2
Review: Evaluating and Regulating Biomarker Use
INTRODUCTION
The context within which this study is set has developed from the contributions of various scientific fields, industries, and government bodies. From toxicology to cardiology, from the food industry to the drug industry, and from the Food and Drug Administration (FDA) to the federal courts, biomarkers and the scientific evidence needed to substantiate their use have been topics of discussion for several decades. Along with a brief review of biomarker evaluation methods and their uses, this chapter seeks to describe critical areas of background information so that readers from different fields can gain a more comprehensive understanding of the policy and regulatory issues with respect to biomarkers.
Methods for evaluation of biomarkers and surrogate endpoints have been reviewed successfully and systematically in the recent past (Lassere, 2008; Shi and Sargent, 2009). This chapter will direct the readers toward appropriate reviews, and it will discuss the evolution of thinking at the FDA—focusing on the Center for Food Safety and Applied Nutrition (CFSAN), in particular—regarding surrogate endpoints. It will also discuss the evolution in thinking in academic and industry communities, to a lesser extent. The contents of this chapter are as follows:
-
Use of biomarkers in areas as diverse as scientific research, medical practice, product development, and public health policy
-
Use of biomarkers as surrogate endpoints
-
Evaluation frameworks proposed from academia and industry
-
The broader context of biomarker and surrogate endpoint evaluation by the FDA, including the legal and regulatory basis for claims made on CFSAN-regulated products
Examples are included on blood pressure as a surrogate endpoint, HIV/AIDS drug development, arrhythmia suppression interventions, exercise tolerance in congestive heart failure, and kidney toxicity biomarkers.
SURVEY OF BIOMARKER USES
Biomarkers have a wide array of uses in a variety of fields. These fields include medicine, oral health, mental health, nutrition, environmental health, toxicology, developmental biology, and basic scientific research. They are used to study the safety and efficacy of interventions, develop understanding of the mechanisms of disease, make good decisions in clinical care, and guide the policies that impact public health. Table 2-1 gives a list of several categories of biomarker use.
For the uses in Table 2-1, any biomarker would need to be evaluated to ensure that data supporting the biomarker’s association with the disease or condition of interest and the analytical validation of the test are adequate for the proposed use. In situations, however, where biomarker data will not or is not yet anticipated to be submitted to the FDA for a regulatory purpose or used by professional societies or other groups for clinical practice guidelines or other decision-making processes impacting public health or the practice of medicine, this may be an informal process. Ideally, evaluations are already done by clinicians, product developers, government regulators, professional societies, and scientists; this report’s contribution is to propose a systematic process for biomarker evaluation.
Use of Biomarkers and Surrogate Endpoints for Clinical Efficacy Studies and Formation of Clinical Practice Guidelines
Surrogate endpoints were defined in Chapter 1 and can be found in several locations in Table 2-1. First, they have been used in approvals of products or claims for drugs, biologics, devices, foods, and supplements. This will be discussed further in several subsections of this chapter’s section on evolution of regulatory perspectives on surrogate endpoints and in Chapter 5. Second, they have been used in the formulation of clinical practice guidelines. As defined by an Institute of Medicine (IOM) committee in 1990, “practice guidelines are systematically developed statements to assist practitioner and patient decisions about appropriate health care
TABLE 2-1 Categories of Biomarker Use
Use |
Description |
Discovery |
Identification of biochemical, image, or other biomarkers associated with a disease, condition, or behavior of interest; biomarkers identified may be screened for many potential uses, including as a target for intervention to prevent, treat, or mitigate a disease or condition |
Early product development |
Biomarkers used for target validation, compound screening, pharmacodynamic assays, safety assessments, and subject selection for clinical trials, and as endpoints in early clinical screening (i.e., phase I and II trials) |
Surrogate endpoints for claim and product approvals |
Biomarkers used for phase III clinical testing and biomarkers used to substantiate claims for product marketing |
Clinical endpoints |
Biomarkers used as endpoints for clinical trials that measure how a patient feels, functions, or survives; for example, measures of depression, blindness, and muscle weakness are biomarkers that may be used as clinical endpoints |
Clinical practice |
Biomarkers used by clinicians for uses such as risk stratification, disease prevention, screening, diagnosis, prognosis, therapeutic monitoring, and posttreatment surveillance |
Clinical practice guidelines |
Biomarkers used to make generalized recommendations for healthcare practitioners in the areas of risk stratification, disease prevention, treatment, behavior/lifestyle modifications, and more |
Comparative efficacy and safety |
Biomarkers used in clinical studies looking at the relative efficacy, safety, and cost effectiveness of any or all interventions used for a particular disease or condition, including changes in behavior, nutrition, or lifestyle; these studies are a component of comparative effectiveness research |
Public health practice |
Biomarkers used to track public health status and make recommendations for prevention, mitigation, and treatment of diseases and conditions at the population level |
for specific clinical circumstances” (IOM, 1990). Clinical practice guidelines and the systematic reviews that inform them are the subjects for two current IOM studies;1 the reports are expected in 2011. A guideline regarding treatment of a particular disease may identify target levels for specific biomarkers. In order to arrive at a recommendation for a particular biomarker level, clinical trial and observational data must be evaluated. It is possible that more trials will measure a particular surrogate endpoint in addition to or rather than the clinical endpoint of interest. In these cases, it may be desirable to include data from trials that did not measure the clinical endpoints of interest in the systematic reviews.
It is useful to mention that professional societies play an essential role in helping stakeholders understand the best ways to use biomarker-related information in clinical practice. One way in which professional societies assist in the understanding and use of biomarker data is through the promulgation of clinical practice guidelines. The committee recognized that clinical practice guidelines could use the committee’s proposed biomarker evaluation framework in reaching decisions. Other methods of rigorous, systematic review, including the Cochrane Collaboration, may also be valuable in assessing the evidence associated with clinical practice guidelines. One consideration that bodies involved in the work of determining the best clinical practice guideline may need to make is that of cost effectiveness. The committee viewed this topic as being beyond the statement of task for this study and well studied elsewhere, but the committee recognizes that comparisons of interventions looking at the number of quality-adjusted life-years gained through use of an intervention or relative to no intervention are useful.
The IOM recently released a report, Initial National Priorities for Comparative Effectiveness Research (IOM, 2009c), which identified six characteristics of comparative effectiveness research, or CER (Box 2-1). In general, use of surrogate endpoints in CER would not fulfill the fourth characteristic of comparative effectiveness research, as identified in the report (IOM, 2009c). Quoted below is the report’s description of this characteristic of CER:
CER measures outcomes—both benefits and harms—that are important to patients.
The committee is using the term “effectiveness” in reference to the extent to which a specific intervention, procedure, regimen, or service does what it is intended to do when used under real-world circumstances.
1 |
Standards for Developing Trustworthy Clinical Practice Guidelines (http://www8.nationalacademies.org/cp/projectview.aspx?key=49125) and Standards for Systematic Reviews of Clinical Effectiveness Research (http://www8.nationalacademies.org/cp/projectview.aspx?key=49124). |
BOX 2-1 Characteristics of Comparative Effectiveness Research (CER)
SOURCE: IOM (2009c). |
This can be contrasted with “efficacy,” which is the extent to which an intervention produces a beneficial result under controlled conditions (Cochrane, 1971; Higgins and Green, 2008). This implies an important distinction between much clinical research and CER, in that CER places high value on external validity, or the ability to generalize results to real-world decision making. Harms or risks of unintended consequences are also outcomes of interest, because they influence the net benefits of an intervention. Including and giving weight to patient-reported outcomes is particularly important for CER studies in which patient ratings of effectiveness or adverse events may differ from clinical measures. Finally, resource utilization may be highly relevant to net benefits when comparing the full clinical course of interventions over time. Cost-effectiveness analysis is a useful tool of CER, allowing evaluation of the full range of
treatment outcomes in relationship to the difference in costs. Robust evidence of comparative clinical effectiveness is a building block necessary for resource allocation decisions. Moreover, just as clinical effects may vary in different settings, costs vary as well, so a given set of cost-effectiveness results is often not generalizable. (IOM, 2009c)
Comparative effectiveness research is meant to fill gaps in evidence that prevent comparison of available treatments (IOM, 2009c) with a focus on outcome measurements that are tangible to the person rather than biomarkers or putative surrogate endpoints. Occasionally, it may be impractical for many of these studies to examine clinical endpoints; careful selection of surrogate endpoints after significant interaction with patient groups and expert investigators would be necessary. Finally, surrogate endpoints can be found in public health practice when there is a need to estimate the health of populations or short-term impacts of longer-term programs for prevention, treatment, or mitigation of infectious or chronic diseases when health outcomes important to patients cannot be measured. For example, reporting to stakeholders about interventions to decrease diseases and conditions of importance in the population, such as stroke or heart attack, may be done by measuring and reporting blood pressure as a surrogate for the desired improvement in health status, although measuring health outcomes important to patients such as stroke or quality of life would be preferable as guidance to public health interventions unless such measures were deemed impractical.
Surrogate Endpoints: Successes
The most widely discussed use of surrogate endpoints is in phase III clinical studies used to support applications for new drugs, biologics, and devices and to support claims on foods and supplements. In his presentation to the committee during its April public workshop, Dr. Robert Temple of the Center for Drug Evaluation and Research (CDER) at the FDA outlined the reasons why researchers and clinicians use surrogate endpoints (Temple, 2009).
These reasons include when the clinical endpoint is rare or takes years to develop; when the surrogate endpoints seem to be obviously linked to the clinical endpoint of interest (e.g., tumor size in cancer or maintenance of regular heart rhythm in arrhythmia patients); and when other treatments exist, to alleviate the difficulties of conducting trials when a new intervention must be proven as non-inferior to existing treatments. In addition, although it may be possible to use a clinical endpoint in a population at high risk for the disease or condition, studying a population at relatively lower risk using the clinical endpoint may be too burdensome
since the number of subjects required would be very large. Dr. Temple noted that the idea of a surrogate endpoint is to enable faster, smaller, more efficient clinical trials that can address urgent needs and facilitate the advancement of medicine.
Two notable successes of the use of surrogate endpoints are discussed in the next sections: blood pressure and HIV-1 RNA. The first example details the history of the evaluation of blood pressure as a surrogate endpoint. It may be surprising to readers that blood pressure as a surrogate endpoint for cardiovascular disease endpoints was hotly debated for decades before reaching its current status. Still, there is no broad agreement that blood pressure is a universal surrogate endpoint (Carter, 2002; Psaty et al., 1996). Even though these examples describe successful use of surrogate endpoints, important caveats are also described. Dr. Temple and others have noted surprises and mistakes in the selection and use of surrogate endpoints, and so several examples of these are discussed after the sections on blood pressure and HIV-1 RNA.
Blood Pressure
Blood pressure is often looked to as an exemplar surrogate endpoint for cardiovascular mortality and morbidity due to the levels and types of evidence that support its use. More than 75 antihypertensive agents in more than 9 therapeutic classes demonstrate the wide availability of agents to treat hypertension (Israili et al., 2007). Although new antihypertensive drugs are approved on the basis of blood pressure reductions, blood pressure’s history as a surrogate endpoint is unusual in that many drugs used to treat hypertension (thiazides, methyldopa, reserpine, hydralazine, guanethidine) were approved prior to the FDA’s effectiveness requirement or the availability of clinical trial data supporting the impact of blood pressure control on cardiovascular outcomes (Desai et al., 2006).
The status of blood pressure as a surrogate endpoint for cardiovascular disease endpoints was debated for decades (Perry et al., 1978). Even as one of the most well-established surrogate endpoints, an effect on blood pressure may not fully capture the benefit—or risk—of an intervention.
Although some issues are still outstanding, the benefits of blood pressure control are mostly well understood due to comprehensive epidemiologic and clinical trial evidence. Hypertension has been identified as the most common risk biomarker for cardiovascular morbidity and mortality, with a World Health Organization report suggesting that hypertension is the single most important preventable cause of premature death in developed countries (Ezzati et al., 2002). Data suggest that in the United States, hypertension is responsible for 35 percent of myocardial infarctions
and strokes, 49 percent of episodes of heart failure, and 24 percent of premature deaths (Wolff and Miller, 2007). Hypertension affects one in four U.S. adults, but the majority of those affected remain either untreated or undertreated in spite of the substantial health benefits gained from modest blood pressure reductions (Wang and Vasan, 2005).
Epidemiological, clinical trial data Williams (2005) suggested that the blood pressure–cardiovascular outcomes relationship is substantiated by one of the strongest evidence bases in clinical medicine. Epidemiologic studies consistently demonstrate the relationship between blood pressure and cardiovascular mortality and morbidity, including one meta-analysis of nine studies that demonstrated an association between diastolic blood pressure and coronary heart disease and stroke in 420,000 subjects (MacMahon et al., 1990). Observational studies have also demonstrated the robustness of blood pressure’s relationship to heart disease in adults; despite different assessment parameters (systolic alone, diastolic alone, or systolic and diastolic), the relationship is maintained (Desai et al., 2006). This relationship has also been confirmed in diverse populations, including different genders, adult age groups, and race/ethnicities. In children, this relationship does not hold (Brady and Feld, 2009).
Both placebo- and active-controlled clinical trials conducted in the past three to four decades have demonstrated that pharmacologic reductions in blood pressure reduce cardiovascular mortality and morbidity (Desai et al., 2006). While earlier trials compared hypertension agents against placebo, the growing evidence base supporting the benefit of hypertension therapy necessitated head-to-head trials comparing two or more agents, which reduced power of the studies and required much larger numbers of patients to see an effect (Williams, 2005). Many different therapeutic agents—including diuretics, beta blockers, angiotension converting enzyme (ACE) inhibitors, calcium channel blockers, and angiotensin receptor blockers—are approved to lower blood pressure.
Effects of blood pressure-lowering drugs Impact on blood pressure may or may not capture an intervention’s entire risk–benefit balance. Different classes of agents, or even agents within a specific class, may have multiple effects, one of which is lowering blood pressure (NHLBI Working Group, 2005). For example, ACE inhibitors are known to have at least 10 pharmacologic effects (Borer, 2004). This notion has generated trials testing whether agents have beneficial effects that go beyond blood pressure lowering. ALLHAT (Antihypertensive and Lipid Lowering Treatment to Prevent Heart Attack Trial) compared the efficacy of four different drug classes (a calcium channel blocker, an ACE inhibitor, an alpha adrenergic blocker, and a diuretic) for initial therapy of hypertension. Study results
demonstrated that three classes of drugs (calcium channel blocker, ACE inhibitor, and diuretic) could not be distinguished for the primary endpoint, coronary heart disease (CHD) mortality and non-fatal myocardial infarction, but the lower cost diuretics were superior in regard to secondary outcomes and should be the preferred first step therapy (ALLHAT Officers and Coordinators, 2002). The alpha adrenergic blocker arm of the trial was dropped because of the significantly higher incidence of combined cardiovascular events in the alpha adrenergic blocker arm compared to the diuretic, including a two-fold relative risk of congestive heart failure compared to the diuretic (ALLHAT Officers and Coordinators, 2000).
Other conclusions have also been drawn from these large, prospective head-to-head comparison trials; some investigators suggest that it is the blood pressure reduction, rather than the specific drug used, that confers cardiovascular benefit (Williams, 2005). In an analysis of 147 randomized trials, investigators found that all classes of blood pressure-lowering drugs have similar effects in reducing coronary heart disease events and strokes for a given level of blood pressure reduction, with the exception of an extra protective effect of beta blockers administered shortly after myocardial infarction and minor protective effect of calcium channel blockers in stroke (Law and Morris, 2009). Although there is still some ambiguity about the use of differing blood pressure agents, the fact that pharmacologically distinct agents have directionally similar effects on cardiovascular outcomes has provided more support for the use of blood pressure as a surrogate endpoint for coronary heart disease and stroke.
Regulatory use of blood pressure as a surrogate endpoint The consistent demonstration that diverse blood pressure-lowering agents confer cardiovascular benefits, as well as the substantial epidemiological data linking hypertension to cardiovascular events, provides the basis for the FDA’s use of blood pressure as a surrogate endpoint (Desai et al., 2006; Temple, 1999). However, clear guidance on the use of surrogate endpoints within the FDA is lacking because the Food, Drug, and Cosmetic Act does not specifically state which endpoints—or criteria—can be used for drug approval. Through case law, the FDA has the authority to deny approval of a drug on the basis of its effect on the surrogate endpoint if the surrogate endpoint’s clinical value is unknown.2 In 1992, FDA regulation provided a new method for drug approval on the basis of effects on a surrogate endpoint, called accelerated approval, for serious or life-threatening conditions without available therapy. The regulation stated that drugs could be approved on the basis of surrogate endpoint data if it “is reasonably
likely, based on epidemiologic, therapeutic, pathophysiologic, or other evidence, to predict clinical benefit”3 and required confirmatory clinical evidence. The regulation also referenced “well-established” surrogates on which drug approval had been based, but did not define well-established endpoints. Temple (1999) noted that “well-established” surrogates would need to be more than “reasonably likely” to predict benefit.
