Monitoring and Summative Evaluation of Community Interventions1
Why: Why develop a Community-Level Obesity Intervention Monitoring and Summative Evaluation Plan? Monitoring and summative evaluation of local interventions is critically important both to guide community action and to inform national choices about the most effective and cost-effective interventions for funding, dissemination, and uptake by other communities.
What: What is a Community-Level Obesity Intervention Monitoring and Summative Evaluation Plan? Complementary to the Community Obesity Assessment and Surveillance Plan (in Chapter 7), a Monitoring and Summative Evaluation Plan for community-level obesity interventions is a template to help communities to monitor implementation of the intervention and evaluate the long-term outcomes and population impacts such as behavior change, reduced prevalence of obesity, and improved health.
How: How should a Community-Level Obesity Intervention Monitoring and Summative Evaluation Plan be implemented? A template for customizing plans for monitoring and summative evaluation identifies priorities to accommodate local differences in terms of opportunities for change, context, resources available for evaluating strategies recommended in the Accelerating Progress in Obesity Prevention report, and stakeholder input. Because innovations in obesity prevention often receive their initial test at the community level, rigorous and practical methods are desirable to build national knowledge. Combining knowledge from both experimental studies and practice experience can inform national evaluation by casting light on the prevalence of strategies, their feasibility, and their ease of implementation.
_____________
1 A portion of this chapter content was drawn from commissioned work for the Committee by Allen Cheadle, Ph.D., Group Health Cooperative; Suzanne Rauzon, M.P.H., University of California, Berkeley; Carol Cahill, M.L.S., Group Health Cooperative; Diana Charbonneau, M.I.T., Group Health Cooperative; Elena Kuo, Ph.D., Group Health Cooperative; and Lisa Schafer, M.P.H., Group Health Cooperative.
This chapter presents guidance to develop plans for monitoring and evaluating2 community-level obesity prevention interventions.3 Flexibility in developing community-level monitoring and summative evaluation plans is appropriate given the variety of user needs (as summarized in Chapter 2), local context, and available resources. Monitoring and evaluating community-level efforts to prevent obesity is critical for accelerating national progress in obesity prevention and for providing evidence to inform a national plan for evaluation. Community-level evaluation encompasses the issues of learning not only “what works,” but also the relative feasibility to implement interventions in different situations and the comparative effectiveness of various strategies—the extent to which they work. This information is essential to improving a national plan for evaluation. In line with “what works,” monitoring of the implementation of interventions also informs local implementers on how to improve and manage interventions. It casts light on how and why these interventions may prevent obesity. Finally, it encompasses translating effective interventions for implementation on a broader scale and determining the contexts in which they are and are not effective (i.e., generalizability). This learning will allow greater return on national investments in obesity prevention.
DEFINITION OF COMMUNITY-LEVEL INTERVENTIONS
As described in Chapter 7, the Committee defines community level as activities conducted by local governmental units (e.g., cities, counties), school districts, quasi-governmental bodies (e.g., regional planning authorities, housing authorities, etc.) and private-sector organizations (e.g., hospitals, businesses, child care providers, voluntary health associations, etc.). Communities vary widely with respect to population size, diversity, context, and impact of obesity. Community capacities for monitoring and summative evaluation are also highly variable, with a wide range of expertise and resources for collecting and using data to evaluate the implementation and effectiveness of interventions.
Community intervention monitoring and summative evaluation can be focused on programs, systems, policies, or environmental changes, or any combination of these in multi-faceted initiatives.
• A local program focuses on a specific sub-population of a community, most often takes place in a single setting or sector (e.g., schools), is usually administered by a single organization, and deploys a limited set of services or health promotion strategies. In the past, local efforts focused mostly on counseling, education, and behavior-change programs delivered directly to individuals as well as some broader school-based and community-based programs. Published reports showed modest effects of these programs when done alone (e.g., Anderson et al., 2009; Waters et al., 2011), so the field has moved to incorporating them into more comprehensive or multilevel interventions.
• A community-level initiative is a multi-level, multi-sector set of strategies focused on a defined geographic community or population, and it typically includes policy, program, and environmental changes in different parts of the community (e.g., government, business, schools, com-
_____________
2 As defined in Chapter 1, monitoring is the tracking of the implementation of interventions compared to standards of performance. Evaluation is the effort to detect changes in output, outcomes, and impacts associated with interventions and to attribute those changes to the interventions.
3Interventions refer to programs, systems, policies, environmental changes, services, products, or any combination of these multi-faceted initiatives.
munity organizations). Multi-component mass media campaigns, such as the Home Box Office (HBO) Institute of Medicine (IOM) campaign The Weight of the Nation (TWOTN), that utilize community screenings, learning toolkits, and local events, also fall into this category. Based on experience with control of tobacco and other drugs, multi-component initiatives hold greater potential to prevent obesity than do programs or individual strategies by themselves (IOM, 2012a).
This chapter covers some important considerations for monitoring and summative evaluation that exist across obesity prevention programs and community-level initiatives. The chapter emphasizes the particular challenges and opportunities of community-level evaluation, for which evaluation methods are less well established and evolving.
THE SPECIAL CHALLENGES OF COMMUNITY-LEVEL INITIATIVES
Evaluators have less control over community-level initiatives than they do over research-based programs or nationally guided efforts such as U.S. Department of Agriculture’s (USDA’s) feeding programs and other federal transportation initiatives. This makes the monitoring of implementation essential and the use of rigorous evaluation methods more challenging (Hawkins et al., 2007; Sanson-Fisher et al., 2007). Any evaluation must weigh trade-offs between internal and external validity, feasibility, and ethics versus experimental control, and intrusiveness versus free choice among participants. These decisions become more difficult for initiatives that arise from community decision making (Mercer et al., 2007). For example, communities will institute their own mix of local policies and environmental changes, making random assignment to a particular intervention (program or policy), and thus attribution of cause and effect with outcomes, more difficult. Exposure to certain elements of a community initiative can sometimes be determined by random assignment, but exposure to the entire “package” usually cannot.4 Characteristics of a community influence both the implementation and the outcome of the intervention being evaluated, requiring assessment of community contextual influences (IOM, 2010; Issel, 2009). In general, the field needs to develop efficient and valid methods for community evaluations including the documentation of the unfolding, sequencing, and building of multiple changes in communities and systems over time (Roussos and Fawcett, 2000) and synergies among these changes (Jagosh et al., 2012).
Community-level intervention on policy, environment, and systems is a relatively new approach, and therefore evidence of the effectiveness of most of these strategies is limited. In particular, more empirical evidence is needed about whether, and to what extent, changing food environments promotes healthier eating (Osei-Assibey et al., 2012). There also is some uncertainty about which specific changes in the built environment will lead to increases in physical activity (Heath et al., 2006; Kahn et al., 2002). Appropriate methods are emerging to evaluate community-level impact, but most studies continue to be cross-sectional (an observation made at one point in time or interval) (Booth et al., 2005; Heath et al., 2006; Papas et al., 2007). Several strategies with evidence of effectiveness are listed in the Centers for Disease Control
_____________
4 Although random assignment of communities to entire policies and systems has not, to the Committee’s knowledge, been attempted in the obesity prevention field, both the United States and other countries have randomly assigned place and people to policies in the past, as in the case of the RAND Health Insurance Experiment (Brook et al., 2006) and Mexico’s Seguro Popular experiment (King et al., 2009). Since the 1980s, researchers have randomized entire communities to multi-faceted prevention initiatives, but the experiments are often costly and relatively rare, with limited generalizability (COMMIT Research Group, 1995; Farquhar et al., 1990; Merzel and D’Afflitti, 2003; Wagner et al., 2000).
and Prevention (CDC) Community Guide (Community Preventive Services Task Force, 2013), as well as in “What Works for Health,” a resource associated with the County Health Rankings model of assessing community needs (County Health Rankings, 2013). However, to date, CDC and IOM recommendations for strategies to include in community-level initiatives tend to rely on expert opinion (IOM, 2009; Khan et al., 2009).
Evidence points to comprehensive, community-level initiatives as the most promising approach to promote and sustain a healthy environment (Ashe et al., 2007; Doyle et al., 2006; Glanz and Hoelscher, 2004; IOM, 2009; Khan et al., 2009; Ritchie et al., 2006; Sallis and Glanz, 2006; Sallis et al., 2006), particularly when supported by state or national mass media and other components that communities cannot afford (CDC, 2007). Related work on tobacco control programs, notably from the California and Massachusetts model programs, demonstrated how national and state mass media can support local programs with resources (Koh et al., 2005; Tang et al., 2010).
To address the special monitoring and summative evaluation challenges of community-level initiatives, the Committee commissioned5 a review of published literature, as well as unpublished evaluation studies and online descriptions, to identify initiatives that have been or are currently being evaluated. Cheadle and colleagues (2012) conducted a search for years 2000-2012 using PubMed and websites of agencies that aggregate reports on obesity prevention interventions, such as the Agency for Healthcare Research and Quality Innovations Exchange and the Robert Wood Johnson Foundation’s (RWJF’s) Active Living Research program. The review found 37 community-level initiatives that included sufficient detail concerning their intervention and evaluation methods. These included 17 completed initiatives that included population-level outcome results (3 negative studies, 14 positive) (see Table H-1 in Appendix H). Another 20 initiatives are either in process or do not measure population-level behavior change (see Table H-2 in Appendix H). Some of the largest and potentially most useful evaluations are in progress. In particular, many independent evaluations of CDC’s Communities Putting Prevention to Work initiatives are being conducted; and a large-scale National Institutes of Health–funded Healthy Communities Study is doing a retrospective examination of associations between the intensity of more than 200 community programs and policies and community obesity rates in more than 200 areas across the United States (see Appendix H) (National Heart, Lung, and Blood Institute, 2012).
TOWARD A COMMUNITY-LEVEL MONITORING AND SUMMATIVE EVALUATION PLAN
As noted in Chapter 2 and the L.E.A.D. (Locate evidence, Evaluate it, Assemble it, and Inform Decisions) framework (IOM, 2010), local monitoring and summative evaluation plans should be driven by the information needs of end users and the contexts of decisions, not on preconceptions of what evaluation is about. Common measures of progress are highly desirable, because they permit comparison of interventions and aggregation of studies into a body of evidence. However, uniformity of methods is not desirable, because the contexts of local interventions are so diverse. Moreover, available resources dictate the types of data collection and analysis that are appropriate and feasible. This chapter discusses the choices available within available resources. With the pursuit of more universal agreement on and provisions for indicators and surveillance measures recommended in earlier chapters, more would be available and feasible.
_____________
5 Commissioned for the Committee by Allen Cheadle, Ph.D., Group Health Cooperative; Suzanne Rauzon, M.P.H., University of California, Berkeley; Carol Cahill, M.L.S., Group Health Cooperative; Diana Charbonneau, M.I.T., Group Health Cooperative; Elena Kuo, Ph.D., Group Health Cooperative; Lisa Schafer, M.P.H., Group Health Cooperative.
