3
Integrative Architectures for Modeling the Individual Combatant
We have argued in the introduction that in order to make human behavior representation more realistic in the setting of military simulations, the developers will have to rely more on behavioral and organizational theory. In Chapters 3 through 11 we present scientific and applied developments that can contribute to better models. Chapters 4 through 8 review component human capacities, such as situation awareness, decision making, planning, and multitasking, that can contribute to improved models.
However, to model the dismounted soldier, and for many other purposes where the behavior of intact individuals is required, an integrative model that subsumes all or most of the contributors to human performance capacities and limitations is needed. Although such quantitative integrative models have rarely been sought by psychologists in the past, there is now a growing body of relevant literature. In the few cases in which these integrative models have been applied to military simulations, they have focused on specific task domains. Future integrative model developers will likely be interested in other domains than the ones illustrated here. Because each of our examples embodies a software approach to integrative models, we are referring to these developments as integrative modeling architectures. They provide a framework with behavioral content that shows promise of providing a starting point for model developers who wish to apply it to their domains. Each has its own strengths and weaknesses and we do not explicitly recommend any one. The developers' choices depend entirely on their goals and objectives in integrative model development. In Chapter 13, we do not recommend converging on a single integrative architecture, although we do argue that it is important to adopt modular structures that will allow easier interoperability among developed models.
The chapter begins with a general introduction to integrative architectures that review their various components; this discussion is couched in terms of a stage model of information processing. Next we review 10 such architectures, describing the purpose, assumptions, architecture and functionality, operation, current implementation, support environment, validation, and applicability of each. The following section compares these architectures across a number of dimensions. This is followed by a brief discussion of hybrid architectures as a possible research path. The final section presents conclusions and goals in the area of integrative architectures.
GENERAL INTRODUCTION TO INTEGRATIVE ARCHITECTURES
A general assumption underlying most if not all of the integrative architectures and tools reviewed in this chapter is that the human can be viewed as an information processor, or an information input/output system. In particular, most of the models examined are specific instantiations of a modified stage model of human information processing. The modified stage model is based on the classic stage model of human information processing (e.g., Broadbent, 1958). The example given here is adapted from Wickens (1992:17). Such a stage model is by no means the only representation of human information processing as a whole, but it is satisfactory for our purposes of introducing the major elements of the architectures to be discussed.
In the modified stage model, sensing and perception models transform representations of external stimulus energy into internal representations that can be operated on by cognitive processes (see Figure 3.1).
Memory consists of two components. Working memory holds information temporarily for cognitive processing (see below). Long-term memory is the functional component responsible for holding large amounts of information for long periods of time. (See also Chapter 5.)
Cognition encompasses a wide range of information processing functions. Situation awareness refers to the modeled individual combatant's state of knowledge about the environment, including such aspects as terrain, the combatant's own position, the position and status of friendly and hostile forces, and so on (see also Chapter 7). Situation assessment is the process of achieving that state of knowledge. A mental model is the representation in short- and long-term memory of information obtained from the environment. Multitasking models the process of managing multiple, concurrent tasks (see also Chapter 4). Learning models the process of altering knowledge, factual or procedural (see also Chapter 5). Decision making models the process of generating and selecting alternatives (see also Chapter 6).
Motor behavior, broadly speaking, models the functions performed by the neuromuscular system to carry out the physical actions selected by the above-mentioned processes. Planning, decision making, and other "invisible" cognitive
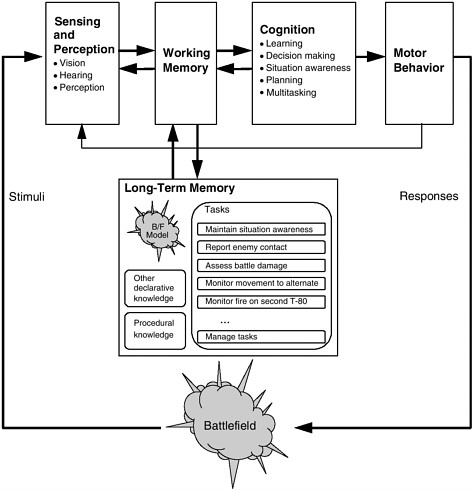
FIGURE 3.1 Modified stage model. NOTE: Tasks shown are derived from the vignette presented in Chapter 2.
behaviors are ultimately manifested in observable behaviors that must be simulated with varying degrees of realism, depending on actual applications; aspects of this realism include response delays, speed/accuracy tradeoffs, and anthropometric considerations, to name but a few.