Despite the lack of clarity in the regulations concerning surrogate endpoints, the FDA accepts surrogate endpoints for drug approval and as the basis for authorized health claims. However, different divisions and centers within the FDA accept different surrogate endpoints. For example, the Cardio-Renal Division within the CDER accepts blood pressure reduction as a surrogate endpoint for cardiovascular event reduction, but requires direct clinical benefit measurement for other endpoints, while the Metabolic-Endocrine Division also accepts LDL-C lowering as a surrogate endpoint for cardiovascular events (Borer, 2004). The Metabolic-Endocrine Division also accepts use of glycosylated hemoglobin level and blood glucose control as surrogate endpoints for diabetes control (Borer, 2004). Even so, the FDA has recognized the inadequacy of small six-month trials that address effects of type 2 diabetes mellitus treatments on HbA1c, and now the FDA requires large-scale randomized cardiovascular safety clinical endpoint trials be conducted pre- and post-approval.
Within CFSAN, blood pressure is recognized as a surrogate endpoint for hypertension (FDA, 1999). Hypertension is considered a disease-related health condition. As discussed earlier, hypertension—high blood pressure—is recognized as a strong risk factor for cardiovascular disease. CFSAN has authorized a health claim for low-sodium foods based on the surrogate endpoint–disease-related condition relationship, stating either “diets low in sodium may reduce the risk of high blood pressure, a disease associated with many factors” or “development of hypertension or high blood pressure depends on many factors. [This product] can be part of a low sodium, low salt diet that might reduce the risk of hypertension or high blood pressure.”4
HIV Drug Development
One of the motivations for the earliest efforts at surrogate endpoint evaluation arose from the acute need for effective therapeutics early in the HIV/AIDS epidemic. The early trials of anti-HIV therapies used progression to AIDS or death as the clinical outcome measures. These studies could be short in some settings, like those in which the effects of the
intervention were large and participants had advanced disease (Fischl et al., 1987; Hammer et al., 1997). Studies could also be short when they were large enough so that only a small percentage of patients who progress to advanced disease drove the principal finding (Volberding et al., 1994). However, the latter type of study could produce misleading results in that a small number of patients destined to progress quickly might benefit from an intervention, like AZT monotherapy, while an even larger number might experience no benefit and even positive harm following the conclusion of the study, because of factors like the development of resistance to the drug under study and others with similar mechanisms of action. Such concerns underscored the need for a more rapid means of evaluating the benefit of antiviral therapy that might reflect risk or benefit to a larger proportion of the study population more rapidly.
Early in the AIDS epidemic, it was observed that clinical disease progression was associated with a decline of CD4+ T-lymphocytes (CD4 cells); in the 1990s, a virologic measure that both responded to therapy and predicted outcomes was developed (HIV-1 RNA). The earliest approval of a drug based on a biomarker—didanosine was approved in 1991—used CD4 cell count; however, the development of measurement of plasma HIV-1 RNA by polymerase chain reaction (PCR), which made a direct measurement of viral replication possible, rapidly became the standard endpoint in HIV clinical trials. In the mid-1990s, representatives from industry, drug regulatory agencies, and academia sought to formally evaluate CD4 cell count and HIV-1 RNA as surrogate endpoints for disease progression in clinical trials and in patient management (Hughes et al., 1998).
To evaluate HIV-1 RNA and CD4 cell count as surrogate endpoints, the HIV Surrogate Marker Collaborative Group, a group involving statisticians and clinicians from pharmaceutical companies and government-funded cooperative clinical trials groups, was formed. The HIV Surrogate Marker Collaborative Group undertook a meta-analysis of clinical trials to evaluate treatment-mediated changes in HIV-1 RNA and CD4 cell count as surrogate endpoints (HIV Surrogate Marker Collaborative Group, 2000). The meta-analysis found that HIV-1 RNA and CD4 cell count have independent value as prognostic biomarkers. However, the meta-analysis also found that short-term changes in the values of these biomarkers were not adequate surrogate endpoints for determining the impact of an intervention on long-term clinical endpoints such as progression to AIDS and death (HIV Surrogate Marker Collaborative Group, 2000). Their analysis also showed that changes in HIV-1 RNA explained only about half of the benefit of treatment. However, these results mostly reflected the experience of patients on drug regimens that were not capable of suppressing most patients’ viral loads below levels of assay detection.
In 2002, the FDA issued a guidance for industry that advocated the use of HIV-1 RNA in plasma as the primary basis for assessing efficacy of antiretroviral drugs for accelerated and traditional approval, although it had begun approving drugs based on evidence of lower levels of plasma HIV-1 RNA a few years earlier (Behrman, 1999). Additionally, it recommended that “changes in CD4 cell counts be consistent with observed HIV-1 RNA changes when considering approval of an antiretroviral drug” (FDA, 2002). In most cases, approval was based on demonstrations that new drugs, used in combination with existing drugs, were able to suppress virus among patients who had not been previously exposed to therapy and had virus that was sensitive to at least one other agent in the regimen. An important distinction must be made between using HIV-1 RNA as a surrogate for a clinical endpoint in a setting where virus can be fully suppressed and a setting where virus is only partly, and often therefore temporarily, suppressed. Complete viral suppression often leads to durable suppression, perhaps because of the lower risk of development of viral resistance mutations in patients without replicating virus. Tolerable drugs that produce durable suppression are likely to benefit patients because such suppression is associated with steady improvements in CD4 and reduced risk of clinical events associated with HIV infection.
The value of HIV-1 RNA as a surrogate in settings where suppression of HIV-1 RNA is partial is much more problematic and contingent on context, because partial HIV suppression invites development of new drug resistance mutations that limit the future usefulness of the drugs under study and similar drugs. Therefore a drug that induces a temporary reduction in HIV-1 RNA, while perhaps valuable in reducing risk of clinical disease over a short interval, may reduce the possibility of later construction of a durable three-drug regimen. Such loss of future drug options is an important consequence of drug treatment that is not captured by plasma HIV-1 RNA levels (Jiang et al., 2003). Another important factor is viral fitness, which is affected by treatment and may also be relevant for long-term outcomes (Deeks and Martin, 2007).
As a consequence the use of HIV-1 RNA as a surrogate for clinical endpoint in settings where viral suppression is not complete has not been supported with evidence and probably cannot be. As mentioned above, the relative benefit of different degrees of partial HIV suppression are highly context specific and dependent on the availability of other drugs. De Gruttola et al. (2006), in a discussion of the approval of tipranavir in exactly such a context, recommended that only complete suppression of plasma HIV-1 RNA be used in such studies, and that partial suppression endpoints not be used in clinical trials.
Historically, it is important to note that the FDA’s guidance to industry occurred prior to the approval of newer types of antiretroviral drugs
that use different mechanisms than those formally evaluated in the meta-analysis (Hughes, 2005). More potent antiretroviral drugs, which can fully suppress HIV-1 viral load, have since become standard of care. This suggests that although HIV-1 RNA has become the primary endpoint to determine efficacy in many antiretroviral trials, collection of additional and longer term information that relates to both risk and benefit—especially in studies of newer types of antiretroviral drugs—is warranted.
In conclusion, the rapid development of HIV drugs in the 1990s was enabled through the use of surrogate endpoints. While this use of surrogate endpoints inspired the creation of the Critical Path Initiative, the process of biomarker evaluation used was not systematic and so was not easily translated into other disease areas. Nonetheless, the success of this effort to speed approvals of HIV drugs highlighted the value that a systematic biomarker evaluation process could have for drug regulation in general.
Cautionary Statements Regarding the Use of Surrogate Endpoints
Remarkably, the cautionary voices speaking about the risks of using surrogate endpoints have been repeating the same messages for 20 years. What has been changing is the continually increasing amount of data supporting their arguments. In 1989, Ross Prentice initiated the conversation about surrogate endpoints with his influential paper, which provided a statistical definition of a surrogate endpoint. In this paper, he wrote, “I am somewhat pessimistic concerning the potential of the surrogate endpoint concept” (Prentice, 1989). This statement was made in acknowledgment of the hope, already palpable, that a surrogate endpoint, once shown useful for one intervention, would be extensible to other interventions and that relative reductions in one risk factor would be comparable to others for a given clinical endpoint.
Editorials in the early 1990s looked at the rapid advances—and mistakes—enabled through use of surrogate endpoints at the beginning of the HIV/AIDS epidemic (Cotton, 1991; De Gruttola et al., 1997; Holden, 1993; Lagakos and Hoth, 1992). The potential benefits and hazards of the use of surrogate endpoints have been understood since the beginning of this discussion. In 1991, Cotton noted several standing questions in relation to use of surrogate endpoints in the treatment of HIV/AIDS. Due to contemporaneous failures of surrogate endpoints in cardiology trials, researchers were wary when they did not understand the role a surrogate played in disease pathogenesis and progression. They noted that the role and importance of a biomarker may change over the course of a disease, such that extension of results in a population with more advanced disease may not translate to a population with less advanced disease and vice
versa. Finally, researchers were not confident in the analytical validation of the tests being used to measure the surrogate endpoints (Cotton, 1991). In 1992, Lagakos and Hoth noted that experience from use of CD4 cell count as a surrogate endpoint in HIV/AIDS trials led to the idea that “it seems unrealistic to expect that any single marker can fully explain all of a drug’s clinical effects.” Furthermore, they recommended that “we cannot confidently abandon clinical endpoints as the basis for judging efficacy in these large trials…. It is therefore important that we continue to conduct comparative efficacy trials that collect data on both clinical outcomes and surrogate markers to establish CD4 count or other markers as valid surrogates for clinical effect” (Lagakos and Hoth, 1992). In 1993, Holden noted the desire of some to obtain a list of preapproved surrogate endpoints has been worrying to regulators because of the relevance of a biomarker’s context of use in every application. In the article, Holden summarized a statement of Sidney Wolfe of the Public Citizen Health Research Group, saying that “drug companies could abuse [approvals of surrogate endpoints by the FDA] by failing to do careful clinical trials once they get a marker approved…. If clinical trials don’t pan out, it might be very hard to ban the unapproved drug” that had been provisionally approved on the basis of the proposed surrogate endpoint (Holden, 1993).
Several of these warnings have been repeated since the early 1990s. Psaty et al. (1996) pointed out that different blood pressure-lowering interventions do not result in the same effects on clinical outcomes for a given reduction in blood pressure. De Gruttola et al. (1997) noted that unless disease mechanism of action is understood, uncertainty is inherent in the assumption that the surrogate can predict all of an intervention’s effect. Schatzkin and Gail (2002) discussed use of surrogate endpoints in cancer research in 2002; they again noted the difficult balance between strong evidence that a surrogate endpoint has predictive value for the clinical endpoint and use of surrogates to achieve new drug approvals before full clinical trials using clinical endpoints can be completed. In the same year, DeMets and Califf (2002) reviewed principles of cardiovascular research and focused on the important distinctions between putative surrogate endpoints and clinical endpoints, reviewing multiple cases in which naïve use of putative surrogates had endangered patients with cardiovascular disease. In these cases, therapies, including antiarrhythmic, heart failure, and antiatherosclerosis treatments that had been assumed to be beneficial based on putative surrogate endpoints were indeed detrimental to health when confirmatory trials were done, usually because of off-target effects of systemically administered drugs. Manns et al. (2006) cited problems with the use of surrogate endpoints in a 2006 editorial. They discussed the opportunity cost of making decisions about allocation of healthcare resources (monetary, professional, and tangible),
treatment decisions to use one treatment and forgo others, and allocation of research funding. The authors suggest that “it would seem prudent for [clinical practice guideline] developers to refrain from recommending the use of new agents until they have been proved to improve clinically meaningful outcomes” (Manns et al., 2006). Krumholz and Lee (2008) wrote in the New England Journal of Medicine that although use of surrogate endpoints can simplify the practice of medicine, it can do so at the cost of quality and outcomes. In 2009, Colatsky noted that surrogate endpoint biomarkers, low-density lipoprotein cholesterol (LDL-C) levels and carotid intima-media thickness (IMT) in this example, do not always correlate well with one another, making interpretation of trial results difficult (Colatsky, 2009).
These cautionary statements have gathered strength as some surrogate endpoints have failed. Examples of these failures and the reasons for their occurrence are discussed in the next section.
Failure of Surrogate Endpoints: Reasons and Examples
Putative surrogate endpoints often fail to predict clinical outcomes. In 1996, Fleming and DeMets published a paper explaining the failures in surrogate endpoints that had occurred mostly during the late 1980s and early 1990s (Fleming and DeMets, 1996). As described in Figure 2-1, according to Fleming and DeMets (1996), several factors explain the failure of surrogate endpoints: (1) the surrogate endpoint does not involve the same pathophysiologic process that results in the clinical outcome; (2) the intervention affects only one pathway mediated through the surrogate, of several possible causal pathways of the disease; (3) the surrogate is not part of the causal pathway of the intervention’s effect, or is insensitive to its effect; and (4) the intervention has mechanisms of action independent of the disease process. As noted in Figure 2-2, the most promising setting in which to qualify a surrogate endpoint occurs when the surrogate is on the only causal pathway of the disease process, and the intervention’s entire effect on the clinical outcome is mediated through its effect on the surrogate (Fleming and DeMets, 1996). However, even in the best of circumstances, it is possible for surrogate endpoints to be misleading by either overestimating or underestimating an intervention’s effect on clinical outcomes.
A number of biomarkers have been proposed as rational surrogate endpoints, but have failed to demonstrate usefulness for that purpose upon further scrutiny in clinical trials. One example was the use of beta-carotene and retinol as biomarkers for cancer, cardiovascular disease, and (later) cataract risk, and as interventions for chemoprevention of these diseases. Observational studies indicated that lower dietary intakes of
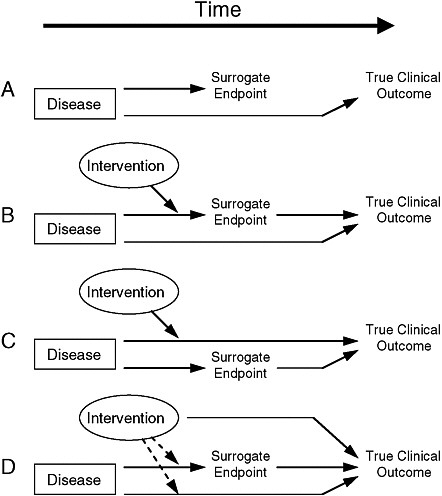
FIGURE 2-1 Reasons for failure of surrogate endpoints. (A) The surrogate is not in the causal pathway of the disease process. (B) Of several causal pathways of disease, the intervention affects only the pathway mediated through the surrogate. (C) The surrogate is not in the pathway of the intervention’s effect or is insensitive to its effect. (D) The intervention has mechanisms of action independent of the disease process. Dotted lines = mechanisms of action that might exist.
SOURCE: Fleming and DeMets (1996). Reprinted, with permission, from the Annals of Internal Medicine. Copyright 1996 by American College of Physicians.
beta-carotene and lower serum levels of beta-carotene were associated with greater risk of cancer. It is useful to note that while serum level of beta-carotene is a biomarker for adequate intake of the nutrient and a proposed surrogate endpoint for prevention of cancer and atherosclerotic disease, supplementation of the diet with beta-carotene is an intervention to either address deficiencies or conditions for which it is used as a sur-
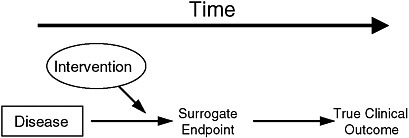
FIGURE 2-2 The setting that provides the greatest potential for the surrogate endpoint to be valid.
SOURCE: Fleming and DeMets (1996). Reprinted, with permission, from the Annals of Internal Medicine. Copyright 1996 by American College of Physicians.
rogate. Beta-carotene was shown to have in vitro antioxidant effects, and supplementing the diet with beta-carotene as a dietary supplement was expected to lower risk for atherosclerotic disease and cancer. However, its use in large population studies with mortality as the endpoint was not shown to lower risk for atherosclerosis or cancer; instead, it was shown to increase cancer incidence (Omenn et al., 1996; Peto et al., 1981). Betacarotene will be discussed further in Chapter 4.
In another example, elevated serum levels of homocysteine were found to be associated with greater risk for atherosclerotic disease in observational associations and serum homocysteine was thought to be a surrogate endpoint. Homocysteine can exacerbate endothelial dysfunction, thrombosis, and other risk mechanisms for atherosclerosis. Folic acid was shown to decrease levels of circulating homocysteine. Researchers were confident that cardiovascular endpoints of death and vascular morbidity would be reduced with the administration of folic acid supplements. During this period, the use of folic acid supplements was found to decrease fetal development of neural tube defects when administered to pregnant women, and grain products were fortified with folic acid in the United States and other countries. The incidence of neural tube defects decreased following fortification. However, atherosclerotic disease, either coronary heart disease or peripheral vascular disease, did not decrease following folic acid fortification or with the administration of folic acid supplements in several large clinical trials despite important decreases in serum homocysteine levels with both interventions (Clarke et al., 2007).
From these examples, it is apparent that without a detailed understanding of a biomarker’s role in the disease or treatment mechanism, biomarker evaluation can be difficult. The recent failure of some sur-
rogate endpoints to predict clinical outcomes has elicited concern over guidelines and performance measures used in clinical decision making. Traditionally, clinicians focus on reducing risk factors below certain levels to prevent disease; for example, clinical guidelines and performance measures “encourage treatment geared toward achieving ambitious goals for levels of glycated hemoglobin, lipids, and blood pressure” (Krumholz and Lee, 2008). In light of recent trials that demonstrate a reduction in a risk biomarker without a corresponding reduction in risk, Krumholz and Lee suggest a rethinking of risk factor reduction. Instead of focusing on just the amount a risk biomarker is reduced, clinicians should also be aware of the strategy involved in risk reduction. According to Krumholz and Lee (2008), “We are now beginning to appreciate that a strategy’s effect on a risk biomarker may not predict its effect on patient outcomes.” Since it is recognized that “[s]ome strategies are known to improve patient outcomes, whereas others are known to affect only risk-factor levels or other intermediate outcomes,” Krumholz and Lee believe that guidelines and performance measures should not specify targets without strategies used to achieve them. Additionally, practice guidelines and performance measurement should discuss risks of disease and adverse events in a more sophisticated and explicit way so that an assessment of net clinical benefit can be made (Krumholz and Lee, 2008).