Tailoring the Plan to End-User Needs
To establish “what works” (effectiveness), outcomes need to be attributed to the community intervention. This requires high-quality measurement and design, consistent with resources and logistical constraints (Shadish et al., 2002). Although rigorous methods are more common in research projects, they are also feasible for community evaluations, and some examples of best practices when conducting evaluations are described in Appendix H. On the other hand, to demonstrate local progress, stakeholders may be satisfied with intervention monitoring and summative evaluation that measures good implementation and an improvement in outcomes, without worrying much about causal attribution to a specific obesity prevention effort. Yet, other purposes lie somewhere between these, as with measures of progress in specific settings and population segments, and these are important for generalizable knowledge. For example, by knowing the particular combination of interventions in particular communities and observing relative improvements in those communities, without being overly strict about causal attribution, the field can better understand the types of interventions (or combinations of interventions) that are most likely to be associated with desired outcomes, their prevalence and feasibility nationally, as well as the dose of environmental change (i.e., strength of intervention, duration, and extent of reach to affect the target population) likely required to achieve them. This information can then inform the priorities for more rigorous tests of effectiveness.
Tailoring the Plan to Available Resources
Almost universally, local monitoring and summative evaluation has limited resources (Rossi et al., 2004). Therefore, evaluation needs to tailor the methods to answer the highest priority questions. Infrastructure improvements as outlined in Chapter 3 may alleviate the situation, but even then most local evaluation budgets are likely to be quite small without the assistance of outside funders.6 Rigorous methods may seem out of reach for many local evaluations, and the cost of data collection can be daunting given the scarcity of local surveillance information. Still, useful evaluation can be conducted, even when expensive data collection is not feasible and methods have limited rigor. As seen below, some relatively simple additions to design and measurement can greatly improve the monitoring and summative evaluation plan, thus adding to national knowledge about community interventions.
Tailoring the Plan to the Intervention Context and Logic
In community-level interventions, the number and kind of strategies are highly diverse and may vary substantially from one initiative to another, as communities implement programs, policies, and environmental changes that address their specific issues and context. Also, there is potential for community engagement to increase over time after community changes take place, thus leading to more community changes. For evaluation, this situation is radically different from conventional programs, in which (ideally) a well-defined linear set of activities is tested, improved, and disseminated for adoption in other locations. This situation poses special issues for planning, design, measurement, and analysis.
_____________
6 In 2012, the Community Transformation Grant awards ranged from $200,000 to $10 million (CDC, 2012). CDC recommended that 10 percent be used for evaluation (Laura Kettel-Khan, Office of the Director, Division of Nutrition, Physical Activity and Obesity, CDC, April 2013).
COMPONENTS OF A COMMUNITY-LEVEL OBESITY INTERVENTION MONITORING AND SUMMATIVE EVALUATION PLAN
The components of a community-level monitoring and summative evaluation plan are seen in a proposed template (see Box 8-1). Within those components, considerable flexibility is needed. The core of any plan includes engaging stakeholders, identifying resources, having a logic model or theory of change, selecting the right focus, using appropriate measures, collecting quality data, using appropriate analytic methods, interpreting or making sense of the data, and disseminating the findings.
BOX 8-1
Components of a Community-Level Obesity Intervention Monitoring and Summative Evaluation Plan
Purpose: To guide community action and to inform national choices about the most effective and cost-effective strategies identified in the Accelerating Progress in Obesity Prevention report for funding, dissemination, and uptake by other communities.
1. Design stakeholder involvement.
a. Identify stakeholders.
b. Consider the extent of stakeholder involvement.
c. Assess desired outcomes of monitoring and summative evaluation.
d. Define stakeholder roles in monitoring and summative evaluation.
2. Identify resources for monitoring and summative evaluation.
a. Person-power resources
b. Data collection resources
3. Describe the intervention’s framework, logic model, or theory of change.
a. Purpose or mission
b. Context or conditions
c. Inputs: resources and barriers
d. Activities or interventions
e. Outputs of activities
f. Intended effects or outcomes
There are many good resources on monitoring and summative evaluation methods, so this chapter does not repeat them (Cronbach, 1982; Fetterman and Wandersman, 2005; Fitzpatrick et al., 2010; Patton, 2008; Rossi et al., 2004; Shadish et al., 2002; Wholey et al., 2010). For example, this report does not include a discussion on analytic methods. Certain issues, however, are central to developing an effective local evaluation of obesity prevention. For this reason, the chapter devotes a good bit of attention to stakeholder involvement, emerging methods, and interpretation of findings.
4. Focus the monitoring and summative evaluation plan.
a. Purpose or uses: What does the monitoring and summative evaluation aim to accomplish?
b. Priorities by end-user questions, resources, context
c. What questions will the monitoring and summative evaluation answer?
d. Ethical implications (benefit outweighs risk)
5. Plan for credible methods.
a. Stakeholder agreement on methods
b. Indicators of success
c. Credibility of evidence
6. Synthesize and generalize.
a. Disseminate and compile studies
b. Learn more from implementation
c. Ways to assist generalization
d. Shared sense-making and cultural competence
e. Disentangle effects of interventions
SOURCE: Adapted from A Framework for Program Evaluation: A Gateway to Tools. The Community Tool Box, http://ctb.ku.edu/en/tablecontents/sub_section_main_1338.aspx (accessed November 12, 2013).
Designing Stakeholder Involvement
Some commonly identified stakeholder groups include those operating the intervention, such as staff and members of collaborating organizations, volunteers, and sponsors, and priority groups served or affected by the intervention, such as community members experiencing the problem, funders, public officials, and researchers. Some stakeholder groups are not immediately apparent, and guidance on the general subject is available (e.g., Preskill and Jones, 2009). Two aspects are specifically important for planning community-level obesity prevention monitoring and evaluation: community participation and cultural competence.
Community Participation in Obesity Monitoring and Summative Evaluation Plans
Community participation is beneficial for the planning of most program monitoring and summative evaluation; it is essential for the evaluation of community-level initiatives. Yet, in the commissioned literature review of 37 community-level evaluations, only 6 mentioned participation at all and that was in the context of the intervention rather than the evaluation (see Appendix H). As seen in Chapter 2, community coalitions are often the driving force behind community-level initiatives. Community engagement and formative evaluation are critically linked. Without community engagement, the community may have inadequate trust in the evaluation process to make strategy improvements based on evaluation findings and recommendations. Community participation may also facilitate access to data, not only qualitative but also quantitative data kept by organizations and not available to the public, that evaluators would otherwise not be aware of or able to collect. Other benefits have been well described. The primary disadvantages include time burden on community members and a lack of skill in community engagement on the part of many evaluators (Israel et al., 2012; Minkler and Wallerstein, 2008).
Participatory approaches to community monitoring and summative evaluation reflect a continuum of community engagement and control—from deciding the logic model and evaluation questions to making sense of the data and using them to improve obesity prevention efforts. In less participatory approaches, the evaluator has more technical control of the evaluation (Shaw et al., 2006). In more participatory approaches, communities and researchers/evaluators share power to a greater extent when posing evaluation questions, making sense of results, and using the information to make decisions, although there may be trade-offs with this approach, too (Fawcett and Schultz, 2008; Mercer et al., 2008).
The Special Role of Cultural Competence in Obesity Monitoring and Summative Evaluation Plans
As noted in Chapter 5, there is a national urgency to evaluate and address the factors that lead to racial and ethnic disparities in obesity prevalence. Community interventions to address such disparities require cultural competence in both the interventions and their evaluations. Participatory methods facilitate the use of cultural competence.
The American Evaluation Association (2011) states: “Evaluations cannot be culture free. Those who engage in evaluation do so from perspectives that reflect their values, their ways of viewing the world, and their culture. Culture shapes the ways in which evaluation questions are conceptualized, which in turn influence what data are collected, how the data will be collected and analyzed, and how data are interpreted” (Web section, The Role of Culture and Cultural Competence in Quality Education).
Ethical, scientific, and practical reasons call for culturally competent evaluation: ethical, because professional guidelines specify evaluation that is valid, honest, respectful of stakeholders, and considerate of the general public welfare; scientific, because misunderstandings about cultural context create systematic error that threatens validity; and cultural assumptions, because the theories underlying interventions reflect implicit and explicit assumptions about how things work.
The practical reason to consider culture in evaluating of obesity prevention efforts is that the record is mixed about the effectiveness of cultural competence in health promotion programs (e.g., Robinson et al., 2010). Culturally competent evaluation can help the field to address this mixed result by assuring that interventions are, in fact, consistent with a population’s experience and expectations. Evaluation has demonstrated the effectiveness of cultural tailoring in some areas (Bailey et al., 2008; Hawthorne et al., 2008). Culturally tailored media materials and targeted programs reach more of the intended population (Resnicow et al., 1999). Culturally competent evaluation can assess whether interventions focus on issues of importance to the cultural group; whether interventions address where and how people eat, shop, and spend recreational time; and which environmental changes produce the most powerful enablers for more healthful nutrition and physical activity.
Identifying Resources for Monitoring or Summative Evaluation
Monitoring and summative evaluation plans can maximize resources in two areas, person-power and data collection. Regarding person-power, evaluations can draw on the expertise of local colleges and universities and of health departments, which will generally improve evaluation quality and potentially lower the cost. Faculty in schools offering degrees in health professions are often required or encouraged by accrediting bodies to provide community service, which they often do through evaluations. Students will find evaluation projects suitable for service-learning opportunities and internships. For example, the Council on Education for Public Health requires that tenure and promotion strongly consider community service and that student experiences include service learning with community organizations (Council on Education for Public Health, 2005). Free services are not always high-quality services, however, and may lack consistency and follow-up. The Community-Campus Partnerships for Health offers useful guidance for maximizing the quality of evaluation activities provided as service (Community-Campus Partnerships for Health, 2013). The guiding principles for evaluation outlined in Chapter 3, which are endorsed by researchers’ professional associations, can also help.
Data collection is generally the highest-cost component of evaluations. Using available information where applicable, such as local surveillance and other community assessment and surveillance (CAS) data, can minimize the cost. Making data collection a by-product of prevention activity can also lower cost, as in the collection of participation rosters, media tracking, and public meeting minutes. Community resident volunteers can collect data using methods such as photovoice (see Chapter 7 and Appendix H) and environmental audits,7 thus adding both person-power and data.
_____________
7 Observations to identify interventions being implemented in a particular area.
Describing the Intervention Framework, Logic Model, or Theory of Change
Frameworks, logic models, and theories of change are heuristics—experience-based techniques for problem solving, learning, and discovery designed to facilitate and guide decision making. A logic model is not a description of the intervention itself, but rather a graphic depiction of the rationale and expectations of the intervention. A theory of change is similar to a logic model except that it also describes the “mechanisms through which the intervention’s inputs and activities are thought to lead to the desired outcomes” (Leviton et al., 2010b, p. 215).
