end-stage renal disease (Wallace et al., 2006), though the higher cTnT levels did not appear to mark acute events. Longitudinal
research demonstrates that cTnI concentrations increase with age, even in patients without CHD, indicating “silent myocardial damage” (Zethelius et al., 2006).
Clinical data from several different trials show higher risk of mortality in individuals with elevated cTn with contemporary assays. Thus, it is likely that higher sensitivity assays will assist researchers in describing this area more accurately. cTn levels provide a threshold for higher risk for patients who present with acute ischemia. For example, the Rancho Bernardo Study revealed that older individuals (70 years of age and older), both men and women, with elevated troponins (greater than 99th percentile using contemporary assays) had an higher risk of all-cause and cardiovascular death (Daniels et al., 2008b). Similarly, the Uppsala study revealed that elderly individuals (65 years of age and older) with elevated cTnI, as measured with a highly sensitive Beckman assay, had impaired cardiac performance and higher cardiovascular risk (Eggers et al., 2008). Indeed, even values below the 99th-percentile value for the whole group—but above the value that might have been used had only younger individuals been included—were at higher risk. The Prevention of Events with ACE inhibition (PEACE) trial has shown that there is a positive correlation between cTnT levels measured with a high-sensitivity assay not yet released in the United States and both cardiac death and heart failure (Omland et al., 2009). Many of these values were below the putative 99th-percentile value. Thus, the assays continue to evolve. This evolution leads to the conclusion that utilization analysis (step 1c of the biomarker evaluation framework) and as-needed and continuing reevaluation of analytical validation and qualification (steps 1a and 1b), upon which utilization decisions are based, are essential.
High levels of cTn (greater than 99th percentile of the reference range) are associated with other causes of cardiac injury (e.g., cardiac surgery, pulmonary embolism, congestive heart failure [Missov et al., 1997], ablation [Katritsis et al., 1998], and myocarditis [Lauer et al., 1997]), as well as with non-cardiac diseases (e.g., sepsis [Guest et al., 1995; Spies et al., 1998], preeclampsia [Fleming et al., 2000], end-stage renal disease [Needham et al., 2004], extensive burns [Chen et al., 2000], high-dose chemotherapy [Cardinale et al., 2006], and stroke [James et al., 2000]), even with contemporary assays. This list will increase as more sensitive and more precise assays evolve. Box 4-1 highlights conditions associated with elevated cTn in the absence of overt ischemic heart disease. For many but not all of these latter conditions, elevated levels of cTn can be linked to direct toxic effects. Thus, although cTn is a highly sensitive biomarker of cardiac injury, it is not specifically an MI biomarker.
Though it is apparent that elevated cTn is associated with a higher risk of mortality, there is limited evidence that decreasing troponin levels
BOX 4-1 Conditions Associated with High Cardiac Troponins Critically ill patients, especially those with diabetes, respiratory failure, gastrointestinal bleeding, and sepsis High-dose chemotherapy Primary pulmonary hypertension Pulmonary embolism Renal failure Subarachnoid hemorrhage Scorpion envenoming Drug toxicity (e.g., Adriamycin, 5-fluorouracil, Herceptin, snake venoms, carbon monoxide poisoning) Hypothyroidism Burns, especially if total surface burn area is >30% Infiltrative diseases (e.g., amyloidosis, hemochromatosis, sarcoidosis, scleroderma) Acute neurological diseases (e.g., cerebrovascular accident, subarachnoid bleeds) Vital exhaustion Sepsis and septic shock Stroke Ultra-endurance exercise Postoperative noncardiac surgery patients SOURCES: Jaffe (2001), adapted from Ammann et al. (2004) and Wu and Jaffe (2008). Reprinted with permission, Copyright 2001, Springer Science + Business Media. |
through interventions improves mortality risk. As these clinical trials indicate, patients with even small elevations in cTn can be identified as having higher risk (Hamm and Braunwald, 2000; Heidenreich et al., 2001). Results from the TACTICS-TIMI 18 trial indicate that these patients derive clinical benefit from an early invasive strategy of coronary angiography and revascularization (Cannon et al., 2001; Morrow et al., 2001). Thus, continued collection of data in this area will be useful to elucidate whether this principle will apply to other areas.
As indicated in Box 4-1, elevated cTn with contemporary assays is associated with high-dose chemotherapy and may be a predictor of chronic cardiotoxicity. The development of cardiotoxicity in a cancer patient is a strong indicator for discontinuing chemotherapy (Pai and Nahata, 2000). Therefore, preventing cardiotoxicity in cancer patients is important for both cardiac outcomes and therapeutic opportunities (Cardinale et al.,
2006). Cancer patients with elevated cTnI who were treated with enalapril, an ACE inhibitor that is thought to inhibit the development of oxygen free-radicals, were found to have an observable, significant reduction in the development of cardiotoxicity (Cardinale et al., 2006). These trials were not conducted using the high-sensitivity assays, however, and so it is not known whether smaller detectable cTnI elevations would have the same predictive capability.
This discussion indicates that cTn has prognostic value, but that there are limited data available for determining whether interventions targeting troponin impact outcomes in a broad set of diseases.
Troponin: Utilization
In 2007, the National Academy of Clinical Biochemistry (NACB) formed a committee to recommend guidelines for using cTn in etiologies other than ACS and heart failure. The committee determined that cTnT and cTnI could be used to risk-stratify patients with end-stage renal disease. cTnT is more frequently elevated for reasons that are unclear at present because, in general, cTnT and cTnI are otherwise generally equivalent clinically. Additionally, in patients with end-stage renal disease, changes in cTn elevation may be indicative of adverse prognoses, including coronary heart disease and death (Apple et al., 2002; DeFilippi et al., 2003). However, no therapeutic interventions are known to reduce cardiovascular risk based solely on the results of cTn testing in patients with end-stage renal disease (NACB Writing Group Members et al., 2007). The benefits and risks of such interventions are not fully defined (Scirica and Morrow, 2004). Thus, renal function is an important covariate in any cTn analysis. The NACB committee also determined that a high level of evidence suggests the measurement of cTn can define risk among patients who are critically ill, including those with sepsis (NACB Writing Group Members et al., 2007).
At present high-sensitivity cTn has been applied as a safety biomarker. Few data support other roles. Safety biomarkers are biomarkers used in preclinical (animal testing, often) and early clinical testing of drug and device candidates for a number of common types of toxicities. A recent IOM workshop explored the current status of development of safety biomarkers, especially in the organ systems of the heart, liver, and kidneys (IOM, 2009).
There are valuable uses of cTn as a biomarker, including as a risk biomarker in phase I studies to indicate safety problems with tested drugs and to collect further information about the biomarker. In the case above, it is not totally clear when the values rise above the critical threshold for risk. It may be that a change in troponin level within the normal reference
range is sufficient, or it may be that a value above the 99th percentile is required. Furthermore, if an increase is important, one needs to determine analytical and biological variation to confirm that the changes exceed normal physiology and analytic error. Therefore, this analyte is not quite ready for widespread clinical application in chronic disease settings.
Troponin: Lessons Learned
The most appropriate use identified for high-sensitivity troponin assays is as a safety biomarker in early clinical trials, such as phase I trials. Further information is necessary to further validate the preanalytic and analytic characteristics of these new high-sensitivity troponin assays. Information on biological variation (i.e, the reference change value that includes both analytical and biological variation) is needed. This includes not only the biology, but also the precision of the measurements. Finally, as in many of the case studies, context for the use of this biomarker is key. Due to evolving scientific understanding and test capabilities, it is not expected that the utility of these assays will be static.
LDL4 AND HDL AS BIOMARKERS FOR CARDIOVASCULAR RISK
Lipoprotein particles are complex structures composed of lipids (chiefly cholesterol, phospholipids, and triglycerides), and proteins (apolipoproteins and others). The understanding of lipoproteins’ structure, function, and role in CVD is shaped by more than 80 years of research (Cohn et al., 1946; Gofman et al., 1954; Macheboeuf, 1929; McNamara et
4 |
It is important to highlight an issue with the commonly used terminology for cholesterol. A key point to recognize is that all major estimators of coronary risk do not include LDL cholesterol but rather total cholesterol-to-HDL ratio. Also, LDL cholesterol is rarely measured, most often being estimated from other fractions. The problem arises from the conflation of risk factors with biomarkers for benefit of intervention (i.e., surrogate endpoints). As is described in Chapter 1, these are not the same. Inclusion of total cholesterol and HDL cholesterol in global CVD risk prediction models obviates the need to include LDL cholesterol. It is also true that change in LDL cholesterol is more appropriate as a biomarker for effects of intervention on CVD risk. While it has some limitations in this regard, it is not reasonable to use total cholesterol or total cholesterol/HDL ratios as biomarkers for intervention since cholesterol is too non-specific (in particular, it can be decreased by lowering HDL cholesterol, which may not be beneficial); and we have no evidence that reducing the total cholesterol to HDL ratio by increasing HDL is beneficial. One step toward dealing with this dilemma, although imperfect, is to move from LDL to non-HDL cholesterol (total cholesterol minus HDL) as both a risk marker and treatment target. This at least addresses the two limitations of LDL cholesterol noted above. Although the terminology is awkward and has not caught on clinically, non-HDL cholesterol has been adopted in a limited way by the National Cholesterol Education Program Adult Treatment Panel III (NCEP ATPIII), and is on the list of issues for further discussion in the Adult Treatment Panel IV (ATPIV). |
al., 2006; Olson, 1998; Pederson, 1947). By the mid-20th century, scientists had defined and measured all lipoprotein classes (Gofman et al., 1954), and preparative ultracentrifugation allowed for the separation of major lipoproteins (Havel et al., 1955). This cumulative research facilitated the development of clinical lab assays for LDLs and HDLs that could be applied in large epidemiologic studies and in clinical practice (Burstein and Samaille, 1958).
Since then, studies have established the cardiovascular risk associated with elevated concentrations of LDL cholesterol (LDL-C), abnormal proportions of LDL and HDL particles (Gofman and Lindgren, 1950; Gofman et al., 1950a, 1950b), and the reduction in cardiovascular risk associated with high HDL cholesterol (HDL-C) concentration (Robins, 2001; Scanu, 1966). That led to therapeutic interventions to induce reduction in cardiovascular disease risk by lowering LDL (Lipid Research Clinics Program, 1984a, 1984b), particularly through the use of 3-hydroxy-3-methylglutaryl coenzyme A (HMG CoA) reductase inhibitors (statins) (4S Study Group, 1994; Collins et al., 2002; Downs et al., 1998; LaRosa et al., 2005; Ridker et al., 2008a).
The FDA has used drug-induced LDL-C lowering as a surrogate endpoint for improved patient outcomes in approving new chemical entities; approval does not necessarily require confirmation of clinical benefits via outcomes studies. Because the FDA’s Center for Drug Evaluation and Research (CDER) considers LDL-C to be a qualified surrogate endpoint, if a drug is safe and effectively lowers LDL-C, the drug’s approval can be based on these lipid-lowering effects combined with the absence of a safety signal in the absence of definitive clinical outcome data (Nissen, 2008). Although a low level of HDL-C may signal a higher coronary heart disease risk than a moderately high LDL-C level (Robins, 2001), HDL-C has not yet qualified as a surrogate endpoint for cardiovascular risk because there is no evidence that HDL-raising interventions can improve outcomes. The FDA’s Center for Food Safety and Nutrition also considers LDL-C as a qualified surrogate endpoint, so authorized health claims for cardiovascular disease risk reduction can be approved for foods containing ingredients that have been shown to lower LDL-C, with few restrictions on what other ingredients the food may contain and in the absence of information about the effect of the food on the clinical outcomes of those who consume it.
LDL and HDL: Analytical Validation
Cardiovascular biomarkers need to have not only prognostic value, but they also need to be readily measurable via standardized high-quality assays that can be correctly interpreted (Zaninotto et al., 2007). The
National Cholesterol Education Program of the National Institutes of Health has put forth precision and accuracy standards for LDL-C testing (Bachorik and Ross, 1995). As a result, the laboratory measurement of LDL-C is a reliable and reproducible measure most commonly determined using the Friedewald formula, which estimates LDL-C from measurements of total cholesterol, triglycerides, and HDL-C. Because this calculation does not directly measure LDL-C, it has limitations; more recent homogeneous assay methods are capable of directly measuring LDL-C (Nauck et al., 2002). However, the levels obtained by direct measurement of LDL-C do not show improved performance over those calculated using the Friedewald formula (Miller et al., 2002; Schectman et al., 1996; Yu et al., 2000), and the lower values obtained by this measurement may result in misclassification of CVD risk using current risk categories (Mora et al., 2009b). The CDC’s Cholesterol Reference Method Laboratory Network certifies manufacturers of clinical diagnostic products that measure total cholesterol, HDL-C, and LDL-C and has certified five assays for measuring LDL-C.
Lipoprotein measurements other than LDL-C may also be considered in assessment of the degree of atherosclerosis and the endpoint of CVD-related morbidity and mortality. For some populations, there is increasing evidence that LDL particle number (LDL-P), small LDL particle concentration, non-HDL cholesterol, and apolipoprotein B (apoB) may have stronger associations with CVD risk than LDL-C (Contois et al., 2009; Cromwell et al., 2007; El Harchaoui et al., 2007; Jiang et al., 2004; Sniderman, 2002) because measurement of LDL-C does not consistently reflect levels of these other measures. The number of apoB-containing lipoprotein particles has been frequently reported to be more strongly associated with CVD risk than LDL-C (Barter et al., 2006; Sniderman and Marcovina, 2006). Thus, apoB may be a legitimate candidate for a biomarker for CVD risk (Miremadi et al., 2002). Also, there is less analytic variability in measuring apoB than in measuring LDL-C. Some data suggest that apoB, as well as LDL-P (another measure of LDL particle number), may have an important role in judging CVD risk in patients with elevated triglycerides and reduced HDL-C (El Harchaoui et al., 2007); validation and confirmation in other studies is needed.
Particles of LDL are heterogeneous, and smaller, denser LDL particles are more strongly related to risk than large LDL (Berneis and Krauss, 2002; Krauss, 1995; Krauss and Burke, 1982; Mora et al., 2009a; Musunuru et al., 2009; St-Pierre et al., 2005; Williams et al., 2003). However, most studies have suggested that peak LDL size measurement alone does not add information beyond that obtained by measuring LDL-C, triglyceride levels, and HDL-C (Campos et al., 1995; Mykkanen et al., 1999; Sacks and Campos, 2003).
Although HDL-C quantifies the amount of cholesterol contained within HDL particles, this measure does not necessarily correlate with the number of HDL particles or the differences between particle types. HDL particles can be classified according to their size and density as well as their protein composition; some evidence suggests these subclassifications may coincide with the functional properties of HDL (Joy and Hegele, 2008a, 2008b). Additionally, apolipoprotein A1 (apoA1) holds promise for measuring the correlation between HDL and CVD risk (Knopp et al., 2008). Until more is known about HDL particles and their functionality, standardization of HDL measurements other than HDL-C may not be feasible.
LDL and HDL: Qualification
LDL is associated with the disease process for atherosclerotic CVD because cholesterol is directly involved in the pathological disease process. The disease process, though, is influenced by numerous other mediators, including chemokines, cytokines, growth factors, proteases, adhesion molecules, hemostasis regulators, and receptors (Lopes-Virella and Virella, 2003; Virella and Lopes-Virella, 2008). These factors may also influence plaque progression and instability (Tardif et al., 2006). Additionally, genetic diseases, such as familial hypercholesterolemia, support the concept that CHD is influenced by high levels of LDL-C (Ballantyne, 2002).
The biological complexity of HDL structure and function complicates the ability to fully understand its beneficial mechanisms. The complex macromolecule possesses, to name a few, antioxidant, anti-inflammatory, antithrombotic, and endovascular properties (Griffin et al., 1999; Nofer et al., 2002; Steinberg et al., 1989), thereby challenging the isolation of specific HDL effects. Reverse cholesterol transport (RCT) is considered to be the primary mechanism by which HDL protects against atherogenesis (Rader, 2006). A current working model of RCT involves eight critical steps and several organs (e.g., liver, intestine, kidneys), enzymes (e.g., cholesteryl ester transfer protein [CETP], hepatic lipase), and lipoproteins and lipids. Any of these molecules could potentially be targeted when aiming to increase HDL-C levels. However, the optimum target for intervention is unknown (Joy and Hegele, 2008a). Furthermore, Tangier disease, in which individuals have a relatively low CVD mortality regardless of markedly reduced levels of HDL, complicates our understanding of the links between HDL and CVD (Oram, 2001).
Several observational studies have addressed the associations among age, cholesterol levels, and mortality in an older population. The Framingham Study, for example, showed that in the average population over
age 70, no statistical relationship is apparent among age, cholesterol, and mortality (Kronmal et al., 1993; Schatz et al., 2001) and that cholesterol may, in fact, be associated with longevity in individuals 85 years and older (Weverling-Rijnsburger et al., 1997). Other studies, though, have shown a reduction in the coronary heart disease death and nonfatal MI among individuals aged 70–82 years treated with pravastatin compared to placebo (Shepherd et al., 2002).
Epidemiologic studies have consistently demonstrated that higher CVD risk is also observed with lower levels of HDL-C (Knopp et al., 2008; Robins, 2001). Observational evidence indicates that raising HDL may independently reduce CVD risk (Gordon and Rifkind, 1989). However, while HDL-C is correlated with CVD risk in large studies, HDL-C levels are not necessarily indicative for individual patients due to the lack of understanding of the molecule’s cardioprotective properties (Briel et al., 2009; Joy and Hegele, 2008a).
