THE PERILS OF THE LEARNING MODEL FOR MODELING ENDOGENOUS TECHNOLOGICAL CHANGE
William D. Nordhaus14
Yale University
October 18, 2010
Abstract
Modeling of technological change has been a major empirical and analytical problem for many years. One approach to modeling technology is learning or experience curves, which originated in techniques used to estimate cost functions in manufacturing modeling. They have recently been introduced in policy models of energy and global warming economics to make the process of technological change endogenous. It is not widely appreciated that this is a dangerous modeling strategy. The present study has three points. First, it shows that there is a fundamental statistical identification problem in trying to separate learning from exogenous technological change and that the estimated learning coefficient will generally be biased upwards. Second, we present two empirical tests that illustrate the potential bias in practice and show that learning parameters are not robust to alternative specifications. Finally, we show that an overestimate of the learning coefficient will provide incorrect estimates of the total marginal cost of output and will therefore bias optimization models to tilt toward technologies that are incorrectly specified as having high learning coefficients.
The Challenge of Endogenous Technological Change
Most studies and models of environmental and climate-change policy—indeed of virtually all aspects of economic policy—have sidestepped the thorny issue of endogenous technological change or induced innovation. These terms refer to the impact of economic activity and policy upon research, development, invention, innovation, productivity change, and the diffusion of new technologies. Most models assume that technological change is exogenous, that is, it proceeds with a rate and direction that is determined by fundamental scientific and technological forces but is unaffected by prices or tax and regulatory incentives.
This shortcoming has been recognized for many years. It arises both because of the lack of a firm empirical understanding of the determinants of technological change as well as because of the inherent difficulties in the modeling of economic processes with externalities and increasing returns to scale. While we suspect that we know the direction of the omission of induced innovation—to overestimate the cost of emissions reductions and the trend increase in climate change—we have little sense of the magnitude of the effect or the importance of this omission. Would including induced innovation have a large or small impact on climate change and on climate-change policies? This is a major open question.
There have been two major approaches to including induced innovation—the Romer model and the learning model. The Romer model of induced innovation arose in the 1960s in an attempt to understand why technological change appears to have been largely labor saving.15 More recently, theories of induced technological change have
14 |
This is a summary version of the full study available as William Nordhaus, “The Perils of the Learning Model For Modeling Endogenous Technological Change,” NBER Working Paper 14638, January 2009, available at http://www.econ.yale.edu/~nordhaus/homepage/recent_stuff.htm. I am grateful for comments from Christopher Magee, Nebosja Nakicenovic, Ed Rubin, and John Weyant, as well as participants at the Energy Modeling Forum, the Santa Fe Institute, and two workshops at the U.S. National Academy of Sciences. |
15 |
See R.R., Nelson, “The Simple Economics of Basic Scientific Research,” Journal of Political Economy, 67:297-306, 1959; Kenneth J. Arrow, “Economic Welfare and the Allocation of Resources for Invention,” in Richard Nelson, The Rate and Direction of Inventive Activity, Princeton University Press for National Bureau of Economic Research, 1962; C. C. von Weitzsaecker, “Tentative Notes on a Two-Sector Model with Induced Technical Progress,” Review of Economic Studies, 33, 95, July 1966, 245-51. |
been resuscitated as the “new growth theory,” pioneered by Paul Romer and others.16 The thrust of this research is to allow for investment in knowledge-improving activities. Such investments improve society’s technologies, and a higher level of investment in knowledge will change society’s production possibilities and may improve the long-run growth rate of the economy. Virtually all studies of induced innovation have been theoretical.17 With few exceptions, they do not lay out a set of testable hypotheses or ones that can be used to model the innovation process at an industrial level.18
The alternative approach to modeling induced innovation is the learning model. This approach has become particularly popular in recent years as models increase the granularity of the technological description down to individual technologies. It has also been attractive in policy studies because it can rationalize early investments in technologies that are presently uneconomical but have the promise, if they can “move down the learning curve,” of being competitive in the future.
The present study examines the analytical and statistical basis of learning models. This is a technical study because the issue that is raised is primarily a statistical issue of identification of learning functions. The basic message, however, is simple. First, the paper shows that there is a fundamental statistical identification problem in trying to separate learning from exogenous technological change. As a result of the identification problem, estimated learning coefficients will generally be biased upwards. Second, we present two empirical tests that illustrate the potential bias in practice and show that learning parameters are not robust to alternative specifications. Finally, we show that an overestimate of the learning coefficient will generally lead to an underestimate of the total marginal cost of output; because of this underestimate, optimization models tend to tilt toward technologies that are incorrectly specified as having high learning coefficients. This implies that policy proposals that rely upon learning models are likely to overestimate the returns to research investments to the extent that they use estimated learning coefficients.
The present note provides the basic results of the study. The full study with tables and figures is available as the background paper cited in the first footnote.
The Fundamental Identification Problem
Models of learning and experience have a long history in studies of manufacturing productivity.19 Because of their perceived successes in technological forecasting, they have recently been introduced in policy models of energy and global warming economics to make the process of technological change endogenous.
This approach has serious dangers. We proceed to examine this issue in three steps. In the present section, we show that there is a fundamental statistical identification problem in trying to separate learning from exogenous
technological change and that the estimated learning coefficient will generally be biased upwards. In the next section, we present two empirical tests to examine the potential bias in practice. In the final section, we show that an overestimate of the learning coefficient will generally lead to an underestimate of the total marginal cost of output and tile policies toward technologies that are incorrectly specified as having high learning coefficients.
The basic idea is that productivity improves or costs decline as workers or firms gain experience with a production process. While there can be little doubt that productivity benefits from experience, the exact mechanism is poorly understood. In particular, it is unclear whether the learning is embodied in individual workers and firms, whether there are interindustry or international spillovers, and whether the improvements lead to durable technological changes, and even whether the learning effects can be distinguished from other technological changes.
In this section, we focus on the problem of identifying differences in productivity due to learning from exogenous changes. We begin by showing why it is impossible without further identifying assumptions to distinguish learning from exogenous technological change, and why the learning coefficient is generally biased upwards. To simplify for this exposition, we assume that all processes are exponential. Output (Qt) is assumed to grow at constant growth rate g, so . Cumulative output at time t (Yt) is therefore:
(1)
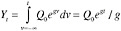
Taking the logarithmic derivative of (1) shows that the growth rate of Yt is g.
The experience curve is assumed to have a true experience coefficient, b. In addition, there is an assumed constant rate of exogenous technological change at rate h. The cost function is therefore:
(2)