For the monitoring and summative evaluation plan, one ideally can turn to the logic model or theory used in the planning of the program, but often this was not developed or made explicit in the earlier program planning, and must be constructed retrospectively.
There are many options to choose from among formats for logic models and theories of change. The choice depends on what will have the most clarity and ease of presentation for the user audience (Leviton et al., 2010b). Figure 8-1 illustrates a graphic depiction of the presumed components and causal pathways in local-level obesity prevention efforts. Not all evaluations will include all the elements or all the pathways, which is to be expected in areas with such diversity of local initiatives. Building on Figure 8-1, Table 8-1 provides some detail on generic logic model components, with the potential program components listed in the first row and potential community-level components in the second row. Outputs and outcomes resulting from programs are also commonly seen in multi-faceted community initiatives.
In building logic models, the components must be clarified. Although not appearing in the table, the purpose or mission describes the problem or goal to which the program, effort, or initiative is addressed, and context or conditions mean the situation in which the intervention will take place and factors that may affect outcomes. Inputs represent resources such as time, talent, equipment, information, and money. Inputs also include barriers such as a history of conflict, environmental factors, and economic conditions. The activities are the specifics of what the intervention will do to affect change and improve outcomes, while outputs are direct evidence of having performed the activities, such as products or participation in services by a target group. Activities and outputs are logically connected to short-, intermediate-, and long-term outcomes: for example, engagement of local decision makers is presumed to help to achieve
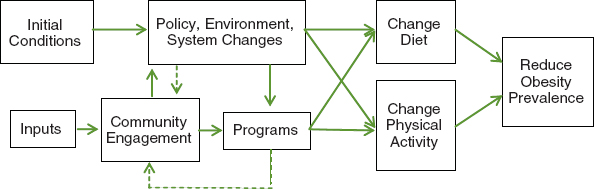
FIGURE 8-1 Generic logic model or theory of change for community obesity prevention.
NOTES: Not all interventions will include programs, policies, and environmental changes or systems changes. Not all interventions will focus on both diet and physical activity. Dashed lines indicate potential for interventions to increase community engagement over time.
TABLE 8-1 Generic Logic Model for Community-Level Initiatives to Prevent Obesity
Inputs | Outputsa | Outcomes (Impact)a | ||||
Short term | Intermediate | Ultimate | ||||
Program Components |
Initial:
• resources • staff • public opinion • community norms |
• program activities • outreach • media messages |
• target group program participants |
Changes in:
• knowledge • attitudes |
Improved:
• physical activity • diet |
|
Multi-Faceted Initiatives |
• public opinion • community norms • policies identified • policy opportunities • advocacy allies |
• decision makers engaged • public meetings attended • community organized • advocates recruited and trained • enforcement of changes monitored |
Increases in:
• public support • resources • advocacy, allies, and power |
Changes in:
• policies • environments • systems |
• changes in community norms • change sustained in environment • change sustained in policy and system |
Improved:
• prevalence of obesity and overweight • population health |
a Outputs and outcomes resulting from program components are also commonly seen in the multi-faceted initiatives.
changes in policy and environment, which are presumed to change diet or physical activity and, therefore, help to achieve healthy weight for a greater portion of the population.
Logic models and theories of change help greatly to assess the plausibility that particular interventions can achieve their goals. Is it plausible—believable—that the connecting arrows of a logic model or the assumptions of a theory are likely to produce the outcomes predicted? Evaluating implausible interventions wastes resources and is needlessly discouraging for the field. Logic models and theories of change also cast light on the “dose” of intervention (i.e., intensity, duration, and reach) that is likely to be necessary to achieve change. The low-cost technique of evaluability assessment helps to establish plausibility, indicates which intervention components are ready for evaluation, and pinpoints areas for improvement in implementation or the mix of strategies involved (Leviton et al., 2010b).
Focusing the Obesity Monitoring and Summative Evaluation Plan
The framework, logic model, or theory of change helps to focus the monitoring and summative evaluation plan: what the evaluation aims to accomplish. By prioritizing based on user needs, resources, and context, the choices often become very clear. Limited resources do not have to imply reduced rigor, and below, in the section titled “Planning for Credible Methods,” some suggestions are offered to improve rigor.
The Importance of Focusing on Outputs and Short- and Intermediate-Term Outcomes
A good local monitoring and summative evaluation plan requires a dose of realism. People tend to focus automatically on health impacts such as a change in obesity prevalence, obesity-related diseases such as diabetes, or perhaps in diet and physical activity. Yet in local evaluations, this focus may be premature or overly ambitious, insofar as it may take years for changes in behavior and health to become apparent. Certainly, it is essential to learn “what works” to achieve health improvements, but a premature rush to evaluating behavior and health outcomes can lead to negative findings, with a chilling effect on innovation (Shadish et al., 1991).
Most community plans should focus monitoring and summative evaluation earlier in the logic model sequence than they do. This could be done instead of, concurrently with, or in preparation for, assessing behavior and health outcomes. Program evaluation should focus first on monitoring adequate implementation and dose (i.e., strength or intensity of intervention, duration, and reach); and community-level initiatives should focus on achieving the amount and kind of policy, environment, or systems changes sufficient to achieve population-level outcomes (dose). As an example, the 2008 Physical Activity Guidelines Advisory Committee has determined that 60 minutes of daily physical activity prevents childhood obesity (HHS, 2008); an intervention to increase daily physical activity can be well evaluated by monitoring implementation of the policy or program or measuring the minutes of physical activity. Although assessing weight changes may be desirable, it is not necessary and may not be feasible or affordable in the short term.
There are other reasons for community evaluations to focus earlier in the sequence of activities, outputs, outcomes, and population impacts. Most local evaluations are of short duration, so effects on behavior or health outcomes might not be seen during the evaluation time period. Without local or statewide surveillance systems, the cost of measuring behaviors and obesity is often prohibitive. But building knowledge depends on gaining local experience with the short- and intermediate-term outcomes, which will tell us whether the dose of intervention was likely to be sufficient to achieve a population-level change in behaviors or obesity (see below). This challenge also applies to mass media programs or campaigns, such as the HBO/IOM TWOTN.
Monitoring and documenting implementation will assist in program improvement and assessing progress toward achieving system, policy, and environmental changes. Methods such as empowerment evaluation (Fetterman and Wandersman, 2005) and the Getting to Outcomes framework (Chinman et al., 2008) are especially helpful for program improvement, because they focus on the needs of implementers and on strategy for improvement. Assuring implementation is a particular problem in evaluating change in institutions such as school districts and large worksites where obesity and related health issues are not a priority, or where changes are distributed across several sectors (such as education, child care, or transportation), organizations, or organization subunits such as parks or schools within a district. In the community measurement approach developed by the University of Kansas (Fawcett and Schultz, 2008), community and evaluation partners use key informant interviews and report reviews to document and score instances of community/system changes (i.e., programs, policies, practices, built environment) and to characterize aspects related to their dose (e.g., strength of change strategy, duration, and reach; sectors and levels in which implemented).
Assessment of Local Assets and Resources
A local obesity prevention policy monitoring system can not only spark and inform local policy development efforts, but also enhance policy evaluations (Chriqui et al., 2011). Such a system monitors the adoption and implementation of policies over time, includes specific criteria to rate the strength of policies, and describes the number of people affected by the policy (reach). However, few if any communities have such a monitoring system or the capacity to develop one. Table 8-2 summarizes issues to consider when designing a policy-monitoring system. Data on the built environment and status of policy adoption and implementation is generally not found in routinely collected data. Built environment audits and local policy-monitoring systems can fill this gap.
Data on the built and food environment can stimulate local action and also provide important data for evaluation and research (Brownson et al., 2009; Glanz, 2009; Sallis, 2009; Sallis and Glanz, 2009). These data can be used to, for example, determine the presence or absence of an environmental feature (e.g., a park or grocery store); assess the quality of the feature; document disparities in access; and evaluate progress (IOM, 2012b).
Three categories of environmental data are being used: (1) perceived measures obtained by telephone interview or self-administered questionnaires; (2) observational measures obtained using systematic observational methods (audits) that are collected “objectively” and “unobtrusively” (Cheadle et al., 1992; Saelens et al., 2003); and (3) archival data sets that are often layered and analyzed with geographic information systems. An emerging audit method is the use of remote assessment, such as Google Earth (Rundle et al., 2011; Taylor et al., 2011). These data can be used to drive public health actions at the local level (Fielding and Frieden, 2004). For example, collection of local data (bicycle and pedestrian counts) in Columbia, Missouri, has helped to document the effects of built environment changes (e.g., improved street design, sidewalks around schools, activity-friendly infrastructure) (Sayers et al., 2012).
Special Issues for Community-Level Initiatives
Multi-component community-level interventions have the special challenge of complexity. Interventions occur at several social-ecologic levels and with activities spanning the full spectrum of community change, from development of policy, systems, capacity, and infrastructure change to resulting changes in environments and programs. Specifying which combination of interventions is optimal for health effects is not possible in most community-level evaluations—evaluating many sites using different combinations of interventions offers our best chance to determine “what works” in combination.
Local evaluation planners, however, have several options to cope with complexity. Most evaluations collect far more data than will be analyzed or used, so simplicity may be a virtue even in evaluation of community-level initiatives. One approach is to invest more resources in evaluation of a specific component of a multi-strategy initiative, rather than trying to evaluate all of them. The choice depends on the logic model, because it allows the plan to consider components that are
• ready for evaluation;
• more likely to have an impact in the time frame of the evaluation;
• plausible to achieve sufficient “dose” to change behavior, environments, or health outcomes;
TABLE 8-2 Considerations When Designing a Local Monitoring System
Consideration | Key Factors |
System purpose |
• To understand the policy adoption and/or implementation process • To catalog on-the-books policies to assess policy change, readiness, implementation, and/or impact • To monitor changes in policy adoption over time • To facilitate multi-site collaborations across jurisdictions with similar policy surveillance systems |
Policy jurisdiction |
• County • Municipal • School district |
Policy type |
• Legislative (e.g., ordinances, resolutions) • Administrative (e.g., regulations, executive orders) • Judicial decrees (e.g., case law) • Policy documents without the force of law (e.g., master or comprehensive plans, agency internal policies and memos, position papers, etc.) |
Periodicity |
• Will the system include only prospective policy adoption or changes or will it include retrospectively adopted policies? • How often will updates be conducted (e.g., weekly, monthly, quarterly, annually)? |
Policy status |
• Introduced/proposed policies • Enacted policies • Policies effective as of a certain date |
Type of data to include |
• Quantitative data documenting the policy presence/absence and/or the detailed policy elements (e.g., policy scope and strength) • Qualitative data describing the policy content (including, possibly, keywords for searching the system) |
Policy coding |
• For policy surveillance systems, how will the policies be evaluated to assess the scope and strength? —What will be the scientific basis for measures of the scope and strength of the policies? • Will the coding scheme allow for the coding of new, innovative policy approaches? |
Inclusion of policy process data |
• Barriers and facilitators to policy adoption and/or implementation —Role and resources of key stakeholders/champions —Local contextual factors (e.g., socioeconomic and demographic factors, political climate, industry influences, etc.) —Costs —Key factors influencing or inhibiting adoption and/or implementation |
Inclusion of policy outcome/impact data |
• Quantitative and/or qualitative data on the impact of a given policy • Short-, intermediate-, and long-term indicators of policy impact • Unintended consequences of the policy (positive and negative) |
Resource availability and constraints |
• Ongoing funding versus one-time funding to support the staffing required to develop and maintain the system over time —Data systems/programmer support —Policy analyst support —Evaluation research support |
SOURCE: Information summarized from Chriqui et al., 2011.