As Krumholz and Lee (2008) pointed out, changes in surrogate endpoints do not always correspond with changes in clinical outcomes. Data from additional clinical trials have supplemented the notion that effects on proposed surrogate endpoints may fail to predict clinical outcomes. Nambi and Ballantyne (2007) emphasized that “we must use a great deal of caution before substituting a surrogate for a clinical endpoint” because the scientific community has been misled by biomarkers in the past. Patients and the credibility of science in the eyes of the public can be negatively impacted when the scientific community is misled by a biomarker. Fleming and DeMets (1996) further noted that “a review of recent experiences with surrogates is sobering, revealing many cases for which biological markers were correlates of clinical outcomes but failed to predict the effect of treatment on the clinical outcome.” The following examples related to cardiovascular disease (CVD)—arrhythmia suppression, exercise tolerance in congestive heart failure, and lowering lipids—were outlined by Fleming and DeMets as telling examples of failed surrogate endpoints.
Arrhythmia Suppression
As described by Fleming and DeMets (1996), an example of the failure of a surrogate endpoint to predict clinical outcomes is the reduction
of ventricular ectopic contractions for decreased cardiovascular mortality. When drugs were being developed and clinically tested, it was well known that compared to patients without ventricular arrhythmia, ventricular arrhythmia was independently associated with a significant increase in the risk of death related to cardiac complications, including sudden death (Bigger et al., 1984; Cardiac Arrhythmia Suppression Trial [CAST] Investigators, 1989; Echt et al., 1991; Mukharji et al., 1984; Ruberman et al., 1977). Researchers hypothesized that suppression of ventricular arrhythmias after myocardial infarction would reduce the rate of death. Scientists were so confident in this hypothesis that three drugs were approved by the FDA—encainide, flecainide, and moricizine—using arrhythmia suppression as the surrogate endpoint in phase III clinical trials. To illustrate the confidence scientists had in arrhythmia suppression as a surrogate endpoint, many of them believed that randomizing patients to either one of the study drugs or a placebo would be unethical. After approvals based on positive echocardiogram data, a feasibility trial was first conducted to determine whether a placebo-controlled trial would be safe enough to undertake (Cardiac Arrhythmia Pilot Study [CAPS] Investigators, 1986, 1988; CAST Investigators, 1989; Emanuel and Miller, 2001; Ruskin, 1989). After approval, more than 200,000 people eventually took these drugs each year, despite the lack of data evaluating the reduction of arrhythmias on mortality rates. The Cardiac Arrhythmia Suppression Trial (CAST) was designed to assess the drugs’ impact on survival for patients who had had myocardial infarction and at least 10 premature beats per hour. Both the encainide and flecainide arms of the trial were terminated early when 33 sudden deaths occurred, as compared to only 9 in the matching placebo group. In total, 56 patients in the encainide and flecainide groups died, compared to 22 patients in the placebo group. Later data confirmed that patients taking moricizine were also at increased risk for death (Fleming and DeMets, 1996).
In addition to the CAST study, two other examples of failed surrogate endpoints have occurred with arrhythmia treatment. Quinidine had been used for many years to restore and maintain sinus rhythm in patients with atrial fibrillation. However, a meta-analysis indicated that quinidine increased the mortality rate from 0.8 percent to 2.9 percent, which out-weighed the benefit of maintaining sinus rhythm (Fleming and DeMets, 1996). According to Lesko and Atkinson (2001), “unanticipated adverse consequences of drug therapy are a frequent confounding factor when biomarkers [such as maintaining normal sinus rhythm] are relied on as surrogates for definitive endpoints.” Ventricular tachycardia, in the case of lidocaine drug therapy, was also shown to be an inadequate surrogate endpoint. Although a meta-analysis indicated lidocaine therapy produced a one-third reduction in the risk of ventricular tachycardia, it was also
accompanied by a one-third increase in death rate (Fleming and DeMets, 1996). The failure of surrogate endpoints (e.g., maintenance of normal sinus rhythm and reduction of risk of ventricular tachycardia) to predict clinical endpoints “underlies much of the controversy surrounding the use of surrogate endpoints as the basis for regulatory evaluation of new therapeutic entities” (Lesko and Atkinson, 2001).
Exercise Tolerance in Congestive Heart Failure
Decreased cardiac output, decreased exercise capacity, and high risk of death are conditions associated with congestive heart failure, noted Fleming and DeMets (1996). Heart failure is a leading problem in cardiology; for example, 12 percent of a cohort of individuals age 65 or over were found to have symptomatic heart failure (Afzal et al., 2007). Heart failure patients may experience shortness of breath, congestion in the lungs, difficulty exercising, swelling in the legs, and quality-of-life–reducing effects. During the time leading up to the Prospective Milrinone Survival Evaluation (PROMISE) trial, cardiac output and ejection fraction had been used as surrogate endpoints, while exercise tolerance and symptomatic improvement had been used as intermediate endpoints. The PROMISE trial was requested by the FDA, which was concerned about long-term adverse effects of milrinone (Fleming and DeMets, 1996). Milrinone, a drug that was used to treat congestive heart failure, was shown to increase total mortality in the PROMISE trial, even though earlier studies demonstrated milrinone’s effectiveness in improving cardiac output and increasing exercise tolerance. The drug flosequinan, a vasodilator that reduces cardiac workload, was also conditionally approved by the FDA to treat congestive heart failure in patients who did not respond to or tolerate other drugs. However, the Prospective Flosequinan Longevity Evaluation (PROFILE) trial demonstrated that flosequinan increased total mortality, even though it improved exercise tolerance. According to Fleming and DeMets (1996), “[a]lthough cardiac output, ejection fraction, and exercise tolerance are correlated with longer survival of patients with congestive heart failure, a treatment-induced improvement in those measurements is not a reliable predictor of the effect of treatment on mortality rates.”
EVALUATION FRAMEWORKS
Biomarkers differ in their contexts of use and thus in the types of evidence needed for evaluation. Furthermore, use of surrogate endpoints for collection of evidence in support of policy or regulatory decisions is subject to the challenges and risks discussed in the previous sections (see Figures 2-1 and 2-2 and associated discussion). For additional detail, see Figure 2 in the paper by Boissel et al. (1992), outlining an approach for
selection of surrogate endpoints. As each of these figures illustrate, the evaluation of a biomarker as a surrogate endpoint is particularly challenging because of the biological complexity of human disease and response to drugs and nutrients. Neither correlation of the biomarker with clinical outcome nor biological plausibility is sufficient to establish the usefulness of a biomarker as a surrogate endpoint. Moreover, qualification of a biomarker for a particular disease or treatment does not necessarily translate to qualification for related uses or even for an essentially identical use at a different point of time (and thus a different context of use).
Several frameworks for biomarker qualification and several for biomarker assay validation have been published. Appendix A presents a time line of critical developments in the discussion about biomarker and surrogate endpoints evaluation, republished with permission from the 2008 review in Statistical Methods in Medicine by Lassere. Terminology is presented as it was by Lassere, which was consistent with the original publications. Since 2007, there have been a few important publications, which have also been tabulated in Appendix A.
The next section discusses the evolution of thought on association and causation between exposure to a pathogenic agent, biomarkers, and incidence and mortality from disease. Several examples of the evaluation and use of surrogate endpoints in drug development are then discussed. The last two sections address the two main directions in the discussion of biomarker evaluation: those focusing on statistical methods and those focusing on qualitative methods. The reason is that while it is straight-forward to establish a statistical association, it is difficult to definitively establish causality. Qualitative criteria have been used to fill this gap in the quantitative methods. Furthermore, decisions sometimes must be made when sufficient data are not available to make a quantitative analysis, and so qualitative methods are used.
Biomarker–Clinical Endpoint Relationships: Association Versus Causation
Many students of biology and epidemiology are familiar with Koch’s postulates for determining the cause of infectious diseases. These postulates state that in order to conclude that a particular infectious agent is the cause of a disease, the following conditions must be fulfilled:
-
The agent must be associated with all cases of the disease;
-
The agent must be isolable and cultured from a diseased organism;
-
The cultured agent must be able to infect a new host; and
-
The agent must be reisolable from the host in postulate 3.
These postulates were developed in the 1880s, and in the 1900s, scientists sought to establish causality in diseases that were not infectious, such as cancer. In a report outlining the evidence supporting a causal link between smoking and lung cancer, an advisory committee to the Surgeon General of the Public Health Service outlined five criteria for the case of non-infectious or chronic diseases: the strength, specificity, temporality, and consistency of the association (Advisory Committee to the Surgeon General, 1964). These criteria were refined when, in 1965, Sir Austin Bradford Hill discussed these criteria in a famous lecture to the section of occupational medicine of the UK’s Royal Society of Medicine (Hill, 1965). The criteria are now known as Hill’s criteria and are outlined in Box 2-2. Since the 1960s, these criteria have been used in environmental health, toxicology, pharmacology, epidemiology, and medicine.
Surrogate endpoints have been discussed for a little over 20 years. In 1989, Ross Prentice defined the term “surrogate endpoint” in his paper entitled “Surrogate endpoints in clinical trials: Definition and operational criteria” (Prentice, 1989). This paper was accompanied by three other papers in an issue of Statistics in Medicine exploring the possible use of biomarkers as surrogate endpoints, using examples from cancer (Ellenberg and Hamilton, 1989), cardiovascular disease (Wittes et al., 1989), and ophthalmologic disorders (Hillis and Seigel, 1989). As discussed briefly in the previous chapter, the Prentice criteria specify that a biomarker under consideration as a potential surrogate endpoint must correlate with the clinical outcome it is meant to replace and that the biomarker must capture the entire effect of the intervention on the clinical endpoint (Prentice, 1989). Further development of statistical methods has occurred since 1989, as statisticians search for methods to ease the burden of the second criterion (Fleming, 2005). These approaches include meta-analysis of data from multiple trials (Alonso et al., 2006; Burzykowski et al., 2004; Buyse and Molenberghs, 1998; Buyse et al., 2000; Hughes, 2002; Hughes et al., 1995) as well as addressing the following: (1) the proportion of treatment effect described by the surrogate endpoint; (2) the relative effect and adjusted association; and (3) the surrogate threshold effect. These methods are summarized in Lassere’s (2008) review, and several of them are discussed in detail in this chapter’s section on statistical approaches to biomarker evaluation.
Nonetheless, surrogate endpoints were used before these conversations began. One of the best examples of this is blood pressure, which is used as a surrogate endpoint for CVD clinical outcomes. Blood pressure represents the historical course of biomarker evaluation, gradual accumulation of data, and agreement among stakeholders on the utility of a biomarker, as described in the earlier section on the history of the evaluation of blood pressure as a surrogate endpoint.
BOX 2-2 Hill’s Criteria
SOURCE: Hill (1965). |
HIV/AIDS drug development provides another historical example of the use of surrogate endpoints. On October 11, 1988, frustrated with the length of time-to-approval for new therapies to treat HIV infection, ACT-UP, an AIDS patient advocacy group, staged a demonstration in front of FDA headquarters. Eight days later, on October 19, Frank Young, then commissioner of the FDA, announced regulations by which review times would be shortened for drugs designed to treat “life-threatening or severely debilitating” diseases (Arno and Feiden, 1988; AVERT, 2009; FDA, 1988). For that reason, HIV/AIDS drugs were some of the first to be approved explicitly on the basis of surrogate endpoints, and served as the foundation for the laws on accelerated approval of drugs and biologics. HIV/AIDS was also the first example of a more systematic, prospective approach to biomarker evaluation, although its precedent was not easily translatable into general guidance.
Finally, after the early 1990s, much of the literature has focused on the use of surrogate endpoints to approve oncology drugs. There is a substantial literature in this area, which is discussed in relation to use of
tumor size as a surrogate endpoint for cancer treatment interventions in Chapter 4. Research and development in oncology has been working to implement broader use of biomarkers, but this effort continues with lack of a standard approach.
Statistical Approaches to Biomarker Evaluation
Although randomized clinical trials with clinically meaningful endpoints provide the most rigorous means of assessing benefit of an intervention, such trials may be lengthy and expensive, and not always feasible. Therefore considerable interest has been shown in development of a framework for “statistical validation” of surrogates for clinical endpoints that can reliably provide information more quickly and cheaply about medical interventions. While much work has been done in this area, there remains no widely accepted research paradigm for statistical validation, in the way that, for example, randomized clinical trials provide such a paradigm for comparing new to existing therapies. Below we describe why no single paradigm is likely to arise soon, or perhaps ever. We also show, however, that existing frameworks and methods are useful for investigating the properties of surrogate endpoints.
It is useful to restate Prentice’s influential definition of a statistically valid surrogate, which required that a test of the null hypothesis of no relationship of the surrogate endpoint to the treatment assignment must also be a statistically valid test of the corresponding null hypothesis based on the true endpoint (Prentice, 1989). Statistical validation was based on two conditions: (1) correlation of the surrogate with the true clinical endpoint; and (2) the ability of the surrogate to fully capture the treatment’s “net effect” on the clinical endpoint. As described by Fleming and DeMets (1996), the net effect is the aggregate effect accounting for all mechanisms of action of the intervention. Considerable effort has been made to assess the degree to which this second condition holds in a variety of settings, but such analyses are complicated by difficulty in reliably estimating the quantities of interest and in the need for extensive assumptions (see below).
An alternative approach is based on meta-analyses across studies. Daniels and Hughes (1997) used Bayesian methods to construct prediction intervals for the true difference in clinical outcome associated with a given estimated treatment effect on the potential surrogate. By “borrowing” information regarding estimates of the effects of treatment on the clinical endpoint, and on the relationships between the surrogate and the clinical endpoint given treatment from previous studies, one predicts effects of a new treatment from data on the surrogate.
An important recent paper by Joffe and Greene (2009) attempts to pro-
vide a broader intellectual framework, using ideas from causal inference, that subsumes several different approaches (including those described above) and also provides insight into why this research is so challenging. They describe four different frameworks for statistical validation of surrogacy, and show connections among them. The first is based on the Prentice criteria described above. A second considers the estimation of direct and indirect effects of treatment; the latter are those mediated through a biomarker. Joffe and Greene describe these two approaches as belonging to a category of causal effects frameworks, in which knowledge of the effects of the treatment on the surrogate and of the surrogate on the clinical outcome is used to predict the effect of the treatment on the clinical outcome.
The use of causal graphs modeling shows the challenge of basing a statistical validation procedure on the Prentice criteria. For true surrogate markers, there should be no direct effect of treatment independent of the marker, but instead all of the effect should be mediated by the surrogate. If there were no other causes of the clinical endpoint besides the treatment and the surrogate, analyses would be straightforward; in reality many other factors are likely to be involved. While randomization assures that treatment is not associated causally with any confounding variable, there is no reason to believe this to be true for the surrogate. In fact, the relationship of surrogate to clinical endpoint may well be confounded by other variables, each of which may or may not be measured. Joffe and Greene point out that even if the surrogate mediates the entire effect of treatment on the outcome (a most unlikely situation), the presence of confounding factors would imply that the treatment is not independent of the endpoint given the surrogate—in other words the Prentice criteria will not be met.
Model-based estimation of direct and indirect effects, possibly making use of the causal modeling approaches of Robins and Greenland (1992, 1994), offer some hope of addressing this issue, but such methods still require strong assumptions. One such assumption is that the intervention directly affects the surrogate, which in turn affects the clinical endpoint. Another is that one can control for confounding of the effect of the surrogate on the clinical outcome by proper inclusion of baseline covariates in a regression. In reality, baseline covariates may not be sufficient—an occasion that arises when a postrandomization covariate, influenced by treatment, affects the surrogate and is independently associated with outcome. For example, suppose that a blood pressure medication induced fatigue and therefore caused a reduction in the amount of exercise patients undertook; such an adverse consequence of treatment could affect both blood pressure and clinical events, such as time to myocardial infarction. Procedures are available to permit assessment of surrogacy in this situation, but
they require that the confounding be controllable, through measurement and appropriate modeling. Unfortunately, there can be no way to test such that confounding can be appropriately controlled.
The third framework mentioned by Joffe and Greene is that of meta-analysis. As described above, meta-analysis investigates the relationship of the effects of treatment on surrogates with its effects on clinical outcomes over a series of trials. The fourth framework is defined in terms of the ideas of principal stratification, developed by Frangakis and Rubin (2002). These approaches belong to the causal-association paradigm, in which the effect of treatment on the surrogate is associated, across studies or population groups, with its effect on the clinical outcome, thereby allowing prediction of the effect on the clinical outcome from the effect on the surrogate.
For the meta-analysis approach, the average value of the surrogate measured in each trial should be able to predict the outcome for that trial. Of course, such an approach requires variability in the effect of treatment on the surrogate across studies. This approach may be the most promising because of its avoidance of the need for strong assumptions regarding confounding; nonetheless, even in this case, interpretation must be made with care. For example, Daniels and Hughes (1997) demonstrated that the change in CD4 count was associated with clinical endpoints (time to new AIDS definition or death). But in their example, all of the studies with large treatment and surrogate effects compared active treatments to placebo, whereas all of the studies with small treatment or surrogate effects had active controls. Therefore, extension of the results to a setting where a trial with an active control had a strong surrogate effect may not be warranted, as the biological processes might be quite different in this case than among those that were studied.