Each of the architectures reviewed incorporates these components to some extent. What is common to the architectures is not only their inclusion of submodels of human behavior (sensing, perception, cognition, and so on), but also the integration of the submodels into a large and coherent framework. It would be possible to bring a set of specific submodels of human behavior together in an ad hoc manner, with little thought to how they interact and the emergent properties that result. But such a model would not be an integrative architecture. On the contrary, each of these integrative architectures in some way
reflects or instantiates a unified theory of human behavior (at least in the minds of its developers) in which related submodels interact through common representations of intermediate information processing results, and in which consistent conceptual representations and similar tools are used throughout.
REVIEW OF INTEGRATIVE ARCHITECTURES
This section reviews 11 integrative architectures (presented in alphabetical order):
-
Adaptive control of thought (ACT-R)
-
COGnition as a NEtwork of Tasks (COGNET)
-
Executive-process interactive control (EPIC)
-
Human operator simulator (HOS)
-
Micro Saint
-
Man machine integrated design and analysis system (MIDAS)
-
MIDAS redesign
-
Neural networks
-
Operator model architecture (OMAR)
-
Situation awareness model for pilot-in-the-loop evaluation (SAMPLE)
-
Soar
The following aspects of each architecture are addressed:
-
Its purpose and use
-
Its general underlying assumptions
-
Its architecture and functionality
-
Its operation
-
Features of its current implementation
-
Its support environment
-
The extent to which it has been validated
-
The panel's assessment of its applicability for military simulations
It should be noted that the discussion of these models is based on documentation available to the panel at the time of writing. Most of the architectures are still in development and are likely to change—perhaps in very fundamental ways. The discussion here is intended to serve as a starting point for understanding the structure, function, and potential usefulness of the architectures. The organizations responsible for their development should be contacted for more detailed and timely information.
Adaptive Control of Thought (ACT-R)1
ACT-R is a "hybrid" cognitive architecture that aspires to provide an integrated
account of many aspects of human cognition (see also the later section on hybrid architectures). It is a successor to previous ACT production-system theories (Anderson, 1976, 1983), with emphasis on activation-based processing as the mechanism for relating a production system to a declarative memory (see also Chapter 5).
Purpose and Use
ACT-R as originally developed (Anderson, 1993) was a model of higher-level cognition. That model has been applied to modeling domains such as Tower of Hanoi, mathematical problem solving in the classroom, navigation in a computer maze, computer programming, human memory, learning, and other tasks. Recently, a theory of vision and motor movement was added to the basic cognitive capability (Byrne and Anderson, 1998), so theoretically sound interaction with an external environment can now be implemented.
Assumptions
In general, ACT-R adheres to the assumptions inherent in the modified stage model (Figure 3.1), with the minor exception that all processors, including the motor processors, communicate through the contents of working memory (not directly from cognition).
ACT-R assumes that there are two types of knowledge—declarative and procedural—and that these are architecturally distinct. Declarative knowledge is represented in terms of chunks (Miller, 1956; Servan-Schreiber, 1991), which are schema-like structures consisting of an isa pointer specifying their category and some number of additional pointers encoding their contents. Procedural knowledge is represented in production rules. ACT-R's pattern-matching facility allows partial matches between the conditions of productions and chunks in declarative memory (Anderson et al., 1996).
Both declarative and procedural knowledge exist permanently in long-term memory. Working memory is that portion of declarative knowledge that is currently active. Thus, the limitation on working memory capacity in ACT-R concerns access to declarative knowledge, not the capacity of declarative knowledge.
ACT-R assumes several learning mechanisms. New declarative chunks can be learned from the outside world or as the result of problem solving. Associations between declarative memory elements can be tuned through experience. New productions can be learned through analogy to old procedural knowledge. Production strengths change through experience. The visual attention model embedded within ACT-R assumes a synthesis of the spotlight metaphor of Posner (1980), the feature-synthesis model of Treisman (Treisman and Sato, 1990), and the attentional model of Wolfe (1994).
Details of the ACT-R architecture have been strongly guided by the rational analysis of Anderson (1990). As a consequence of that rational analysis, ACT-R
is a production system tuned to perform adaptively given the statistical structure of the environment.