• innovative so they add to national knowledge, or might become part of a cross-site evaluation; and
• more likely to be institutionalized or maintained over time.
It is quite feasible to conduct rigorous studies of intervention components this way, and for reasonable cost. The Food Trust8 is using this approach in two current randomized experiments, evaluating the effects of placement of healthy foods in Philadelphia’s corner stores and offering price incentives to consumers of whole milk to try low-fat and skim milk (Personal communication, Allison Karpyn, Director of Research and Evaluation, The Food Trust, April 3, 2013).
Planning for Credible Methods
Criteria for Credible Methods
A plan for credible evaluation needs to consider both scientific credibility and face validity or clarity for stakeholders. Some best practices for both scientific credibility and face validity are well within reach of local evaluators, and examples are presented in Appendix H. Scientific credibility means using the highest quality measures recommended in the National Collaborative on Childhood Obesity Research Registry that are also consistent with resources and local expertise, and a design that is appropriate for the questions to be asked. Even better would be the more consistent use of common measures for policy, environmental, systems, and behavioral changes, because they permit comparison across communities and interventions.
Indicators will only have credibility with stakeholders if they agree to them as accurate reflections of intervention reality and what would constitute intervention success. In particular, community-level initiatives may garner some opposition, so the face validity of measures deserves strong consideration. In the same way, credibility is enhanced by transparency about the design and analysis.
Planning for Measurement and Data Collection
For the measurement and monitoring of implementation, evaluators need to balance information against respondent burden and intrusiveness, as well as cost. Monitoring of implementation will be most complete and successful if it provides useful feedback to program implementers themselves (Rossi et al., 2004). Policy and system changes often require advocacy or community organizing. To analyze and track progress in this area, the technique of Power Analysis (Pateriya and Castellanos, 2003) is useful. Power Analysis identifies, for any community organizing effort, who is for, against, or neutral about a suggested change, as well as their power to affect the success of the effort. Changes over time in the array of allies, opponents, and their power over the situation are highly revealing and helpful to community organizers.
Issues around the measurement of changes in knowledge, attitudes, and community norms are well-covered elsewhere (Fishbein and Ajzen, 2010). Prevention programs generally need to pay more attention to the measurement of cost, given the importance of this issue to many potential users of evaluation. Lucid, helpful resources are available for this purpose (Haddix et al., 2002). Given the response burden in measuring costs that many prevention projects experience, as well as the variation in costs based on the
_____________
8 See http://www.thefoodtrust.org/index.php (accessed November 12, 2013).
region of the United States (New York versus the rural south, for example), it might be helpful to document the staff time, material and other resources, and volunteer effort, rather than the actual dollar cost (IOM, 2012b). This would permit local users elsewhere in the nation to not only understand the level of effort and resources needed, but also consider the costs based on local conditions.
For evaluating changes in policy, environment, or systems, recommended tools are available through online inventories (see Table H-3 in Appendix H for a partial list), but instruments have proliferated and can be quite lengthy and complex (Saelens and Glanz, 2009). For most evaluations, it is helpful to use shorter measures that focus on aspects of the environment most closely related to the strategic aims of intervention, rather than use more comprehensive tools. For instance, to evaluate the effects of the Kansas City Chronic Disease Coalition (a CDC Racial and Ethnic Approaches to Community Health [REACH] 2010 initiative), Collie-Akers et al. (2007) documented, characterized, and graphically displayed the unfolding of community/ systems changes over time; and this composite measure of the comprehensive intervention was associated with changes in diet among African American women in this low-income community.
Some of the indicators generated through CAS are useful for initiative evaluations if they capture environmental or policy changes appropriate to the intervention(s). These data can provide a low-cost alternative to primary data collection, comparison communities, and a longer time frame of available data. Mixed-methods approaches that combine quantitative and qualitative methods are recommended where possible. Photovoice and digital story telling using videos are particularly helpful in enabling the cultural groups most affected by the obesity epidemic to document and evaluate efforts on their behalf (Hannay et al., 2013; Wang et al., 2004). Where resources are very low, the monitoring and evaluation plan might consist of a retrospective assessment, using key informants to report on changes, supplemented by photos or other documentation. Documented qualitative changes, accompanied by specific examples and photos can be especially powerful in engaging community and providing pilot data that can be used to expand efforts and obtain additional funding for evaluation.
Monitoring and summative evaluation of behavioral and weight outcomes is challenging, given the time and resource limitations of most community interventions. “Gold standard” methods to capture changes in food and caloric intake patterns and minutes of physical activity, such as multiple 24-hour recalls and accelerometer studies, are labor intensive and time consuming. Anthropometric assessment of body mass index (BMI) is a reliable measurement technique when conducted by well-trained staff who follow rigorous protocols (Berkson et al., 2013), but it is expensive, especially outside of health care or institutional (e.g., schools) settings. BMI and behavioral measures can also be collected with self-reported measures using brief phone and/or paper surveys, but collecting primary survey data is costly. Existing secondary data (e.g., the Behavioral Risk Factor Surveillance System) in most parts of the United States lack sufficient respondents at the county or neighborhood level. Self-reported assessment of food intake, physical activity, height, and weight can be challenging in children (Dietz et al., 2009) and certain populations (e.g., elderly, racial/ethnic groups with language barriers) (IOM, 2012b).
Community interventions seek population-level impact, which presents two additional data collection challenges. First, they must sample and measure health behaviors and outcomes at the population level. This is a challenge, in part because we presume that community-level interventions will have small effects given the array of factors that shape physical activity and dietary behaviors (Koepsell et al., 1992; Merzel and D’Afflitti, 2003). Yet, even small effects from community-level changes will have importance at the population level (Homer et al., 2010). Unfortunately, small population-level changes are difficult
to detect because the measurement and sampling error associated with population-level surveys require large sample sizes, which are costly to collect (Atienza and King, 2002; Koepsell et al., 1992; Merzel and D’Afflitti, 2003). In addition, it is difficult to obtain response rates that are representative of the entire population of a community without a substantial investment in multiple contacts to obtain completed surveys (Bunin et al., 2007; Curtin et al., 2005). In the absence of local-level surveillance information, a lower-cost option may be developing proxy measures or reasonable markers for population-level outcomes: for example, changes in the prevalence of obesity in children by measuring BMI in all 4th, 8th, and 11th graders as a marker of changes in food consumption (Hoelscher et al., 2010; personal communication, Allison Karpyn, Director of Research and Evaluation, The Food Trust, April 3, 2013).
The second challenge concerns the length of follow-up. It is likely that community environmental and programmatic changes must be sustained over a long period of time for significant population-level impact. Most primary population-level data collection, however, is constrained by the funding period of the initiative, with data collection ending at or soon after intervention funding stops. To have a reasonable chance of detecting longer-term changes, some of the data collection resources must be shifted to one or more years beyond the initiative period, which means reducing sample sizes at each data collection occasion or finding more inexpensive, less comprehensive methods that permit a larger overall number of surveys over longer periods of time. One advantage of logic model designs (see below in the next section) is that they focus long-term follow-up data collection only in those communities where the dose or extent of community changes suggests there is likely to be an observed impact. Or, inversely, they can focus retrospective collection of data on implementation or intermediate outcomes of community policies, programs, and environmental changes on those communities for which improvement in health outcomes were noted.
Planning for Design and Analysis
The local monitoring and summative evaluation plan needs to consider designs and analyses that are suitable to end-user questions. In many cases, the question “what works?” cannot be answered by the most common or feasible designs. In line with the L.E.A.D. framework (IOM, 2010), causal attribution is not always necessary; it is not the only useful question to be asked. Even the weaker designs may be sufficient to demonstrate progress for local decision makers. Many designs are useful for monitoring implementation and delivering an intervention. Many designs can help to reduce uncertainty about which interventions are most promising or powerful for obesity prevention for subsequent more-definitive evaluations. With a few simple additions, weaker designs can become substantially stronger for assessing effectiveness as the program investment rises. In this section, various designs are presented as a range of options for consideration in line with end-user purposes.
Randomized experiments, whether at the individual or cluster level (e.g., schools, worksites, or randomly assigned communities) remain the gold standard for attributing outcomes to a program or community-level initiative. The methods for experiments are well established, but they are more suited to research than to most community-level evaluations. Testing what works for complex community-level initiatives is challenging given the cost and difficulty of randomizing entire communities (Merzel and D’Afflitti, 2003), and, as a result, the field has moved away from a focus on multi-site studies to a focus on individual communities. There are still group-randomized trials at levels less aggregated than community (e.g., schools, worksites) and at least one retrospective, non-experimental study is currently under way (see Appendix H, Healthy Communities Study).
The pre-/post-intervention design is most commonly used in single-community evaluations. Although it can be useful for other purposes, it is not adequate to assess effectiveness. In line with the L.E.A.D. framework (IOM, 2010), such evaluations may be enough to satisfy local decision makers that progress is being made. Unfortunately, for determining “what works” this design suffers from threats to validity that offer many plausible alternative explanations for any observed changes in behavior or health status (Shadish et al., 2002). An alternative explanation based on selection bias, for instance, is that citizens may be predisposed to engage in healthful behavior; consequently they also demand, and obtain, health promotion programs, environmental changes, and relevant policies. Secular trends may also be responsible for change, in that increasing attention to obesity prevention over time may produce behavior changes, increased programs, and changes in policy and environment to meet citizen demands. Other alternative explanations include local history (i.e., something else happened in the intervention community concurrent with the intervention itself), seasonality (i.e., influencing changes in food intake and physical activity behavior), and, potentially, regression to the mean. The bottom line is that no pattern of change or lack of change in any outcome at a single site can be interpreted as a causal statement in the absence of a comparison group, given the range of predictable, regularly occurring alternative explanations.