Epidemiologic studies describe increasing CVD risk with increasing LDL-C even when other risk factors are present (Knopp et al., 2008). Circumstantial evidence shows that greater decreases in LDL-C translate into greater positive effects than smaller decreases (Cannon et al., 2004), further indicating the strength of association between LDL-C and CVD risk. This association is not, however, equal across all ages and genders (Aronow, 2006; Berra, 2000; Knopp et al., 2005).
CVD is a complex disease with multiple determinants; LDL and HDL particles are only two of the involved components. Nevertheless, a large body of evidence supports LDL-C, in particular, as a biomarker that is extremely good for predicting CVD risk. Several decades of research have established a significant correlation between LDL-C and CHD (Castelli et al., 1986; Goldstein et al., 1973; Watanabe et al., 1985). There are examples where lower LDL-C clearly and consistently indicates lower risk for CVD, such as in familial hypercholesterolemia (van Aalst-Cohen et al., 2004). Numerous clinical trials have shown that reducing LDL-C decreases the incidence of CHD-related clinical events (4S Study Group, 1994; Cannon et al., 2004; Lipid Research Clinics Program, 1984c). These trials indicate that interventions can reduce CVD risk and provide credence that LDLC accurately captures clinical impacts (Tardif et al., 2006). Statin trials in particular support the use of LDL-C as a biomarker for CVD risk. The West of Scotland Coronary Prevention Study (WOSCOPS) is one of the seminal studies establishing that the administration of a statin (pravastin) reduced cardiovascular mortality and morbidity (Shepherd et al., 1995). Other clinical studies have confirmed the value of secondary prevention using statins in patients who have documented CHD or who have suffered an acute cardiovascular event (4S Study Group, 1994; Collins et al., 2002; Schwartz et al., 2001). Thus, FDA-approved statins can make
labeling claims for the reduction of cardiovascular risk when they have specifically proven, through outcome studies, to reduce cardiovascular risk in addition to reducing LDL-C.
However, there is a good deal of controversy within research and patient care communities regarding limitations on LDL-C’s utility as a biomarker in CVD. Targeting only LDL-C to reduce CVD risk may, in some populations and for some patients, miss other, potentially more relevant attributes of the disease pathway (e.g., fibrates). Much of the research examining the relationship between LDL-C and CVD risk demonstrates correlation between LDL-C levels and patient outcomes (4S Study Group, 1994; Cannon et al., 2004; Lipid Research Clinics Program, 1984c). But because CVD is a multifactorial disease process, and because many of the drugs used to treat it have pleiotropic effects (Liao and Laufs, 2005), one biomarker is unlikely to ever be a perfect surrogate endpoint for use in CVD clinical trials. Studies exist in which LDL-C was robustly decreased yet cardiac events were not reduced: specifically, estrogen replacement does result in lower LDL-C levels (Herrington et al., 2000; Hulley et al., 1998). Nonetheless, Hulley et al. concluded in 1998 that estrogen replacement therapy did not confer a cardiovascular benefit in a randomized clinical trial. Herrington et al. also concluded in 2000 that estrogen or estrogen plus medroxyprogesterone acetate did not provide any cardiovascular benefit in women with coronary atherosclerosis.
Intervention studies involving HDL-C have not been consistent, and there is little consistent evidence that raising HDL confers predicted benefit. However, there is evidence that raising HDL independently in apoA1 transgenic mice reduces atherosclerosis (Plump et al., 1992), and there has been intense interest in the development and application of pharmaceutical agents designed to raise HDL-C (Canner et al., 1986; The Coronary Drug Project Research Group, 1975; Frick et al., 1987; Rubins et al., 1999).
In the latter half of the 1980s, scientists described individuals and families that lacked CETP, resulting in raised HDL levels (Inazu, 1990; Tall, 1993). In about 1990, the hypothesis that raising HDL had an antiatherogenic effect began to be discussed in the scientific literature. In 1992, experiments demonstrated that rodents lacking CETP were found to have elevated HDL and were resistant to atherosclerosis (Jiang et al., 1992; Tall, 1993). Soon after these developments, Pfizer began development of a cholesteryl ester transfer protein inhibitor, torcetrapib. The Investigation of Lipid Management to Understand its Impact in Atherosclerotic Events (ILLUMINATE) trial investigated whether torcetrapib would decrease cardiovascular disease risk. The trial was prematurely terminated because of a higher risk of death and cardiac events, despite evidence of increasing HDL-C and decreasing LDL-C and imaging studies of carotid and
coronary vessels showed no benefit. While a known off-target effect of torcetrapib is an increase in blood pressure, the study was unable to rule out adverse or non-beneficial effects related to CETP inhibition (Barter et al., 2007), raising questions about whether raising HDL is an effective strategy for preventing CVD. A recent meta-analysis concluded that increasing HDL levels alone does not reduce risk of coronary heart disease events or deaths (Briel et al., 2009).
This notable example also illustrates that lowering LDL-C does not always correlate with improved patient outcomes; that the manipulation of lipid-processing pathways to lower LDL-C may result in a multiplicity of effects; and that when new drugs are used in human subjects, they may display unanticipated mechanisms of action that result in off-target effects (Barter et al., 2007).
Despite the disappointment of torcetrapib, several pharmaceutical interventions associated with increasing HDL-C appear to reduce atherosclerosis, principally through the administration of niacin (Canner et al., 1986; The Coronary Drug Project Research Group, 1975; Nofer et al., 2002), fibrates (Frick et al., 1987; Rubins et al., 1999), and reconstituted HDL (Nissen et al., 2003; Tardif et al., 2007). The 2001 Veterans Affairs High-Density Lipoprotein Intervention Trial (VA-HIT), treatment with gemfibrozil (fibric acid), decreased CVD without a change in LDL-C, but with an increase in HDL-C and a decrease in triglycerides (Robins, 2001). Thus, there is need for ongoing research in HDL-C elevation for the prevention of CVD risk.
As previously indicated, the statin trials consistently demonstrate that lowering LDL-C can reduce CVD risk, while other LDL-lowering interventions have not always resulted in lower CVD risk. Nevertheless, exceptions to this pattern indicate that LDL-C is not the only contributor to the CVD pathway; there is no consistent relationship between the decrease in LDL and the magnitude of risk reduction with statins (Hayward et al., 2006). The VA-HIT trial, for instance, decreased CVD risk without lowering LDL-C (Robins, 2001) and, as pointed out above, the ILLUMINATE trial decreased LDL-C levels, but increased CVD risk (Barter et al., 2007). In the ENHANCE trial (Effect of Combination Ezetimibe and High-Dose Simvastatin versus Simvastatin Alone on the Atherosclerotic Process in Patients with Heterozygous Familial Hypercholesterolemia trial), the additional reductions in LDL brought about through use of ezetimibe did not result in fewer cardiovascular events in the study population (Krumholz and Lee, 2008). The relationship between LDL-C and CVD risk is not perfect in all circumstances and for all populations.
There are also areas of inconsistency with regard to HDL-C and CVD risk. Low HDL is a component of atherogenic dyslipidemia, which is characterized by higher triglycerides, remnant lipoproteins, and small LDL
particle size; the magnitude of the contribution of HDL to CVD risk independent of this dyslipidemia has not been firmly established (Musunuru et al., 2009; Walter, 2009).
LDL and HDL: Utilization
An often-cited article published nearly three decades ago listed 243 coronary risk factors (Hopkins and Williams, 1981). Since then, the complexity of this list has increased (Hoefner, 2008). Consequently, a single biomarker (e.g., LDL-C or HDL-C) may be insufficient to accurately predict CVD risk for all patients. For example, as previously discussed, age and triglyceride level may complicate the non-linear dynamic between LDL-C and CVD risk.
There is a continuum of risk for CVD for which there are markers other than LDL-C and HDL-C. For example, combined hyperlipidemia, in which patients have elevated LDL, hypertriglyceridemia, and low HDL, may have a higher risk for CVD, for instance, than patients with normal LDL-C, but high CRP. For these latter patients, measurement of LDL-C alone will not provide full insight into their potential disease pathways. The benefits of statins may be in part related to pleiotropic effects (e.g., anti-inflammatory effects, decrease in triglycerides) and not solely due to lowering LDL-C (Nissen, 2008; Ridker, 2007). Furthermore, because lipo-protein transport consists of a system involving VLDL, LDL, and HDL, any intervention may affect all these lipoproteins (Knopp et al., 2008). The multiple effects of any treatment must be considered, as must the patient’s individual risk profile.
If raising HDL is beneficial, the benefit may depend on the method used: weight loss, exercise, niacin, and fibrates appear to be beneficial but not specific to raising HDL; estrogen is not beneficial (despite lowering LDL); and raising HDL by CETP inhibition (i.e., torceptrapib) is inconclusive because of off-target effects and the inability to assess impact on CVD. Although there is modest evidence for the benefit on atherosclerosis of infusing native ApoA1 or modified ApoA1, it has proven difficult to devise a therapeutic intervention that specifically raises HDL without decreasing triglycerides and confounding the metabolic profile.
There is variable utility in using lipid profiles for prognostication at different points in the CVD spectrum and in different patient populations. In normotensive patients lipid profiles have been shown to be linked to arterial pressure and vessel stiffness (Marques-Vidal et al., 1996). Some studies have shown that in patients with essential hypertension, LDL-C and HDL-C independently predict risk of cardiovascular events (Verdecchia et al., 2004). In patients with atherosclerosis, some evidence
supports the measurement and modification of triglyceride levels to reduce risk for CVD events (Durrington, 1998; Manninen et al., 1992).
In patients with established CHD, things are a little more complicated. In one study low serum cholesterol was associated with an improved outcome in patients with CHD, but with a worse outcome in patients without CHD (Califf et al., 1992; Sakatini et al., 2005). In other studies lipid levels in patients with severe CHD were not predictive of survival (Feenstra et al., 1998), but initiation of statin therapy was associated with improved outcome (Horne et al., 2000). A study of lipid profiles in patients with angina pectoris found that reduced HDL-C was more strongly associated with higher coronary risk than other lipid measurements (Bolibar et al., 2000).
In the case where myocardial infarction has occurred, results vary somewhat in terms of the predictive value of lipid profiles. Some of the evidence points to worse outcomes with elevated lipid profiles in the first 24 hours post-MI, and advocate for statin use as early as possible to improve outcomes (Gorecki et al., 2004). HDL-C was highlighted as more independently predictive than LDL-C or total cholesterol in one study that examined the prognostic value of these variables in predicting recurrent events during acute coronary syndrome (Correia et al., 2009). In a study examining the role of biomarkers in premature MI (meaning in young patients), lipid profiles were shown to have no prognostic value for outcomes, and greater emphasis was placed on other variables such as smoking, ejection fraction, and serum homocysteine levels (Pineda et al., 2009).
LDL and HDL: Lessons Learned
As one of the FDA-qualified surrogate endpoints for CVD, LDL concentration is often viewed as the benchmark biomarker.
The evidence supporting LDL as a biomarker rests almost entirely on the measurement of LDL cholesterol even though this substance is only one part of the lipid transport system. Both apolipoprotein B and the quantity and the composition of LDL particles themselves have potential to be more accurate measures of LDL for some populations (Berneis and Krauss, 2002; Tardif et al., 2006), showing that even for qualified biomarkers, developing standard measures is an ongoing process.
The strength of LDL as a surrogate endpoint is not absolute due to the heterogeneity of cardiovascular disease processes, the heterogeneity of LDL-lowering drug effects, and the heterogeneity of LDL particles themselves. Because cardiovascular disease is a multifactorial chronic disease, a single risk factor for the disease (e.g., LDL-C) cannot fully account for all the variability that leads to a particular outcome (Libby
and Theroux, 2005; Tardif et al., 2006). This report’s case study of CRP suggests that inflammation, for example, may also affect the cardiovascular disease pathway. Furthermore, age, gender, and genetic factors have been shown to complicate these already complex disease dynamics; as a result, lowering LDL-C can never be considered a “perfect” indicator across all population groups. That said, there is high probability that lowering LDL for several interventions decreases risk of cardiovascular disease (4S Study Group, 1994; Cannon et al., 2004; Knopp et al., 2008; Lipid Research Clinics Program, 1984c), and LDL, although not perfect, is one of the best biomarkers for cardiovascular disease.
Interventions to address a multifactorial disease introduce potentially unforeseen effects, particularly when the causal disease pathways, the mechanisms of action of the intervention, and the characteristics of the biomarker itself are not fully understood. High-density lipoprotein does not qualify as a surrogate endpoint for cardiovascular disease risk because these characteristics, particularly the latter, introduce high levels of variability.
BETA-CAROTENE
Beta-carotene (β-carotene), a pigment-producing molecule in the skin of several fruits and vegetables, is a member of the plant carotenoid family. β-carotene from dietary and supplemental sources is partially converted to vitamin A. Two µg of supplemental all-trans-β-carotene or 12 µg of dietary all-trans-β-carotene have a bioequivalency to 1 µg of all-trans-retinol or 1 retinol activity equivalent unit (IOM, 2000a). Due to this pro-vitamin-A activity, β-carotene in large enough amounts can prevent vitamin A deficiency. Vitamin A is an essential nutrient with important roles in normal vision, gene expression, reproduction, embryonic development, growth, and immune function (IOM, 2000a).
Although many publications use the terms vitamin A and β-carotene interchangeably, only β-carotene—but not preformed vitamin A—has been associated with potential antioxidant activity (IOM, 2000b). However, an IOM committee charged with evaluating the purported antioxidant nutrients concluded that although β-carotene and other carotenoids display in vitro antioxidant activity, the evidence that they act as in vivo antioxidants in humans is controversial (IOM, 2000b). Due to possible differences in antioxidant properties of the various carotenoids, it is important to specify which carotenoid(s) is being discussed.
Beyond the body of research done to evaluate a role for β-carotene in cardiovascular health and reduction of cancer risk, β-carotene has also been studied in other disease contexts. A supplement containing a combination of zinc, vitamin C, vitamin E, and β-carotene had a small
beneficial effect in the secondary prevention of some patients with diagnosed age-related macular degeneration (a leading cause of blindness in elderly people) (Chong et al., 2007). However, a specific effect attributable to β-carotene apart from the other nutrients in this multinutrient supplement cannot be made. Moreover, it is not possible to determine if the mechanism of action for this multinutrient supplement was due to its antioxidant properties or to other biological effects. Although β-carotene has also been studied as a possible treatment for cataracts, osteoarthritis, Alzheimer’s disease, and cystic fibrosis, the results are inconclusive (Gritz et al., 2006; Heliovaara et al., 1994; Kneckt et al., 1992; Wood et al., 2001).
To date, the IOM has not defined a specific Dietary Reference Intake (DRI) value for either an adequate or a safe intake of β-carotene for the general population of apparently healthy people (IOM, 2000a, 2000b). It did specify biological activity equivalents for the conversion of different β-carotene sources to vitamin A (as noted above). They concluded that the only adverse event associated with high or long-term doses of β-carotene from food sources is yellowing of the skin (IOM, 2000b). Therefore, researchers were surprised when supplemental sources of β-carotene used in several clinical trials were associated with higher risk of lung cancer in participants who were smokers or former smokers (IOM, 2000b). The latter trial results were particularly surprising given that numerous observational studies had shown that people who frequently consumed β-carotene-containing fruits and vegetables had a lower incidence of cancer and CVD.
The history of the use of β-carotene as an intervention for prevention of disease and as a biomarker of protection against disease is discussed in detail in the qualification step of the evaluation framework, below. This extensive experience with β-carotene provides for application of the proposed framework to a well-studied biomarker for several conditions, specifically cancer, CVD, and eye diseases. The steps in this process are presented in the next three sections and will be illustrated with the rationale and findings from these studies.
Beta-Carotene: Analytical Validation
The initial observations of the inverse association between the consumption of fruits and vegetables and several conditions were based on measures of dietary intake. The measurement methods included food frequency questionnaires and 24-hour recalls. The association remained robust despite the differences in measurement approach. The intake of carotenoids, including β-carotene, was also related to the presence of disease. The concentration of carotenoids was measured in blood, as it is easily accessible, and found to convey accurate information about the
consumption of fruits and vegetables. Extensive experience with dietary and blood assessments led to the conclusion that “blood concentrations of carotenoids are the best biologic markers for consumption of fruits and vegetables” (IOM, 2000b).
At the time that the hypotheses about β-carotene and chronic disease risk were most popular and were the basis for funding decisions on intervention trials, little or no attention was paid to the question of the reproducibility, accuracy, and precision of the measurement of serum β-carotene. Moreover, because it was assumed that β-carotene’s effectiveness was likely due to its in vivo antioxidant properties, a major limitation was the absence of a reliable prognostic assay for in vivo assessment of antioxidant activity that has clinical predictive value. There is no experimental technique that isolates β-carotene and measures its in vivo antioxidant capabilities when bombarded by several oxidative products. It is commonly regarded to reflect time-dependent β-carotene intakes, and by some researchers, to indicate body stores of β-carotene.