In contrast to the meta-analytic approaches, the principal surrogacy approach focuses on the association of the individual-level effects on surrogate and outcome. As is true in general of principal stratification, the group for whom the causal effects of treatment are defined is not observable, because for each individual, the surrogate can be observed only on one treatment and not the other(s). Full description of this approach is beyond the scope of this chapter, but such analyses are most likely to be useful in settings whether there is a strong effect of treatment on both surrogate and endpoint.
In conclusion, no simple paradigm for evaluation of surrogates is possible; consistency of findings across all of the approaches described by Joffe and Greene would probably provide the most convincing evidence. But the statistical methods do not in themselves provide the type of compelling evidence that a randomized trial with nearly complete follow-up can provide. Both a deep understanding of biological context combined
with a thorough knowledge of causal research are necessary for any attempt at statistical validation of markers.
Decision Analysis Approaches to Biomarker Evaluation
Decision theory allows for logical and reproducible decision-making based on both quantitative and qualitative inputs. For biomarker evaluation, decision theory may be useful for the utilization step, and many principles from decision theory can be found throughout the report. Dr. Rebecca Miksad from Harvard University gave a presentation to the committee on decision theory as it could be applied to biomarker evaluation at the committee’s April 2009 workshop. In the presentation, Miksad defined decision science as a “field of science which rigorously and quantitatively evaluates the short and long term outcomes of complex clinical situations through analysis of clinical decisions” (Miksad, 2009). Decision analysis formalizes complex decision-making processes involving ambiguity in data, variation in data interpretation, competing benefits and risks, gaps in information, and personal preferences when applicable. Decision analysis requires that decision makers break down decisions into their component parts and make any assumptions explicit. Miksad identified five unique features of decision analysis in her presentation (Box 2-3).
While analytical sensitivity and specificity of biomarker tests are important aspects of analytical validation, it is also important to take variability between individual interpreters of data. Receiver operating characteristic (ROC) graphs are a common decision analysis tool for accomplishing this goal. An ROC graph plots the impact of data interpretation variability on use of a given decision threshold, such as a cutoff value for a diagnostic test, for example (IOM, 2005). The x-axis of an ROC curve is the likelihood of a false positive result, or 1-specificity, while the y-axis of an ROC curve is the likelihood of a false negative result, or the sensitivity (IOM, 2005). ROC curves are described in Figure 2-3.
During decision analysis, all possible choices are mapped onto a decision tree. Then, mathematical models are used to compare possible outcomes of each choice. From these models, decision makers can then choose the most appropriate course of action or identify areas where more information is needed.
Miksad outlined important questions that can be addressed using decision analysis for biomarker evaluation (Miksad, 2009):
-
What are the optimal characteristics and analytical thresholds for the biomarker assays themselves?
-
What are the positive and negative predictive values of the biomarker assays?
BOX 2-3 Five Unique Features of Decision Analysis for Surrogate Endpoint Evaluation
SOURCE: Miksad Presentation (2009). Reprinted, with permission, from Rebecca Miksad. Copyright 2009 by Rebecca Miksad. |
-
Does use of the biomarker assay lead to improved clinical outcomes?
-
What are the areas of uncertainty that lead to the largest differences in predicted affects on clinical outcomes?
-
Is additional data needed before use of the biomarker can be adopted?
Decision theory can be useful as a way to formalize the biomarker evaluation framework. While each biomarker evaluation would require a unique decision analysis, these analyses would provide stakeholders with a transparent accounting of the assumptions and subjective judgments that were needed for making specific decisions. In addition, these analyses would provide details on where biomarkers may benefit from the collection of additional data.
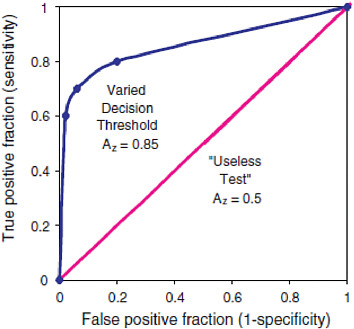
FIGURE 2-3 Receiver operating characteristic (ROC) graph of a varying decision threshold compared with a “useless test.” The best-fit curve drawn through these points is the ROC curve, which represents the overall performance of the diagnostic test across all possible interpretations (decision thresholds). The overall accuracy of this test under varying conditions is determined by the area under the complete curve, 0.85. The leftmost point shows low sensitivity and high specificity. The middle point shows moderate sensitivity and specificity. The rightmost point shows high sensitivity and low specificity. Yet because they all lie on the same curve they have the same overall statistical accuracy, which is quantified by AZ. The 45-degree-angle line represents a series of guesses between two choices, as in a coin toss. This would be considered a “useless test” if the outcome of the test was dichotomous (for example cancer vs. no cancer) for diagnostic purposes. For instance, radiologists reading mammograms with their eyes closed would tend to fall on this line. The number of true positives would approach the number of false negatives. The area under such a curve, 0.5, represents 50 percent accuracy of the test. In contrast, the ROC curve for a test with 100 percent accuracy will trace the y-axis up at a false-positive fraction of zero and follow along the top of the graph at a true-positive fraction of one. The area under such a curve would be 1.0 and represent a perfect test.
SOURCE: IOM (2005).
Qualitative Approaches to Biomarker Evaluation—Drug Development
This section describes one of the biomarker evaluation frameworks presented in the tables in Appendix A. In particular, this section discusses
efforts made through public–private partnerships to develop a standardized, fit-for-purpose biomarker evaluation process. Beginning in the late 1990s and early 2000s, drug developers began participating in the development of biomarker evaluation processes (Colburn, 1997, 2000; Wagner, 2002). This effort was further strengthened by the formation of public–private partnerships such as the Biomarker Consortium and other Foundation for National Institutes of Health (NIH) efforts, as well as the Critical Path Institute (C-Path). The frameworks proposed in collaborations with pharmaceutical industry representatives strive for several characteristics: reproducibility, clear process, risk management, and incremental or fit-for-purpose evaluations (Altar, 2008; Altar et al., 2008; Lathia et al., 2009; Wagner, 2002, 2008; Wagner et al., 2007; Williams et al., 2006). In addition, several also consider cost effectiveness in frameworks to make decisions on biomarker evaluation (Altar et al., 2008).
A 2008 paper proposed use of an “evidence map” for use in biomarker evaluations (Table 2-2) (Altar et al., 2008). This map was developed as a collaboration between pharmaceutical industry representatives, a representative from the Foundation for NIH, and an FDA representative. The paper subsequently received attention from FDA staff at a conference entitled “2008 Cardiovascular Biomarkers and Surrogate Endpoints Symposium: Building a Framework for Biomarker Application.” Briefly, the evaluation method proposed involves use of a committee to make decisions based on data and non-quantitative factors, such as public tolerability of the proposed decision. The first step in the process is for the committee to define and agree on a purpose and context of use for the biomarker. The next step is to assess the potential benefits and harms of the future success or failure of the biomarker in its proposed use. The third step is to come to an agreement about the tolerability for risk for the particular biomarker, given its proposed purpose and context of use. The fourth step is to assess the evidentiary status of the biomarker through use of the evidence map. During this step, the purpose and context-of-use combination is given a grade the biomarker needs to achieve in order to be deemed qualified. The final step is to summarize the committee’s proceedings for the stakeholders.
The authors of the paper tested this framework with a panel of experts at a workshop, and found it to be useful; they also suggested next steps to improve the framework (Altar et al., 2008). This framework provided some of the basis for Recommendations 1 and 2.
In 2009, many industry authors of the Altar et al. (2008) paper published a paper commenting on the use of surrogate endpoints for drug approvals. They described characteristics of successful surrogate endpoints: biologic plausibility, prognostic value, and a positive correlation
between an intervention’s effect on the surrogate endpoint and the clinical endpoint (Lathia et al., 2009). A representative from CDER commented on their paper in the same issue, providing important examples of how biomarkers can be used to speed drug development without being used as surrogate endpoints (Gobburu, 2009).
Inclusion of Cost-Effectiveness Analysis in Biomarker Evaluation
A controversial issue in the drug development community is whether or not cost–benefit analysis should be part of a biomarker evaluation process. In 2006, Williams and colleagues outlined principles for biomarker evaluation that were the basis for the 2008 evidence map discussed above (Williams et al., 2006). Principle 8 was that “post hoc review of cost effectiveness should be performed at regular intervals as new information is available and conclusions recorded systematically as to how this should modify the qualification and use” (Williams et al., 2006). In 2008, this idea was discussed again: “some individuals from industry expressed great concern about the use and potential misuse of cost–benefit analyses and principles and did not wish to see them used here” (Altar et al., 2008).
Some additional considerations of the committee considered during its deliberations included the following:
-
The FDA does not include analysis of cost in decisions to approve drugs or in other regulatory decisions.
-
In their 2009 study entitled The use of surrogate outcomes in model-based cost-effectiveness analyses: a survey of UK Health Technology Assessment reports, Taylor and Elston stated that their “literature searches found no empirical studies examining the use of surrogate outcomes in [health technology assessments] and [cost-effectiveness models] therein.”
-
Conclusions regarding the cost-effectiveness analysis on drug development processes cannot be definitively drawn until evidence relating the use of a new intervention with clinical outcomes is available.
An explanation of why the committee did not include cost-effectiveness analysis as part of its biomarker evaluation process is included in Chapter 3.
TABLE 2-2 Altar et al. (2008) Proposed Evidence Map for Biomarker Qualification
Evidence Type |
Grade D |
Grade D+/C- |
Grade C |
Theory on biological plausibility |
Observed association only |
Theory, indirect evidence of relevance of the biomarker from animals |
As for lower grade but evidence is direct |
Interaction with pharmacologic target |
Biomarker identifies target in in vitro binding |
|
|
Pharmacologic mechanistic response |
In vitro evidence that the drug affects the biomarker |
In vitro evidence that multiple members of this drug class affects the biomarker |
In vivo evidence that this drug affects biomarker in animals |
Linkage to clinical outcome of a disease or toxicity |
|
Biomarker epidemiologically associated with outcome without any intervention |
Biomarker associated with change in outcome from intervention in another drug class |
Mathematics replication, confirmation |
|
An algorithm is required to interpret the biomarker and was developed from the dataset |
|
Accuracy and precision (analytic validation) |
|
|
|
Relative performance |
|
Does not meet performance benchmark |
|
SOURCE: Altar et al. (2008). Adapted, with permission, from Macmillian Publishers Ltd: Clinical Pharmacology and Therapeutics. Altar, C. A., D. Amakye, D. Buonos, J. Bloom, G. Clack, R. Dean, V. Devanarayan, D. Fu, S. Furlong, C. Girman, L. Hinman, C. Lathia, |
Grade C+/B- |
Grade B |
Grade B+/A- |
Grade A |
Theory, indirect evidence of relevance in humans |
Theory, direct evidence in humans, non-causal pathway possible |
As for lower grade, but biomarker on causal path |
Human evidence based on mathematical model of biology showing biomarker is on causal pathway |
Biomarker identifies target in in vivo binding in animals |
Biomarker identifies target in in vivo studies or from human tissue, no truth standard |
|
Biomarker identifies target in in vivo studies or from tissues in humans, with accepted truth standard |
As for lower grade but effect shown across drug class |
Human evidence that this drug affects the biomarker OR animal evidence of specificity |
Human evidence across this mechanistic drug class |
Human evidence that multiple members of this drug class affect the biomarker and the effect is specific to this class/mechanism |
As for lower grade but in this drug class |
As for lower grade but multiple drug classes albeit inconsistent or a minority of disease effect |
|
As for lower grade but consistent linkage and explains majority of disease effect |
Algorithm was developed from a different dataset and applied here prospectively |
|
|
Algorithm developed from different dataset, replicated prospectively in other sets and applied prospectively here |
Sources of technical variation are unknown but steps are taken to ensure consistent test application |
Major sources of variation known and controlled to be less than biological signal; standardization methods applied |
|
All major sources of technical imprecision are known, and controlled test/assay accuracy is defined against standards |
Similar performance to benchmark |
|
|
Exceed performance of benchmark or best alternative biomarker |
L. Lesko, S. Madani, J. Mayne, J. Meyer, D. Raunig, P. Sager, S. A. Williams, P. Wong, and K. Zerba. 2008. A prototypical process for creating evidentiary standards for biomarkers and diagnostics. Clinical Pharmacology and Therapeutics 83(2):368–371, Copyright 2007. |
EVOLUTION OF REGULATORY PERSPECTIVES ON SURROGATE ENDPOINTS
Table 2-3 outlines the regulations and guidances pertaining to surrogate endpoints and the FDA. FDA regulatory authority for drugs, biologics, devices, foods, and supplements is discussed in detail in Chapter 5. While not discussed in detail, the NIH has historically played a vital role in the discovery, development, and regulatory perspective toward biomarkers; this is discussed briefly in Box 2-4.
2006–2008: FDA Pilot Process for Biomarker Qualification
Federico Goodsaid and Felix Frueh developed a biomarker qualification pilot process at the FDA, in collaboration with C-Path (Goodsaid, 2008a, 2008b; Goodsaid and Frueh, 2006, 2007a, 2007b; Goodsaid et al., 2008). The FDA pilot process for biomarker qualification was designed to qualify biomarkers incrementally, based on the data that are available for drug development or clinical applications. A biomarker would first be qualified in a narrow context of use, and then the context of use would be expanded as additional information became available. The
TABLE 2-3 List of Regulations and Guidances Pertaining to Surrogate Endpoints
Regulation or Guidance |
Significance |
21 C.F.R. 314.510 |
Accelerated approval: drugs. “Surrogate - Approval based on a surrogate endpoint or on an effect on a clinical endpoint other than survival or irreversible morbidity.”a |
21 C.F.R. 601.41 |
Accelerated approval: biologics. “Surrogate - Approval based on a surrogate endpoint or on an effect on a clinical endpoint other than survival or irreversible morbidity.”a |
Guidance for industry: Available therapy (FDA, 2004) |
This guidance states that “the approval of one therapy under the accelerated approval regulations (either on the basis of a surrogate endpoint or with restricted distribution) should not preclude the approval under the accelerated approval regulations of additional therapies.” |
Regulation or Guidance |
Significance |
21 C.F.R. 314.520 |
Postmarket authority of Food and Drug Administration (FDA) for drug accelerated approvals: “Restricted - Approval with restrictions to assure safe use.”a |
21 C.F.R. 601.42 |
Postmarket authority of FDA for biologic accelerated approvals: “Restricted - Approval with restrictions to assure safe use.”a |
21 C.F.R. parts 862–872, among others |
The C.F.R. mentions surrogate endpoints in exceptions to the exemption of class I and II medical devices from premarket review: devices measuring analytes that are to serve as surrogate endpoints must undergo premarket review. |
Guidance for industry and FDA staff: Postmarket surveillance under section 522 of the Federal Food, Drug, and Cosmetic Act (CDRH, 2006) |
Postmarket surveillance may be requested when “premarket evaluation of the device may have been based on surrogate markers. Once the device is actually marketed, postmarket surveillance may be appropriate to assess the effectiveness of the device in detecting or treating the disease or condition, rather than the surrogate.” |
Guidance for industry: Clinical studies section of labeling for human prescription drug and biological products—Content and format (FDA, 2006a) |
This guidance document recommends that manufacturers include more information in the Clinical Studies section of the label when “The study uses an unfamiliar endpoint (e.g., a novel surrogate endpoint), or there are important limitations and uncertainties associated with an endpoint.” |
Guidance for industry: Clinical data needed to support the licensure of seasonal inactivated influenza vaccines (CBER, 2007) |
The document states that “For influenza vaccines, the immune response elicited following receipt of the vaccine may serve as a surrogate endpoint that is likely to predict clinical benefit, that is, prevention of influenza illness and its complications.” |
Guidance for industry: Clinical trial endpoints for the approval of cancer drugs and biologics (FDA, 2007) |
The document describes current and past thought on use of non-survival endpoints in oncology approvals. A table comparing important cancer endpoints is presented. |
Regulation or Guidance |
Significance |
Guidance for industry and FDA staff: Clinical study designs for catheter ablation devices for treatment of atrial flutter (CDRH, 2008) |
The document states that “acute procedural success may be appropriate to serve as a surrogate effectiveness endpoint for catheters provided all of the following device characteristics are present:
|
Guidance for industry: Evidence-based review system for the scientific evaluation of health claims (CFSAN, 2009a) |
Includes the definition of surrogate endpoint discussed in Chapter 1. The document lists the four currently accepted surrogate endpoints for health claims: “(1) serum low-density lipoprotein (LDL) cholesterol concentration, total serum cholesterol concentration, and blood pressure for cardiovascular disease; (2) bone mineral density for osteoporosis; (3) adenomatous colon polyps for colon cancer; and (4) elevated blood sugar concentrations and insulin resistance for type 2 diabetes.” However, it also stipulates that biomarkers not on the biological pathway of a particular nutrient–disease risk link may not be used as surrogate endpoints for development of health claims. |
NOTE: |
qualification process, as outlined in Figure 2-4, involves FDA reviewers, outside experts, and advisory committees. The process started with a two-page letter submitted to the FDA. The letter includes a description of the biomarker, an accurate definition of the context of use that the biomarker is being proposed for, and a list of the data supporting the request. Submissions are made by companies, consortia, and academics.