The nonequivalent comparison group design is stronger than the single-group pretest-posttest design for community-level interventions. In this design outcomes are measured pre- and post-intervention in intervention communities and carefully selected comparison communities. The evaluation of Shape up Somerville, often cited as a successful community-level initiative, is an example of this approach and employed a single community with two comparison communities (Economos et al., 2007). However, results from nonequivalent comparison group designs are still subject to alternative interpretations. The most obvious is that the comparison group is, by definition, nonequivalent, and any measured or unmeasured differences between program and control sites could explain any differences in outcomes. Evaluators may attempt to adjust statistically for initial differences. However, error connected with measurement can actually introduce statistical bias, and it will not be clear whether the findings were over or under adjusted (Shadish et al., 2002).
Logic model designs start with a theory of change about the mechanism by which the comprehensive community initiative is intended to achieve its long-term outcomes and then create indicators for each step in the logic model. If the temporal pattern of change is consistent with that specified in the logic model, and if intermediate outcomes specified in advance are plausibly related to the outcomes, then the intervention is more likely to have been the cause of the population-level changes. These designs are more definitive about causal attribution than the pre- and post-intervention designs, although the results can still be open to alternative explanations, in particular, selection bias. Nevertheless, with a myriad of potential factors affecting obesity, logic model designs are useful to identify the strategies that are most likely to have power for prevention. They are useful for the complexity and emergent nature of community-level initiatives, and, in various ways, they inform the field about the amount and kind of community/system changes—and associated time and effort—that will be required to achieve results. Two examples appear in Appendix H.
The advantage of logic model designs is that they are more “specific,” that is, better able to rule out false positives where a favorable population-level change occurred that was not the result of the initiative. Thus, if a behavioral outcome improves but there are no corresponding community changes, or if the intervention does not have a sufficient dose (i.e., strength of intervention, duration, and reach), then it is
much harder to conclude that the intervention was responsible for the observed positive outcome. A challenge of the logic model approach is that it requires an accurate assessment of the amount and kind (dose) of changes in the community/environment (e.g., Collie-Akers et al., 2007). Intervention intensity, duration, and fidelity have been found to be associated with size of effects in other evaluation fields, and they are widely recognized as important concepts, although the concept of reach is not always addressed (Hulleman and Cordray, 2009).
By adding even a few design features, evaluations become stronger to assess effectiveness. Even two pre-intervention measurements, rather than a single baseline measurement, can help to reduce uncertainties about secular trends in behavior or health outcomes and increase reliability of measures. With local- or state-level surveillance systems, it may even become feasible to use short interrupted time series (or multiple base line designs), a far preferable design that helps to control for several alternative explanations (Shadish et al., 2002). Causal modeling, also called path analysis, builds on the logic model approach by establishing that an intervention precedes the outcomes in time, then applies regression analysis to examine the extent to which the variance in outcomes is accounted for by the intervention compared to other forces. The “population dose” approach also uses causal modeling in analysis, but causal modeling can be used independently of dose measurement—it is a statistical control concept (see Appendix H for additional information on the “population dose” approach). Although it confines itself to examining associations, the Healthy Communities Study is an especially rigorous example of causal modeling in that it includes measures of both the amount and intensity of community programs/policies (the dose) and childhood obesity rates (the intended outcome) (see Appendix H). Finally, the regression-discontinuity design rules out most alternative explanations and provides similar estimates to those of experimental designs, provided that its assumptions are met (Shadish et al., 2002). Yet it is under-utilized in prevention research (see Appendix H for an example of regression-discontinuity design).
Synthesis and Generalization
Disseminating and Compiling Studies
Understanding the extent of community-level changes required to bring about health outcomes is the first step toward generalized knowledge and spread of effective prevention. Local evaluations are vital to this process, because there will be some overlap in the mix of intervention components, creating potential to identify the ones with power to effect change. Yet compiling and synthesizing the results of local evaluations are challenges, for at least two reasons. First, measures of policy, environment, and even behavioral changes are not yet collected using commonly accepted measures that can be compared and synthesized. Cost information is rare, although recent federal efforts in Community Transformation Grants (CTGs) and Communities Putting Prevention to Work (CPPW) may soon cast light on the issue of resources necessary for these efforts. Second, website locations for an end user of evaluation to visit and find the desired information are in flux—the Cochrane Collaboration and the Task Force on Community Preventive Services are the main repositories for systematic reviews, but their emphasis on strength of evidence tends to underrate the weight of evidence from evaluations conducted under less controlled conditions. Evaluation results are scattered in peer-reviewed and non-peer-reviewed publications, across many websites and at presentations at multiple conferences. In the interest of generalized knowledge, more needs to be done to aggregate study findings about what combinations of strategies work and under what conditions.
If studies using various designs (e.g., multiple baselines, causal modeling, regression-discontinuity, pre-post measurement, and nonequivalent comparison communities) all reach the same conclusions about behavior change, then this is to the good. Heterogeneous studies provide a stronger inference about causation than do a large number of studies that are all vulnerable to the same alternative explanations. The availability of multiple single-community evaluations suggests that building the evidence base about community-level interventions will depend on many evaluations of individual strategies—or combinations of strategies—rather than a handful of large-scale experimental or quasi-experimental studies. Finally, disparate findings from multiple evaluations can offer insight as to the applicability of some interventions for some populations and the inappropriateness of those same interventions for other populations (Green and Glasgow, 2006).
Learning More from Implementation Monitoring
A wealth of local evaluation information will likely become available from CDC’s CPPW, REACH, and CTG initiatives, as well as other national initiatives with multiple local sites. Yet, there is no central forum or repository to understand barriers and facilitators to implementation, assess costs and cost-effectiveness of alternatives, or to gain an improved understanding about what can be implemented for a given amount of time and resources, and what can be learned from important variations in implementation. It is critically important to synthesize and assemble this information as these large national initiatives conclude. They are essential for translation and scaling up, as well as for generalizability about the effectiveness of obesity prevention.
Although evaluation generally looks to outcome studies to understand generalizability, this conventional interpretation is not sufficient (Green and Glasgow, 2006). The conventional view is that one must accrue randomized controlled trials or at least quasi-experiments to establish external validity (Shadish et al., 2002). Yet, this view ignores issues concerning feasibility, cost, and context of implementation of the same intervention in diverse settings. Practitioner knowledge about what is or can be implemented in a given setting, not to mention their special knowledge of the population’s preferences and current knowledge, attitudes, and behavior, is essential. Generalizability also means reducing uncertainty about what will work in a given setting, population, and with available resources (Cronbach, 1982). This information is potentially available from the evaluation reports regarding government investments—such as CPPW, CTG, and REACH—as well as foundation initiatives.
Using Common Outcome Measures to Assist Comparisons and Generalizability
Preventing obesity requires an adjustment of daily calorie intake and expenditure, changes that can be achieved through many policy and environmental changes (Wang et al., 2012). As more is learned about the policy, environmental, and systems changes that lead to behavior and weight changes, it may eventually become possible to project these outcomes for local evaluations rather than measure them directly. However, this can only happen if the measures employed in research and evaluation become commonly accepted and used and are translatable into calories ingested or expended. Comparable cost measurement is also vitally needed. If these improvements were made, then strategies could be compared for their effectiveness and cost-effectiveness. With more systematic attention to measuring costs and outcomes in a commonly accepted fashion, obesity prevention could achieve the same ability to translate interventions to the bottom line for health, cost-effectiveness, and quality of life that the health sector has
seen for hypertension control (Weinstein and Stason, 1976) or HIV prevention (Farnham et al., 2010). In fact, Wang et al. (2012) developed the energy gap framework to estimate the effects on childhood obesity of a wide range of prevention activities. They reviewed the literature on interventions affecting youth diet, activity levels, energy balance, and weight, examining calorie intake or expenditure where this information was available, and also estimating the reach of the interventions. They have developed a Web-based tool9 to allow users to project the impact of policy, environmental, and program changes on childhood obesity at the population level.
Shared Interpretation of Results and Cultural Competence
The Committee acknowledges that each community is unique in its aims, context, and broader determinants of health. Yet, across communities, when local people, such as those experiencing health disparities consistently point to preferences for particular obesity prevention strategies, when they “vote with their feet” for participation and engagement, or when they consistently interpret community conditions such as built environment features in particular ways, then it behooves evaluators to listen. Methods are available to synthesize and interpret qualitative data such as photovoice and focus groups (Yin and Heald, 1975), and these can be combined in mixed-method studies to better understand outcomes and address disparities in obesity for the most vulnerable populations (Yin, 2008). Participatory evaluation approaches provide an opportunity for understanding of the findings. By engaging community and scientific partners together in systematically reflecting on the data, there will often be a better answer to questions such as
• What are we seeing? (e.g., Is there an association between the level of the intervention and improvement or worsening in outcomes?)
• What does it mean? (e.g., What does this suggest about the amount and kind of intervention strategies that may be necessary?)
• Implications for adjustment? (e.g., Given what we are learning, what adjustments should we make in our efforts?) (Fawcett et al., 2003).
Disentangling Effects of Interventions
Certain communities are starting to report reductions in obesity. The difficulty is that there is rarely one single intervention that made a difference, and the different components of the comprehensive intervention (e.g., programs, policies, and built environment features) came online at different time points. For example, Philadelphia has reported a reduction in childhood obesity from 21.5 percent to 20.5 percent over a 3-year period, and a wide variety of school and community interventions—different policies, programs, and environmental changes in multiple sectors—may be responsible (Robbins et al., 2012). But which initiatives—combinations of programs and policies—have the greatest potential to achieve this result? What about cost-effectiveness? Which ones should other communities replicate? What combination of interventions had the most power?
One response is that the unique context of a given community makes for a complex set of events that is difficult to interpret. Yet this is not sufficient. True, complexity and context make for unique
_____________
9 See http://www.caloriccalculator.org (accessed November 12, 2013).
combinations of interventions and outcomes in any given community. However, new patterns can be seen when one steps back from complexity and looks at differences and similarities across community initiatives. Looking across many communities, it may become possible to identify the interventions that are consistently associated with improvements in outcomes. It may even be possible to derive theories to explain the patterns after the fact, a practice that has become very useful for evaluation in complex areas such as quality improvement in medicine (Dixon-Woods et al., 2011). Theories of importance to community-level initiatives might include organizational change theory, for example (Glanz and Bishop, 2010). Such patterns might be identified, provided that the outcomes of local community-level evaluations become more readily available through fully published details of the interventions and their implementation and context (Green et al., 2009). For example, Philadelphia instituted major changes in the public schools and in the communities surrounding the schools. If these features are consistently seen in urban communities where obesity has declined, then at a minimum they rise to the top of priorities for further study. This is yet another way that single site, pre-post evaluations (perhaps complemented with logic model designs) can have value. Combined with research projects that improve measurement of the community intervention and introduce a variety of controls (perhaps using nonequivalent control, regression discontinuity, or interrupted time series designs), such instances reduce uncertainty about the best investments for scarce prevention resources.