“Exogenous technological change” in this context denotes all sources of cost declines other than the learning-curve-determined technological change. It would include inter alia spillovers from outside the industry, the returns to research and development, economies of scale and scope, as well as exogenous fundamental inventions.
Assume that prices are proportional to current instantaneous marginal cost, so the rate of decline in cost (ct) equals the decline in price (pt), which is given by:
(3)

Because marginal cost is constant, price is under these assumptions exogenous to current demand. Demand is determined by a demand function with constant price elasticity (ε), elasticity of per capita demand with respect to aggregate output (λ), where the growth in aggregate per capita output is (Wt), and constant population growth is n. These yield the growth in output (demand) as:
(4)

We can substitute zt= λwt+ n = the autonomous (non-price-induced) growth rate of demand. Solving (3) and (4), we get the following reduced-form equations for the rate of cost (price) decline and the rate of output growth. Since the growth rates are constant, we suppress the time subscripts. Price decline is:

or
(5)

which is the equation for cost (price). The equation for output growth is:

or
(6)

From equations (5) and (6), we can calculate the slope of the behavioral learning curve, β. This is equal to p/g, or:
(7)
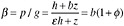
where
(8)

Note how difficult it would be to separate the true learning parameter (b) from the tangle of coefficients in (7).20 To obtain the true learning parameter, we would need to have reliable estimates of the rate of exogenous technological change, the demand elasticity, and the rate of autonomous growth of demand.
Furthermore, the empirical experience parameter will be unbiased (β = b) only when exogenous technological change is zero (h = 0). It will be biased if h ≠ 0. The size of the bias is determined by the sign of (1 − εb). For demand elasticities that are relatively low (less than 4), we would expect that εb > 1, in which case the bias is upwards.
A numerical example will illustrate the result. A typical industry might have a price elasticity of ε = 1, an exogenous demand growth of z = 0.04 per year, and a rate of exogenous technological change of 0.01 per year. With a zero learning effect (true b = 0), we have from (7) that:

In this case, therefore, the empirical learning coefficient is 0.2 even though the actual learning coefficient is zero.
Next, change the assumption to add a true experience curve coefficient of b = 0.25. Then the empirical learning coefficient becomes:

In this case, the empirical experience coefficient is biased upwards from 0.25 to 0.4.
The general conclusion is that because of the interaction of demand, output growth, exogenous technological change, and learning, behavioral learning curves will generally have an upward bias in estimated learning coefficients. The only general case in which the coefficient is unbiased is when exogenous (non-learning) technological change is zero.
Some Empirical Tests
We can take actual data on output and productivity to show the difficulty in measuring learning from historical data. For a first example, we take U.S. data on multifactor productivity in the non-farm business sector for the period 1948-2007. While this is a highly aggregated data set, it has very high quality input and output data that are constructed by U.S. statistical agencies. The data are available at www.bls.gov.
We construct cumulative output for the period 1800 to 2007. These are based on government data for 1929 to 2007 and extrapolate backwards from 1929 using an assumed constant growth rate of 3.9 percent per year. I then estimate bivariate learning functions and exogenous technological change rates. The estimated rate of exogenous technological change for the 1948-2007 period is 0.0105 (±0.00038). A learning equation without exogenous technological change has a learning coefficient of 0.278 (±0.0093). If we combine the two variables, learning and time, the learning coefficient rises to the implausibly high level of 2.1491 (±0.27). The results also show very high autocorrelation of the residuals. For example, the simplest learning model has an estimated first-order serial correlation of the residuals of 0.89 (±0.047). This simple example shows that learning models generally have coefficients that are not robust to specification changes.
As a second example, we estimate learning parameters for 34 major industry groups (see the Bureau of Economic Analysis (BEA) web site for the industry definitions).21 For this purpose, we use data on industry output from the U.S. national income and product accounts. We select only those industries where the output and prices are “well measured.”22
The basic approach is to assume that output is produced by a constant returns to scale production function either with or without learning, as above. Average hourly earnings (AHE) are assumed to represent a reasonable proxy for the cost of production with unchanging technology.23 Prices are proportional to average instantaneous costs, and learning is assumed to be excluded from pricing, or if included to be a multiplicative factor.24 Under these assumptions, the rate of change in the ratio of the AHE to the product price (call this the real price decline) will equal the rate of cost decline given by equation (2). We can then examine the relationship between the real price decline and factors such as learning as represented by cumulative output or time.
The data on output and inputs have been prepared by the BEA and provide both gross output and price indexes for the period 1947-2007 for major industry groups and for 1959-2007 for all other industry groups. For each group, we estimate cumulative output for the initial year as the first-year output index divided by the growth rate for the first 7 years.25
For these data, two results are clear. First, the learning coefficients are wildly variable. Figure 1 [in the paper named in footnote 14] shows a histogram of the empirical learning coefficients estimated from the linearized version of equation (2) with zero exogenous technological change. Clearly, the estimates of the learning parameter are highly variable. For the 34 industries, assuming zero exogenous technological change, only 4 have estimated empirical learning coefficients in the plausible range between 0 and 0.5. The mean and median are well above the plausible range, which suggests a positive bias in the estimation. Additionally, the results are highly sensitive to an AR1 correction, which produce yet different learning coefficients. (See the background paper cited in the first footnote for the detailed results.)
In addition, we estimated the learning coefficients with exogenous technological change [i.e., with h estimated in the linearized version of equation (2)]. The estimates with exogenous technological change are very different from those without exogenous technological change. The correlation between the two estimates is 0.009. Clearly,
21 |
See http://www.bea.gov. |
22 |
The notion of well-measured industries is discussed in William Nordhaus, “Baumol’s Diseases: A Macroeconomic Perspective,” Berkeley Journal of Macroeconomics, Volume 8, Issue 1, 2008, Article 9, pp. 1-37. |
23 |
The reference on the use of wages as a proxy for technological change is analyzed in William Nordhaus, “Baumol’s Diseases: A Macroeconomic Perspective,” Berkeley Journal of Macroeconomics, Volume 8, Issue 1, 2008, Article 9, pp. 1-37. |
24 |
Prices might reflect life-cycle costs if the firm believes that there are learning effects (see for example C. Lanier Benkard, “Learning and Forgetting: The Dynamics of Aircraft Production,” American Economic Review, 2000, vol. 90, pp. 1034-1054). However, as long as the processes are exponential and the learning coefficient is constant, the effect of learning on the ratio of marginal cost to price would be invariant over time. |
25 |
The BEA data are available at http://www.bea.gov/industry/index.htm#annual. |
the estimates are highly sensitive to the specification. These tests show that the estimates of the learning coefficients are not robust to specifications. Moreover, the estimates are often well outside the theoretically acceptable range.
Readers might wonder about whether it is appropriate to use such aggregated data to estimate learning equations. One advantage of the industry data is that we have very carefully prepared indexes of output and price, so the measures are close to the ideal. The appendix in the background study shows the conditions under which elemental processes can be aggregated to determine an aggregate learning function. The results indicate that there are three sources of potential bias. The first bias would arise if the growth rate in individual industries deviated from exponential growth; the second bias relates to the correlation between the learning coefficient and output growth; and the third bias comes from potential biases in productivity measurement.
The Perils of Learning in Optimization Models
Learning has become a favorite tool for modeling technological change in many models of the energy sector and of global warming. It is convenient because learning-by-doing is one of the few “theories” of technological change that is easily included in models because of its simple specification.26 It is a dangerous modeling technique, however, because the estimated learning rates are biased upwards and because these approaches therefore seriously underestimate the marginal cost of output. We showed the first point above and address the second in this section.
The danger in using learning to model exogenous technological change arises when the models select technologies on the basis of their cost characteristics. Learning models have total marginal costs that are lower than current marginal costs because an additional unit of output lowers all future costs as producers move down the learning curve. We can see this point by starting with a total cost function, which is defined as the present value of all current and future production (V0), given by the following:27
(9)