BOX 2-4 FDA’s Risk Communication Advisory Committee The FDA’s Risk Communication Advisory Committee was created in 2008 with the following purpose: The Committee advises the Commissioner of the Food and Drugs or designee on methods to effectively communicate risk associated with products regulated by the Food and Drug Administration and in discharging responsibilities as they relate to helping to ensure safe and effective drugs for human use and any other product for which the Food and Drug Administration has regulatory responsibility. The Committee reviews and evaluates strategies and programs designed to communicate with the public about the risks and benefits of FDA-regulated products so as to facilitate optimal use of these products. It also reviews and evaluates research relevant to such communication to the public by both FDA and other entities, and facilities interactively sharing risk and benefit information with the public to enable people to make informed independent judgments about use of FDA-regulated products. (FDA, 2010) The committee is currently chaired by Dr. Baruch Fischoff, professor in the Departments of Social & Decision Sciences and Engineering & Public Policy at Carnegie Mellon University. The committee has ten additional members. The committee meets four times a year. In 2009, the committee discussed topics such as
SOURCE: FDA (2010). |
The next step is the recruitment of a biomarker qualification review team. A briefing document is requested from the group submitting the request, and then a face-to-face meeting is held between the review team and the group submitting the request. The gaps in evidence are evaluated, revised data packages are requested, and the process goes back and forth until the package is as complete as possible. Then, the review team writes a document, and a regulatory briefing is submitted (Goodsaid et al., 2008). Goodsaid emphasized in his presentation at the Cardiovascular Markers of Disease (CMOD) conference that “biomarker qualification is the process by which data are provided to show that exploratory biomarkers are qualified for application in a specific context of use,” and that “the context of use for a biomarker is the general area of biomarker application, specific
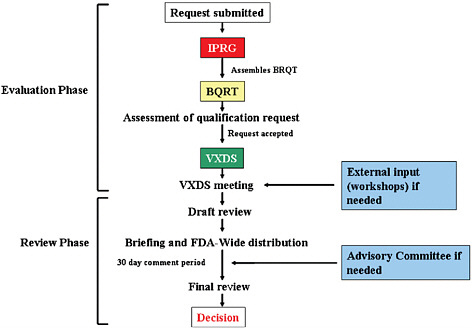
FIGURE 2-4 Outline of the Food and Drug Administration’s (FDA’s) biomarker qualification pilot process.
NOTE: BQRT = Biomarker Qualification Review Team; IPRG = Interdisciplinary Pharmacogenomic Review Group; VXDS = voluntary data submission.
SOURCE: Goodsaid et al. (2008). Reprinted with permission from Elsevier. Copyright 2008, Elsevier.
applications/implementations and critical factors which define where a biomarker is to be used and how the information from measurement of this biomarker is to be integrated in drug development and regulatory review” (Goodsaid, 2008a).
Melanie Blank, medical officer in the Division of Cardiovascular and Renal Products in CDER, has also discussed the FDA pilot process for biomarker qualification and how the evidentiary standards would be higher when the consequences of false results are graver (Blank, 2008): the qualification process as it would be applied to several problems such as how efficacy biomarkers can help in large, expensive drug trials where the clinical endpoint is rare and delayed, how safety biomarkers contribute when there is late discovery of toxicity resulting in late abandonment of the drug development program, and how safety biomarkers contribute when there are no sensitive methods to detect observed preclinical toxicities.
Example: Biomarkers of Kidney Toxicity
Dr. Joseph Bonventre of Harvard University spoke to the committee members at their first meeting. He has been involved on FDA committees as well as the only academic participant in the Critical Path Institute’s biomarker qualification effort, which was done in collaboration with Federico Goodsaid at the FDA and industry partners. The Predictive Safety Testing Consortium (PSTC), as part of the Critical Path Institute’s efforts in the area of biomarker evaluation, assembled a panel of scientists to evaluate potential safety biomarkers of acute kidney injury. These biomarkers are needed for use in “early diagnosis, to monitor severity and progression of disease, predict an outcome without an intervention, better stratify patients for clinical trials, predict who will respond to an intervention, [determine whether] the intervention [is] working ([through use of a] surrogate [endpoint]), and to identify therapeutic targets for an intervention” (Bonventre, 2009).
The most commonly used biomarkers for kidney injury are functional biomarkers rather than biomarkers of injury: serum creatinine and blood urea nitrogen. As in many organ systems, there are different stages of injury: risk, damage, reduction in function, organ failure, and death. Complications are associated with each stage. Elevations of serum creatinine and blood urea nitrogen above established normal ranges occur only after significant renal damage is present. Biomarkers of injury were the target of the preclinical studies.
Preclinical studies were conducted under the context of the PSTC, mostly internally at the FDA or in industry. Conferences were held early in the process with the European Medicines Agency (EMEA) and the Japanese drug regulatory agency. Following the process outlined in the previous section, seven biomarkers were validated and qualified: KIM-1, albumin, total protein, 2-microglobulin, cystatin C, urinary clusterin, and urinary trefoil factor 3.
As a result of the new biomarkers and validation information obtained in these studies, creatinine is no longer sufficient for showing safety at the FDA. The final step in the process occurred in June 2008, when the FDA and EMEA released a statement: “In the first use of a framework allowing submission of a single application to the two agencies, the FDA and the EMEA worked together to allow drug companies to submit the results of seven new tests that evaluate kidney damage during animal studies of new drugs” (FDA, 2008a). The need for better safety biomarkers relating to kidney toxicity and efforts to address this issue are also described in the IOM’s recent workshop summary Accelerating the Development of Biomarkers for Drug Safety (IOM, 2009a).
Surrogate Endpoints in Nutrition: Foods, Supplements, and Public Health
The following sections describe the types of claims found on food packaging in the United States and how biomarkers play a role in their evidentiary substantiation.
Health Claim Definition
Health claims for foods and dietary supplements are “voluntary statements that characterize the relation between a substance and its ability to reduce the risk of disease or health-related condition” (Schneeman, 2007). Third-party references, written statements, symbols, or vignettes (e.g., brand names including the word heart or heart symbols) that relate a food substance to reduced risk of disease are considered health claims. Implied health claims are statements, symbols, vignettes, and other forms of communication that suggest a relationship between a substance and a disease or health-related condition.5
Health claims consist of two parts, a substance (specific food or component of food, including a dietary supplement) and a disease or health-related condition (damage to an organ, part, structure, or system of the body such that it does not function properly or a state of health leading to such dysfunction).6 In addition, health claims are directed to the general population or population subgroups (e.g., the elderly, women) with the intent to assist the consumer in maintaining healthful dietary practices (CFSAN, 2009a).
As a point of history, prior to the 1990 legislation authorizing health claims, a claim on a food label that referred to a disease condition resulted in the product being classified as a drug and subject to drug regulations. However, emerging science of the 1970s and 1980s had begun to demonstrate a relationship between dietary substances and reduced risk of disease. Taylor and Wilkening (2008) note that “it seemed untenable that only drug products could mention diseases on their labels and even less tenable that food substances with the potential to reduce risk be regulated as drugs.” To avoid drug status,7 health claims cannot assert or imply that they prevent, treat, or mitigate disease, but instead only to reduce the risk of disease.
Legal Basis for Health Claims and Review of Evidence for Health Claims
The Federal Food, Drug, and Cosmetic Act (FDCA) authorizes the Food and Drug Administration to regulate food and dietary supplement labels. In respect to health claims, the FDCA has been amended over time by the 1990 Nutrition Labeling and Education Act (NLEA), the 1994 Dietary Supplement and Health Education Act (DSHEA), and the Food and Drug Administration Modernization Act of 1997. A 1999 court decision (Pearson v. Shalala) further influenced the FDA’s process of evaluating health claims by allowing claims of lesser evidence, accompanied with qualifying language.
The NLEA made nutrition labeling on most foods mandatory and allowed health claims that are based on significant scientific agreement (SSA), or:
based on the totality of publicly available scientific evidence (including evidence from well-designed studies conducted in a manner which is consistent with generally recognized scientific procedures and principles), that there is significant scientific agreement among experts qualified by scientific training and experience to evaluate such claims, that the claim is supported by such evidence.8
DSHEA further amended the FDCA to provide for the use of health claims and nutrient content claims on eligible supplement products, and to provide for the use of structure/function claims. The FDA Modernization Act amended the FDCA to allow health claims based on an authoritative statement of a scientific body of the U.S. government or the National Academy of Sciences. In 1999, the U.S. Court of Appeals found that the SSA standard was overly stringent and violated First Amendment rights by constricting commercial free speech.9 The court found that claims that did not meet the SSA standard were legal if accompanied by appropriate qualifying language.
In 2009, CFSAN completed a guidance for industry that outlined the agency’s current thinking on the process for evaluating scientific evidence for a health claim, the meaning of the SSA standard, and credible scientific evidence to support qualified health claims (CFSAN, 2009a). In the evidence-based review system for the scientific evaluation of health claims, CFSAN has outlined a process to evaluate the strength of the scientific evidence to support a claim about a substance/disease relationship. First, the agency conducts a literature search to identify studies that evaluate the substance/disease relationship, primarily in humans. Studies
are categorized into intervention studies, observational studies, research synthesis studies, and animal and in vitro studies, and are evaluated and assessed for methodological quality. The agency then sets out to evaluate the totality of scientific evidence about a substance/disease relationship by considering study type, methodological quality rating, number of the various types of studies and sample sizes, relevance of the body of scientific evidence to the U.S. population or target subgroup, replications of findings, and overall consistency of the scientific evidence. Assessing whether the SSA standard is met and specifying the approved claim language are also part of this evidence-based review system.
According to Kathy Ellwood and Paula Trumbo’s presentation at the first committee meeting, there is no difference in how the scientific evidence is reviewed for an SSA-level claim or qualified health claim: “Health claims represent a continuum of scientific evidence that extends from very limited or inconclusive evidence to consensus, with evidence supporting SSA health claims lying closer to consensus” (Trumbo and Ellwood, 2009). In the scientific review of evidence for health claims, “Surrogate endpoints of disease risk” considered valid by the FDA’s Center for Food Safety and Applied Nutrition include serum LDL cholesterol, total serum cholesterol, and blood pressure for cardiovascular disease; bone mineral density for osteoporosis; adenomatous colon polyps for colon cancer; and elevated blood sugar concentrations and insulin resistance for type 2 diabetes (CFSAN, 2009a). Health claims based on surrogate endpoints include both authorized and qualified claims (Table 2-4). It is important to note that structure/function claims, nutrient content claims, and dietary guidance statements are not based on this scientific evidence review. Because most of these claims do not make reference to disease or health-related conditions, surrogate endpoints are generally not relevant to these types of claims.
Types of Health Claims
Health claims based on significant scientific agreement (authorized health claims) According to Schneeman, the SSA standard “is based on a high level of confidence in the validity of the relation between the substance and the disease or health-related condition” (Schneeman, 2007) and considers the totality of publicly available evidence. When the NLEA was implemented, it required the FDA to consider health claims for 10 specific relationships, of which 8 were approved (Taylor and Wilkening, 2008):
-
Calcium and osteoporosis;
-
Sodium and hypertension;
-
Dietary fat and cancer;
-
Dietary saturated fat and cholesterol and CHD;
-
Fiber-containing grain products, fruits, and vegetables and cancer;
-
Fruits, vegetables, and grain products containing fiber, especially soluble fiber, and CHD;
-
Fruits and vegetables and cancer; and
-
Folic acid and neural tube defects.
In addition to these initial approved health claims, the NLEA provided a petition process for the consideration of future health claims, involving the petitioner submitting all relevant scientific findings to the FDA. Through this process, an additional seven claims have been approved. Approved health claims that were based on surrogate endpoint data are shown in Table 2-5.
Health claims approved under the SSA standard require specific claim language to be followed. For example, the model health claim language approved for sodium and high blood pressure includes: “Development of hypertension or high blood pressure depends on many factors. [This
TABLE 2-4 Health Claims Based on Surrogate Endpoints
TABLE 2-5 Qualified Health Claims Approved by the Food and Drug Administration
Category of Disease |
Approved Qualified Health Claims |
Cancer |
Tomatoes and prostate, ovarian, gastric, and pancreatic cancers Calcium and colon/rectal cancer and calcium and colon/rectal polyps Green tea and risk of breast, prostate cancer Selenium and site-specific cancers Antioxidant vitamins C and E and risk of certain cancers |
Cardiovascular disease |
Folic acid, vitamin B6, vitamin B12 and vascular disease Walnuts and coronary heart disease Nuts and coronary heart disease Omega-3 fatty acids and reduced risk of coronary heart disease Corn oil and corn oil-containing products and a reduced risk of heart disease Unsaturated fatty acids from canola oil and reduced risk of coronary heart disease Monounsaturated fatty acids from olive oil and coronary heart disease |
Cognitive function |
Phosphatidylserine and cognitive function and dementia |
Diabetes |
Chromium picolinate and a reduced risk of insulin resistance, type 2 diabetes |
Hypertension |
Calcium and hypertension, pregnancy-induced hypertension, and preeclampsia |
Neural tube defects |
Folic acid and neural tube defects |
SOURCE: CFSAN (2009b). |
product] can be part of a low sodium, low salt diet that might reduce the risk of hypertension or high blood pressure.”10
Health claims based on authoritative statements The Food and Drug Administration Modernization Act of 1997 specified that the FDA’s scientific review process could be circumvented if other scientific bodies of the U.S. government or the National Academy of Sciences11 had issued authoritative statements about the substance/disease relationship. Authoritative statements from the National Academy of Sciences were
used to approve three additional health claims—the relationship between whole grains and heart disease, the relationship between certain cancers and potassium, and the relationship between high blood pressure and stroke (Taylor and Wilkening, 2008).
Qualified health claims Litigation over the SSA standard for dietary supplements resulted in an FDA process to approve claims with lesser evidence, given additional qualifying language (qualified health claims). In Pearson v. Shalala, appellants argued that the high SSA standard impeded First Amendment commercial free speech. According to Schneeman (2007), “courts indicated that the FDA had not presented any data that potentially misleading claim language would not be cured by qualifying language enabling consumers to understand the nature of the evidence supporting a claim.” The FDA used a mechanism known as enforcement discretion to allow for the use of qualified health claims (rather than through authorization and publication in the Federal Register, as required in the NLEA for SSA health claims) (Taylor and Wilkening, 2008).
As part of a guidance on interim procedures for health claims, FDA proposed a scientific ranking system for health claims, where A-level evidence refers to SSA-level health claims and B-, C-, and D-level evidence refers to the differing levels of evidence for qualified health claims (see Figure 2-5). This ranking system is not used. The FDA approved a B-level qualified health claim for the relationship between walnuts and coronary heart disease. The qualifying language approved was: “supportive but not conclusive research shows that eating 1.5 ounces per day of walnuts, as part of a low saturated fat and low cholesterol diet and not resulting in increased caloric intake, may reduce the risk of coronary heart disease. See nutrition information for fat [and calorie] content” (CFSAN, 2004). The relationship between selenium and cancer was approved as a C-level health claim with the associated qualifying language: “Selenium may reduce the risk of certain cancers. Some scientific evidence suggests that consumption of selenium may reduce the risk of certain forms of cancer. However, [the] FDA has determined that this evidence is limited and not conclusive” (CFSAN, 2003).
An example of qualifying language for a D-level qualified health claim is the relationship between tomatoes/tomato sauce and prostate cancer. The disclaimer language the FDA approved included “very limited and preliminary scientific research suggests that eating one-half to one cup of tomatoes and/or tomato sauce a week may reduce the risk of prostate cancer. [The] FDA concludes that there is little scientific evidence supporting this claim” (CFSAN, 2005). Likewise, the relationship between tomatoes and pancreatic cancer was also approved as a D-level qualified health claim with the associated disclaimer: “one study suggests
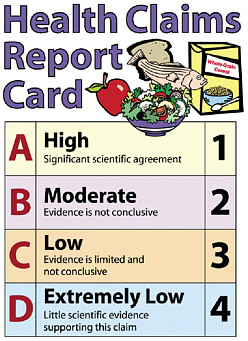
FIGURE 2-5 2003 Food and Drug Administration ranking system for health claims. Claims that met the significant scientific agreement standard were considered A-level claims and were unqualified (requiring no disclaimer). Qualified claims (levels B through D) required disclaimers, such as “evidence is not conclusive.”
SOURCES: FDA (2003). See also Mitka (2003).
that consuming tomatoes does not reduce the risk of pancreatic cancer, but one weaker, more limited study suggests that consuming tomatoes may reduce this risk. Based on these studies, [the] FDA concludes that it is highly unlikely that tomatoes reduce the risk of pancreatic cancer” (CFSAN, 2005).
To date, dozens of qualified health claim petitions have been submitted to the FDA. Qualified health claim petitions have been approved for several categories of disease, including cancer, cardiovascular disease, cognitive function, diabetes, hypertension, and neural tube defects (see Table 2-5). On the FDA’s website, the denied petitions for qualified health claims are also listed, and include lycopene and cancer, green tea and reduced risk of cardiovascular disease, vitamin E and heart disease, among others (a total of 15 letters of denial have been produced, with one petition—soy protein and cancer—withdrawn) (CFSAN, 2009b).