However, it will not always be possible to detect which intervention made the most difference. It is important to keep documenting the outcome of interest, as would a historian, documenting key events and contextual changes that occur on the timeline.
A Better System to Identify Interventions That Are Suitable for Evaluation
Across communities and interventions, the wealth of potential leverage points to intervene is daunting—an “embarrassment of riches” thanks to the social ecological model. In addition to disentangling the powerful leverage points in existing evaluations, it may be possible to approach the problem differently, through the Systematic Screening and Assessment (SSA) Method (Leviton et al., 2010a). Whereas synthesis relies on collecting the results of existing evaluations, the SSA Method collects promising programs and then determines whether they are worthwhile evaluating. The SSA Method was initially used in collaboration among RWJF, CDC, and the ICF Macro contract research firm to screen 458 nominations of policy and environmental change initiatives to prevent childhood obesity. An expert panel reviewed these nominations and selected 48 that underwent evaluability assessments to assess both their potential for population-level impact and their readiness for evaluation. Of these, 20 were deemed to be both promising and ready for evaluation, and at least 6 of the top-rated innovations have now undergone evaluation. Byproducts of this process included some insights about the combinations of program components that were plausible to achieve population-level outcomes. Out of the array of potential leverage points, at least some were identified as having more payoffs, in advance of costly evaluation.
EXAMPLE: OPPORTUNITIES AND CHALLENGES OF EVALUATING COMMUNITY-LEVEL COMPONENTS OF THE WEIGHT OF THE NATION
Some of the opportunities and challenges for measuring progress in obesity prevention at the community level can be illustrated using TWOTN as an example. The TWOTN video and collateral
campaign—a nationally developed program—can be employed locally to engage stakeholders to take action as part of a multi-component awareness, advocacy, and action strategy (see Chapter 1 for description). One approach to assessing the local contributions of TWOTN, as distinguished from national contributions (see Chapter 6 for description of measurement opportunities of the national components), is to evaluate such local efforts consistent with their stated aims and an articulated logic model or theory of change. The following describes current community-level evaluations that are in process and how the use of a logical model as described in this chapter could focus the analysis and improve the evaluation information. Two local-level evaluations of TWOTN are in process. First, Kaiser Permanente surveyed people who conducted small-group screenings of TWOTN and planners and supporters of community-level activities. The surveys focused on participation, usefulness of media and written materials, and intended changes (Personal communication, Sally Thompson Durkan, Kaiser Permanente, April 29, 2013).
Second, CDC Prevention Research Centers (PRCs), led by the University of North Carolina, Chapel Hill, are identifying locally hosted screenings, conducting a pretest and immediate posttest, and following up with 6-week Web surveys of participants willing to be contacted by e-mail. They ask about message credibility, self-efficacy for both individual- and community-level change, community capacity for change, intention to make individual change as well as influence policy and environmental change, and support for three obesity-related policies. The follow-up survey queries respondents about action taken on the single item they identified as a focus of their activity in the posttest.
These CDC PRC efforts will provide some information about community-level activities subsequent to screenings. The community-level evaluations could be more useful if they analyzed their data using the logic model design described above. For example, if schools utilize TWOTN-derived products, such as the three follow-on children’s movies released in May 2013, then one might assess changes in knowledge about obesity before versus after viewing the movies. Lacking a logic model, or even in addition to the logic model, content analysis of the movies could provide an indication of the particular themes and information that are being emphasized. Any other specific objectives of the children’s movies would need to be specified in advance and measured before and after their viewing. This would be strengthened if measured for comparison in nearly identical classrooms, schools, or other units not exposed, with the pre-post differences between units the measure of effect. This, in turn, would be further strengthened if multiple units exposed and not exposed were randomly assigned to receive or not receive the exposure to the video and other TWOTN components. Implementing these steps will require a sustained commitment of resources to support measurement of the community components of the campaign.
Other approaches recommended at the 2012 IOM Workshop (IOM, 2012b) (see Chapter 5) might also be considered. Regardless of research design, the Committee would emphasize the importance of
• utilizing strong theoretical or logic models (Cheadle et al., 2003; Julian, 1997);
• monitoring reach or dosage, which is actually a critical step in the logic model for any health promotion program or mass media campaign (Cheadle et al., 2012; Glasgow et al., 2006; Hornik, 2002);
• conducting multiple waves of measurement, the more the better, preferably both before and after a campaign (Shadish et al., 2002); and
• replicating and more structured reporting on the reach, effectiveness (with whom), adoption by organizations, implementation, and maintenance to enhance external validity or adaptability to other settings (Glasgow et al., 1999).
The mass media literature emphasizes the importance of exposure to the message (Hornik, 2002; IOM, 2012b), which is closely associated with or equivalent to reach and dose; the literature on small-group and community-based interventions emphasizes the parallel concept of participation in the intervention (Glasgow et al., 2006). It is inherently obvious that an intervention, whether it is a mass media campaign or a community-based intervention, cannot affect people’s attitudes or behaviors unless they are exposed to and participate in it. The reach or exposure might amount to as little as a touch, with the associated outcome being the person’s or group’s awareness or recognition of some feature of the event or message, or as much as intensive engagement, measured by a higher level of recall, knowledge, and reaction to the event or message, discussion with others, engagement in new behaviors, and possibly attribution of a behavior change to the intervention.
SUMMARY
Community-level monitoring and summative evaluations are vital for guiding local action and informing national choices about the most effective and cost-effective obesity prevention interventions recommended in the IOM Accelerating Progress in Obesity Prevention (APOP) report for funding, dissemination, and uptake by other communities. The depth and rigor of the evaluations should depend on user needs, the resources available, and the context. Although the highest quality designs and measurement are always helpful, resources may not be available to use them and user questions may not require them. If the monitoring and summative evaluation plan considers resource levels in the context of end-user needs, then key outcomes are likely to be addressed, as summarized in Table 8-3.
Even a few modest additions can greatly improve the credibility and quality of community monitoring and summative evaluation measurement and designs. Yet even the less preferred and less rigorous evaluation designs and measurement can be helpful in aggregate, at the national level, to reduce uncertainty about priority strategies for adoption and further study. Monitoring and summative evaluation plans should at a minimum incorporate the elements of stakeholder involvement; identify and leverage resources; describe the intervention’s framework, logic model, or theory of change; focus the monitoring and evaluation plan; use credible methods; and synthesize and generalize the findings. Given the existing gaps in the current infrastructure for monitoring and summative evaluation of APOP report strategies identified by the Committee, Chapter 10 provides seven recommendations (and a set of potential actions and actors) to support the successful implementation of the components of the Community-Level Obesity Intervention Monitoring and Summative Evaluation Plan.
TABLE 8-3 Recommended Approaches for Key Outcomes of Community Monitoring and Summative Evaluation, by Level of Evaluation Resources
Resources | Key Outcomes | ||
Documenting environmental change | Estimating the dose | Measuring population-level impact | |
Low (5-10%)a |
Oral and written progress reporting annually from community coordinators Observation of selected key strategies |
Intensity (strength, duration, reach) estimates based on progress report information and the literature when available |
Secondary data, when available at an appropriate geographic level |
Medium (10-15%) |
Oral and written progress reporting at regular intervals jointly by evaluators and community coordinators Use of environmental and policy assessment tools for selected key strategies |
Intensity (strength, duration, reach) estimates based on progress reporting information, literature when available, and program evaluations of selected key strategies |
Secondary data, if available School-based surveys of youth food and physical activity attitudes and behaviors |
High (>15%) |
Oral and written progress reporting at regular intervals jointly by evaluators and community coordinators Use of comprehensive and validated environmental and policy assessment tools for all key strategies |
Intensity (strength, duration, reach) estimates based on progress reporting information, literature when available, and program evaluations of all key strategies |
Secondary data, if available School-based surveys of youth Mail/phone surveys of adults |
a Percentages indicate the amount of resources for evaluation, as a percentage of the intervention budget.
SOURCE: Adapted from Community Tool Box (http://ctb.ku.edu/en/default.aspx, accessed Novemer 12, 2013).
REFERENCES
American Evaluation Association. 2011. American Evaluation Association public statement on cultural competence in evaluation. http://www.eval.org/p/cm/ld/fid=92 (accessed June 3, 2013).
Anderson, L. M., T. A. Quinn, K. Glanz, G. Ramirez, L. C. Kahwati, D. B. Johnson, L. R. Buchanan, W. R. Archer, S. Chattopadhyay, G. P. Kalra, D. L. Katz, and Task Force on Community Preventive Services. 2009. The effectiveness of worksite nutrition and physical activity interventions for controlling employee overweight and obesity: A systematic review. American Journal of Preventive Medicine 37(4):340-357.
Ashe, M., L. M. Feldstein, S. Graff, R. Kline, D. Pinkas, and L. Zellers. 2007. Local venues for change: Legal strategies for healthy environments. Journal of Law, Medicine, and Ethics 35(1):138-147.
Atienza, A. A., and A. C. King. 2002. Community-based health intervention trials: An overview of methodological issues. Epidemiologic Reviews 24(1):72-79.
Bailey, E. J., S. G. Kruske, P. S. Morris, C. J. Cates, and A. B. Chang. 2008. Culture-specific programs for children and adults from minority groups who have asthma. Cochrane Database of Systematic Reviews (2):CD006580.
Berkson, S. S., J. Espinola, K. A. Corso, H. Cabral, R. McGowan, and V. R. Chomitz. 2013. Reliability of height and weight measurements collected by physical education teachers for a school-based body mass index surveillance and screening system. Journal of School Health 83(1):21-27.
Booth, K. M., M. M. Pinkston, and W. S. Poston. 2005. Obesity and the built environment. Journal of the American Dietetic Association 105(5 Suppl 1):S110-S117.
Brook, R. H., E. B. Keeler, K. N. Lohr, J. P. Newhouse, J. E. Ware, W. H. Rogers, A. Ross Davies, C. D. Sherbourne, G. A. Goldberg, P. Camp, C. Kamberg, A. Leibowitz, J. Keesey, and D. Reboussin. 2006. The health insurance experiment: A classic RAND study speaks to the current health care reform debate. Santa Monica, CA: RAND Corporation.
Brownson, R. C., C. M. Hoehner, K. Day, A. Forsyth, and J. F. Sallis. 2009. Measuring the built environment for physical activity: State of the science. American Journal of Preventive Medicine 36(4 Suppl):S99-S123, e1-e12.