Concurrent with the development of associations between β-carotene intake (and blood levels) and cancer, cardiovascular disease, and retinal degeneration, research was directed to addressing the putative biologic mechanism of antioxidant activity. These measures endeavored to assess the potential to counteract oxidative damage occurring within nucleic acid or macromolecules resulting from the presence of free oxygen radicals in cells. For instance, oxidized LDL was found to produce atherosclerotic lesions in animals, and β-carotene could modulate this effect (Steinberg, 1997). Despite these links to oxidative potential and its modification, assays that measure the ability of an intervention to alter oxidative potential in humans proved inconsistent and unreliable. Because the collective experience indicated that these measures of oxidative potential could not be used in population studies, β-carotene measurement became the accepted standard for assessing a component of antioxidant exposure. However, β-carotene function is not limited to its antioxidant properties. Equally noteworthy, other antioxidants from various dietary sources exist and modulate cell activity. These considerations suggest that the antioxidant hypothesis regarding disease risk is not tested necessarily by β-carotene studies. In effect, the associations between β-carotene intake, blood levels, and disease are not supported by measures of the putative biologic process.
Beta-Carotene: Qualification
Several large intervention trials were undertaken based on the inverse relationships described in large observational and in vitro studies of cancer, CVD, and eye diseases (IOM, 2000b). The in vitro and animal studies,
regardless of the quantitative method used, consistently provided a plausible biologic basis to explain the prevention of the atherosclerotic process by supporting the idea of antioxidants limiting free radicals pervasive in CVD as well as cancer and retinal damage. For example, observational evidence including population studies found inverse relationships between dietary β-carotene and coronary heart disease (Liu et al., 2001). These findings were concordant with in vitro studies of β-carotene as an antioxidant. Operating under one or more notions of β-carotene as a risk biomarker, a surrogate endpoint, and a beneficial intervention, several large-scale randomized clinical trials were funded and designed to evaluate whether increasing β-carotene intake might lower the risk of cancer, CVD, and eye disease.
Three primary prevention clinical trials (mentioned below), which used β-carotene and/or other agents to augment baseline β-carotene serum levels, refuted the commonly accepted view about β-carotene. Two of the studies, initiated in populations at high risk of lung cancer by virtue of cigarette smoking or asbestos exposure, found increasing serum β-carotene increased the risk of lung cancer morbidity and mortality, whereas the other did not assert an advantageous or adverse effect regarding use of β-carotene in the prevention of cancer and CVD. Secondary CVD prevention trials also did not exhibit results of a beneficial or adverse impact in using the carotenoid. However, studies monitoring the impact of β-carotene on preventing eye diseases have produced conflicting findings.
The Alpha Tocopherol and Beta Carotene Prevention (ATBC) Study, begun in 1985, examined the incidence of lung cancer in 29,133 Finnish smokers. Researchers administered 20 mg of β-carotene, 50 mg of alphatocopherol, and a combination of the two supplements to three respective cohorts. They followed each subject for 5 to 8 years, reported an “excessive cumulative incidence of lung cancer was observed after 18 months (for the 7,278 men taking only β-carotene and 7,287 men who took a combination of β-carotene and alpha-tocopherol) and progressively thereafter, resulting in an 18% difference in incidence by the end of the study (95% CI = 3 to 36 percent, P = 0.01) between the participants who received β-carotene and those who did not” (Heinonen and Albanes, 1994). The number of new lung cancer cases was 876 (Heinonen and Albanes, 1994). Death increased by 8 percent for those in the β-carotene treatment group compared to the control (Heinonen and Albanes, 1994).
Further support of β-carotene’s role in promoting increased risk came from another National Cancer Institute–supported study, the Beta Carotene and Retinol Efficacy Trial (CARET). Organized in 1983 and randomized in 1985, this multicenter, double-blind, randomized controlled trial assessed whether 30 mg of β-carotene and 25,000 IU of retinol decreased
the likelihood of developing and dying from primary lung cancer or cardiovascular disease in 18,314 smokers, former smokers, and workers exposed to asbestos. The incidence rates for lung cancer mortality and CVD mortality were significantly higher in the active treatment cohort compared to the control. The relative risk for developing lung cancer in the active treatment group was 1.28 (95% CI = 1.04 to 1.57, P = 0.02) compared to the placebo-controlled subjects. The study confirmed 286 primary lung cancers. The relative risk for lung cancer–associated death in the active treatment group was 1.46 (95% CI = 1.07 to 2.00), and the relative risk for all-cause death was 1.17 (95% CI = 1.03 to 1.33). Of the 388 reported cases of lung cancer, 254 people died. With respect to CVD mortality, the relative risk was 1.26 (95% CI = 0.99 to 1.61). CARET was terminated prematurely based on its disconcerting results and the prior ATBC findings obtained during the study. Follow-up continued for an additional 5 years (Omenn et al., 1996).
The Physicians Health Study I, consisting of a randomized two-by-two factorial trial of β-carotene to prevent cancer and aspirin to prevent coronary heart disease, enlisted 22,071 male physicians, some of whom were smokers. Participants received either 50 mg of β-carotene, 325 mg of aspirin, or placebo on alternate days (Hennekens et al., 1996).The aspirin arm of the study was concluded first with evidence for prevention of coronary heart disease, and the β-carotene arm was continued for a total of 12 years. Researchers ascertained that there was neither benefit nor harm from β-carotene supplementation with respect to morbidity or mortality. The relative risk of lung cancer in men who took β-carotene compared to healthy men who consumed the placebo was 0.98 (95% CI = 0.91–1.06). Nevertheless, 1,273 men in the β-carotene group developed lung neoplasms. β-carotene did not provide significant benefit or harm on the number of myocardial infarctions (468 in the β-carotene group vs. 489 in the placebo group), strokes (367 vs. 382), deaths due to cardiovascular causes (338 vs. 313), all-important cardiovascular events (967 vs. 972), or deaths from all causes (979 vs. 968) (Hennekens et al., 1996).
Recently, two studies of female health professionals have reported similar findings. The Women’s Antioxidant Cardiovascular Study employed a factorial design to test 50 mg of β-carotene administered every alternate day, 500 mg of vitamin C daily, and 600 IU of vitamin E daily in the secondary prevention of CVD. The trial found no benefit or harm with β-carotene on a primary combined cardiovascular endpoints (RR = 1.02, CI = 0.92–1.13) or individual CVD endpoints (Cook et al., 2007). There were also no beneficial or harmful effects for vitamin C or vitamin A. The Women’s Health Study, a primary prevention study of 39,876 women 45 years and older, found no benefit or harm for either cancer or CVD with β-carotene supplementation (Lee et al., 1999).
Several of these findings complemented those summarized in the IOM report in 2000 indicating that β-carotene did not modulate the risk for CVD (IOM, 2000b). While β-carotene was highly promoted prior to these studies, the lack of efficacy led researchers, physicians, and policy makers to recognize β-carotene is not an effective intervention for CVD or cancer.
During this period, the inverse relationship between fruit and vegetable intake and eye conditions (development of cataract and retinal disease) attracted the interest of vision researchers. Survey data from the first National Health and Nutrition Examination Survey was consistent with an inverse association between antioxidants and retinal disease (Goldberg et al., 1988). Intake of vitamin A was negatively correlated with macular degeneration (Goldberg et al., 1988). Experimental studies had found that visible and ultraviolet light can damage the retina through production of superoxide radicals, and it was proposed that antioxidants might protect vision by modulating the effect of superoxide radicals (Feeney and Berman, 1976; Goldberg et al., 1988). The Physicians Health Study I provided an opportunity to test the potential effect of an antioxidant, β-carotene. This study of 22,071 U.S. physicians found, after 12 years of follow-up of this cohort for eye conditions, that there was no benefit or harm with β-carotene supplementation (relative risk for β-carotene = 0.96, 0.78–1.20) (Christen et al., 2007).
In 1990, the National Eye Institute initiated a placebo-controlled randomized trial of antioxidant vitamins (including β-carotene) or zinc or a combination of zinc and antioxidant vitamins to determine the effects on eye cataracts and acute macular degeneration (AMD) when compared to placebo (AREDS Research Group, 2001). This study, the Age-Related Eye Disease Study (AREDS), was reported in 2001 and found that the combination of antioxidants and zinc reduced the risk of AMD in those suffering from early stages of the disease (AREDS Research Group, 2001); the odds ratio was 0.72 (0.52–0.98). There was no effect on cataract formation and no significant adverse effects overall were reported. It was advised that the use of this treatment was contraindicated in smokers because of the prior studies of β-carotene and lung cancer in smokers. The combination of the three antioxidants and zinc has become the standard of care to slow progression of AMD. However, a follow-up trial (AREDS 2) is under way to test whether an antioxidant combination that does not contain β-carotene is effective.
To date, increasing β-carotene serum levels has no demonstrated value in predicting the development of cancer or CVD. Neither the subjects in control cohorts of the large clinical trials who had baseline levels of β-carotene nor those who had elevated β-carotene were necessarily protected from a neoplasm or a cardiac event. For example, 443 of the 14,573
men not given β-carotene in the ATBC study developed cancer (Albanes et al., 1996). In the same trial, nearly equal numbers of men given β-carotene and those not given the supplement died from a cardiovascular disease that was not ischemic heart disease, hemorrhagic stroke, or ischemic stroke (Albanes et al., 1996). To a certain extent, however, some argue that the clinical trials supported the observational studies. Upon examination of the cumulative incidence of lung cancer after 0 to 18 months of randomization in the CARET trial, the observed incidence between active treatment and placebo groups was nearly identical (Omenn et al., 1996). The results of the Physicians’ Health Study (PHS) trial conferred similar information. Of interest, the PHS was able to confirm a positive benefit for aspirin 325 mg every other day on prevention of CVD for a cohort of the same size as the Feeney and Berman (1976) β-carotene cohort. Given the size of the study population and the statistical power, it is apparent that β-carotene does not have prognostic value.
Prior to the major randomized clinical trials, the available evidence was limited to animal and observational studies. Nearly all of these studies were consistent in showing an association between serum β-carotene levels and chronic disease risk. Different methodologies and repetition of studies with β-carotene found similar results for the chronic diseases.
Beta-Carotene: Utilization
Prematurely, β-carotene was considered a biomarker that researchers and physicians could utilize for prognostic and predictive means. Many in the scientific and lay communities viewed the inverse association between β-carotene and cancer and CVD as indicative of the likelihood of developing either chronic disease. Additionally, some considered β-carotene useful for prevention given that it appeared as though it could reduce the onset, development, and death from these diseases. It was evident in ATBC and CARET that β-carotene in sufficient quantity could also cause adverse effects under certain conditions. Unfortunately, results from the large clinical trials lagged behind public endorsement of β-carotene as a beneficial intervention to prevent cancer and CVD. Hence, the context and application were incorrectly and prematurely assigned.
Nevertheless, β-carotene has been successfully used as a biomarker of intake of fruits and vegetables and an effective intervention to address vitamin A deficiency. Data supporting uses beyond these are still lacking.
Beta-Carotene: Lessons Learned
Regulatory agencies and the scientific community can draw several lessons from the application of β-carotene in the committee’s framework.
First, appropriate study of the biomarker for qualification is essential. Conclusions about the benefits of β-carotene were made before phase III trials were concluded. Emphasis on thorough scientific research at the preclinical stage could be a cost-effective approach to preventing mismanagement of time, energy, and finances. The qualification component of the biomarker evaluation framework emphasizes that the scientific basis for a biomarker is robust, accurate, and consistent, too. Consideration of multiple pathways by which β-carotene (and other biomarkers) exerts influence on a disease state should be examined as part of the qualification step of the framework. Chronic diseases usually involve more than one pathway to trigger the condition, which suggests that researchers should investigate how a biomarker might be implicated in several pathways to better elucidate its role in chronic disease pathology. Also, designating the appropriate study population is crucial to correctly determining the context-specific effects of a biomarker.
In sum, β-carotene is a good biomarker of fruit and vegetable consumption, but not a good surrogate endpoint for interventions aimed at preventing chronic diseases.
REFERENCES
4S Study Group. 1994. Randomised trial of cholesterol lowering in 4444 patients with coronary heart disease: The Scandinavian Simvastatin Survival Study (4S). Lancet 344(8934):1383–1389.
Albanes, D., O. P. Heinonen, P. R. Taylor, J. Virtamo, B. K. Edwards, M. Rautalahti, A. M. Hartman, J. Palmgren, L. S. Freedman, J. Haapakoski, M. J. Barrett, P. Pietinen, N. Malila, E. Tala, K. Liippo, E. R. Salomaa, J. A. Tangrea, L. Teppo, F. B. Askin, E. Taskinen, Y. Erozan, P. Greenwald, and J. K. Huttunen. 1996. Alpha-tocopherol and beta-carotene supplements and lung cancer incidence in the alpha-tocopherol, beta-carotene cancer prevention study: Effects of base-line characteristics and study compliance. Journal of the National Cancer Institute 88(21):1560–1570.
Albert, M. A., E. Danielson, N. Rifai, and P. M. Ridker. 2001. Effect of statin therapy on C-reactive protein levels: The Pravastatin Inflammation/CRP Evaluation (PRINCE), a randomized controlled trial and cohort study. Journal of the American Medical Association 286(1):64–70.
Albert, M. A., R. J. Glynn, J. E. Buring, and P. M. Ridker. 2004. C-reactive protein levels among women of various ethnic groups living in the United States (from the Women’s Health Study). American Journal of Cardiology 93(10):1238–1242.
Ammann, P., M. Pfisterer, T. Fehr, and H. Rickli. 2004. Raised cardiac troponins: Causes extend beyond acute coronary syndromes. British Medical Journal 328(7447):1028–1029.
Apple, F. S. 1999. Clinical and analytical standardization issues confronting cardiac troponin I. Clinical Chemistry 45(1):18–20.
Apple, F. S. 2009. A new season for cardiac troponin assays: It’s time to keep a scorecard. Clinical Chemistry 55(7):1303–1306.
Apple, F. S., M. M. Murakami, L. A. Pearce, and C. A. Herzog. 2002. Predictive value of cardiac troponin I and T for subsequent death in end-stage renal disease. Circulation 106(23):2941–2945.
Apple, F. S., A. H. B. Wu, A. S. Jaffe, M. Panteghini, R. H. Christenson, NACB Committee Members, C. P. Cannon, G. Francis, R. L. Jesse, D. A. Morrow, L. K. Newby, A. B. Storrow, W. H. W. Tang, IFCC Committee on Standardization of Markers of Cardiac Damage Members, F. Pagani, J. Tate, J. Ordonez-Llanos, and J. Mair. 2007. National Academy of Clinical Biochemistry and IFCC Committee for Standardization of Markers of Cardiac Damage Laboratory Medicine Practice Guidelines: Analytical issues for biomarkers of heart failure. Circulation 116(5):e95–e98.
AREDS Research Group (Age-Related Eye Disease Study Research Group). 2001. A randomized, placebo-controlled, clinical trial of high-dose supplementation with vitamins C and E, beta carotene, and zinc for age-related macular degeneration and vision loss: AREDS report no. 8. Archives of Ophthalmology 119(10):1417–1436.
Aronow, W. S. 2006. Management of hyperlipidemia with statins in the older patient. Clinical Interventions in Aging 1(4):433–438.
Babuin, L. and A. S. Jaffe. 2005. Troponin: The biomarker of choice for the detection of cardiac injury. Canadian Medical Association Journal 173(10):1191–1202.
Bachorik, P. S., and J. W. Ross. 1995. National Cholesterol Education Program recommendations for measurement of low-density lipoprotein cholesterol: Executive summary. The National Cholesterol Education Program Working Group on Lipoprotein Measurement. Clinical Chemistry 41(10):1414–1420.
Ballantyne, C. M. 2002. Familial hypercholesterolaemia: Optimum treatment strategies. International Journal of Clinical Practice 130(Suppl):22–26.
Barter, P. J., C. M. Ballantyne, R. Carmena, M. Castro Cabezas, M. J. Chapman, P. Couture, J. de Graaf, P. N. Durrington, O. Raergeman, J. Frohlich, C. D. Furberg, C. Gagne, S. M. Haffner, S. E. Humphries, I. Jungner, R. M. Krauss, P. Kwiterovich, S. Marcovina, C. J. Packard, T. A. Pearson, K. S. Reddy, R. Rosenson, N. Sarrafzadegan, A. D. Sniderman, A. F. Stalenhoef, E. Stein, P. J. Talmud, A. M. Tonkin, G. Walldius, and K. M. Williams. 2006. Apo B versus cholesterol in estimating cardiovascular risk and in guiding therapy: Report of the thirty-person/ten-country panel. Journal of Internal Medicine 259(3):247–258.
Barter, P., M. Caulfield, M. Eriksson, S. M. Grundy, J. J. P. Kastelein, M. Komajda, J. Lopez-Sendon, L. Mosca, J.-C. Tardif, D. D. Waters, C. L. Shear, J. H. Revkin, K. A. Buhr, M. R. Fisher, A. R. Tall, and B. Brewer. 2007. Effects of torcetrapib in patients at high risk for coronary events. New England Journal of Medicine 357(21):2109–2122.
Beenken, S. W., M. M. Urist, Y. Zhang, R. Desmond, H. Krontiras, H. Medina, and K. I. Bland. 2003. Axillary lymph node status, but not tumor size, predicts locoregional recurrence and overall survival after mastectomy for breast cancer. Annals of Surgery 237(5):732–739.
Benjamin, R. S., H. Choi, H. A. Macapinlac, M. A. Burgess, S. R. Patel, L. L. Chen, D. A. Podoloff, and C. Charnsangavej. 2007. We should desist using RECIST, at least in GIST. Journal of Clinical Oncology 25(13):1760–1764.
Berneis, K. K., and R. M. Krauss. 2002. Metabolic origins and clinical significance of LDL heterogeneity. Journal of Lipid Research 43(9):1363–1379.
Berra, K. 2000. Women, coronary heart disease, and dyslipidemia: Does gender alter detection, evaluation, or therapy? Journal of Cardiovascular Nursing 14(2):59–78.