Architecture and Functionality
ACT-R's declarative and procedural knowledge work together as follows. All production rules in ACT-R have the basic character of responding to some goal (encoded as a special type of declarative memory element), retrieving information from declarative memory, and possibly taking some action or setting a subgoal. In ACT-R, cognition proceeds forward step by step through the firing of such production rules.
All production instantiations are matched against declarative memory in parallel, but the ACT-R architecture requires only one of these candidate productions to fire on every cycle. To choose between competing productions, ACT-R has a conflict-resolution system that explicitly tries to minimize computational cost while still firing the production rule most likely to lead to the best result. The time needed to match a production depends on an intricate relationship among the strength of the production, the complexity of its conditions, and the level of activation of the matching declarative memory elements. In addition to taking different times to match, productions also differ in their contribution to the success of the overall task. This value is a relationship among the probability that the production will lead to the goal state, the cost of achieving the goal by this means, and the value of the goal itself. The architecture chooses a single production by satisfying: when the expected cost of continuing the match process exceeds the expected value of the next retrieved production, the instantiation process is halted, and the production that matches the highest value is chosen. To implement this conflict-resolution scheme, ACT-R models require many numerical parameters. Although most early ACT-R models set these parameters by matching to the data they were trying to explain, more recent models have been able to use stable initial estimates (Anderson and Lebiere, forthcoming).
As mentioned above, ACT-R includes several learning mechanisms. Declarative knowledge structures can be created as the encoding of external events (e.g., reading from a screen) or created in the action side of a production. The base-level activation of a declarative knowledge element can also be learned automatically. Associative learning can automatically adjust the strength of association between declarative memory elements. ACT-R learns new procedural knowledge (productions) through inductive inferences from existing procedural knowledge and worked examples. Finally, production rules are tuned through
learning strengths and updating of the estimates of success-probability and cost parameters. All of these learning mechanisms can be turned on and off depending on the needs of the model. 2
Operation
Most existing ACT-R models stand alone; all of the action is cognitive, while perception and motor behavior are finessed. However, some models have been built that interact with an external world implemented in Macintosh Common Lisp or HyperCard™. To our knowledge, no ACT-R models currently interact with systems not implemented by the modelers themselves (e.g., with a commercially available system such as Flight Simulator™ or a military system).
Initial knowledge, both declarative and procedural, is hand-coded by the human modeler, along with initial numerical parameters for the strength of productions, the cost and probability of success of productions with respect to a goal, the base-level activation of declarative knowledge structures, and the like. (Default values for these parameters are also available, but not assumed to work for every new task modeled.) The output of an ACT-R model is the trace of productions that fire, the way they changed working memory, and the details of what declarative knowledge was used by those productions. If learning is turned on, additional outputs include final parameter settings, new declarative memory elements, and new productions; what the model learns is highly inspectable.
Current Implementation
The currently supported versions of ACT-R are ACT-R 3.0 and ACT-R 4.0. ACT-R 3.0 is an efficient reimplementation of the system distributed with Rules of the Mind (Anderson, 1993), while ACT-R 4.0 implements a successor theory described in Atomic Components of Thought (Anderson and Lebiere, 1998). Since both systems are written in Common Lisp, they are easily extensible and can run without modification on any Common Lisp implementation for Macintosh, UNIX, and DOS/Windows platforms. ACT-R models can run up to 100 times as fast as real time on current desktop computers, depending on the complexity of the task.
Support Environment
In addition to the fully functional and portable implementation of the ACT-R system, a number of tools are available. There is a graphical environment for the development of ACT-R models, including a structured editor; inspecting, tracing, and debugging tools; and built-in tutoring support for beginners. A perceptual/motor layer extending ACT-R's theory of cognition to perception and action is also available. This system, called ACT-R/PM, consists of a number of modules for visual and auditory perception, motor action, and speech production,
which can be added in modular fashion to the basic ACT-R system. Both ACT-R and ACT-R/PM are currently available only for the Macintosh, but there are plans to port them to the Windows platform or to some platform-independent format, such as CLIM.