Bunin, G. R., L. G. Spector, A. F. Olshan, L. L. Robison, M. Roesler, S. Grufferman, X. O. Shu, and J. A. Ross. 2007. Secular trends in response rates for controls selected by random digit dialing in childhood cancer studies: A report from the children’s oncology group. American Journal of Epidemiology 166(1):109-116.
CDC (Centers for Disease Control and Prevention). 2007. Best practices for comprehensive tobacco control programs—October 2007. Atlanta, GA: U.S. Department of Health and Human Services, Centers for Disease Control and Prevention, National Center for Chronic Disease Prevention and Health Promotion, Office on Smoking and Health.
CDC. 2012. Community transformation grants (CTG). http://www.cdc.gov/communitytransformation/index.htm (accessed April 10, 2013).
Cheadle, A., E. Wagner, T. Koepsell, A. Kristal, and D. Patrick. 1992. Environmental indicators: A tool for evaluating community-based health-promotion programs. American Journal of Preventive Medicine 8(6):345-350.
Cheadle, A., W. L. Beery, H. P. Greenwald, G. D. Nelson, D. Pearson, and S. Senter. 2003. Evaluating the California Wellness Foundation’s Health Improvement Initiative: A logic model approach. Health Promotion Practice 4(2):146-156.
Cheadle, A., P. M. Schwartz, S. Rauzon, E. Bourcier, S. Senter, and R. Spring. 2012. Using the concept of “population dose” in planning and evaluating community-level obesity prevention initiatives. Clinical Medicine & Research 10(3):184-185.
Chinman, M., S. Hunter, P. Ebener, S. Paddock, L. Stillman, P. Imm, and A. Wandersman. 2008. The Getting to Outcomes demonstration and evaluation: An illustration of the prevention support system. American Journal of Community Psychology 41(3-4):206-224.
Chriqui, J. F., J. C. O’Connor, and F. J. Chaloupka. 2011. What gets measured, gets changed: Evaluating law and policy for maximum impact. Journal of Law, Medicine & Ethics 39:21-26.
Collie-Akers, V. L., S. B. Fawcett, J. A. Schultz, V. Carson, J. Cyprus, and J. E. Pierle. 2007. Analyzing a community-based coalition’s efforts to reduce health disparities and the risk for chronic disease in Kansas City, Missouri. Preventing Chronic Disease 4(3):A66. http://www.cdc.gov/pcd/issues/2007/jul/06_0101.htm (accessed June 4, 2013).
COMMIT Research Group. 1995. Community intervention trial for smoking cessation (COMMIT): I. Cohort results from a four-year community intervention. American Journal of Public Health 85(2):183-192.
Community-Campus Partnerships for Health. 2013. Community-campus partnerships for health. Service learning. http://depts.washington.edu/ccph/servicelearningres.html (accessed June 3, 2013).
Community Preventive Services Task Force. 2013. The guide to community preventive services: What works to promote health. http://www.thecommunityguide.org/index.html (accessed April 10, 2013).
Council on Education for Public Health. 2005. Accreditation criteria schools of public health. http://www.ceph.org/assets/SPH-Criteria.pdf (accessed June 3, 2013).
County Health Rankings. 2013. What works for health. http://www.countyhealthrankings.org/programs (accessed May 31, 2013).
Cronbach, L. J. 1982. Designing evaluations of educational and social programs. San Francisco, CA: Jossey-Bass.
Curtin, R., S. Presser, and E. Singer. 2005. Changes in telephone survey nonresponse over the past quarter century. Public Opinion Quarterly 69(1):87-98.
Dietz, W. H., M. T. Story, and L. C. Leviton. 2009. Issues and implications of screening, surveillance, and reporting of children’s BMI. Pediatrics 124(Suppl 1):S98-S101.
Dixon-Woods, M., C. L. Bosk, E. L. Aveling, C. A. Goeschel, and P. J. Pronovost. 2011. Explaining Michigan: Developing an ex post theory of a quality improvement program. Milbank Quarterly 89(2):167-205.
Doyle, S., A. Kelly-Schwartz, M. Schlossberg, and J. Stockard. 2006. Active community environments and health: The relationship of walkable and safe communities to individual health. Journal of the American Planning Association 72(1):19-31.
Economos, C. D., R. R. Hyatt, J. P. Goldberg, A. Must, E. N. Naumova, J. J. Collins, and M. E. Nelson. 2007. A community intervention reduces BMI z-score in children: Shape Up Somerville first year results. Obesity (Silver Spring) 15(5):1325-1336.
Farnham, P. G., D. R. Holtgrave, S. L. Sansom, and H. I. Hall. 2010. Medical costs averted by HIV prevention efforts in the United States, 1991-2006. Journal of Acquired Immune Deficiency Syndromes 54(5):565-567.
Farquhar, J. W., S. P. Fortmann, J. A. Flora, C. B. Taylor, W. L. Haskell, P. T. Williams, N. Maccoby, and P. D. Wood. 1990. Effects of communitywide education on cardiovascular disease risk factors. The Stanford Five-City Project. Journal of the American Medical Association 264(3):359-365.
Fawcett, S. B., and J. A. Schultz. 2008. Supporting participatory evaluation using the Community Tool Box online documentation system. In Community-based participatory research for health, edited by M. Minkler and N. Wallerstein. San Francisco, CA: Jossey-Bass. Pp. 419-424.
Fawcett, S. B., R. Boothroyd, J. A. Schultz, V. T. Francisco, V. Carson, and R. Bremby. 2003. Building capacity for participatory evaluation within community initiatives. Journal of Prevention and Intervention in the Community 26(2):21-36.
Fetterman, D. M., and A. Wandersman, eds. 2005. Empowerment evaluation. Principles in practice. New York: The Guilford Press.
Fielding, J. E., and T. R. Frieden. 2004. Local knowledge to enable local action. American Journal of Preventive Medicine 27(2):183-184.
Fishbein, M., and I. Ajzen. 2010. Predicting and changing behavior: The reasoned action approach. New York: Taylor & Francis Group.
Fitzpatrick, J. L., J. R. Sanders, and B. R. Worthen. 2010. Program evaluation: Alternative approaches and practical guidelines. 4th ed. Upper Saddle River, NJ: Pearson.
Glanz, K. 2009. Measuring food environments: A historical perspective. American Journal of Preventive Medicine 36(4 Suppl):S93-S98.
Glanz, K., and D. B. Bishop. 2010. The role of behavioral science theory in development and implementation of public health interventions. Annual Review of Public Health 31:399-418.
Glanz, K., and D. Hoelscher. 2004. Increasing fruit and vegetable intake by changing environments, policy and pricing: Restaurant-based research, strategies, and recommendations. Preventive Medicine 39(Suppl 2):S88-S93.
Glasgow, R. E., T. M. Vogt, and S. M. Boles. 1999. Evaluating the public health impact of health promotion interventions: The RE-AIM framework. American Journal of Public Health 89(9):1322-1327.
Glasgow, R. E., L. M. Klesges, D. A. Dzewaltowski, P. A. Estabrooks, and T. M. Vogt. 2006. Evaluating the impact of health promotion programs: Using the RE-AIM framework to form summary measures for decision making involving complex issues. Health Education Research 21(5):688-694.
Green, L. W., and R. E. Glasgow. 2006. Evaluating the relevance, generalization, and applicability of research: Issues in external validation and translation methodology. Evaluation and the Health Professions 29(1):126-153.
Green, L. W., R. E. Glasgow, D. Atkins, and K. Stange. 2009. Making evidence from research more relevant, useful, and actionable in policy, program planning, and practice: Slips “twixt cup and lip.” American Journal of Preventive Medicine 37(6S1)S187-S191.
Haddix, A. C., S. M. Teutsch, and P. S. Corso. 2002. Prevention effectiveness: A guide to decision analysis and economic evaluation. New York: Oxford University Press.
Hannay, J., R. Dudley, S. Milan, and P. K. Leibovitz. 2013. Combining photovoice and focus groups: Engaging Latina teens in community assessment. American Journal of Preventive Medicine 44(3 Suppl 3):S215-S224.
Hawkins, N. G., R. W. Sanson-Fisher, A. Shakeshaft, C. D’Este, and L. W. Green. 2007. The multiple baseline design for evaluating population-based research. American Journal of Preventive Medicine 33(2):162-168.
Hawthorne, K., Y. Robles, R. Cannings-John, and A. G. Edwards. 2008. Culturally appropriate health education for type 2 diabetes mellitus in ethnic minority groups. Cochrane Database of Systematic Reviews (3):CD006424.
Heath, G. W., R. C. Brownson, J. Kruger, R. Miles, K. E. Powell, L. T. Ramsey, and Task Force on Community Preventive Services. 2006. The effectiveness of urban design and land use and transport policies and practices to increase physical activity: A systematic review. Journal of Physical Activity and Health 3(Suppl 1):S55-S76.
HHS (Department of Health and Human Services). 2008. Physical activity guidelines for Americans. Washington, DC: Department of Health and Human Services.
Hoelscher, D. M., S. H. Kelder, A. Perez, R. S. Day, J. S. Benoit, R. F. Frankowski, J. L. Walker, and E. S. Lee. 2010. Changes in the regional prevalence of child obesity in 4th, 8th, and 11th grade students in Texas from 2000-2002 to 2004-2005. Obesity (Silver Spring) 18(7):1360-1368.
Homer, J., B. Milstein, K. Wile, J. Trogdon, P. Huang, D. Labarthe, and D. Orenstein. 2010. Simulating and evaluating local interventions to improve cardiovascular health. Preventing Chronic Disease 7(1):A18. http://www.cdc.gov/pcd/issues/2010/jan/08_0231.htm (accessed June 4, 2013).
Hornik, R. C. 2002. Exposure: Theory and evidence about all the ways it matters. Social Marketing Quarterly 8(3):31-37.
Hulleman, C. S., and D. S. Cordray. 2009. Moving from the lab to the field: The role of fidelity and achieved relative intervention strength. Journal of Research on Educational Effectiveness 2(1):88-110.
IOM (Institute of Medicine). 2009. Local government actions to prevent childhood obesity. Washington, DC: The National Academies Press.
IOM. 2010. Bridging the evidence gap in obesity prevention: A framework to inform decision making. Washington, DC: The National Academies Press.
IOM. 2012a. Accelerating progress in obesity prevention: Solving the weight of the nation. Washington, DC: The National Academies Press.
IOM. 2012b. Measuring progress in obesity prevention: Workshop report. Washington, DC: The National Academies Press.
Israel, B. A., E. Eng, A. J. Schulz, and E. A. Parker. 2012. Methods for community-based participatory research for health. 2nd ed. San Francisco, CA: Jossey-Bass.
Issel, L. M. 2009. Health program planning and evaluation: A practical systematic approach for community health. 2nd ed. Sudbury, MA: Jones and Bartlett Publishers.