Berruti, A., D. Generali, M. P. Brizzi, M. Ardine, L. Dogliotti, and A. Bottini. 2005. Is pathologic complete response a valid surrogate parameter of treatment efficacy in HER2 positive breast cancer patients undergoing primary chemotherapy plus trastuz[u]mab? Journal of Clinical Oncology 23(31):8130–8132.
Berruti, A., M. P. Brizzi, D. Generali, M. Ardine, L. Dogliotti, P. Bruzzi, and A. Bottini. 2008. Presurgical systemic treatment of nonmetastatic breast cancer: Facts and open questions. Oncologist 13(11):1137–1148.
Bhakdi, S., J. Torzewski, M. Klouche, and M. Hemmes. 1999. Complement and atherogenesis. Binding of CRP to degraded, nonoxidized LDL enhances complement activation. Arterioscler Thromb Vasc Biol 19(10):2348–2354.
Bisoendial, R. J., J. J. P. Kastelein, J. H. M. Levels, J. J. Zwaginga, B. van den Bogaard, P. H. Reitsma, J. C. M. Meijers, D. Hartman, M. Levi, and E. S. G. Stores. 2005. Activation of inflammation and coagulation after infusion of C-reactive protein in humans. Circulation Research 96(7):714–716.
Böhm-Vélez, M., E. Mendelson, R. Bree, H. Finberg, E. K. Fishman, H. Hricak, F. Laing, D. Sartoris, A. Thurmond, and S. Goldstein. 2000. Ovarian cancer screening. American College of Radiology. ACR Appropriateness Criteria. Radiology 215(Suppl):861–871.
Bolibar, I., A. Von Eckardstein, G. Assmann, S. Thompson, ECAT Angina Pectoris Study Group, and European Concerted Action on Thrombosis and Disabilities. 2000. Shortterm prognostic value of lipid measurements in patients with angina pectoris. The ECAT Angina Pectoris Study Group: European Concerted Action on Thrombosis and Disabilities. Thrombosis and Haemostasis 84(6):955–960.
Briel, M., I. Ferreira-Gonzalez, J. J. You, P. J. Karanicolas, E. A. Akl, P. Wu, B. Blechacz, D. Bassler, X. Wei, A. Sharman, I. Whitt, S. Alves da Silva, Z. Khalid, A. J. Nordmann, Q. Zhou, S. D. Walter, N. Vale, N. Bhatnagar, C. O’Regan, E. J. Mills, H. C. Bucher, V. M. Montori, and G. H. Guyatt. 2009. Association between change in high density lipoprotein cholesterol and cardiovascular disease morbidity and mortality: Systematic review and meta-regression analysis. BMJ 338:b231.
Buckley, B. M., R. Fu, M. Freeman, K. Rogers, and M. Helfand. 2009. C-reactive protein as a risk factor for coronary heart disease: A systematic review and meta-analyses for the U.S. Preventive Services Task Force. Annals of Internal Medicine 151(7):483–495.
Buijs, M., I. R. Kamel, J. A. Vossen, C. S. Georgiades, K. Hong, and J. F. Geschwind. 2007. Assessment of metastatic breast cancer response to chemoembolization with contrast agent enhanced and diffusion-weighted MR imaging. Journal of Vascular and Interventional Radiology 18(8):957–963.
Burstein, M., and J. Samaille. 1958. Determination of serum beta-lipoproteins after selective precipitation by heparin. La Presse Médicale 66:974–975.
Cademartiri, F., G. Luccichenti, E. Maffei, M. Fusaro, A. Palumbo, P. Soliani, M. Sianesi, M. Zompatori, G. Crisi, and G. R. Krestin. 2008. Imaging for oncologic staging and follow-up: Review of current methods and novel approaches. Acta Bio-Medica 79(2):85–91.
Calabro, P., E. Golia, and E. T. Yeh. 2009. CRP and the risk of atherosclerotic events. Seminars in Immunopathology 31(1):79–94.
Califf, R. M., K. S. Pieper, W. R. Harlan Jr., and K. L. Lee. 1992. Serum cholesterol in patients undergoing cardiac catheterization for suspected coronary artery disease: Diagnostic and prognostic implications. Annals of Epidemiology 2(1–2):137–145.
Campos, H., G. O. Roederer, S. Lussier-Cacan, J. Davignon, and R. M. Krauss. 1995. Predominance of large LDL and reduced HDL2 cholesterol in normolipidemic men with coronary artery disease. Arteriosclerosis, Thrombosis, and Vascular Biology 15(8):1043–1048.
Canner, P. L., K. G. Berge, N. K. Wenger, J. Stamler, L. Friedman, R. J. Prineas, and W. Friedewald. 1986. Fifteen year mortality in Coronary Drug Project patients: Long-term benefit with niacin. Journal of the American College of Cardiology 8(6):1245–1255.
Cannon, C. P. 2010. Balancing the benefits of statins versus a new risk—diabetes. The Lancet 375(9716):700–701.
Cannon, C. P., W. S. Weintraub, L. A. Demopoulos, R. Vicari, M. J. Frey, N. Lakkis, F. J. Neumann, D. H. Robertson, P. T. Delucca, P. M. Dibattiste, C. M. Gibson, and E. Braunwald. 2001. Comparison of early invasive and conservative strategies in patients with unstable coronary syndromes treated with the glycoprotein IIb/IIIa inhibitor tirofiban. New England Journal of Medicine 344(25):1879–1887.
Cannon, C. P., E. Braunwald, C. H. McCabe, D. J. Rader, J. L. Rouleau, R. Belder, S. V. Joyal, K. A. Hill, M. A. Pfeffer, and A. M. Skene. 2004. Intensive versus moderate lipid lowering with statins after acute coronary syndromes. New England Journal of Medicine 350(15):1495–1504.
Cardinale, D., M. T. Sandri, A. Martinoni, A. Tricca, M. Civelli, G. Lamantia, S. Cinieri, G. Martinelli, C. M. Cipolla, and C. Fiorentini. 2000. Left ventricular dysfunction predicted by early troponin I release after high-dose chemotherapy. Journal of the American College of Cardiology 36(2):517–522.
Cardinale, D., M. T. Sandri, A. Martinoni, E. Borghini, M. Civelli, G. Lamantia, S. Cinieri, G. Martinelli, C. Fiorentini, and C. M. Cipolla. 2002. Myocardial injury revealed by plasma troponin I in breast cancer treated with high-dose chemotherapy. Annals of Oncology 13(5):710–715.
Cardinale, D., A. Colombo, M. T. Sandri, G. Lamantia, N. Colombo, M. Civelli, G. Martinelli, F. Veglia, C. Fiorentini, and C. M. Cipolla. 2006. Prevention of high-dose chemotherapy-induced cardiotoxicity in high-risk patients by angiotensin-converting enzyme inhibition. Circulation 114(23):2474–2481.
Carrió, I. 2008. EANM-ESR white paper on multimodality imaging. European Journal of Nuclear Medicine and Molecular Imaging 35(3):673.
Casas, J. P., T. Shah, A. D. Hingorani, J. Danesh, and M. B. Pepys. 2008. C-reactive protein and coronary heart disease: A critical review. Journal of Internal Medicine 264(4):295–314.
Castelli, W. P. 1996. Lipids, risk factors and ischaemic heart disease. Atherosclerosis 124(Suppl): S1–S9.
Castelli, W. P., R. J. Garrison, P. W. Wilson, R. D. Abbott, S. Kalousdian, and W. B. Kannel. 1986. Incidence of coronary heart disease and lipoprotein cholesterol levels. The Framingham Study. Journal of the American Medical Association 256(20):2835–2838.
Chan, P. S., B. K. Nallamothu, R. A. Hayward. 2009. Rosuvastatin in patients with elevated c-reactive protein. New England Journal of Medicine 350(10):1039.
Chen, Y. N., Z. R. Luo, L. J. Zeng, M. Y. Wu, Y. Z. Wu, and Z. Y. Lin. 2000. Cardiac troponin I: A marker for post-burn cardiac injury. Annals of Clinical Biochemistry 37(4):447–451.
Cheung, Y. C., S. C. Chen, M. Y. Su, L. C. See, S. Hsueh, H. K. Chang, Y. C. Lin, and C. S. Tsai. 2003. Monitoring the size and response of locally advanced breast cancers to neoadjuvant chemotherapy (weekly paclitaxel and epirubicin) with serial enhanced MRI. Breast Cancer Research and Treatment 78(1):51–58.
Choi, H. 2005. Critical issues in response evaluation on computed tomography: Lessons from the gastrointestinal stromal tumor model. Current Oncology Reports 7(4):307–311.
Choi, H., C. Charnsangavej, S. C. Faria, H. A. Macapinlac, M. A. Burgess, S. R. Patel, L. L. Chen, D. A. Podoloff, and R. S. Benjamin. 2007. Correlation of computed tomography and positron emission tomography in patients with metastatic gastrointestinal stromal tumor treated at a single institution with imatinib mesylate: Proposal of new computed tomography response criteria. Journal of Clinical Oncology 25(13):1753–1759.
Chong, E. W., T. Y. Wong, A. J. Kreis, J. A. Simpson, and R. H. Guymer. 2007. Dietary antioxidants and primary prevention of age related macular degeneration: Systematic review and meta-analysis. BMJ 335(7623):755.
Christen, W. G., J. E. Manson, R. J. Glynn, J. M. Gaziano, E. Y. Chew, J. E. Buring, and C. H. Hennekens. 2007. Beta carotene supplementation and age-related maculopathy in a randomized trial of US physicians. Archives of Ophthalmology 125(3):333–339.
Christensen, E. 2008. Choosing the best endpoint. Journal of Hepatology 49(4):672–673.
Citron, M. L. 2004. Dose density in adjuvant chemotherapy for breast cancer. Cancer Investigation 22(4):555–568.
Cohn, E. J., L. E. Strong, W. C. Hughes, D. J. Mulford, J. N. Ashworth, M. Melin, and H. L. Taylor. 1946. Preparation and properties of serum and plasma proteolyproteins. IV. A system for the separation into fractions of the proteins lipoprotein components of biological tissues and fluids. Journal of the American Chemical Society 68:459–475.
Collins, R., J. Armitage, S. Parish, P. Sleight, and R. Peto. 2002. MRC/BHF Heart Protection Study of cholesterol lowering with simvastatin in 20,536 high-risk individuals: A randomised placebo-controlled trial. Lancet 360(9326):7–22.
Contois, J. H., J. P. McConnell, A. A. Sethi, G. Csako, S. Devaraj, D. M. Hoefner, G. R. Warnick, and AACC Lipoproteins and Vascular Diseases Division Working Group on Best Practices. 2009. Apolipoprotein B and cardiovascular disease risk: Position statement from the AACC Lipoproteins and Vascular Diseases Division Working Group on Best Practices. Clinical Chemistry 55(3):407–419.
Cook, N. R. 2007. Use and misuse of the receiver operating characteristic curve in risk prediction. Circulation 115(7):928–935.
Cook, N. R. 2008. Statistical evaluation of prognostic versus diagnostic models: Beyond the ROC curve. Clinical Chemistry 54(1):17–23.
Cook, N. R., and P. M. Ridker. 2009. Advances in measuring the effect of individual predictors of cardiovascular risk: The role of reclassification measures. Annals of Internal Medicine 150(11):795–802.
Cook, N. R., J. E. Buring, and P. M. Ridker. 2006. The effect of including C-reactive protein in cardiovascular risk prediction models for women. Annals of Internal Medicine 145(1):21–29.
Cook, N. R., C. M. Albert, J. M. Gaziano, E. Zaharris, J. MacFadyen, E. Danielson, J. E. Buring, and J. E. Manson. 2007. A randomized factorial trial of vitamins C, E, and beta-carotene in the secondary prevention of cardiovascular events in women: Results from the Women’s Antioxidant Cardiovascular Study (WACS). Archives of Internal Medicine 167(15):1610–1618.
The Coronary Drug Project Research Group. 1975. Clofibrate and niacin in coronary heart disease. Journal of the American Medical Association 231(4):360–381.
Correia, L. C., M. S. Rocha, and J. P. Esteves. 2009. HDL-cholesterol level provides additional prognosis in acute coronary syndromes. International Journal of Cardiology 136(3):307–314.
Cromwell, W. C., J. D. Otvos, M. J. Keyes, M. J. Pencina, L. Sullivan, R. S. Vasan, P. W. Wilson, and R. B. D’Agostino. 2007. LDL particle number and risk of future cardiovascular disease in the Framingham Offspring Study—Implications for LDL management. Journal of Clinical Lipidology 1(6):583–592.
Danesh, J., R. Collins, P. Appleby, and R. Peto. 1998. Association of fibrinogen, C-reactive protein, albumin, or leukocyte count with coronary heart disease: Meta-analyses of prospective studies. Journal of the American Medical Association 279(18):1477–1482.
Danesh, J., P. Whincup, M. Walker, L. Lennon, A. Thomson, P. Appleby, J. R. Gallimore, and M. B. Pepys. 2000. Low grade inflammation and coronary heart disease: Prospective study and updated meta-analyses. British Medical Journal 321(7255):199–204.
Danesh, J., J. G. Wheeler, G. M. Hirschfield, S. Eda, G. Eiriksdottir, A. Rumley, G. D. O. Lowe, M. B. Pepys, and V. Gudnason. 2004. C-reactive protein and other circulating markers of inflammation in the prediction of coronary heart disease. New England Journal of Medicine 350(14):1387–1397.
Daniels, L. B., G. A. Laughlin, P. Clopton, A. S. Maisel, and E. Barrett-Connor. 2008a. Minimally elevated cardiac troponin T and elevated N-terminal pro-B-type natriuretic peptide predict mortality in older adults. Results from the Rancho Bernardo Study. Journal of the American College of Cardiology 52(6):450–459.
Daniels, L. B., G. A. Laughlin, M. J. Sarno, R. Bettencourt, R. L. Wolfert, and E. Barrett-Connor. 2008b. Lipoprotein-associated phospholipase A2 is an independent predictor of incident coronary heart disease in an apparently healthy older population. The Rancho Bernardo Study. Journal of the American College of Cardiology 51(9):913–919.
de Beer, F. C., A. K. Soutar, M. L. Baltz, I. Trayner, A. Feinstein, and M. B. Pepys. 1982. Low density and very low density lipoproteins are selectively bound by aggregated C-reactive protein. Journal of Experimental Medicine 156(1):230–242.
DeFilippi, C., S. Wasserman, S. Rosanio, E. Tiblier, H. Sperger, M. Tocchi, R. Christenson, B. Uretsky, M. Smiley, J. Gold, H. Muniz, J. Badalamenti, C. Herzog, and W. Henrich. 2003. Cardiac troponin T and C-reactive protein for predicting prognosis, coronary atherosclerosis, and cardiomyopathy in patients undergoing long-term hemodialysis. Journal of the American Medical Association 290(3):353–359.
Desar, I. M., C. M. van Herpen, H. W. van Laarhoven, J. O. Barentsz, W. J. Oyen, and W. T. van der Graaf. 2009. Beyond RECIST: Molecular and functional imaging techniques for evaluation of response to targeted therapy. Cancer Treatment Reviews 35(4):309–321.
Dhani, N., D. Tu, D. J. Sargent, L. Seymour, and M. J. Moore. 2009. Alternate endpoints for screening phase II studies. Clinical Cancer Research 15(6):1873–1882.
Dose Schwarz, J., M. Bader, L. Jenicke, G. Hemminger, F. Jänicke, and N. Avril. 2005. Early prediction of response to chemotherapy in metastatic breast cancer using sequential 18F-FDG PET. Journal of Nuclear Medicine 46(7):1144–1150.
Downs, J. R., M. Clearfield, S. Weis, E. Whitney, D. R. Shapiro, P. A. Beere, A. Langendorfer, E. A. Stein, W. Kruyer, and A. M. Gotto. 1998. Primary prevention of acute coronary events with lovastatin in men and women with average cholesterol levels. Journal of the American Medical Association 279:1615–1622.
Durrington, P. N. 1998. Triglycerides are more important in atherosclerosis than epidemiology has suggested. Atherosclerosis 141(Suppl 1):S57–S62.
Eggers, K. M., L. Lind, H. Ahlström, T. Bjerner, C. Ebeling Barbier, A. Larsson, P. Venge, and B. Lindahl. 2008. Prevalence and pathophysiological mechanisms of elevated cardiac troponin I levels in a population-based sample of elderly subjects. European Heart Journal 29(18):2252–2258.
Eisenhauer, E. A., P. Therasse, J. Bogaerts, L. H. Schwartz, D. Sargent, R. Ford, J. Dancey, S. Arbuck, S. Gwyther, M. Mooney, L. Rubinstein, L. Shankar, L. Dodd, R. Kaplan, D. Lacombe, and J. Verweij. 2009. New response evaluation criteria in solid tumours: Revised RECIST guideline (version 1.1). European Journal of Cancer 45(2):228–247.
El Harchaoui, K., W. A. van der Steeg, E. S. G. Stroes, J. A. Kuivenhoven, J. D. Otvos, N. J. Wareham, B. A. Hutten, J. J. P. Kastelein, K.-T. Khaw, and S. M. Boekholdt. 2007. Value of low-density lipoprotein particle number and size as predictors of coronary artery disease in apparently healthy men and women: The EPIC-Norfolk Prospective Population Study. Journal of the American College of Cardiology 49(5):547–553.