We consider an increment θ of output at time 0, which yields a changed present-value cost as follows:
(10)

Taking the derivative of (10) with respect to current output yields total marginal cost. From equations (5) and (6), we have the growth of output (g) and the decline in cost (p), yielding the total marginal cost as:
(11)

Equation (11) shows that total marginal cost is equal to current marginal cost times 1 minus a “learning discount.” The learning discount is linear in the learning coefficient and the growth rate of output, and is inverse to the dis-
count rate minus the growth of costs. Since all the terms in the discount are positive, this implies that the discount is positive if there is learning.
As an example, suppose that the growth of output is 10 percent per year for a dynamic new product, that the discount rate is 5 percent per year, and that the learning rate is 0.3. Total marginal cost is 63 percent of the current marginal cost. In other words, because an extra unit is produced today, future units get a productivity bonus of 0.37 additional units.
Note that this additional 0.37 units is spread over a huge number of units, so there is little incentive for any individual unit to enter into a Coasean bargain with current producers. However, in a frictionless competitive world without rent-seeking and with perfect information, a Pigovian “learning curve” subsidy of 37 percent would be an efficient policy to induce higher output and move the economy down the learning curve. Another approach, which is often advocated, is for the government to purchase or subsidize early plants so as to stimulate learning.
We can also see the dangers of using learning curves to subsidize early production or choose a portfolio of projects if the learning parameters are incorrectly calculated. Suppose, for example, that the true learning parameter is 0.1 and because of the biased discussed above the estimated parameter is 0.3. With a 3 percent discount rate and a 10 percent growth rate, the learning discount is overestimated by a factor of two. (For a full set of calculations, see the background paper.)
This bias becomes particularly important in energy and global warming models that are designed to choose among different emerging technologies and where the technology is assumed to have an important learning component. For example, suppose that a policy calculation solves for future paths of solar and wind technologies based on current cost and different learning coefficients. Based on high learning rates, the model might suggest that technology A is a good bet for research and development. But this recommendation would be incorrect if the learning coefficient is based on a biased estimate of learning.
The point to emphasize here is that, in analyses that pick technologies on the basis of total discounted cost of production (as is entirely appropriate), then an upward bias in the learning rate can have a major impact on the apparent benefit of technologies with learning. The estimated costs can easily be underestimated by a factor of two. This danger is reinforced because, as shown in the first section, of the tendency to estimate learning rates in bivariate relationships, which will generally lead to strong upward biases in the learning coefficient.