Jagosh, J., A. C. Macaulay, P. Pluye, J. Salsberg, P. L. Bush, J. Henderson, E. Sirett, G. Wong, M. Cargo, C. P. Herbert, S. D. Seifer, L. W. Green, and T. Greenhalgh. 2012. Uncovering the benefits of participatory research: Implications of a realist review for health research and practice. Milbank Quarterly 90(2):311-346.
Julian, D. A. 1997. The utilization of the logic model as a system level planning and evaluation device. Evaluation and Program Planning 20(3):251-257.
Kahn, E. B., L. T. Ramsey, R. C. Brownson, G. W. Heath, E. H. Howze, K. E. Powell, E. J. Stone, M. W. Rajab, and P. Corso. 2002. The effectiveness of interventions to increase physical activity. A systematic review. American Journal of Preventive Medicine 22(4 Suppl):73-107.
Khan, L., K. Sobush, D. Keener, K. Goodman, A. Lowry, J. Kakietek, S. Zaro. 2009. Recommended community strategies and measurements to prevent obesity in the United States. Morbidity and Mortality Weekly Report 58(RR-7):1-26.
King, G., E. Gakidou, K. Imai, J. Lakin, R. T. Moore, C. Nall, N. Ravishankar, M. Vargas, M. M. Tellez-Rojo, J. E. Avila, M. H. Avila, and H. H. Llamas. 2009. Public policy for the poor? A randomised assessment of the Mexican universal health insurance programme. Lancet 373(9673):1447-1454.
Koepsell, T. D., E. H. Wagner, A. C. Cheadle, D. L. Patrick, D. C. Martin, P. H. Diehr, E. B. Perrin, A. R. Kristal, C. H. Allan-Andrilla, and L. J. Dey. 1992. Selected methodological issues in evaluating community-based health promotion and disease prevention programs. Annual Review of Public Health 13:31-57.
Koh, H. K., C. M. Judge, H. Robbins, C. C. Celebucki, D. K. Walker, and G. N. Connolly. 2005. The first decade of the Massachusetts tobacco control program. Public Health Reports 120(5):482-495.
Leviton, L. C., L. Kettel Khan, and N. Dawkins, eds. 2010a. The Systematic Screening and Assessment Method: Finding innovations worth evaluating. New Directions in Evaluation (125):1-118.
Leviton, L. C., L. Kettel-Khan, D. Rog, N. Dawkins, and D. Cotton. 2010b. Evaluability assessment to improve public health policies, programs, and practices. Annual Review of Public Health 31:213-233.
Mercer, S. L., B. J. DeVinney, L. J. Fine, L. W. Green, and D. Dougherty. 2007. Study designs for effectiveness and translation research: Identifying trade-offs. American Journal of Preventive Medicine 33(2):139-154.
Mercer, S. L., L. W. Green, M. Cargo, M. Potter, M. Daniel, R. S. Olds, and E. Reed-Gross. 2008. Reliability-tested guidelines for assessing participatory research projects. In Community-based participatory research for health: From process to outcomes. 2nd ed., edited by M. Minkler and N. Wallerstein. San Francisco, CA: Jossey-Bass. Pp. 407-418.
Merzel, C., and J. D’Afflitti. 2003. Reconsidering community-based health promotion: Promise, performance, and potential. American Journal of Public Health 93(4):557-574.
Minkler, M., and N. Wallerstein. 2008. Introduction to CBPR: New issues and emphasis. In Community-based participatory research for health: From process to outcomes. 2nd ed., edited by M. Minkler and N. Wallerstein. San Francisco, CA: Jossey-Bass. Pp. 5-24.
National Heart, Lung, and Blood Institute. 2012. Healthy communities study. http://www.nhlbi.nih.gov/hcs/index.htm (accessed April 10, 2013).
Osei-Assibey, G., S. Dick, J. Macdiarmid, S. Semple, J. J. Reilly, A. Ellaway, H. Cowie, and G. McNeill. 2012. The influence of the food environment on overweight and obesity in young children: A systematic review. BMJ Open 2:e001538. doi: 10.1136/.
Papas, M. A., A. J. Alberg, R. Ewing, K. J. Helzlsouer, T. L. Gary, and A. C. Klassen. 2007. The built environment and obesity. Epidemiologic Reviews 29:129-143.
Pateriya, D., and P. Castellanos. 2003. Power tools manual. A manual for organizations fighting for justice. Los Angeles, CA: Strategic Concepts in Organizing and Policy Education.
Patton, M. Q. 2008. Utilization-focused evaluation. 4th ed. Thousand Oaks, CA: Sage Publications.
Preskill, H., and N. A. Jones. 2009. Practical guide for engaging stakeholders in the evaluation process. Princeton, NJ: Robert Wood Johnson Foundation.
Resnicow, K., T. Baranowski, J. S. Ahluwalia, and R. L. Braithwaite. 1999. Cultural sensitivity in public health: Defined and demystified. Ethnicity and Disease 9(1):10-21.
Ritchie, L. D., P. B. Crawford, D. M. Hoelscher, and M. S. Sothern. 2006. Position of the American Dietetic Association: Individual-, family-, school-, and community-based interventions for pediatric overweight. Journal of the American Dietetic Association 106(6):925-945.
Robbins, J. M., G. Mallya, M. Polansky, and D. F. Schwarz. 2012. Prevalence, disparities, and trends in obesity and severe obesity among students in the Philadelphia, Pennsylvania, school district, 2006-2010. Preventing Chronic Disease 9. http://dx.doi.org/10.5888/pcd9.120118 (accessed June 4, 2013).
Robinson, T. N., D. M. Matheson, H. C. Kraemer, D. M. Wilson, E. Obarzanek, N. S. Thompson, S. Alhassan, T. R. Spencer, K. F. Haydel, M. Fujimoto, A. Varady, and J. D. Killen. 2010. A randomized controlled trial of culturally tailored dance and reducing screen time to prevent weight gain in low-income African American girls: Stanford GEMS. Archives of Pediatrics and Adolescent Medicine 164(11):995-1004.
Rossi, P. H., M. W. Lipsey, and H. E. Freeman. 2004. Evaluation: A systematic approach. 7th ed. Thousand Oaks, CA: Sage Publications.
Roussos, S. T., and S. B. Fawcett. 2000. A review of collaborative partnerships as a strategy for improving community health. Annual Review of Public Health 21:369-402.
Rundle, A. G., M. D. Bader, C. A. Richards, K. M. Neckerman, and J. O. Teitler. 2011. Using Google Street View to audit neighborhood environments. American Journal of Preventive Medicine 40(1):94-100.
Saelens, B. E., and K. Glanz. 2009. Work group I: Measures of the food and physical activity environment: Instruments. American Journal of Preventive Medicine 36(4 Suppl):S166-S170.
Saelens, B. E., J. F. Sallis, J. B. Black, and D. Chen. 2003. Neighborhood-based differences in physical activity: An environment scale evaluation. American Journal of Public Health 93(9):1552-1558.
Sallis, J. F. 2009. Measuring physical activity environments: A brief history. American Journal of Preventive Medicine 36(4 Suppl):S86-S92.
Sallis, J. F., and K. Glanz. 2006. The role of built environments in physical activity, eating, and obesity in childhood. Future of Children 16(1):89-108.
Sallis, J. F., and K. Glanz. 2009. Physical activity and food environments: Solutions to the obesity epidemic. Milbank Quarterly 87(1):123-154.
Sallis, J. F., R. B. Cervero, W. Ascher, K. A. Henderson, M. K. Kraft, and J. Kerr. 2006. An ecological approach to creating active living communities. Annual Review of Public Health 27:297-322.
Sanson-Fisher, R. W., B. Bonevski, L. W. Green, and C. D’Este. 2007. Limitations of the randomized controlled trial in evaluating population-based health interventions. American Journal of Preventive Medicine 33(2):155-161.
Sayers, S. P., J. W. LeMaster, I. M. Thomas, G. F. Petroski, and B. Ge. 2012. Bike, walk, and wheel: A way of life in Columbia, Missouri, revisited. American Journal of Preventive Medicine 43(5 Suppl 4):S379-S383.
Shadish, W. R., T. D. Cook, and L. C. Leviton. 1991. Foundations of program evaluation: Theorists and their theories. Newbury Park, CA: SAGE Publications.
Shadish, W. R., T. D. Cook, and D. T. Campbell. 2002. Experimental and quasi-experimental designs for generalized causal inference. 2nd ed. Boston, MA: Houghton Mifflin.
Shaw, I. F., J. C. Greene, and M. M. Mark. 2006. The SAGE handbook of evaluation. London: SAGE Publications.
Tang, H., E. Abramsohn, H. Y. Park, D. W. Cowling, and W. K. Al-Delaimy. 2010. Using a cessation-related outcome index to assess California’s cessation progress at the population level. Tobacco Control 19(Suppl 1):i56-i61.
Taylor, B. T., P. Fernando, A. E. Bauman, A. Williamson, J. C. Craig, and S. Redman. 2011. Measuring the quality of public open space using Google Earth. American Journal of Preventive Medicine 40(2):105-112.
Wagner, E. H., T. M. Wickizer, A. Cheadle, B. M. Psaty, T. D. Koepsell, P. Diehr, S. J. Curry, M. Von Korff, C. Anderman, W. L. Beery, D. C. Pearson, and E. B. Perrin. 2000. The Kaiser Family Foundation Community Health Promotion Grants Program: Findings from an outcome evaluation. Health Services Research 35(3):561-589.
Wang, C. C., S. Morrel-Samuels, P. M. Hutchison, L. Bell, and R. M. Pestronk. 2004. Flint photovoice: Community building among youths, adults, and policymakers. American Journal of Public Health 94(6):911-913.
Wang, Y. C., C. T. Orleans, and S. L. Gortmaker. 2012. Reaching the Healthy People goals for reducing childhood obesity: Closing the energy gap. American Journal of Preventive Medicine 42(5):437-444.
Waters, E., A. de Silva-Sanigorski, B. J. Hall, T. Brown, K. J. Campbell, Y. Gao, R. Armstrong, L. Prosser, and C. D. Summerbell. 2011. Interventions for preventing obesity in children. Cochrane Database of Systematic Reviews Issue 12. Art. No.: CD001871. DOI: 10.1002/14651858.CD001871.pub3.
Weinstein, M. C., and W. B. Stason. 1976. Hypertension: A policy perspective. Cambridge, MA: Harvard University Press.
Wholey, J. S., H. P. Hatry, and K. E. Newcomber. 2010. Handbook of practical program evaluation. 3rd ed. San Francisco, CA: Jossey-Bass.
Yin, R. K. 2008. Case study research: Design and methods. 4th ed. Thousand Oaks, CA: Sage.
Yin, R. K., and K. A. Heald. 1975. Using the case survey method to analyze policy studies. Administrative Science Quarterly 20:371-381.
This page intentionally left blank.