Elliott, P., J. C. Chambers, W. Zhang, R. Clarke, J. C. Hopewell, J. F. Peden, J. Erdmann, P. Braund, J. C. Engert, D. Bennett, L. Coin, D. Ashby, I. Tzoulaki, I. J. Brown, S. Mt-Isa, M. I. McCarthy, L. Peltonen, N. B. Freimer, M. Farrall, A. Ruokonen, A. Hamsten, N. Lim, P. Froguel, D. M. Waterworth, P. Vollenweider, G. Waeber, M.-R. Jarvelin, V. Mooser, J. Scott, A. S. Hall, H. Schunkert, S. S. Anand, R. Collins, N. J. Samani, H. Watkins, and J. S. Kooner. 2009. Genetic loci associated with C-reactive protein levels and risk of coronary heart disease. Journal of the American Medical Association 302(1):37–48.
Eng-Wong, J., J. Orzano-Birgani, C. K. Chow, D. Venzon, J. Yao, C. E. Galbo, J. A. Zujewski, and S. Prindiville. 2008. Effect of raloxifene on mammographic density and breast magnetic resonance imaging in premenopausal women at increased risk for breast cancer. Cancer Epidemiology, Biomarkers, and Prevention 17(7):1696–1701.
Feeney, L., and E. R. Berman. 1976. Oxygen toxicity: Membrane damage by free radicals. Investigative Ophthalmology 15(10):789–792.
Feenstra, J., B. A. in’t Veld, P. D. van der Linden, D. E. Grobbee, and B. H. Stricker. 1998. Risk factors for mortality in users of ibopamine. British Journal of Clinical Pharmacology 46(1):71–77.
FHS (Framingham Heart Study). 2009. Framingham Heart Study: A project of the National Heart, Lung and Blood Institute and Boston University. http://www.framinghamheartstudy.org/about/index.html (accessed October 20, 2009).
Fishman, E. K., E. Mendelson, M. Böhm-Vélez, R. Bree, H. Finberg, H. Hricak, F. Laing, D. Sartoris, A. Thurmond, S. Goldstein, and J. Walsh. 2000. Staging and follow-up of ovarian cancer. American College of Radiology. ACR Appropriateness Criteria. Radiology 215(Suppl):899–902.
Fleming, S. M., T. O’Gorman, J. Finn, H. Grimes, K. Daly, and J. J. Morrison. 2000. Cardiac troponin I in pre-eclampsia and gestational hypertension. British Journal of Obstetrics and Gynaecology 107(11):1417–1420.
Fleming, T. R., M. D. Rothmann, and H. L. Lu. 2009. Issues in using progression-free survival when evaluating oncology products. Journal of Clinical Oncology 27(17):2874–2880.
Folsom, A. R., L. E. Chambless, C. M. Ballantyne, J. Coresh, G. Heiss, K. K. Wu, E. Boerwinkle, T. H. Mosley, P. Sorlie, G. Diao, and A. R. Sharrett. 2006. An assessment of incremental coronary risk prediction using C-reactive protein and other novel risk markers: The Atherosclerosis Risk in Communities Study. Archives of Internal Medicine 166(13):1368– 1373.
Foot, D. K., R. P. Lewis, T. A. Pearson, and G. A. Beller. 2000. Demographics and cardiology, 1950–2050. Journal of the American College of Cardiology 35(5 Suppl B):66B–80B.
Frick, M. H., O. Elo, K. Haapa, O. P. Heinonen, P. Heinsalmi, P. Helo, J. K. Huttunen, P. Kaitaniemi, P. Koskinen, V. Manninen et al. 1987. Helsinki Heart Study: Primary-pre-vention trial with gemfibrozil in middle-aged men with dyslipidemia. Safety of treatment, changes in risk factors, and incidence of coronary heart disease. New England Journal of Medicine 317(20):1237–1245.
Funaioli, C., C. Pinto, F. Di Fabio, D. Santini, C. Ceccarelli, E. De Raffaele, S. Fanti, P. Castellucci, C. Longobardi, F. Buggi, and A. A. Martoni. 2007. 18FDG-PET evaluation correlates better than CT with pathological response in a metastatic colon cancer patient treated with bevacizumab-based therapy. Tumori 93(6):611–615.
Gaines Das, R. E., and S. Poole. 1993. The international standard for interleukin-6. Evaluation in an international collaborative study. Journal of Immunological Methods 160(2):147– 153.
Galanis, E., J. C. Buckner, M. J. Maurer, R. Sykora, R. Castillo, K. V. Ballman, and B. J. Erickson. 2006. Validation of neuroradiologic response assessment in gliomas: Measurement by RECIST, two-dimensional, computer-assisted tumor area, and computer-assisted tumor volume methods. Neuro-Oncology 8(2):156–165.
Gehan, E. A., and M. C. Tefft. 2000. Will there be resistance to the RECIST (Response Evaluation Criteria in Solid Tumors)? Journal of the National Cancer Institute 92(3):179–181.
Gofman, J. W., and F. Lindgren. 1950. The role of lipids and lipoproteins in atherosclerosis. Science 111(2877):166–171.
Gofman, J. W., H. B. Jones, F. T. Lindgren, T. P. Lyon, H. A. Elliott, and B. Strisower. 1950a. Blood lipids and human atherosclerosis. Circulation 2(2):161–178.
Gofman, J. W., F. T. Lindgren, H. B. Jones, T. P. Lyon, and B. Strisower. 1950b. Lipoproteins and atherosclerosis. Journal of Gerontology 6:105–119.
Gofman, J., O. De Lalla, and F. F. Glazier, N. K. Lindgren, F. T. 1954. The serum lipoprotein transport system in health, metabolic disorders, atherosclerosis, and coronary heart disease. Plasma 2:413–484.
Goldberg, J., G. Flowerdew, E. Smith, J. A. Brody, and M. O. Tso. 1988. Factors associated with age-related macular degeneration: Analysis of data from the first National Health and Nutrition Examination Survey. American Journal of Epidemiology 128(4):700–710.
Goldstein, J. L., W. R. Hazzard, H. G. Schrott, E. L. Bierman, and A. G. Motulsky. 1973. Hyperlipidemia in coronary heart disease: Lipid levels in 500 survivors of myocardial infarction. Journal of Clinical Investigation 52:1533–1543.
Goldstein, D., B. S. Tan, M. Rossleigh, W. Haindl, B. Walker, and J. Dixon. 2005. Gastrointestinal stromal tumours: Correlation of F-FDG gamma camera-based coincidence positron emission tomography with CT for the assessment of treatment response—An AGITG study. Oncology 69(4):326–332.
Gordon, D. J., and B. M. Rifkind. 1989. High-density lipoprotein—The clinical implications of recent studies. New England Journal of Medicine 321(19):1311–1316.
Gorecki, A., B. Bednarz, T. Jaxa-Chamiec, P. Maciejewski, R. Lukaszewicz, L. Ceremuzynski, and A. Dyduszynski. 2004. Lipid profile during the first 24 hours after myocardial infarction has significant prognostic value. Kardiologia Polska 60(3):229–236, 237.
Greenland, P., M. D. Knoll, J. Stamler, J. D. Neaton, A. R. Dyer, D. B. Garside, P. W. Wilson. 2003. Major risk factors as antecedents of fatal and nonfatal coronary heart disease events. Journal of the American Medical Association 290(7):891-897.
Griffin, J. H., K. Kojima, C. L. Banka, L. K. Curtiss, and J. A. Fernández. 1999. High-density lipoprotein enhancement of anticoagulant activities of plasma protein S and activated protein C. Journal of Clinical Investigation 103(2):219–227.
Gritz, D. C., M. Srinivasan, S. D. Smith, U. Kim, T. M. Lietman, J. H. Wilkins, B. Priyadharshini, R. K. John, S. Aravind, N. V. Prajna, R. Duraisami Thulasiraj, and J. P. Whitcher. 2006. The Antioxidants in Prevention of Cataracts Study: Effects of antioxidant supplements on cataract progression in South India. British Journal of Ophthalmology 90(7):847–851.
Grossi, F., O. Belvedere, G. Fasola, T. Ceschia, S. Meduri, A. Sibau, C. Sacco, M. Bazzocchi, G. Talmassons, A. Morelli, F. Barbone, and A. Sobrero. 2004. Tumor measurements on computed tomographic images of non-small cell lung cancer were similar among cancer professionals from different specialties. Journal of Clinical Epidemiology 57(8):804–808.
Guest, T. M., A. V. Ramanathan, P. G. Tuteur, K. B. Schechtman, J. H. Ladenson, and A. S. Jaffe. 1995. Myocardial injury in critically ill patients: A frequently unrecognized complication. Journal of the American Medical Association 273(24):1945–1949.
Hamm, C. W., and E. Braunwald. 2000. A classification of unstable angina revisited. Circulation 102(1):118–122.
Havel, R. J., H. A. Eder, and J. H. Bragdon. 1955. The distribution and chemical composition of ultracentrifugally separated lipoproteins in human serum. The Journal of Clinical Investigation 34:1345–1353.
Hayward, R. A., T. P. Hofer, and S. Vijan. 2006. Narrative review: Lack of evidence for recommended low-density lipoprotein treatment targets: A solvable problem. Annals of Internal Medicine 145(7):520-530.
Heidenreich, P. A., T. Alloggiamento, K. Melsop, K. M. McDonald, A. S. Go, and M. A. Hlatky. 2001. The prognostic value of troponin in patients with non-ST elevation acute coronary syndromes: A meta-analysis. Journal of the American College of Cardiology 38(2):478–485.
Heinonen, O. P., and D. Albanes. 1994. The effect of vitamin E and beta carotene on the incidence of lung cancer and other cancers in male smokers. New England Journal of Medicine 330(15):1029–1035.
Heliovaara, M., P. Knekt, K. Aho, R. K. Aaran, G. Alfthan, and A. Aromaa. 1994. Serum anti-oxidants and risk of rheumatoid arthritis. Annals of the Rheumatic Diseases 53(1):51–53.
Hennekens, C., J. Buring, J. Manson, M. Stampfer, B. Rosner, N. Cook, C. Belanger, F. LaMotte, J. M. Gaziano, P. Ridker, W. Willett, and R. Peto. 1996. Lack of effect of long-term supplementation with beta-carotene on the incidence of malignant neoplasms and cardiovascular disease. New England Journal of Medicine 334(18):1145–1149.
Henson, J. W., P. Gaviani, and R. G. Gonzalez. 2005. MRI in treatment of adult gliomas. Lancet Oncology 6(3):167–175.
Herrington, D. M., D. M. Reboussin, K. B. Brosnihan, P. C. Sharp, S. A. Shumaker, T. E. Snyder, C. D. Furberg, G. J. Kowalchuk, T. D. Stuckey, W. J. Rogers, D. H. Givens, and D. Waters. 2000. Effects of estrogen replacement on the progression of coronary-artery atherosclerosis. New England Journal of Medicine 343(8):522–529.
HHS (Department of Health and Human Services). 1990. The health benefits of smoking cessation: A report of the Surgeon General. Rockville, MD: HHS.
HHS. 1996. Clinton administration announces plan to speed development and approval of cancer drugs. http://www.hhs.gov/news/press/1996pres/960329.html (accessed February 17, 2010).
Hirschfield, G. M., R. Gallimore, J. A. Gilbertson, M. Benson, and M. B. Pepys. 2003. Systemic inflammation and atherogenesis in apoE knockout mice expressing transgenic human c-reactive protein. Circulation 108(Suppl IV):IV-41. Abstract.
Hirschfield, G. M., J. R. Gallimore, M. C. Kahan, W. L. Hutchinson, C. A. Sabin, G. M. Benson, A. P. Dhillon, G. A. Tennent, and M. B. Pepys. 2005. Transgenic human c-reactive protein is not proatherogenic in apolipoprotein E-deficient mice. Proceedings of the National Academy of Sciences of the United States of America 102(23): 8309-8314.
Hlatky, M. A. 2008. Expanding the orbit of primary prevention—Moving beyond JUPITER. New England Journal of Medicine 359(21):2280–2282.
Hoefner, D. M. 2008. The cholesterol controversy continues. MLO: Medical Laboratory Observer 40(4):24, 26, 28 passim.
Hohenberger, P., and E. Wardelmann. 2006. [Surgical considerations for gastrointestinal stroma tumor]. Der Chirurg 77(1):33–40.
Hopkins, P. N., and R. R. Williams. 1981. A survey of 246 suggested coronary risk factors. Atherosclerosis 40(1):1–52.
Horne, B. D., J. B. Muhlestein, J. F. Carlquist, T. L. Bair, T. E. Madsen, N. I. Hart, and J. L. Anderson. 2000. Statin therapy, lipid levels, C-reactive protein and the survival of patients with angiographically severe coronary artery disease. Journal of the American College of Cardiology 36(6):1774–1780.
Hudis, C. A. 2005. Clinical implications of antiangiogenic therapies. Oncology 19(4 Suppl 3):26–31.
Hulley, S., D. Grady, T. Bush, C. Furberg, D. Herrington, B. Riggs, E. Vittinghoff, and for the Heart and Estrogen/progestin Replacement Study Research Group. 1998. Randomized trial of estrogen plus progestin for secondary prevention of coronary heart disease in postmenopausal women. Journal of the American Medical Association 280(7):605–613.
Husband, J. E., L. H. Schwartz, J. Spencer, L. Ollivier, D. M. King, R. Johnson, and R. Reznek. 2004. Evaluation of the response to treatment of solid tumours—A consensus statement of the International Cancer Imaging Society. British Journal of Cancer 90(12):2256–2260.
Hylton, N. 2006. MR imaging for assessment of breast cancer response to neoadjuvant chemotherapy. Magnetic Resonance Imaging Clinics of North America 14(3):383–389, vii.
Imamoto, A., L. M. Beltrán, and J. DiGiovanni. 1991. Evidence for autocrine/paracrine growth stimulation by transforming growth factor-alpha during the process of skin tumor promotion. Molecular Carcinogenesis 4(1):52–60.
Inazu, A., M. L. Brown, C. B. Hesler, L. B. Agellon, J. Koizumi, K. Takata, Y. Muruhama, H. Mabuchi, and A. R. Tall. 1990. Increased high-density lipoprotein levels caused by a common cholesteryl-ester transfer protein gene mutation. New England Journal of Medicine 323(18):1234–1238.
IOM (Institute of Medicine). 2000a. Dietary Reference Intakes for vitamin A, vitamin K, arsenic, boron, chromium, copper, iodine, iron, manganese, molybdenum, nickel, silicon, vanadium, and zinc. Washington, DC: National Academy Press.
IOM. 2000b. Dietary Reference Intakes for vitamin C, vitamin E, selenium, and carotenoids. Washington, DC: National Academy Press.
IOM. 2007. Cancer biomarkers: The promises and challenges of improving detection and treatment. Washington, DC: The National Academies Press.
IOM. 2009. Accelerating the development of biomarkers for drug safety. Washington, DC: The National Academies Press.
Jaffe, A. S. 2001. Elevations in cardiac troponin measurements: False false-positives: The real truth. Cardiovascular Toxicology 1(2):87–92.
Jaffe, A. S., J. Ravkilde, R. Roberts, U. Naslund, F. S. Apple, M. Galvani, and H. Katus. 2000. It’s time for a change to a troponin standard. Circulation 102(11):1216–1220.
James, P., C. J. Ellis, R. M. L. Whitlock, A. R. McNeil, J. Henley, and N. E. Anderson. 2000. Relation between troponin T concentration and mortality in patients presenting with an acute stroke: Observational study. British Medical Journal 320(7248):1502–1504.
Javitt, M. C. 2007. ACR Appropriateness Criteria on staging and follow-up of ovarian cancer. Journal of the American College of Radiology 4(9):586–589.
Jennings, D., N. Raghunand, and R. J. Gillies. 2008. Imaging hemodynamics. Cancer Metastasis Reviews 27(4):589–613.
Jensen, M. M., J. T. Jørgensen, T. Binderup, and A. Kjaer. 2008. Tumor volume in subcutaneous mouse xenografts measured by microCT is more accurate and reproducible than determined by 18F-FDG-microPET or external caliper. BMC Medical Imaging 8:16.
Jiang, X. C., L. B. Agellon, A. Walsh, J. L. Breslow, and A. Tall. 1992. Dietary cholesterol increases transcription of the human cholesteryl ester transfer protein gene in transgenic mice. Dependence on natural flanking sequences. Journal of Clinical Investigation 90(4):1290–1295.
Jiang, R., M. B. Schulze, T. Li, N. Rifai, M. J. Stampfer, E. B. Rimm, and F. B. Hu. 2004. Non-HDL cholesterol and apolipoprotein B predict cardiovascular disease events among men with type 2 diabetes. Diabetes Care 27(8):1991–1997.
Joy, T., and R. A. Hegele. 2008a. Is raising HDL a futile strategy for atheroprotection? Nature Reviews Drug Discovery 7(2):143–155.
Joy, T. R., and R. A. Hegele. 2008b. The failure of torcetrapib: What have we learned? British Journal of Pharmacology 154(7):1379–1381.
Kamel, I. R., and D. A. Bluemke. 2002. Magnetic resonance imaging of the liver: Assessing response to treatment. Topics Magnetic Resonance Imaging 13(3):191–200.
Kannel, W. B., T. R. Dawber, A. Kagan, N. Revotskie, and J. I. Stokes. 1961. Factors of risk in the development of coronary heart disease—six-year follow-up experience. The Framingham Heart Study. Annals of Internal Medicine 55(1):33–50.