Other Types of Claims
Nutrient content claims Nutrient content claims expressly or implicitly characterize a level of a nutrient (e.g., “low in fat,” “high in vitamin C”) in a product (IFT, 2005). Nutrient content claims were established to provide consistent usage throughout the food supply. Prior to the NLEA, nutrient content claims were not standardized, enabling manufacturers to claim “rich in oat bran,” “extremely low in saturated fat,” with “no assurance that the levels in the food were in fact high or low relative to other similar foods or to an overall diet” (Taylor and Wilkening, 2008).
The FDA currently accepts a number of content claims including free, low, lean, extra lean, high, good source, reduced, less, light, fewer, and more. In addition, the FDA has allowable synonyms for each of the core terms (Taylor and Wilkening, 2008). Nutrient content claims have been authorized for substances that have established Daily Reference Values (DRVs) or Reference Daily Intakes (RDIs), collectively referred to as Daily Values (DVs). For example, a label may claim that the food is “high in,” “rich in,” or an “excellent source” of a nutrient if the food provides 20 percent or more of the DVs per RACC (Reference Amount Customarily Consumed) (IFT, 2005). Although foods without established DVs cannot have core content claims, manufacturers can make labeling statements, such as “contains x mg lycopene per serving,” because it does not imply whether the amount of the nutrient is high or low based on DVs, as long as the statement is not misleading (IFT, 2005).
Structure/function claims Claims about the dietary impact of a nutrient on the structure or function of the human body are generally allowed. However, these types of claims cannot suggest that the food or nutrient will cure, mitigate, prevent, or treat disease because that makes it a drug claim. Several structure/function claim examples include “calcium helps build strong bones” or “protein helps build strong muscles.” The Institute of Food Technologists note that there is “considerable uncertainty about how far this type of structure/function claim can be ‘pushed’ before [the] FDA will assert either drug status or health claim status” (IFT, 2005).
Dietary guidance statements Although not considered claims, dietary guidance statements also appear on food labeling. As compared to health claims, dietary guidance statements make reference to either a food substance or a disease, but do not relate these two components in the claim. For example, a dietary guidance statement may say “carrots are good for your health” or “calcium is good for you.” Unlike health claims, truthful, non-misleading dietary guidance statements may be used on food labels without premarket review by the FDA (CFSAN, 2008).
BIOMARKERS AND COMMUNICATION STRATEGIES AT THE FDA
Effective use of biomarkers for many purposes depends on the ability of regulators, health-care practitioners, and even advertisers to clearly communicate information about the biomarkers as well as the risks and benefits related to their use. Biomarker use also depends on the ability of the public and others to understand this information. In this and the next section, communication strategies as well as numeracy are discussed, with attention to topics most relevant to public understanding and acceptance of biomarker use.
Research on effective communications in the clinical setting and with respect to prescription and over-the-counter drugs has shown the dramatic effects that good communication strategies can have on patient outcomes. In the clinical setting, studies have pointed to the need for clinicians to receive training on how to communicate with their patients about potential risks of medical treatment (IOM, 2007b; NCI, 2007; Nicholson, 1999). In a review of effective risk communication strategies for cancer genetic counseling, Julian-Reynier and colleagues (2003) emphasized the importance and challenges of providing standardized information about risks of testing to relevant populations as well as individually tailored information based on the patient’s immediate concerns. Berry explained many issues of risk communication from a psychology perspective in the book Risk, Communication and Health Psychology (Berry, 2004); the understanding and approaches suggested in this book are generally applicable across different health-related settings. The Cochrane Collaboration has reviewed strategies and decision aids for helping patients make decisions about screening tests or health treatments (Edwards et al., 2006; O’Connor et al., 2009). In general, research has found that symbolic representations of probabilistic information, when presented well, are the most effective at enhancing patient–provider communication (Akl et al., 2007; Kim et al., 2009; Lipkus, 2007).
As the primary agency in charge of the safety of foods and drugs, the FDA uses and provides access to a great deal of information on the safety of food, supplements, drugs, biologics, and devices, and on the strength of evidence supporting certain types of health claims on foods and supplements. However, this information can be difficult to access or interpret. Therefore, the main sources of information for clinicians and consumers about the safety, efficacy, and accuracy of product claims that are subject to regulatory review are (1) the labels and package inserts of drugs, biologics, and devices, (2) the drug facts panels found on over-the-counter medication packaging, and (3) the nutrition facts panels and health claims on food packaging.
A recent perspective by Schwartz and Woloshin (2009) in the New
England Journal of Medicine highlighted some of the problems with drug labels:
-
Drug labels are written by drug companies, and not the FDA. As a result, the FDA may overlook omissions, exaggerations, or inconsistencies in the drug labels.
-
For this reason, important information about drug risks may not appear in the final drug label.
-
For the same reason, information about the possible benefits of the drugs also may not appear on the drug label.
-
A reflection of the reviewers’ confidence in the approval decision is rarely reflected in the drug label.
Schwartz and Woloshin noted that the FDA has recognized these problems and has begun to address them. The Risk Communication Advisory Committee was initiated at the FDA in 2008 (see Box 2-4) (FDA, 2008b). A draft guidance not yet finalized was issued in 2006 recommending the use of a prescription drug information highlights panel to “provide immediate access to the information that practitioners most commonly refer to and view as most important” (FDA, 2006b). Inclusion of summaries of the following information was suggested: date of initial U.S. approval, boxed warnings, recent major changes in the label, indications and usage, dosage and administration, dosage forms and strengths, contraindications, warnings and precautions, adverse reactions, drug interactions, and use in special populations.
Effective drug labels have been studied, and the data show that concise, balanced information with symbolic communication aids are useful (Davis et al., 2009; Dowse and Ehlers, 2005; Mansoor and Dowse, 2003; Schwartz et al., 2009). These findings have been discussed at several IOM workshops (IOM, 2007c, 2008), where speakers have suggested that a standardized drug label would improve patient understanding and adherence (IOM, 2008). The challenges of accomplishing this goal were highlighted by Shrank and colleagues (2009) after the conclusion of a study on the ability of a new drug label design to improve patient outcomes in several chronic diseases.
In 2006, the FDA began requiring companies to submit drug label information in an electronic format to enable public access to this information on the FDA website (FDA, 2005). To enhance the usefulness of this information to the public, the committee identified a need to improve the description of the balance of risks and benefits and to expand the product categories included in the database. The website, Drugs@FDA, is not readily found (FDA, 2009). It does not appear on the first 10 pages of
results in a Google search on “FDA electronic drug label,” for example.12 Improvement and expansion of this database and the accessibility of the website would be beneficial.
FURTHER ISSUES WITH USE OF BIOMARKERS
The need for effective communication is important for foods and supplements in addition to drugs, biologics, and devices. A recent report to the FDA Science Board recommended interfacing with universities to improve risk communication (Subcommittee on Science and Technology, 2007). Recommendations 6.1 and 6.2 of the IOM’s The Future of Drug Safety report focused on ways that the FDA Center for Drug Evaluation and Research could improve risk communication with stakeholders (IOM, 2007a). As a result of these recommendations, the Risk Communication Advisory Committee was created at the FDA. To build on these recommendations, this biomarker evaluation report seeks to extend the intent of these recommendations across regulated product categories and a broader range of stakeholders.
Healthcare providers face a challenging task in conveying health-related information to the public. Professional societies can help healthcare providers obtain skills in how to communicate with their patients about the probabilistic nature of health-related evidence and decisions. Professional societies have an important role to play in helping physicians, consumers, dietitians, other healthcare workers, and individuals in the pharmaceuticals, biologics, medical devices, supplements, and food industries to understand the consequences of innumeracy, evidence gaps, and the insufficiency of evidence to predict all outcomes when evidence is based on surrogate endpoints, other biomarkers, short-term clinical trials, or observational studies alone rather than clinical endpoints.
Numeracy
The need to improve health literacy has been widely recognized. The IOM made recommendations for addressing the issue in a 2004 report in which health literacy was defined as “the degree to which individuals have the capacity to obtain, process, and understand basic health information and services needed to make appropriate health decisions” (IOM, 2004). That definition had been in use previously by several other groups (HHS, 2000; IOM, 2004; Selden et al., 2000).
One important component of health literacy is numeracy, the ability
to understand and interpret the integers, decimals, percentages, and fractions encountered in daily life and to perform related arithmetic (Peters et al., 2007). Its importance actually goes far beyond the ability to understand and make health-related decisions for one’s self and family; it is needed for financial transactions, cooking, sewing, building, navigating, and making health-related decisions. Golbeck et al. (2005) define health numeracy as “the degree to which individuals have the capacity to access, process, interpret, communicate, and act on numerical, quantitative, graphical, biostatistical, and probabilistic health information needed to make effective health decisions.”
Lower numeracy is associated with less consumer comprehension of drug labels (Davis et al., 2006; Nelson et al., 2008) and food labels (Levy and Fein, 1998; Rothman et al., 2006). Lower numeracy is also associated with poorer health outcomes (Ancker and Kaufman, 2007; Nelson et al., 2008). A great deal of research focuses on strategies for communication between healthcare providers and patients about risks and probabilities (Akl et al., 2007; Apter et al., 2008; Fagerlin et al., 2005; Montori and Rothman, 2005; Peters et al., 2007).
Innumeracy is a problem that goes beyond the general public, however. Researchers have found that numeracy does not necessarily correlate as closely with education as literacy (Jacobson, 2007; Nelson et al., 2008). Nelson et al. (2008) and others recommend the use of short assessments by practitioners so they can better tailor their communication to their patients (Keller and Siergrist, 2009). Furthermore, healthcare practitioners themselves must deal with innumeracy. The adoption and practice of evidence-based medicine depends on physicians’ ability to understand and communicate risk and other probabilistic information (Jacobson, 2007; Nusbaum, 2006; Rao, 2008). Innumeracy among other health professionals also needs to be addressed. For example, researchers have examined this issue in nursing (Jukes and Gilchrist, 2006) and psychology (Mulhern and Wylie, 2004).
Numeracy is important to the successful adoption of the biomarker evaluation framework recommended in this report. Understanding biomarker use and the probabilities involved requires comfort with mathematical reasoning. Without adequate numeracy, individuals will have difficulty making decisions under conditions of uncertainty, such as when there are multiple possible outcomes. Without numeracy, regulators will have difficulty explaining to industry the reasoning behind biomarker evaluation, healthcare practitioners will experience difficulty communicating with patients about the probabilities involved with predictions based on biomarkers, and the media will have difficulty in communicating about these topics with the public in general. More work is needed to determine the best ways to communicate probabilistic information and
address innumeracy. The National Research Council has made recommendations on ways to improve numeracy (NRC, 1990, 2005), and the Institute of Medicine has taken several looks at the impact of numeracy on health (IOM, 2001, 2004, 2007b, 2009b). Public support and understanding are important for successful adoption of new policies; informed consumers can help to drive change with respect to careful biomarker evaluation and use.
Cognitive Biases and Impacts of Evidence Gaps
Every day individuals make decisions on the basis of incomplete information on a variety of issues, such as education, safety, diet, health, and more. Although any decision an individual makes may be important in the course of one’s life, arguably the decisions related to health are the most likely to affect the length and quality of one’s life. For this reason, the stakes are high for these decisions, which are often guided by physicians. But just because the stakes are high does not mean more information is available to use to make an informed decision. Health-related decisions have the same uncertainties as other life decisions. In addition, decisions that policy makers and regulators must make to maximize and protect public health also have these uncertainties. To manage both risks and benefits, all stakeholders—including patients, physicians, and regulatory bodies—need access to reliable information about the uncertainties involved in health decisions.
The goal of access to information can be undermined by the strained resources of government agencies, the overload of information presented to consumers, the profit motivation of companies, and the desire by physicians to reassure their patients. The FDA has a unique relationship with all of these stakeholders and the authority to take actions to protect and promote public health. With better risk communication and access to reliable and complete information about the benefits and risks involved in health decisions, agencies like the FDA will be better able to respond and adjust to the most accurate and current data available for its regulatory decisions.
The committee identified two types of evidence gaps observed when surrogate and other types of biomarkers are used to make decisions about the efficacy of a drug or health benefits of a food. First, they do not explain the entire effect of the food or drug on a person. Second, changes in a biomarker caused by a particular drug, food, or other health intervention do not always predict changes in the clinical outcome of interest. Use of surrogate biomarkers, short-term clinical trials, or observational studies alone cannot adequately predict clinical benefit or harm, and in some cases they do not predict clinical benefit or harm at all. This caution is
even more relevant to decisions based solely on biomarkers whose data do not support use as surrogate endpoints. Without information about an intervention’s effect on clinical endpoints, it is impossible to have complete information about the efficacy and safety of the intervention.
Humans tend to oversimplify or ignore evidence gaps in order to make decisions, and are often unaware of evidence gaps. In situations of insufficient or overly complex information, humans use cognitive biases to make decisions; in other words, the types of mistakes people make when making decisions in the absence of complete information are predictable. Tversky and Kahneman explored this area in a famous 1974 paper entitled “Judgment under uncertainty: Heuristics and biases.” In this article, Tversky and Kahneman explored the heuristics of representativeness, availability, and anchoring and the biases in judgment that arise from them. Tversky and Kahneman outlined the following heuristics and related cognitive biases in their important 1974 paper:
-
The representativeness heuristic (the tendency to make judgments based on how well an element matches to preconceptions of a larger group) leads to the following biases:
-
Insensitivity to prior probability of outcomes (this is also known as neglect of probability bias, or ignoring available probabilistic information when making decisions)
-
Insensitivity to sample size
-
Misconceptions of chance
-
Insentivitiy to predictability (also known as neglect of probability bias, or ignoring available probabilistic information when making decisions)
-
The illusion of validity
-
Misconceptions of regression
-
-
The availability heuristic (making decisions based on the most readily available memories or examples) leads to the following biases:
-
Biases due to the retrievability of instances
-
Biases of imaginability
-
Illusory correlation
-
-
Adjustment and anchoring heuristic (anchoring is the tendency to allow some factor to weigh too heavily in a decision)
-
Insufficient adjustment
-
Biases in the evaluation of conjunctive and disjunctive events
-
Anchoring in the assessment of subjective probability distributions
-
Each of these heuristics and biases are explained in the referenced paper (Tversky and Kahneman, 1974). An example of insensitivity to probability bias, also known as neglect of probability bias, is when a
person chooses to eat a nutrient or other substance that has been shown in observational studies to be associated with a reduced risk of disease, while ignoring the fact that this research alone does not confirm a substance’s causal connection to a reduced risk of disease. Because these biases are well known, some may try to take advantage of them to mislead consumers.
Cognitive biases of healthcare professionals in health-related decision making have been studied in the context of emergent (Pines, 2006), acute (Aberegg et al., 2005; Freshwater-Turner et al., 2007), and chronic healthcare settings (Gruppen et al., 1994; Lutfey and McKinlay, 2009; Redelmeier and Shafir, 1995; Roswarski and Murray, 2006), while cognitive biases of patients have been evaluated in regard to illnesses such as myocardial infarction (Khraim and Carey, 2009) and cancer (Han et al., 2006).
Efforts by professional societies can help physicians, dietitians, and other healthcare practitioners be aware of information gaps and common cognitive biases when helping their patients or clients make decisions about their health care. With this knowledge, strategies can be developed and disseminated. In situations where the public and health professionals need to make decisions in the absence of complete, definitive evidence, decision makers need to be able to access balanced, non-misleading data, or they will be likely to make systematic errors in their thinking.
REFERENCES
Aberegg, S. K., E. F. Haponik, and P. B. Terry. 2005. Omission bias and decision making in pulmonary and critical care medicine. Chest 128(3):1497–1505.
Advisory Committee to the Surgeon General. 1964. Report of the Advisory Committee to the Surgeon General. Washington, DC: U.S. Department of Health, Education, and Welfare.
Afzal, A. K S. J. Jacobsen, D. W. Mahoney, J. A. Kors, M. M. Redfield, J. C. Burnett, and R. J. Rodeheffer. 2007. Prevalence and prognostic significance of heart failure stages: Application of the American College of Cardiology/American Heart Association heart failure staging criteria in the community. Circulation 115(12):1563–1570.
Akl, E. A., N. Maroun, G. Guyatt, A. D. Oxman, P. Alonso-Coello, G. E. Vist, P. J. Devereaux, V. M. Montori, and H. J. Schunemann. 2007. Symbols were superior to numbers for presenting strength of recommendations to health care consumers: A randomized trial. Journal of Clinical Epidemiology 60(12):1298–1305.
ALLHAT Officers and Coordinators for the ALLHAT Collaborative Research Group. 2000. Major cardiovascular events in hypertensive patients randomized to doxazosin vs chlorthalidone. Journal of the American Medical Association 283(15):1967–1975.
ALLHAT Officers and Coordinators for the ALLHAT Collaborative Research Group. 2002. Major outcomes in high-risk hypertensive patients randomized to angiotensin-converting enzyme inhibitor or calcium channel blocker vs. diuretic: The Antihypertensive and Lipid-Lowering Treatment to Prevent Heart Attack Trial (ALLHAT). Journal of the American Medical Association 288(23):2981–2997.
Alonso, A., G. Molenberghs, H. Geys, M. Buyse, and T. Vangeneugden. 2006. A unifying approach for surrogate marker validation based on Prentice’s criteria. Statistics in Medicine 25(2):205–221.
Altar, C. A. 2008. The Biomarkers Consortium: On the critical path of drug discovery. Clinical Pharmacology and Therapeutics 83(2):361–364.