Kaplan, I. V., and S. S. Levinson. 1999. When is a heterophile antibody not a heterophile antibody? When it is an antibody against a specific immunogen. Clinical Chemistry 45(5):616–618.
Karrison, T. G., M. L. Maitland, W. M. Stadler, and M. J. Ratain. 2007. Design of phase II cancer trials using a continuous endpoint of change in tumor size: Application to a study of sorafenib and erlotinib in non small-cell lung cancer. Journal of the National Cancer Institute 99(19):1455–1461.
Katritsis, D. G., M. Hossein-Nia, A. Anastasakis, J. Poloniecki, D. W. Holt, A. J. Camm, D. E. Ward, and E. Rowland. 1998. Myocardial injury induced by radiofrequency and low energy ablation: A quantitative study of CK isoforms, CK-MB, and troponin-T concentrations. PACE - Pacing and Clinical Electrophysiology 21(7):1410–1416.
Katrukha, A. G., A. V. Bereznikova, V. L. Filatov, T. V. Esakova, O. V. Kolosova, K. Pettersson, T. Lövgren, T. V. Bulargina, I. R. Trifonov, N. A. Gratsiansky, K. Pulkki, L. M. Voipio-Pulkki, and N. B. Gusev. 1998. Degradation of cardiac troponin I: Implication for reliable immunodetection. Clinical Chemistry 44(12):2433–2440.
Kerbel, R. S. 2006. Antiangiogenic therapy: A universal chemosensitization strategy for cancer? Science 312(5777):1171–1175.
Khot, U. N., M. B. Khot, C. T. Bajzer, S. K. Sapp, E. M. Ohman, S. J. Brener, S. G. Ellis, A. M. Lincoff, and E. J. Topol. 2003. Prevalence of conventional risk factors in patients with coronary heart disease. Journal of the American Medical Association 290(7):898–904.
Kimberly, M. M., H. W. Vesper, S. P. Caudill, G. R. Cooper, N. Rifai, F. Dati, and G. L. Myers. 2003. Standardization of immunoassays for measurement of high-sensitivity C-reactive protein. Phase I: Evaluation of secondary reference materials. Clinical Chemistry 49(4):611–616.
Kimberly, M. M., S. P. Caudill, H. W. Vesper, E. A. Monsell, W. G. Miller, R. Rej, N. Rifai, F. Dati, and G. L. Myers. 2009. Standardization of high-sensitivity immunoassays for measurement of C-reactive protein; II: Two approaches for assessing commutability of a reference material. Clinical Chemistry 55(2):342–350.
Kneckt, P., M. Heliovaara, A. Rissanen, A. Aromaa, and R. K. Aaran. 1992. Serum antioxidant vitamins and risk of cataract. BMJ 305(6866):1392–1394.
Knopp, R. H., P. Paramsothy, B. M. Retzlaff, B. Fish, C. Walden, A. Dowdy, C. Tsunehara, K. Aikawa, and M. C. Cheung. 2005. Gender differences in lipoprotein metabolism and dietary response: Basis in hormonal differences and implications for cardiovascular disease. Current Atherosclerosis Reports 7(6):472–479.
Knopp, R. H., P. Paramsothy, B. Atkinson, and A. Dowdy. 2008. Comprehensive lipid management versus aggressive low-density lipoprotein lowering to reduce cardiovascular risk. American Journal of Cardiology 101(8A):48B–57B.
Kolata, G. 2009. Study dismisses protein’s role in heart disease. The New York Times, July 1, A18.
Krauss, R. M. 1995. Dense low density lipoproteins and coronary artery disease. American Journal of Cardiology 75(6):53B–57B.
Krauss, R. M., and D. J. Burke. 1982. Identification of multiple subclasses of plasma low density lipoproteins in normal humans. Journal of Lipid Research 23(1):97–104.
Kronmal, R. A., K. C. Cain, Z. Ye, and G. S. Omenn. 1993. Total serum cholesterol levels and mortality risk as a function of age: A report based on the Framingham data. Archives of Internal Medicine 153(9):1065–1073.
Krumholz, H. M., and T. H. Lee. 2008. Redefining quality—Implications of recent clinical trials. New England Journal of Medicine 358(24):2537–2539.
LaRosa, J. C., S. M. Grundy, D. D. Waters, C. Shear, P. Barter, J.-C. Fruchart, A. M. Gotto, H. Greten, J. J. P. Kastelein, J. Shepherd, N. K. Wenger, and the Treating to New Targets Investigators. 2005. Intensive lipid lowering with atorvastatin in patients with stable coronary disease. New England Journal of Medicine 352(14):1425–1435.
Lathia, C. D., D. Amakye, W. Dai, C. Girman, S. Madani, J. Mayne, P. MacCarthy, P. Pertel, L. Seman, A. Stoch, P. Tarantino, C. Webster, S. Williams, and J. A. Wagner. 2009. The value, qualification, and regulatory use of surrogate end points in drug development. Clinical Pharmacology and Therapeutics 86(1):32–43.
Latini, R., S. Masson, I. S. Anand, E. Missov, M. Carlson, T. Vago, L. Angelici, S. Barlera, G. Parrinello, A. P. Maggioni, G. Tognoni, and J. N. Cohn. 2007. Prognostic value of very low plasma concentrations of troponin T in patients with stable chronic heart failure. Circulation 116(11):1242–1249.
Lauer, B., C. Niederau, U. Kühl, M. Schannwell, M. Pauschinger, B. E. Strauer, and H. P. Schultheiss. 1997. Cardiac troponin T in patients with clinically suspected myocarditis. Journal of the American College of Cardiology 30(5):1354–1359.
Ledue, T. B., and N. Rifai. 2003. Preanalytic and analytic sources of variations in C-reactive protein measurement: Implications for cardiovascular disease risk assessment. Clinical Chemistry 49(8):1258–1271.
Lee, I. M., N. R. Cook, J. E. Manson, J. E. Buring, and C. H. Hennekens. 1999. Beta-carotene supplementation and incidence of cancer and cardiovascular disease: The Women’s Health Study. Journal of the National Cancer Institute 91(24):2102–2106.
Leimgruber, A., S. Ostermann, E. J. Yeon, E. Buff, P. P. Maeder, R. Stupp, and R. A. Meuli. 2006. Perfusion and diffusion MRI of glioblastoma progression in a four-year prospective temozolomide clinical trial. International Journal of Radiation Oncology, Biology, Physics 64(3):869–875.
Liao, J. K., and U. Laufs. 2005. Pleiotropic effects of statins. Annual Review of Pharmacology and Toxicology 45:89–118.
Libby, P., and P. M. Ridker. 1999. Novel inflammatory markers of coronary risk: Theory versus practice. Circulation 100(11):1148–1150.
Libby, P., and P. Theroux. 2005. Pathophysiology of coronary artery disease. Circulation 111(25):3481–3488.
Lichtenstein, A. V. 2005. Cancer as a programmed death of an organism. Biochemistry (Mosc) 70(9):1055–1064.
Lin, N. U., L. A. Carey, M. C. Liu, J. Younger, S. E. Come, M. Ewend, G. J. Harris, E. Bullitt, A. D. Van den Abbeele, J. W. Henson, X. Li, R. Gelman, H. J. Burstein, E. Kasparian, D. G. Kirsch, A. Crawford, F. Hochberg, and E. P. Winer. 2008. Phase II trial of lapatinib for brain metastases in patients with human epidermal growth factor receptor 2-positive breast cancer. Journal of Clinical Oncology 26(12):1993–1999.
Lipid Research Clinics Program. 1984a. The lipid research clinics coronary primary prevention trial results I. Reduction in incidence of coronary heart disease. Journal of the American Medical Association 251(3):351–364.
Lipid Research Clinics Program. 1984b. The lipid research clinics coronary primary prevention trial results II. The relationship of reduction in incidence of coronary heart disease to cholesterol lowering. Journal of the American Medical Association 251(3):365–374.
Lipid Research Clinics Program. 1984c. The relationship of reduction in incidence of coronary heart disease to cholesterol lowering. Journal of the American Medical Association 252(18):2545–2548.
Liu, S., I. M. Lee, U. Ajani, S. R. Cole, J. E. Buring, J. E. Manson, and Physicians’ Health Study. 2001. Intake of vegetables rich in carotenoids and risk of coronary heart disease in men: The Physicians’ Health Study. International Journal of Epidemiology 30(1):130–135.
Llovet, J. M., A. M. Di Bisceglie, J. Bruix, B. S. Kramer, R. Lencioni, A. X. Zhu, M. Sherman, M. Schwartz, M. Lotze, J. Talwalkar, and G. J. Gores. 2008. Design and endpoints of clinical trials in hepatocellular carcinoma. Journal of the National Cancer Institute 100(10):698–711.
Lloyd-Jones, D., R. Adams, M. Carnethon, G. De Simone, T. B. Ferguson, K. Flegal, E. Ford, K. Furie, A. Go, K. Greenlund, N. Haase, S. Hailpern, M. Ho, V. Howard, B. Kissela, S. Kittner, D. Lackland, L. Lisabeth, A. Marelli, M. McDermott, J. Meigs, D. Mozaffarian, G. P. Sorlie, R. Stafford, J. Steinberger, T. Thom, S. Wasserthiel-Smoller, N. Wong, J. Wylie-Rosett, and Y. Hong. 2009. Heart disease and stroke statistics 2009 update: A report from the American Heart Association Statistics Committee and Stroke Statistics Subcomittee. Circulation 119(3):e21–e181.
Lopes-Virella, M. F., and G. Virella. 2003. The role of immune and inflammatory processes in the development of macrovascular disease in diabetes. Frontiers in Bioscience 8: s750–s768.
Macheboeuf, M. 1929. Recherches sur les phosphoaminolipides et les sterids du serum et du plasma sanguins. II Etude physiochimique de la fraction proteidique la plus riche en phospholipids et in sterides. Bulletin de la Société de chimie biologique 11:485–503.
MacMahon, S., R. Peto, R. Collins, J. Godwin, S. MacMahon, J. Cutler, P. Sorlie, R. Abbott, R. Collins, J. Neaton, R. Abbott, A. Dyer, and J. Stamler. 1990. Blood pressure, stroke, and coronary heart disease, I: Prolonged differences in blood pressure: Prospective observational studies corrected for the regression dilution bias. Lancet 335(8692):765–774.
Manninen, V., L. Tenkanen, P. Koskinen, J. K. Huttunen, M. Manttari, O. P. Heinonen, and M. H. Frick. 1992. Joint effects of serum triglyceride and LDL cholesterol and HDL cholesterol concentrations on coronary heart disease risk in the Helsinki Heart Study. Implications for treatment. Circulation 85(1):37–45.
Marcus, C. D., V. Ladam-Marcus, C. Cucu, O. Bouché, L. Lucas, and C. Hoeffel. 2009. Imaging techniques to evaluate the response to treatment in oncology: Current standards and perspectives. Critical Reviews in Oncology/Hematology 72(3):217–238.
Marques-Vidal, P., J. Amar, J. P. Cambou, and B. Chamontin. 1996. Relationships between blood pressure components, lipids and lipoproteins in normotensive men. Journal of Human Hypertension 10(4):239–244.
McNamara, J. R., G. R. Warnick, and G. R. Cooper. 2006. A brief history of lipid and lipoprotein measurements and their contribution to clinical chemistry. Clinica Chimica Acta 369(2):158–167.
Mensah, G. A., and D. W. Brown. 2007. An overview of cardiovascular disease burden in the United States. Health Affairs 26(1):38–48.
Michos, E. D., and R. S. Blumenthal. 2009. Prevalence of low low-density lipoprotein cholesterol with elevated high sensitivity C-reactive protein. Journal of the American College of Cardiology 53(11):931–935.
Miller, W. G., P. P. Waymack, F. P. Anderson, S. F. Ethridge, and E. C. Jayne. 2002. Performance of four homogeneous direct methods for LDL-cholesterol. Clinical Chemistry 48(3):489–498.
Miremadi, S., A. Sniderman, and J. Frohlich. 2002. Can measurement of serum apolipoprotein B replace the lipid profile monitoring of patients with lipoprotein disorders? Clinical Chemistry 48(3):484–488.
Missov, E., C. Calzolari, and B. Pau. 1997. Circulating cardiac troponin I in severe congestive heart failure. Circulation 96(9):2953–2958.
Monteil, J., N. Mahmoudi, S. Leobon, P. Y. Roudaut, A. El Badaoui, S. Verbeke, L. Venat-Bouvet, J. Martin, V. Le Brun-Ly, S. Lavau-Denes, A. Maubon, P. Bouillet, M. Pouquet, J. C. Vandroux, and N. Tubiana-Mathieu. 2009. Chemotherapy response evaluation in metastatic colorectal cancer with FDG PET/CT and CT scans. Anticancer Research 29(7):2563–2568.
Mora, S., J. D. Otvos, N. Rifai, R. S. Rosenson, J. E. Buring, and P. M. Ridker. 2009a. Lipoprotein particle profiles by nuclear magnetic resonance compared with standard lipids and apolipoproteins in predicting incident cardiovascular disease in women. Circulation 119(7):931–939.
Mora, S., N. Rifai, J. E. Buring, and P. M. Ridker. 2009b. Comparison of LDL cholesterol concentrations by Friedewald calculation and direct measurement in relation to cardiovascular events in 27,331 women. Clinical Chemistry 55(5):888–894.
Morrow, D. A., C. P. Cannon, N. Rifai, M. J. Frey, R. Vicari, N. Lakkis, D. H. Robertson, D. A. Hille, P. T. DeLucca, P. M. DiBattiste, L. A. Demopoulos, W. S. Weintraub, and E. Braunwald. 2001. Ability of minor elevations of troponins I and T to predict benefit from an early invasive strategy in patients with unstable angina and non-ST elevation myocardial infarction: Results from a randomized trial. Journal of the American Medical Association 286(19):2405–2412.
Morrow, D. A., C. P. Cannon, R. L. Jesse, L. K. Newby, J. Ravkilde, A. B. Storrow, A. H. B. Wu, R. H. Christenson, F. S. Apple, G. Francis, and W. Tang. 2007. National Academy of Clinical Biochemistry Laboratory Medicine Practice Guidelines: Clinical characteristics and utilization of biochemical markers in acute coronary syndromes. Clinical Chemistry 53(4):552–574.
Musunuru, K., B. G. Kral, R. S. Blumenthal, V. Fuster, C. Y. Campbell, T. J. Gluckman, R. A. Lange, E. J. Topol, J. T. Willerson, M. Y. Desai, M. H. Davidson, and S. Mora. 2008. The use of high-sensitivity assays for C-reactive protein in clinical practice Nature Clinical Practice. Cardiovascular Medicine 5(10):621–635.
Musunuru, K., M. Orho-Melander, M. P. Caulfield, S. Li, W. A. Salameh, R. E. Reitz, G. Berglund, B. Hedblad, G. Engstrom, P. T. Williams, S. Kathiresan, O. Melander, and R. M. Krauss. 2009. Ion mobility analysis of lipoprotein subfractions identifies three independent axes of cardiovascular risk. Arteriosclerosis Thrombosis and Vascular Biology 29(11):1975–1980.
Mykkanen, L., J. Kuusisto, S. Haffner, M. Laakso, and M. Austin. 1999. LDL size and risk of coronary heart disease in elderly men and women. Arteriosclerosis, Thrombosis, and Vascular Biology 19:2742–2748.
NACB Writing Group Members, A. H. B. Wu, A. S. Jaffe, F. S. Apple, R. L. Jesse, G. L. Francis, D. A. Morrow, L. K. Newby, J. Ravkilde, W. H. Wilson Tang, R. H. Christenson; NACB Committee Members, R. H. Christenson, F. S. Apple, C. P. Cannon, G. L. Francis, R. L. Jesse, D. A. Morrow, L. K. Newby, J. Ravkilde, A. B. Storrow, W. H. Wilson Tang, and A. H. B. Wu. 2007. National Academy of Clinical Biochemistry Laboratory Medicine practice guidelines: Use of cardiac troponin and B-type natriuretic peptide or N-terminal proB-type natriuretic peptide for etiologies other than acute coronary syndromes and heart failure. Clinical Chemistry 53(12):2086–2096.
Nauck, M., G. R. Warnick, and N. M. Rifai. 2002. Methods for measurement of LDL-cholesterol: A critical assessment of direct measurement by homogeneous assays versus calculation. Clinical Chemistry 48:236–254.
NCEP (National Cholesterol Education Program). 2001. Third Report of the National Cholesterol Education Program (NCEP) expert panel on detection, evaluation, and treatment of high blood cholesterol in adults (Adult Treatment Panel III). Bethesda, MD: National Institutes of Health.
Needham, D. M., K. A. Shufelt, G. Tomlinson, J. W. Scholey, and G. E. Newton. 2004. Troponin I and T levels in renal failure patients without acute coronary syndrome: A systematic review of the literature. Canadian Journal of Cardiology 20(12):1212–1218.
Nicoletto, M. O., D. Nitti, L. Pescarini, F. Corbetti, R. Mencarelli, A. Cappetta, A. Galligioni, C. Pogliani, A. Marchet, F. Bozza, C. Ghiotto, L. Griggio, G. Zavagno, M. E. Donach, and C. Di Maggio. 2008. Correlation between magnetic resonance imaging and histopathological tumor response after neoadjuvant chemotherapy in breast cancer. Tumori 94(4):481–488.
Nilsson, J. 2005. CRP—marker or maker of cardiovascular disease? Arterioscler Thromb Vasc Biol 25(8):1527–1528.
Nissen, S. 2008. ENHANCE and ACCORD: Controversy over surrogate end points. Current Cardiology Reports 10(3):159–161.
Nissen, S. E., T. Tsunoda, E. M. Tuzcu, P. Schoenhagen, C. J. Cooper, M. Yasin, G. M. Eaton, M. A. Lauer, W. S. Sheldon, C. L. Grines, S. Halpern, T. Crowe, J. C. Blankenship, and R. Kerensky. 2003. Effect of recombinant ApoA-I Milano on coronary atherosclerosis in patients with acute coronary syndromes: A randomized controlled trial. Journal of the American Medical Association 290(17):2292–2300.
Nofer, J. R., B. Kehrel, M. Fobker, B. Levkau, G. Assmann, and A. von Eckardstein. 2002. HDL and arteriosclerosis: Beyond reverse cholesterol transport. Atherosclerosis 161(1):1–16.
Nordestgaard, B. G. 2009. Does elevated C-reactive protein cause human atherothrombosis? Novel insights from genetics, intervention trials, and elsewhere. Current Opinion in Lipidology 20(5):393–401.
Noterdaeme, O., M. Kelly, P. Friend, Z. Soonowalla, G. Steers, and M. Brady. 2009. Initial assessment of a model relating intratumoral genetic heterogeneity to radiological morphology. British Journal of Radiology 83(986):166–170.
Ogbuanu, I. U., H. Zhang, and W. Karmaus. 2009. Can we apply the Mendelian randomization methodology without considering epigenetic effects? Emerging Themes in Epidemiology 6(3):e1–e10.
Ohara, K., Y. Tanaka, H. Tsunoda, M. Nishida, S. Sugahara, and Y. Itai. 2002. Assessment of cervical cancer radioresponse by serum squamous cell carcinoma antigen and magnetic resonance imaging. Obstetrics and Gynecology 100(4):781–787.
Ollivier, L., J. Leclère, P. Thiesse, D. Di Stefano, and C. Vincent. 2007. [Measurement of tumour response to cancer treatment: Morphologic imaging role]. Bulletin du Cancer 94(2):171–177.
Olson, R. E. 1998. Discovery of the lipoproteins, their role in fat transport and their significance as risk factors. Journal of Nutrition 128(2 Suppl):439S–443S.
Omenn, G. S., G. E. Goodman, M. D. Thornquist, J. Balmes, M. R. Cullen, A. Glass, J. P. Keogh, J. F. L. Meyskens Jr., B. Valanis, J. H. Williams Jr., S. Barnhart, and S. Hammar. 1996. Effects of a combination of beta-carotene and vitamin A on lung cancer and cardiovascular disease. New England Journal of Medicine 334:1150–1155.
Omland, T., J. A. de Lemos, M. S. Sabatine, C. A. Christophi, M. M. Rice, K. A. Jablonski, S. Tjora, M. J. Domanski, B. J. Gersh, J. L. Rouleau, M. A. Pfeffer, E. Braunwald, and the Prevention of Events with Angiotensin Converting Enzyme Inhibition (PEACE) Trial Investigators. 2009. A sensitive cardiac troponin T assay in stable coronary artery disease. New England Journal of Medicine 361(26):2538–2547.
Oram, J. F. 2001. Novel approaches to treating cardiovascular disease: Lessons from Tangier disease. Expert Opinion on Investigational Drugs 10(3):427–438.
Packard, R. R., and P. Libby. 2008. Inflammation in atherosclerosis: From vascular biology to biomarker discovery and risk prediction. Clinical Chemistry 54(1):24–38.
Paffen, E., and M. P. DeMaat. 2006. C-reactive protein in atherosclerosis: A causal factor? Cardiovascular Research 71(1):30–39.
Pai, V. B., and M. C. Nahata. 2000. Cardiotoxicity of chemotherapeutic agents. Incidence, treatment and prevention. Drug Safety 22(4):263–302.
Pantaleo, M. A., M. Nannini, E. Lopci, P. Castellucci, A. Maleddu, F. Lodi, C. Nanni, V. Allegri, M. Astorino, G. Brandi, M. Di Battista, S. Boschi, S. Fanti, and G. Biasco. 2008a. Molecular imaging and targeted therapies in oncology: New concepts in treatment response assessment. A collection of cases. International Journal of Oncology 33(3):443– 452.
Pantaleo, M. A., M. Nannini, A. Maleddu, S. Fanti, V. Ambrosini, C. Nanni, S. Boschi, and G. Biasco. 2008b. Conventional and novel PET tracers for imaging in oncology in the era of molecular therapy. Cancer Treatment Reviews 34(2):103–121.
Panteghini, M., W. Gerhardt, F. S. Apple, F. Dati, J. Ravkilde, and A. H. Wu. 2001. Quality specifications for cardiac troponin assays. Clinical Chemistry and Laboratory Medicine 39(2):175–179.
Park, J. O., S. I. Lee, S. Y. Song, K. Kim, W. S. Kim, C. W. Jung, Y. S. Park, Y. H. Im, W. K. Kang, M. H. Lee, K. S. Lee, and K. Park. 2003. Measuring response in solid tumors: Comparison of RECIST and WHO response criteria. Japanese Journal of Clinical Oncology 33(10):533–537.
Pasceri, V., J. T. Willerson, and E. T. H. Yeh. 2000. Direct proinflammatory effect of C-reactive protein on human endothelial cells. Circulation 102(18):2165–2168.
Paul, A., K. W. Ko, L. Li, V. Yechoor, M. A. McCrory, A. J. Szalai, and L. Chan. 2004. C-reactive protein accelerates the progression of atherosclerosis in apolipoprotein E-deficient mice. Circulation 109(5):647–655.
Pearson, T. 2007. The prevention of cardiovascular disease: Have we really made progress? Health Affairs 26(1):49–60.
Pearson, T. A., G. A. Mensah, R. W. Alexander, J. L. Anderson, R. O. Cannon, M. Criqui, Y. Y. Fadl, S. P. Fortmann, Y. Hong, G. L. Myers, N. Rifai, S. C. Smith, K. Taubert, R. P. Tracy, and F. Vinicor. 2003. Markers of inflammation and cardiovascular disease: Application to clinical and public health practice. Circulation 107(3):499–511.
Pederson, K. O. 1947. On a low-density lipoprotein appearing in normal human plasma. Journal of Physical Chemistry 51:156–163.
Pepys, M. B. 2005. CRP or not CRP? That is the question. Arteriosclerosis, Thrombosis, and Vascular Biology 25(6):1091–1094.
Pepys, M. B., I. F. Rowe, and M. L. Baltz. 1985. C-reactive protein: Binding to lipids and lipoproteins. International Review of Experimental Pathology 27:83–111.
Pepys, M. B., G. M. Hirschfield, G. A. Tennent, J. R. Gallimore, M. C. Kahan, V. Bellotti, P. N. Hawkins, R. M. Myers, M. D. Smith, A. Polara, A. J. A. Cobb, S. V. Ley, J. A. Aquilina, C. V. Robinson, I. Sharif, G. A. Gray, C. A. Sabin, M. C. Jenvey, S. E. Kolstoe, D. Thompson, and S. P. Wood. 2006. Targeting C-reactive protein for the treatment of cardiovascular disease. Nature 440(27):1217–1221.
Pineda, J., F. Marin, P. Marco, V. Roldan, J. Valencia, J. M. Ruiz-Nodar, D. H. Romero, F. Sogorb, and G. Y. Lip. 2009. The prognostic value of biomarkers after a premature myocardial infarction. International Journal of Cardiology EPub ahead of print.
Plump, A. S., J. D. Smith, T. Hayek, K. Aalto-Setala, A. Walsh, J. G. Verstuyft, E. M. Rubin, and J. L. Breslow. 1992. Severe hypercholesterolemia and atherosclerosis in apolipoprotein E- deficient mice created by homologous recombination in ES cells. Cell 71(2):343–353.
Poole, S., D. Walker, R. E. Gaines Das, J. R. Gallimore, and M. B. Pepys. 1998. The first international standard for serum amyloid A protein (SAA). Evaluation in an international collaborative study. Journal of Immunological Methods 214(1–2):1–10.
Pugnale, N., F. Waridel, H. Bouzourène, A. Boubaker, M. Pugnale, R. C. Gaillard, and F. Gomez. 2003. Pharyngeal pituitary non-functioning adenoma with normal intra-sellar gland: Massive tumor shrinkage on octreotide therapy. European Journal of Endocrinology 148(3):357–364.
Rader, D. J. 2006. Molecular regulation of HDL metabolism and function: Implications for novel therapies. Journal of Clinical Investigation 116(12):3090–3100.
Ratain, M. J., R. Mick, R. L. Schilsky, and M. Siegler. 1993. Statistical and ethical issues in the design and conduct of phase I and II clinical trials of new anticancer agents. Journal of the National Cancer Institute 85(20):1637–1643.
Ridker, P. M. 2007. C-reactive protein and the prediction of cardiovascular events among those at intermediate risk. Journal of the American College of Cardiology 49(21):2129– 2138.
Ridker, P. M., M. Cushman, M. J. Stampfer, R. P. Tracy, and C. H. Hennekens. 1997. Inflammation, aspirin, and the risk of cardiovascular disease in apparently healthy men. The New England Journal of Medicine 336(14):973–979.
Ridker, P. M., N. Rifai, M. A. Pfeffer, F. M. Sacks, L. A. Moye, S. Goldman, G. C. Flaker, and E. Braunwald. 1998. Inflammation, pravastatin, and the risk of coronary events after myocardial infarction in patients with average cholesterol levels. Cholesterol Recurrent Events (CARE) Investigators. Circulation 98(9):839–844.
Ridker, P. M., N. Rifai, M. Clearfield, J. R. Downs, S. E. Weis, J. S. Miles, and A. M. Gotto, Jr. 2001. Measurement of c-reactive protein for the targeting of statin therapy in the primary prevention of acute coronary events. New England Journal Medicine 344(26): 1959-1965).
Ridker, P. M., E. Danielson, F. A. H. Fonseca, J. Genest, A. M. Gotto Jr., J. J. P. Kastelein, W. Koenig, P. Libby, A. J. Lorenzatti, J. G. MacFadyen, B. G. Nordestgaard, J. Shepherd, J. T. Willerson, and R. J. Glynn. 2008a. Rosuvastatin to prevent vascular events in men and women with elevated C-reactive protein. New England Journal of Medicine 359(21):2195–2207.
Ridker, P. M., N. P. Paynter, N. Rifai, J. M. Caziano, and N. R. Cook. 2008b. C-reactive protein and parental history improve global cardiovascular risk prediction. The Reynolds Risk Score for Men. Circulation 118(22):2243–2251.
Ridker, P. M., E. Danielson, F. A. H. Fonseca, J. Genest, A. M. Gotto, Jr., J. J. P. Kastelein, W. Koenig, P. Libby, A. J. Lorenzatti, J. G. MacFadyen, B. G. Nordestgaard, J. Shepherd, J. T. Willerson, R. J. Glynn, and JUPITER Trial Study Group. 2009. Reduction in C-reactive protein and LDL cholesterol and cardiovascular event rates after initiation of rosuvastatin: A prospective study of the JUPITER trial. The Lancet 373(9670):1175–1182.
Roberts, W. L. 2004. CDC/AHA Workshop on Markers of Inflammation and Cardiovascular Disease: Application to Clinical and Public Health Practice: Laboratory tests available to assess inflammation—Performance and standardization: A background paper. Circulation 110(25):e572–e576.
Roberts, W. L., C. B. Calcote, B. K. De, V. Holmstrom, C. Narlock, F. S. Apple, and D. Whitehair. 1997. Prevention of analytical false-positive increases of cardiac troponin I on the Stratus II analyzer. Clinical Chemistry 43(5):860–861.
Robins, S. J. 2001. Targeting low high-density lipoprotein cholesterol for therapy: Lessons from the Veterans Affairs High-density Lipoprotein Intervention Trial. American Journal of Cardiology 88(12A):19N–23N.
Ross, R. 1993. The pathogenesis of atherosclerosis: A perspective for the 1990s. Nature 362(6423):801–809.
Ross, R. 1999. Atherosclerosis—An inflammatory disease. The New England Journal of Medicine 340(2):115–126.
Rubins, H. B., S. J. Robins, D. Collins, C. L. Fye, J. W. Anderson, M. B. Elam, F. H. Faas, E. Linares, E. J. Schaefer, G. Schectman, T. J. Wilt, and J. Wittes. 1999. Gemfibrozil for the secondary prevention of coronary heart disease in men with low levels of high-density lipoprotein cholesterol. Veterans Affairs High-Density Lipoprotein Cholesterol Intervention Trial Study Group. New England Journal of Medicine 341(6):410–418.
Sacks, F. M., and H. Campos. 2003. Clinical review 163: Cardiovascular endocrinology: Low-density lipoprotein size and cardiovascular disease: A reappraisal. Journal of Clinical Endocrinology and Metabolism 88(10):4525–4532.
Sakatini, T., T. Shirayama, Y. Suzaki, T. Yamamoto, H. Mani, T. Kawasaki, H. Sugihara, and H. Matsubara. 2005. The association between cholesterol and mortality in heart failure. Comparison between patients with and without coronary artery disease. International Heart Journal 46(4):619–629.
Sandri, M. T., D. Cardinale, L. Zorzino, R. Passerini, P. Lentati, A. Martinoni, G. Martinelli, and C. M. Cipolla. 2003. Minor increases in plasma troponin I predict decreased left ventricular ejection fraction after high-dose chemotherapy. Clinical Chemistry 49(2):248– 252.
Sargent, D. J., L. Rubinstein, L. Schwartz, J. E. Dancey, C. Gatsonis, L. E. Dodd, and L. K. Shankar. 2009. Validation of novel imaging methodologies for use as cancer clinical trial end-points. European Journal of Cancer 45(2):290–299.
Sattar, N., D. Preiss, H. M. Murray, P. Welsh, B. M. Buckley, A. J. de Craen, S. R. Seshasai, J. J. McMurray, D. J. Freeman, J. W. Jukema, P. W. Macfarlane, C. J. Packard, D. J. Stott, R. G. Westendorp, J. Shepherd, B. R. Davis, S. L. Pressel, R. Marchioli, R. M. Marfisi, A. P. Maggioni, L. Travazzi, G. Tognoni, J. Kjekshus, T. R. Pedersen, T. J. Cook, A. M. Gotto, M. B. Clearfield, J. R. Downs, H. Nakamura, Y. Ohashi, K. Mizuno, K. K. Ray, I. Ford. 2010. Statins and risk of incident diabetes: A collaborative meta-analysis of randomised statin trials. Lancet 375(9716):735–742.
Scanu, A. 1966. Forms of human serum high density lipoprotein protein. Journal of Lipid Research 7(2):295–306.
Schatz, I. J., K. Masaki, K. Yano, R. Chen, B. L. Rodriguez, and J. D. Curb. 2001. Cholesterol and all-cause mortality in elderly people from the Honolulu Heart Program: A cohort study. Lancet 358(9279):351–355.
Schectman, G., M. Patsches, and E. A. Sasse. 1996. Variability in cholesterol measurements: Comparison of calculated and direct LDL cholesterol determinations. Clinical Chemistry 42(5):732–737.
Schepkin, V. D., T. L. Chenevert, K. Kuszpit, K. C. Lee, C. R. Meyer, T. D. Johnson, A. Rehemtulla, and B. D. Ross. 2006. Sodium and proton diffusion MRI as biomarkers for early therapeutic response in subcutaneous tumors. Magnetic Resonance Imaging 24(3):273–278.
Scher, H. I., S. Halabi, I. Tannock, M. Morris, C. N. Sternberg, M. A. Carducci, M. A. Eisenberger, C. Higano, G. J. Bubley, R. Dreicer, D. Petrylak, P. Kantoff, E. Basch, W. K. Kelly, W. D. Figg, E. J. Small, T. M. Beer, G. Wilding, A. Martin, and M. Hussain. 2008. Design and end points of clinical trials for patients with progressive prostate cancer and castrate levels of testosterone: Recommendations of the Prostate Cancer Clinical Trials Working Group. Journal of Clinical Oncology 26(7):1148–1159.
Schwartz, G. G., A. G. Olsson, M. D. Ezekowitz, P. Ganz, M. F. Oliver, D. Waters, A. Zeiher, B. R. Chaitman, S. Leslie, and T. Stern. 2001. Effects of atorvastatin on early recurrent ischemic events in acute coronary syndromes. The MIRACL study: A randomized controlled trial. Journal of the American Medical Association 285(13):1711–1718.
Scirica, B. M., and D. A. Morrow. 2004. Troponins in acute coronary syndromes. Progress in Cardiovascular Diseases 47(3):177–188.
Seibert, R. M., C. R. Ramsey, J. W. Hines, P. A. Kupelian, K. M. Langen, S. L. Meeks, and D. D. Scaperoth. 2007. A model for predicting lung cancer response to therapy. International Journal of Radiation Oncology, Biology, Physics 67(2):601–609.
Sevinc, A., and N. S. Turhal. 2008. Please, desist RECIST criteria in GIST, at least in me. Onkologie 31(10):556.
Shah, S. H., and J. A. deLemos. 2009. Biomarkers and cardiovascular disease: Determining causality and quantifying contribution to risk assessment. Journal of the American Medical Association 301(1):92–93.
Shah, V. P., J. P. Casas, J. A. Cooper, I. Tzoulaki, R. Sofat, V. McCormack, L. Smeeth, J. E. Deanfield, G. D. Lowe, A. Rumley, F. G. R. Fowkes, S. E. Humphries, and A. D. Hingo-rani. 2009. Critical appraisal of CRP measurement for the prediction of coronary heart disease events: New data and systematic review of 31 prospective cohorts. International Journal of Epidemiology 38(1):217–231.