Altar, C. A., D. Amakye, D. Buonos, J. Bloom, G. Clack, R. Dean, V. Devanarayan, D. Fu, S. Furlong, L. Hinman, C. Girman, C. Lathia, L. Lesko, S. Madani, J. Mayne, J. Meyer, D. Raunig, P. Sager, S. A. Williams, P. Wong, and K. Zerba. 2008. A prototypical process for creating evidentiary standards for biomarkers and diagnostics. Clinical Pharmacology and Therapeutics 83(2):368–371.
Ancker, J. S., and D. Kaufman. 2007. Rethinking health numeracy: A multidisciplinary literature review. Journal of the American Medical Informatics Association 14(6):713–721.
Apter, A. A., M. K. Paasche-Orlow, J. T. Remillard, I. M. Bennett, E. P. Ben-Joseph, R. M. Batista, H. Hyde, and R. E. Rudd. 2008. Numeracy and communication with patients: They are counting on us. Journal of General Internal Medicine 23(12):2117–2124.
Arno, P. S., and K. L. Feiden. 1988. Against the odds, the story of AIDS drug development, politics and profits. New York: Harper Collins Publishers.
AVERT. 2009. History of AIDS: 1987–1992. http://www.avert.org/aids-history87-92.htm# (accessed October 3, 2009).
Behrman, R. E. 1999. FDA approval of antiretroviral agents: An evolving paradigm. Drug Information Journal 33(2):337–341.
Berry, D. C. 2004. Risk, communication and health psychology. New York: Open University Press.
Bigger, J. T., J. L. Fleiss, R. Kleiger, J. P. Miller, and L. M. Rolnitzky. 1984. The relationships among ventricular arrhythmias, left ventricular dysfunction, and mortality in the 2 years after myocardial infarction. Circulation 69(2):250-258.
Blank, M. 2008. Clinical Evidentiary Standards for Biomarker Qualification. Paper presented at 2008 Cardiovascular Biomarkers and Surrogate Endpoints Symposium: Building a Framework for Biomarker Application, Bethesda, MD, September 12.
Boissel, J. P., J. P. Collet, P. Moleur, and M. Haugh. 1992. Surrogate endpoints: A basis for a rational approach. European Journal of Clinical Pharmacology 43(3):235–244.
Bonventre, J. V. 2009. Biomarkers of Kidney Injury: Dawn of a New Era. Paper read at Institute of Medicine Committee on Qualification of Biomarkers and Surrogate Endpoints in Chronic Disease, Meeting 2, Washington, DC, April 6.
Borer, J. S. 2004. Development of cardiovascular drugs: The U.S. regulatory milieu from the perspective of a participating nonregulator. Journal of the American College of Cardiology 44(12):2285–2292.
Brady, T. M., and L. G. Feld. 2009. Pediatric approach to hypertension. Seminars in Nephrology 29(4):379–388.
Burzykowski, T., G. Molenberghs, and M. Buyse. 2004. The validation of surrogate end points by using data from randomized clinical trials: A case-study in advanced colorectal cancer. Journal of the Royal Statistical Society A 167(Pt 1):103–124.
Buyse, M., and G. Molenberghs. 1998. Criteria for the validation of surrogate endpoints in randomized experiments. Biometrics 54(3):1014–1029.
Buyse, M., G. Molenberghs, T. Burzykowski, D. Renard, and H. Geys. 2000. The validation of surrogate endpoints in meta-analyses of randomized experiments. Biostatistics 1(1):49–67.
CAPS (Cardiac Arrhythmia Pilot Study) Investigators. 1986. The Cardiac Arrhythmia Pilot Study. American Journal of Cardiology 57(1):91–95.
CAPS Investigators. 1988. Effects of encainide, flecainide, imipramine and moricizine on ventricular arrrhythmias during the year after acute myocardial infarction: The CAPS. American Journal of Cardiology 61(8):501–509.
CAST (Cardiac Arrhythmia Suppression Trial) Investigators. 1989. Preliminary report: Effect of encainide and flecainide on mortality in a randomized trial of arrhythmia suppression after myocardial infarction. New England Journal of Medicine 321(6):406–412.
Carter, B. L. 2002. Blood pressure as a surrogate end point for hypertension. Annals of Pharmacotherapy 36(1):87–92.
CBER (Center for Biologics Evaluation and Research). 2007. Guidance for industry: Clinical data needed to support the licensure of seasonal inactivated influenza vaccines. http://www.fda.gov/BiologicsBloodVaccines/GuidanceComplianceRegulatoryInformation/Guidances/Vaccines/ucm074794.htm (accessed September 24, 2009).
CDRH (Center for Devices and Radiological Health). 2006. Guidance for industry and FDA staff: Postmarket surveillance under section 522 of the Federal Food, Drug, and Cosmetic Act. http://www.fda.gov/MedicalDevices/DeviceRegulationandGuidance/GuidanceDocuments/ucm072517.htm (accessed September 24, 2009).
CDRH. 2008. Guidance for industry and FDA staff: Clinical study designs for catheter ablation devices for treatment of atrial flutter. http://www.fda.gov/MedicalDevices/DeviceRegulationandGuidance/GuidanceDocuments/ucm070919.htm (accessed November 10, 2009).
CFSAN (Center for Food Safety and Applied Nutrition). 2003. Selenium and certain cancers (Qualified health claim: Final decision letter). http://www.cfsan.fda.gov/~dms/ds-ltr35.html (accessed March 17, 2009).
CFSAN. 2004. Qualified health claims: Letter of enforcement discretion—walnuts and coronary heart disease (accessed March 17, 2009).
CFSAN. 2005. Qualified health claims: Letter regarding “Tomatoes and prostate, ovarian, gastric and pancreatic cancers (American Longevity Petition).” http://www.cfsan.fda.gov/~dms/qhclyco.html (accessed March 17, 2009).
CFSAN. 2008. A food labeling guide. http://www.cfsan.fda.gov/~dms/2lg-8.html#health (accessed March 26, 2009).
CFSAN. 2009a. Guidance for industry: Evidence-based review system for the scientific evaluation of health claims. http://www.cfsan.fda.gov/~dms/hclmgui6.html (accessed February 25, 2009).
CFSAN. 2009b. Qualified health claims. http://www.foodsafety.gov/~dms/lab-qhc.html (accessed March 17, 2009).
Clarke, R., S. Lewington, P. Sherliker, and J. Armitage. 2007. Effects of B-vitamins on plasma homocysteine concentrations and on risk of cardiovascular disease and dementia. Current Opinion in Clinical Nutrition and Metabolic Care 10(1):32–39.
Cochrane, A. L. 1971. Effectiveness and efficiency: Random reflections on health services. London, England: Nuffield Provincial Hospitals Trust.
Colatsky, T. J. 2009. Reassessing the validity of surrogate markers of drug efficacy in the treatment of coronary artery disease. Current Opinion in Investigational Drugs 10(3): 239–244.
Colburn, W. A. 1997. Selecting and validating biologic markers for drug development. Journal of Clinical Pharmacology 37(5):355–362.
Colburn, W. A. 2000. Optimizing the use of biomarkers, surrogate endpoints, and clinical endpoints for more efficient drug development. Journal of Clinical Pharmacology 40(12 Pt 2):1419–1427.
Cotton, P. 1991. HIV surrogate markers weighed. Journal of the American Medical Association 265(11):1357, 1361–1362.
Daniels, M. J., and M. D. Hughes. 1997. Meta-analysis for the evaluation of potential surrogate markers. Statistics in Medicine 16(17):1965–1982.
Davis, T. C., M. S. Wolf, P. F. Bass, J. A. Thompson, H. H. Tilson, M. Neuberger, and R. M. Parker. 2006. Literacy and misunderstanding prescription drug labels. Annals of Internal Medicine 145(12):887–894.
Davis, T. C., A. D. Federman, P. F. Bass, R. H. Jackson, M. Middlebrooks, R. M. Parker, and M. S. Wolf. 2009. Improving patient understanding of prescription drug label instructions. Journal of General Internal Medicine 24(1):57–62.
De Gruttola, V., T. Fleming, D. Y. Lin, and R. Coombs. 1997. Perspective: Validating surrogate markers—Are we being naive? Journal of Infectious Diseases 175(2):237–246.
De Gruttola, V., C. Flexner, J. Schapiro, M. Hughes, M. van der Laan, and D. R. Kuritzkes. 2006. Drug development strategies for salvage therapy: Conflicts and solutions. AIDS Research and Human Retroviruses 22(11):1106–1109.
Deeks, S. G., and J. N. Martin. 2007. Partial treatment interruptions. Current Opinion in HIV and AIDS 2(1):46–55.
DeMets, D. L., and R. M. Califf. 2002. Lessons learned from recent cardiovascular clinical trials: Part I. Circulation 106(6):746–751.
Desai, M., N. Stockbridge, and R. Temple. 2006. Blood pressure as an example of a biomarker that functions as a surrogate. AAPS Journal 8(1):E146.
Dowse, R., and M. Ehlers. 2005. Medicine labels incorporating pictograms: Do they influence understanding and adherence? Patient Education and Counseling 58(1):63–70.
Echt, D. S., P. R. Liebson, L. B. Mitchell, R. W. Peters, D. Obias-Manno, A. H. Barker, D. Arensberg, A. Baker, L. Friedman, H. L. Greene, M. L. Huther, D. W. Richardson, and the CAST Investigators. 1991. Mortality and morbidity in patients receiving encainide, flecainide, or placebo: The Cardiac Arrhythmia Suppression Trial. New England Journal of Medicine 324(12):781-788.
Edwards, A. G. K., R. Evans, J. Dundon, S. Haigh, K. Hood, and G. J. Elwyn. 2006. Personalised risk communication for informed decision making about taking screening tests. Cochrane Database of Systematic Reviews 2006(4):Art. No. CD001865.
Ellenberg, S. S., and J. M. Hamilton. 1989. Surrogate endpoints in clinical trials: Cancer. Statistics in Medicine 8(4):405–413.
Emanuel, E. J., and F. G. Miller. 2001. The ethics of placebo-controlled trials—A middle ground. New England Journal of Medicine 345(12):915–919.
Ezzati, M., A. D. Lopez, A. Rodgers, S. V. Hoorn, C. J. L. Murray, and C. R. A. C. Group. 2002. Selected major risk factors and global and regional burden of disease. The Lancet 360(9343):1347–1360.
Fagerlin, A., C. Wang, and P. A. Ubel. 2005. Reducing the influence of anecdotal reasoning on people’s health care decisions: Is a picture worth a thousand statistics? Medical Decision Making 25(4):398–405.
FDA (Food and Drug Administration). 1988. Making drugs available for life-threatening diseases. http://www.aegis.com/news/FDA/1988/Fd881001.html (accessed October 3, 2009).
FDA. 1999. Significant scientific agreement in the review of health claims for conventional foods and dietary supplements. http://www.fda.gov/Food/GuidanceComplianceRegulatoryInformation/GuidanceDocuments/FoodLabelingNutrition/ucm059132.htm (accessed October 3, 2009).
FDA. 2002. Guidance for industry: Antiretroviral drugs using plasma HIV RNA measurements— Clinical considerations for accelerated and traditional approval. http://www.fda.gov/cder/guidance/3647fnl.pdf (accessed December 29, 2008).
FDA. 2003. FDA to encourage science-based labeling and competition for healthier dietary choices. http://www.fda.gov/bbs/topics/NEWS/2003/NEW00923.html (accessed March 17, 2009).
FDA. 2004. Guidance for industry: Available therapy. http://www.fda.gov/RegulatoryInformation/Guidances/ucm126586.htm (accessed September 24, 2009).
FDA. 2005a. FDA announces the use of new electronic drug labels to help better inform the public and improve patient safety. http://www.fda.gov/NewsEvents/Newsroom/PressAnnouncements/2005/ucm108509.htm (accessed November 22, 2009).
FDA. 2006a. Guidance for industry: Clinical studies section of labeling for human prescription drug and biological products—Content and format. http://www.fda.gov/RegulatoryInformation/Guidances/ucm127509.htm (accessed September 24, 2009).
FDA. 2006b. Draft guidance for industry: Labeling for human prescription drug and biological products—Implementing the new content and format requirements. http://www.fda.gov/downloads/Drugs/GuidanceComplianceRegulatoryInformation/Guidances/ucm075082.pdf (accessed November 22, 2009).
FDA. 2007. Guidance for industry: Clinical trial endpoints for the approval of cancer drugs and biologics. http://www.fda.gov/downloads/Drugs/GuidanceComplianceRegulatoryInformation/Guidances/UCM071590.pdf (accessed September 24, 2009).
FDA. 2008a. FDA, European Medicines Agency to consider additional test results when assessing new drug safety. http://www.fda.gov/NewsEvents/Newsroom/PressAnnouncements/2008/ucm116911.htm (accessed November 23, 2009).
FDA. 2008b. Risk Communication Advisory Committee. http://www.fda.gov/AdvisoryCommittees/CommitteesMeetingMaterials/RiskCommunicationAdvisoryCommittee/default.htm (accessed November 21, 2009).
FDA. 2009. Drugs@FDA. http://www.accessdata.fda.gov/Scripts/cder/DrugsatFDA/ (accessed November 11, 2009).
FDA. 2010. Risk Communication Advisory Committee. http://www.fda.gov/AdvisoryCommittees/CommitteesMeetingMaterials/RiskCommunicationAdvisoryCommittee/default.htm (accessed March 14, 2010).
Fischl, M. A., D. D. Richman, M. H. Grieco, M. S. Gottlieb, P. A. Volberding, O. L. Laskin, J. M. Leedom, J. E. Groopman, D. Mildvan, R. T. Schooley et al. 1987. The efficacy of azidothymidine (AZT) in the treatment of patients with AIDS and AIDS-related complex. A double-blind, placebo-controlled trial. New England Journal of Medicine 317(4):185–191.
Fleming, T. R. 2005. Surrogate endpoints and FDA’s accelerated approval process. Health Affairs 24(1):67–78.
Fleming, T. R., and D. L. DeMets. 1996. Surrogate end points in clinical trials: Are we being misled? Annals of Internal Medicine 125(7):605–613.
Frangakis, C. E., and D. B. Rubin. 2002. Principal stratification in causal inference. Biometrics 58(1):21–29.
Freshwater-Turner, D. A., R. J. Boots, R. N. Bowman, H. G. Healy, and A. C. Klestov. 2007. Difficult decisions in the intensive care unit: An illustrative case. Anaesthesia and Intensive Care 35(5):748–759.
Gobburu, J. V. S. 2009. Biomarkers in clinical drug development. Clinical Pharmacology and Therapeutics 86(1):26–27.
Golbeck, A. L., C. R. Ahlers-Schmidt, A. M. Paschal, and S. E. Dismuke. 2005. A definition and operational framework for health numeracy. American Journal of Preventive Medicine 29(4):375–376.
Goodsaid, F. 2008a. Impact of the Biomarker Qualification Project on Drug Development. Paper presented at 2008 Cardiovascular Biomarkers and Surrogate Endpoints Symposium: Building a Framework for Biomarker Application, Bethesda, MD, September 12.
Goodsaid, F. 2008b. Markers of Renal Toxicity. Paper presented at 2008 Cardiovascular Biomarkers and Surrogate Endpoints Symposium: Building a Framework for Biomarker Application, Bethesda, MD, September 11.
Goodsaid, F., and F. Frueh. 2006. Process map proposal for the validation of genomic biomarkers. Pharmacogenomics 7(5):773–782.
Goodsaid, F., and F. Frueh. 2007a. Biomarker qualification pilot process at the U.S. Food and Drug Administration. AAPS Journal 9(1):E105–E108.
Goodsaid, F., and F. Frueh. 2007b. Questions and answers about the pilot process for biomarker qualification at the FDA. Drug Discovery Today: Technologies 4(1):9–11.
Goodsaid, F., F. W. Frueh, and W. Mattes. 2008. Strategic paths for biomarker qualification. Toxicology 245:219–223.
Gruppen, L. D., J. Margolin, K. Wisdom, and C. M. Grum. 1994. Outcome bias and cognitive dissonance in evaluating treatment decisions. Academic Medicine 69(10 Suppl): S57–S59.
Hammer, S. M., K. E. Squires, M. D. Hughes, J. M. Grimes, L. M. Demeter, J. S. Currier, J. J. Eron Jr., J. E. Feinberg, H. H. Balfour Jr., L. R. Deyton, J. A. Chodakewitz, and M. A. Fischl. 1997. A controlled trial of two nucleoside analogues plus indinavir in persons with human immunodeficiency virus infection and CD4 cell counts of 200 per cubic millimeter or less. AIDS Clinical Trials Group 320 Study Team. New England Journal of Medicine 337(11):725–733.
Han, P. K., R. P. Moser, and W. M. Klein. 2006. Perceived ambiguity about cancer prevention recommendations: Relationship to perceptions of cancer preventability, risk, and worry. Journal of Health Communication 11(Suppl 1):51–69.
HHS (Department of Health and Human Services). 2000. Healthy People 2010: Understanding and improving health. Washington, DC: HHS.
Higgins, J., and S. Green. 2008. Cochrane handbook for systematic reviews of interventions: Version 5.0.1. http://www.cochrane.org/resources/handbook/index.htm (accessed April 2, 2009).
Hill, A. B. 1965. The environment and disease: Association or causation? Proceedings of the Royal Society of Medicine 58:295–300.
Hillis, A., and D. Seigel. 1989. Surrogate endpoints in clinical trials: Ophthalmologic disorders. Statistics in Medicine 8(4):427–430.