Sharma, U., K. K. Danishad, V. Seenu, and N. R. Jagannathan. 2009. Longitudinal study of the assessment by MRI and diffusion-weighted imaging of tumor response in patients with locally advanced breast cancer undergoing neoadjuvant chemotherapy. NMR in Biomedicine 22(1):104–113.
Shepherd, J., S. M. Cobbe, I. Ford, C. G. Isles, A. R. Lorimer, P. W. MacFarlane, J. H. McKillop, and C. J. Packard. 1995. Prevention of coronary heart disease with pravastatin in men with hypercholesterolemia. New England Journal of Medicine 333(20):1301–1307.
Shepherd, J., G. J. Blauw, M. B. Murphy, E. L. Bollen, B. M. Buckley, S. M. Cobbe, I. Ford, A. Gaw, M. Hyland, J. W. Jukema, A. M. Kamper, P. W. MacFarlane, A. E. Meinders, J. Norrie, C. J. Packard, I. J. Perry, D. J. Stott, B. J. Sweeney, C. Twomey, and R. G. Westendorp. 2002. Pravastatin in elderly individuals at risk of vascular disease (PROSPER): A randomized controlled trial. Lancet 360:1623–1630.
Singh, U., S. Devaraj, and I. Jialal. 2005. C-reactive protein decreases tissue plasminogen activator activity in human aortic endothelial cells: Evidence that C-reactive protein is a procoagulant. Arteriosclerosis, Thrombosis, and Vascular Biology 25(10):2216–2221.
Sniderman, A. D. 2002. How, when, and why to use apolipoprotein B in clinical practice. American Journal of Cardiology 90(8A):48i–54i.
Sniderman, A. D., and S. M. Marcovina. 2006. Apolipoprotein A1 and B. Clinics in Laboratory Medicine 26(4):733–750.
Specht, J. M., S. L. Tam, B. F. Kurland, J. R. Gralow, R. B. Livingston, H. M. Linden, G. K. Ellis, E. K. Schubert, L. K. Dunnwald, and D. A. Mankoff. 2007. Serial 2-[18F] fluoro-2-deoxy-D-glucose positron emission tomography (FDG-PET) to monitor treatment of bone-dominant metastatic breast cancer predicts time to progression (TTP). Breast Cancer Research and Treatment 105(1):87–94.
Spies, C., V. Haude, R. Fitzner, K. Schröder, M. Overbeck, N. Runkel, and W. Schaffartzik. 1998. Serum cardiac troponin T as a prognostic marker in early sepsis. Chest 113(4):1055–1063.
St-Pierre, A. C., B. Cantin, G. R. Dagenais, P. Mauriege, P. M. Bernard, J. P. Despres, and B. Lamarche. 2005. Low-density lipoprotein subfractions and the long-term risk of ischemic heart disease in men: 13-year follow-up data from the Quebec Cardiovascular Study. Arteriosclerosis Thrombosis and Vascular Biology 25(3):553–559.
Stacchiotti, S., P. Collini, A. Messina, C. Morosi, M. Barisella, R. Bertulli, C. Piovesan, P. Dileo, V. Torri, A. Gronchi, and P. G. Casali. 2009. High-grade soft-tissue sarcomas: Tumor response assessment—Pilot study to assess the correlation between radiologic and pathologic response by using RECIST and Choi criteria. Radiology 251(2):447–456.
Stadler, W. M., and M. J. Ratain. 2000. Development of target-based antineoplastic agents. Investigational New Drugs 18(1):7–16.
Stamler, J., O. Vaccaro, J. D. Neaton, and D. Wentworth. 1993. Diabetes, other risk factors, and 12-yr cardiovascular mortality for men screened in the Multiple Risk Factor Intervention Trial. Diabetes Care 16(2):434–444.
Stegger, L., M. Schäfers, M. Weckesser, and O. Schober. 2008. EANM-ESR white paper on multimodality imaging. European Journal of Nuclear Medicine and Molecular Imaging 35(3):677–680.
Steinberg, D. 1997. A critical look at the evidence for the oxidation of LDL in atherogenesis. Atherosclerosis 131(Suppl):S5–S7.
Steinberg, D., S. Parthasarathy, T. E. Carew, J. C. Khoo, and J. L. Witztum. 1989. Beyond cholesterol: Modifications of low-density lipoprotein that increase its atherogenicity. New England Journal of Medicine 320(14):915–924.
Stewart, D. J. 2008. Re: Design of phase II cancer trials using a continuous endpoint of change in tumor size: Application to a study of sorafenib and erlotinib in non-small cell lung cancer. Journal of the National Cancer Institute 100(6):445–446; author reply 446.
Strassburg, J., T. Junginger, T. Trinh, O. Püttcher, K. Oberholzer, R. J. Heald, and P. Hermanek. 2008. Magnetic resonance imaging (MRI)-based indication for neoadjuvant treatment of rectal carcinoma and the surrogate endpoint CRM status. International Journal of Colorectal Disease 23(11):1099–1107.
Tall, A. R. 1993. Plasma cholesteryl ester transfer protein. Journal of Lipid Research 34(8):1255– 1274.
Tardif, J.-C., T. Heinonen, D. Orloff, and P. Libby. 2006. Vascular biomarkers and surrogates in cardiovascular disease. Circulation 113(25):2936–2942.
Tardif, J. C., J. Gregoire, P. L. L’Allier, R. Ibrahim, J. Lesperance, T. M. Heinonen, S. Kouz, C. Berry, R. Basser, M. A. Lavoie, M. C. Guertin, and J. Rodes-Cabau. 2007. Effects of reconstituted high-density lipoprotein infusions on coronary atherosclerosis: A randomized controlled trial. Journal of the American Medical Association 297(15):1675–1682.
Taylor, K. E., J. C. Giddings, and C. W. van den Berg. 2005. C-reactive protein-induced in vitro endothelial cell activation is an artefact caused by azide and lipopolysaccharide. Arteriosclerosis, Thrombosis, and Vascular Biology 25(6):1225–1230.
Therasse, P., E. A. Eisenhauer, and J. Verweij. 2006. RECIST revisited: A review of validation studies on tumour assessment. European Journal of Cancer 42(8):1031–1039.
Thom, T., N. Haase, W. Rosamond, V. Howard, J. Rumsfeld, T. Manolio, Z. Zheng, K. Flegal, C. O’Donnell, S. Kittner, D. Lloyd-Jones, D. J. Goff, Y. Hong, R. Adams, G. Friday, K. Furie, P. Gorelick, B. Kissela, J. Marler, J. Meigs, V. Roger, S. Sidney, P. Sorlie, J. Steinberger, S. Wasserthiel-Smoller, M. Wilson, and P. Wolf. 2006. Heart disease and stroke statistics—2006 update: A report from the American Heart Association Statistics Committee and Stroke Statistics Subcommittee. Circulation 113(6):e85–e151.
Thygesen, K., J. S. Alpert, H. D. White, Biomarker Group, A. S. Jaffe, F. S. Apple, M. Galvani, H. A. Katus, L. K. Newby, J. Ravkilde, ECG Group, B. Chaitman, P. M. Clemmensen, M. Dellborg, H. Hod, P. Porela, Imaging Group, R. Underwood, J. J. Bax, G. A. Beller, R. Bonow, E. E. Van Der Wall, Intervention Group, J.-P. Bassand, W. Wijns, T. B. Ferguson, P. G. Steg, B. F. Uretsky, D. O. Williams, Clinical Investigation Group, P. W. Armstrong, E. M. Antman, K. A. Fox, C. W. Hamm, E. M. Ohman, M. L. Simoons, Global Perspective Group, P. A. Poole-Wilson, E. P. Gurfinkel, J.-L. Lopez-Sendon, P. Pais, S. Mendis, J.-R. Zhu, Implementation Group, L. C. Wallentin, F. Fernandez-Aviles, K. M. Fox, A. N. Parkhomenko, S. G. Priori, M. Tendera, L.-M. Voipio-Pulkki, ESC Committee for Practice Guidelines, A. Vahanian, A. J. Camm, R. De Caterina, V. Dean, K. Dickstein, G. Filippatos, C. Funck-Brentano, I. Hellemans, S. D. Kristensen, K. McGregor, U. Sechtem, S. Silber, P. Widimsky, J. L. Zamorano, Document Reviewers, J. Morais, S. Brener, R. Harrington, D. Morrow, M. Lim, M. A. Martinez-Rios, S. Steinhubl, G. N. Levine, W. B. Gibler, D. Goff, M. Tubaro, D. Dudek, and N. Al-Attar. 2007. Universal definition of myocardial infarction: Kristian Thygesen, Joseph S. Alpert and Harvey D. White on behalf of the Joint ESC/ACCF/AHA/WHF Task Force for the Redefinition of Myocardial Infarction. Eur Heart J 28(20):2525–2538.
Tran, L. N., M. S. Brown, J. G. Goldin, X. Yan, R. C. Pais, M. F. McNitt-Gray, D. Gjertson, S. R. Rogers, and D. R. Aberle. 2004. Comparison of treatment response classifications between unidimensional, bidimensional, and volumetric measurements of metastatic lung lesions on chest computed tomography. Academic Radiology 11(12):1355–1360.
Tuma, R. S. 2006. Sometimes size doesn’t matter: Reevaluating RECIST and tumor response rate endpoints. Journal of the National Cancer Institute 98(18):1272–1274.
Ullrich, R. T., T. Zander, B. Neumaier, M. Koker, T. Shimamura, Y. Waerzeggers, C. L. Borgman, S. Tawadros, H. Li, M. L. Sos, H. Backes, G. I. Shapiro, J. Wolf, A. H. Jacobs, R. K. Thomas, and A. Winkeler. 2008. Early detection of erlotinib treatment response in NSCLC by 3’-deoxy-3’-[F]-fluoro-L-thymidine ([F]FLT) positron emission tomography (PET). PLoS One 3(12):e3908.
van Aalst-Cohen, E. S., A. C. Jansen, S. de Jongh, P. R. de Sauvage Nolting, and J. J. Kastelein. 2004. Clinical, diagnostic, and therapeutic aspects of familial hypercholesterolemia. Seminars in Vascular Medicine 4(1):31–41.
Van Beers, B. E., and V. Vilgrain. 2008. Biomarkers in abdominal imaging. Abdominal Imaging 34(6):663–667.
Van den Berg, C. W., and K. E. Taylor. 2005. Letter in response to Bisoendial et al.: “Activation of inflammation and coagulation after infusion of C-reactive protein in humans.” Circulation Research 97(1):e2.
Verdecchia, P., G. Reboldi, F. Angeli, R. Gattobigio, C. Borgioni, L. Filippucci, F. Poeta, and C. Porcellati. 2004. Prognositc value of lipoprotein fractions in essential hypertension. Blood Pressure 13(5):295–303.
Verschuren, W. M., D. R. Jacobs, B. P. Bloemberg, D. Kromhout, A. Menotti, C. Aravanis, H. Blackburn, R. Buzina, A. S. Dontas, F. Fidanza, M. J. Karvonen, S. Nedeljkovic, A. Nissinen, and H. Toshima. 1995. Serum total cholesterol and long-term coronary heart disease mortality in different cultures: Twenty-five-year follow-up of the seven countries study. Journal of the American Medical Association 274(2):131–136.
Virella, G., and M. F. Lopes-Virella. 2008. Atherogenesis and the humoral immune response to modified lipoproteins. Atherosclerosis 200(2):239–246.
Vokurka, E. A., A. Herwadkar, N. A. Thacker, R. T. Ramsden, and A. Jackson. 2002. Using Bayesian tissue classification to improve the accuracy of vestibular schwannoma volume and growth measurement. American Journal of Neuroradiology 23(3):459-467.
Wahl, R. L., H. Jacene, Y. Kasamon, and M. A. Lodge. 2009. From RECIST to PERCIST: Evolving considerations for PET Response Criteria in Solid Tumors. Journal of Nuclear Medicine 50(Suppl 1):122S–150S.
Wallace, T. W., S. M. Abdullah, M. H. Drazner, S. R. Das, A. Khera, D. K. McGuire, F. Wians, M. S. Sabatine, D. A. Morrow, and J. A. de Lemos. 2006. Prevalence and determinants of troponin T elevation in the general population. Circulation 113(16):1958–1965.
Walter, M. 2009. Interrelationships among HDL metabolism, aging, and atherosclerosis. Arteriosclerosis, Thrombosis, and Vascular Biology 29(9):1244–1250.
Wang, T. J., P. Gona, M. G. Larson, G. H. Tofler, D. Levy, C. Newton-Chen, P. F. Jacque, N. Rifai, J. Selhub, S. J. Robins, E. J. Benjamin, R. D’Agostino, and S. V. Ramachamdan. 2006. Multiple biomarkers for the prediction of first major cardiovascular events and death. New England Journal of Medicine 355(25):2631–2639.
Watanabe, Y., T. Ito, and M. Shiomi. 1985. The effect of selective breeding on the development of coronary atherosclerosis in WHHL rabbits: An animal model for familial hypercholesterolemia. Atherosclerosis 56:71–79.
Weber, W. A. 2009. Assessing tumor response to therapy. Journal of Nuclear Medicine 50(Suppl 1):1S–10S.
Welsh, P., C. J. Packard, and N. Sattar. 2008. Novel antecedent plasma biomarkers of cardiovascular disease: Improved evaluation methods and comparator benchmarks raise the bar. Current Opinion in Lipidology 19(6):563–571.
Weverling-Rijnsburger, A. W. E., G. J. Blauw, A. M. Lagaay, D. L. Knook, A. E. Meinders, and R. G. J. Westendorp. 1997. Total cholesterol and risk of mortality in the oldest old. Lancet 350(9085):1119–1123.
Whicher, J. T. 1998. BCR/IFCC reference material for plasma proteins (CRM 470). Community Bureau of Reference. International Federation of Clinical Chemistry. Clinical Biochemistry 31(6):459–465.
Whitton, C. M., D. Sands, A. R. Hubbard, and P. J. Gaffney. 2000. A collaborative study to establish the 2nd International Standard for Fibrinogen, Plasma. Thrombosis and Haemostasis 84(2):258–262.
Williams, P. T., H. R. Superko, W. L. Haskell, E. L. Alderman, P. J. Blanche, L. G. Holl, and R. M. Krauss. 2003. Smallest LDL particles are most strongly related to coronary disease progression in men. Arteriosclerosis, Thrombosis, and Vascular Biology 23(2):314–321.
Wood, L. G., D. A. Fitzgerald, P. G. Gibson, D. M. Cooper, C. E. Collins, and M. L. Garg. 2001. Oxidative stress in cystic fibrosis: Dietary and metabolic factors. Journal of the American College of Nutrition 20(2):157–165.
Wu, A. H. B., and A. S. Jaffe. 2008. The clinical need for high-sensitivity cardiac troponin assays for acute coronary syndromes and the role for serial testing. American Heart Journal 155(2):208–214.
Yamada, S., T. Gotoh, Y. Nakashima, K. Kayaba, S. Ishikawa, N. Nago, Y. Nakamura, Y. Itoh, and E. Kajii. 2001. Distribution of serum C-reactive protein and its association with atherosclerotic risk factors in a Japanese population: Jichi Medical School Cohort Study. American Journal of Epidemiology 153(12):1183–1190.
Yang, G. C., J. D. Goldberg, and P. X. Ye. 2003. Risk of malignancy in follicular neoplasms without nuclear atypia: Statistical analysis of 397 thyroidectomies. Endocrine Practice 9(6):510-516.
Yaron, G., A. Brill, O. Dashevsky, I. M. Yosef-Levi, E. Grad, H. D. Danenberg, and D. Varon. 2006. C-reactive protein promotes platelet adhesion to endothelial cells: A potential pathway in atherothrombosis. British Journal of Haematology 134(4):426–431.
Young, H., R. Baum, U. Cremerius, K. Herholz, O. Hoekstra, A. A. Lammertsma, J. Pruim, and P. Price. 1999. Measurement of clinical and subclinical tumour response using [18F]-fluorodeoxyglucose and positron emission tomography: Review and 1999 EORTC recommendations. European Organization for Research and Treatment of Cancer (EORTC) PET Study Group. European Journal of Cancer 35(13):1773–1782.
Yu, H. H., R. Joubran, M. Asmi, T. Law, A. Spencer, M. Jouma, and N. Rifai. 2000. Agreement among four homocysteine assays and results in patients with coronary atherosclerosis and controls. Clinical Chemistry 46(2):258–264.
Zacho, J., A. Tybjaerg-Hansen, J. S. Jensen, P. Grande, H. Sillesen, and B. G. Nordestgaard. 2008. Genetically elevated C-reactive protein and ischemic vascular disease. New England Journal of Medicine 359(1897–1908).
Zaninotto, M., M. M. Mion, E. Novello, S. Altinier, and M. Plebani. 2007. New biochemical markers: From bench to bedside. Clinica Chimica Acta 381(1):14–20.
Zethelius, B., N. Johnston, and P. Venge. 2006. Troponin I as a predictor of coronary heart disease and mortality in 70-year-old men: A community-based cohort study. Circulation 113(8):1071–1078.
Zhang, Y. X., W. K. Cliff, G. I. Schoefl, and G. Higgins. 1999. Coronary C-reactive protein distribution: Its relation to development of atherosclerosis. Atherosclerosis 145(2):375–379.