HIV Surrogate Marker Collaborative Group. 2000. Human immunodeficiency virus type 1 RNA level and CD4 count as prognostic markers and surrogate end points: A meta-analysis. AIDS Research and Human Retroviruses 16(12):1123–1133.
Holden, C. 1993. FDA okays surrogate markers. Science 259(5091):32–33.
Hughes, M. D. 2002. Evaluating surrogate endpoints. Controlled Clinical Trials 23(6): 703–707.
Hughes, M. D. 2005. The evaluation of surrogate endpoints in practice: Experience in HIV. In The evaluation of surrogate endpoints, edited by T. Burzykowski, G. Molenberghs, and M. Buyse. New York: Springer. Pp. 295–321.
Hughes, M. D., V. De Gruttola, and S. L. Welles. 1995. Evaluating surrogate markers. Journal of Acquired Immune Deficiency Syndromes and Human Retrovirology 10(Suppl 2):S1–S8.
Hughes, M. D., M. J. Daniels, M. A. Fischl, S. Kim, and R. T. Schooley. 1998. CD4 cell count as a surrogate endpoint in HIV clinical trials: A meta-analysis of studies of the AIDS Clinical Trials Group. AIDS 12(14):1823–1832.
IFT (Institute of Food Technologists). 2005. Functional foods: Opportunities and challenges. Chicago, IL: IFT.
IOM (Institute of Medicine). 1990. Clinical practice guidelines: Directions for a new program. Washington, DC: National Academy Press.
IOM. 2001. Health and behavior: The interplay of biological, behavioral, and societal influences. Washington, DC: National Academy Press.
IOM. 2004. Health literacy: A prescription to end confusion. Washington, DC: The National Academies Press.
IOM. 2005. Saving women’s lives: Strategies for improving breast cancer detection and diagnosis. Washington, DC: The National Academies Press.
IOM. 2007a. The future of drug safety: Promoting and protecting the health of the public. Washington, DC: The National Academies Press.
IOM. 2007b. Understanding the benefits and risks of pharmaceuticals: Workshop Summary. Washington, DC: The National Academies Press.
IOM. 2008. Standardizing medication labels: Confusing patients less: Workshop summary. Washington, DC: National Academies Press.
IOM. 2009a. Accelerating the development of biomarkers for drug safety. Washington, DC: The National Academies Press.
IOM. 2009b. Health literacy, eHealth, and communication: Putting the consumer first: Workshop Summary. Washington, DC: The National Academies Press.
IOM. 2009c. Initial national priorities for comparative effectiveness research. Washington, DC: The National Academies Press.
Israili, Z. H., R. Hernandez-Hernandez, and M. Valasco. 2007. The future of antihypertensive treatment. American Journal of Therapeutics 14(2):121–134.
Jacobson, R. M. 2007. Teaching numeracy to physicians-in-training: Quantitative analysis for evidence-based medicine. Minnesota Medicine 90(11):37–38, 46.
Jiang, H., S. G. Deeks, D. R. Kuritzkes, M. Lallemant, D. Katzenstein, M. Albrecht, and V. De Gruttola. 2003. Assessing resistance costs of antiretroviral therapies via measures of future drug options. Journal of Infectious Diseases 188(7):1001–1008.
Joffe, M. M., and T. Greene. 2009. Related causal frameworks for surrogate outcomes. Biometrics 65(2):530–538.
Jukes, L., and M. Gilchrist. 2006. Concerns about numeracy skills of nursing students. Nurse Education in Practice 6(4):192–198.
Julian-Reynier, C., M. Welkenhuysen, L. Hagoel, M. Decruyenaere, P. Hopwood, and on behalf of the CRISCOM Working Group. 2003. Risk communication strategies: State of the art and effectiveness in the context of cancer genetic services. European Journal of Human Genetics 11(10):725–736.
Keller, C., and M. Siergrist. 2009. Effect of risk communication formats on risk perception depending on numeracy. Medical Decision Making 29(4):483–490.
Khraim, F. M., and M. G. Carey. 2009. Predictors of pre-hospital delay among patients with acute myocardial infarction. Patient Education and Counseling 75(2):155–161.
Kim, H., C. Nakamura, and Q. Zeng-Treitler. 2009. Assessment of pictographs developed through a participatory design process using an online survey tool. Journal of Medical Internet Research 11(1):e5.
Krumholz, H. M., and T. H. Lee. 2008. Redefining quality—Implications of recent clinical trials. New England Journal of Medicine 358(24):2537–2539.
Lagakos, S. W., and D. F. Hoth. 1992. Surrogate markers in AIDS: Where are we? Where are we going? Annals of Internal Medicine 116(7):599–601.
Lassere, M. N. 2008. The biomarker-surrogacy evaluation schema: A review of the biomarker-surrogate literature and a proposal for a criterion-based, quantitative, multidimensional hierarchical levels of evidence schema for evaluating the status of biomarkers as surrogate endpoints. Statistical Methods in Medical Research 17(3):303–340.
Lathia, C. D., D. Amakye, W. Dai, C. Girman, S. Madani, J. Mayne, P. MacCarthy, P. Pertel, L. Seman, A. Stoch, P. Tarantino, C. Webster, S. Williams, and J. A. Wagner. 2009. The value, qualification, and regulatory use of surrogate end points in drug development. Clinical Pharmacology and Therapeutics 86(1):32–43.
Law, M. R., and J. K. Morris. 2009. Use of blood pressure lowering drugs in the prevention of cardiovascular disease: Meta-analysis of 147 randomized trials in the context of expectations from prospective epidemiological studies. British Medicine Journal 338:b1665.
Lesko, L. J., and A. J. Atkinson Jr. 2001. Use of biomarkers and surrogate endpoints in drug development and regulatory decision making: Criteria, validation, strategies. Annual Review of Pharmacology and Toxicology 41:347–366.
Levy, A. S., and S. B. Fein. 1998. Consumers’ ability to perform tasks using nutrition labels. Journal of Nutrition Education 30(4):210–217.
Lipkus, I. M. 2007. Numeric, verbal, and visual formats of conveying health risks: Suggested best practices and future recommendations. Medical Decision Making 27(5):696–713.
Lutfey, K. E., and J. B. McKinlay. 2009. What happens along the diagnostic pathway to CHD treatment? Qualitative results concerning cognitive processes. Sociology of Health and Illness 31(7):1077–1092.
MacMahon, S., R. Peto, J. Cutler, R. Collins, P. Sorlie, J. Neaton, R. Abbott, J. Godwin, A. Dyer, and J. Stamler. 1990. Blood pressure, stroke, and coronary heart disease. Part 1, prolonged differences in blood pressure: Prospective observational studies corrected for the regression dilution bias. Lancet 335(8692):765–774.
Manns, B., W. F. Owen, W. C. Winkelmayer, P. J. Deveraux, and M. Tonelli. 2006. Surrogate markers in clinical studies: Problems solved or created? American Journal of Kidney Diseases 48(1):159–166.
Mansoor, L. E., and R. Dowse. 2003. Effect of pictograms on readability of patient information materials. Annals of Pharmacotherapy 37(7–8):1003–1009.
Miksad, R. 2009. Decision science for qualification of biomarker surrogate endpoints. Paper read at Institute of Medicine Committee on Qualification of Biomarkers and Surrogate Endpoints in Chronic Disease, Meeting 2, Washington, DC, April 6.
Mitka, M. 2003. Food fight over product label claims: Critics say proposed changes will confuse consumers. Journal of the American Medical Association 290(7):871–875.
Montori, V. M., and R. L. Rothman. 2005. Weakness in numbers: The challenge of numeracy in health care. Journal of General Internal Medicine 20(11):1071–1072.
Mukharji, J., R. E. Rude, W. K. Poole, N. Gustafson, L. J. Thomas Jr., H. W. Strauss, A. S. Jaffe, J. E. Muller, R. Roberts, D. S. Raabe, C. H. Croft, E. Passamani, E. Braunwald, J. T. Willerson, and the MILIS Study Group. 1984. Risk factors for sudden death after acute myocardial infarction: Two-year follow-up. American Journal of Cardiology 54(1):31–36.
Mulhern, G., and J. Wylie. 2004. Changing levels of numeracy and other core mathematical skills among psychology undergraduates between 1992 and 2002. British Journal of Psychology 95(Pt 3):355–370.
Nambi, V., and C. M. Ballantyne. 2007. Role of biomarkers in developing new therapies for vascular disease. World Journal of Surgery 31(4):676–681.
NCI (National Cancer Institute). 2007. Patient-centered communication in cancer care. Washington, DC: NCI.
Nelson, W., V. F. Reyna, A. Fagerlin, I. Lipkus, and E. Peters. 2008. Clinical implications of numeracy: Theory and practice. Annals of Behavioral Medicine 35(3):261–274.
NHLBI Working Group on Future Directions in Hypertension Treatment Trials. 2005. Major clinical trials of hypertension: What should be done next? Hypertension 46(1): 1–6.
Nicholson, P. J. 1999. Communicating health risk. Occupational Medicine 49(4):253–256.
NRC (National Research Council). 1990. On the shoulders of giants: New approaches to numeracy. Washington, DC: National Academy Press.
NRC. 2005. Measuring literacy: Performance levels for adults. Washington, DC: The National Academies Press.
Nusbaum, N. J. 2006. Mathematics preparation for medical school: Do all premedical students need calculus? Teaching and Learning in Medicine 18(2):165–168.
O’Connor, A. M., C. L. Bennett, D. Stacey, M. Barry, N. F. Col, K. B. Eden, V. A. Entwistle, V. Fiset, M. Holmes-Rovner, S. Khangura, H. Llewellyn-Thomas, and D. Rovner. 2009. Decision aids for people facing health treatment or screening decisions. Cochrane Database of Systematic Reviews 2009(3): Art. No. CD001431.
Omenn, G. S., G. E. Goodman, M. D. Thornquist, J. Balmes, M. R. Cullen, A. Glass, J. P. Keogh, F. L. Meyskens Jr., B. Valanis, J. H. Williams Jr., S. Barnhart, and S. Hammar. 1996. Effects of a combination of beta-carotene and vitamin A on lung cancer and cardiovascular disease. New England Journal of Medicine 334:1150–1155.
Perry, H. M., A. I. Goldman, M. A. Lavin, H. W. Schnaper, A. E. Fitz, E. D. Frohlich, B. Steele, and H. G. Richman. 1978. Evaluation of drug treatment in mild hypertension: VA-NHLBI feasibility trial. Plan and preliminary results of a two-year feasibility trial for a multicenter intervention study to evaluate the benefits versus the disadvantages of treating mild hypertension. Prepared for the Veterans Administration–National Heart, Lung, and Blood Institute Study Group for Evaluating Treatment in Mild Hypertension. Annals of the New York Academy of Sciences 304:267–287.
Peters, E., J. Hibbard, P. Slovic, and N. Dieckmann. 2007. Numeracy skill and the communication, comprehension, and use of risk–benefit information. Health Affairs 26(3):741–748.
Peto, R., R. Doll, J. D. Buckley, and M. B. Sporn. 1981. Can dietary beta-carotene materially reduce human cancer rates? Nature 290:201–208.
Pines, J. M. 2006. Profiles in patient safety: Confirmation bias in emergency medicine. Academic Emergency Medicine 13(1):90–94.
Prentice, R. L. 1989. Surrogate endpoints in clinical trials: Definition and operational criteria. Statistics in Medicine 8(4):431–440.
Psaty, B. M., D. S. Siscovick, N. S. Weiss, T. D. Koepsell, F. R. Rosendaal, D. Lin, S. R. Heckbert, E. H. Wagner, and C. D. Furberg. 1996. Hypertension and outcomes research: From clinical trials to clinical epidemiology. American Journal of Hypertension 9(2):178–183.
Rao, G. 2008. Physician numeracy: Essential skills for practicing evidence-based medicine. Family Medicine 40(5):354–358.
Redelmeier, D. A., and E. Shafir. 1995. Medical decision making in situations that offer multiple alternatives. Journal of the American Medical Association 273(4):302–305.
Robins, J. M., and S. Greenland. 1992. Identifiability and exchangeability for direct and indirect effects. Epidemiology 3(2):143–155.
Robins, J. M., and S. Greenland. 1994. Adjusting for differential rates of prophylaxis therapy for PCP in high- versus low-dose AZT treatment arms in an AIDS randomized trial. Journal of the American Statistical Association 89(427):737–749.
Roswarski, T. E., and M. D. Murray. 2006. Supervision of students may protect academic physicians from cognitive bias: A study of decision making and multiple treatment alternatives in medicine. Medical Decision Making 26(2):154–161.
Rothman, R. L., R. Housam, H. Weiss, D. Davis, R. Gregory, T. Gebretsadik, A. Shintani, and T. A. Elasy. 2006. Patient understanding of food labels—The role of literacy and numeracy. American Journal of Preventive Medicine 31(5):391–398.
Ruberman, W., E. Weinblatt, J. D. Goldberg, C. W. Frank, and S. Shapiro. 1977. Ventricular premature beats and mortality after myocardial infarction. New England Journal of Medicine 297(14):750–757.
Ruskin, J. N. 1989. The Cardiac Arrhythmia Suppression Trial (CAST). New England Journal of Medicine 321(6):386–388.
Schatzkin, A., and M. Gail. 2002. The promise and peril of surrogate endpoints in cancer research. Nature Reviews Cancer 2(1):19–27.
Schneeman, B. 2007. FDA’s review of scientific evidence for health claims. Journal of Nutrition 137(2):493–494.
Schwartz, L. M., S. Woloshin, and H. G. Welch. 2009. Using a drug facts box to communicate drug benefits and harms: Two randomized trials. Annals of Internal Medicine 150(8):516–527.
Selden, C. R., M. Zorn, S. C. Ratzan, and R. M. Parker. 2000. National Library of Medicine current bibliographies in medicine: Health literacy. NLM Pub. No. CBM 2000-1. Bethesda, MD: National Institutes of Health.
Shi, Q., and D. J. Sargent. 2009. Meta-analysis for the evaluation of surrogate endpoints in cancer clinical trials. International Journal of Clinical Oncology 14(2):102–111.
Shrank, W. H., A. Patrick, P. P. Gleason, C. Canning, C. Walters, A. H. Heaton, S. Jan, M. A. Brookhart, S. Schneeweiss, D. H. Solomon, M. S. Wolf, J. Avorn, and N. K. Choudhry. 2009. An evaluation of the relationship between the implementation of a newly designed prescription drug label at Target pharmacies and health outcomes. Medical Care 47(9):1031–1035.
Subcommittee on Science and Technology. 2007. FDA science and mission at risk. Washington, DC: Food and Drug Administration.
Taylor, C. L., and V. L. Wilkening. 2008. How the nutrition food label was developed, part 2: The purpose and promise of nutrition claims. Journal of the American Dietetic Association 108(4):618–623.
Temple, R. 1999. Are surrogate markers adequate to assess cardiovascular disease drugs? Journal of the American Medical Association 282(8):790–795.
Temple, R. J. 2009. Qualification of Biomarkers as Surrogate Endpoints of Chronic Disease Risk. Paper read at Institute of Medicine Committee on Qualification of Biomarkers and Surrogate Endpoints in Chronic Disease: Meeting 2, Washington, DC, April 6.
Trumbo, P., and K. Ellwood. 2009. Developing a Framework for Biomarker Qualification for Chronic Disease. Paper presented at Institute of Medicine Committee on Qualification of Biomarkers as Surrogate Endpoints for Chronic Disease Risk, Washington, DC, January 12.
Tversky, A. And D. Kahneman. 1974. Judgment under uncertainty: Heuristics and biases. Science 185(4157):1124–1131.
Volberding, P. A., S. W. Lagakos, J. M. Grimes, D. S. Stein, H. H. Balfour Jr., R. C. Reichman, J. A. Bartlett, M. S. Hirsch, J. P. Phair, R. T. Mitsuyasu et al. 1994. The duration of zidovudine benefit in persons with asymptomatic HIV infection. Prolonged evaluation of protocol 019 of the AIDS Clinical Trials Group. Journal of the American Medical Association 272(6):437–442.
Wagner, J. A. 2002. Overview of biomarkers and surrogate endpoints in drug development. Disease Markers 18(2):41–46.
Wagner, J. A. 2008. Strategic approach to fit-for-purpose biomarkers in drug development. Annual Review of Pharmacology and Toxicology 48:631–651.
Wagner, J. A., S. A. Williams, and C. J. Webster. 2007. Biomarkers and surrogate end points for fit-for-purpose development and regulatory evaluation of new drugs. Clinical Pharmacology and Therapeutics 81(1):104–107.
Wang, T. J., and R. S. Vasan. 2005. Epidemiology of uncontrolled hypertension in the United States. Circulation 112(11):1651–1662.
Williams, B. 2005. Recent hypertension trials. Journal of the American College of Cardiology 45(6):813–827.
Williams, S. A., D. E. Slavin, J. A. Wagner, and C. J. Webster. 2006. A cost-effectiveness approach to the qualification and acceptance of biomarkers. Nature Reviews Drug Discovery 5(11):897–902.
Wittes, J., E. Lakatos, and J. Probstfield. 1989. Surrogate endpoints in clinical trials: Cardiovascular diseases. Statistics in Medicine 8(4):415–425.
Wolff, T., and T. Miller. 2007. Evidence for the reaffirmation of the U.S. Preventive Services Task Force recommendation on screening for high blood pressure. Annals of Internal Medicine 147(11):787–791.