The basic system and the additional tools are fully documented, and manuals are available both in MS Word and over the World Wide Web. The Web manuals are integrated using a concept-based system called Interbook, with a tutorial guiding beginners through the theory and practice of ACT-R modeling in 10 lessons. The Web-based tutorial is used every year for teaching ACT-R modeling to students and researchers as part of classes at Carnegie Mellon University (CMU) and other universities, as well as summer schools at CMU and in Europe. The yearly summer school at CMU is coupled with a workshop in which ACT-R researchers can present their work and discuss future developments. The ACT-R community also uses an electronic mailing list to announce new software releases and papers and discuss related issues. Finally, the ACT-R Web site (http://act.psy.cmu.edu) acts as a centralized source of information, allowing users to download the software, access the Web-based tutorial and manuals, consult papers, search the mailing list archive, exchange models, and even run ACT-R models over the Web. The latter capacity is provided by the ACT-R-on-the-Web server, which can run any number of independent ACT-R models in parallel, allowing even beginners to run ACT-R models over the Web without downloading or installing ACT-R.
Validation
ACT-R has been evaluated extensively as a cognitive architecture against human behavior and learning in a wide variety of tasks. Although a comprehensive list of ACT-R models and their validation is beyond the scope of this chapter, the most complete sources of validation data and references to archival publications are Anderson's series of books on the successive versions of ACT (Anderson, 1983, 1990, 1993; Anderson and Lebiere, 1998). The latter reference also contains a detailed comparison of four cognitive architectures: ACT-R, executive-process interactive control (EPIC), Soar, and CAPS (a less well-known neural-cognitive architecture not reviewed here).
Applicability for Military Simulations
The vast majority of ACT-R models have been for relatively small problem solving or memory tasks. However, there is nothing in principle that prevents ACT-R from being applicable to military simulations. There may be some problems associated with scaling up the current implementation to extremely large tasks that require extensive knowledge (only because the architecture has not been pushed in this manner), but any efficiency problems could presumably be
solved with optimized matchers and other algorithmic or software engineering techniques such as those that have been applied to Soar. The intent of some currently funded military research contracts (through the Office of Naval Research) is to use ACT-R to model the tasks of the tactical action officer in submarines (Gray et al., 1997) and of radar operators on Aegis-like military ships (Marshall, 1995), but the work is too preliminary at this time to be reported in the archival literature.
Although ACT-R's applicability to military simulations is as yet underdeveloped, ACT-R is highly applicable to military training in cognitive skill. ACT-R-based intelligent tutors (called cognitive tutors) are being used to teach high-school-level mathematics and computer programming in many schools around the world, including the DoD schools for the children of military personnel in Germany. These cognitive tutors reliably increase the math SAT scores of students by one standard deviation. Any cognitive skill the military currently teaches, such as the operation of a dedicated tactical workstation, could be built into a cognitive tutor for delivery anywhere (e.g., on-board training for Navy personnel).
COGnition as a NEtwork of Tasks (COGNET)
COGNET is a framework for creating and exercising models of human operators engaged in primarily cognitive (as opposed to psychomotor) tasks (Zachary et al., 1992; Zachary et al., 1996).
Purpose and Use
COGNET's primary use is for developing user models for intelligent interfaces. It has also been used to model surrogate operators and opponents in submarine warfare simulators.
Assumptions
COGNET allows the creation of models of cognitive behavior and is not designed for modeling psychomotor behavior. The most important assumption behind COGNET is that humans perform multiple tasks in parallel. These tasks compete for the human's attention, but ultimately combine to solve an overall information processing problem. COGNET is based on a theory of weak task concurrence, in which there are at any one time several tasks in various states of completion, though only one of these tasks is executing. That is, COGNET assumes serial processing with rapid attention switching, which gives the overall appearance of true parallelism.
The basis for the management of multiple, competing tasks in COGNET is a pandemonium metaphor of cognitive processes composed of ''shrieking demons,"
proposed by Selfridge (1959). In this metaphor, a task competing for attention is a demon whose shrieks vary in loudness depending on the problem context. The louder a demon shrieks, the more likely it is to get attention. At any given time, the demon shrieking loudest is the focus of attention and is permitted to execute.
Architecture and Functionality
The COGNET architecture consists of a problem context, a perception process, tasks, a trigger evaluation process, an attention focus manager, a task execution process, and an action effector. It is a layered system in which an outer shell serves as the interface between the COGNET model and other components of the larger simulation. There is no explicit environment representation in COGNET. Rather, COGNET interfaces with an external environment representation through its shell.
The problem context is a multipanel blackboard that serves as a common problem representation and a means of communication and coordination among tasks (see below). It provides problem context information to tasks. Its panels represent different parts of the information processing problem and may be divided into areas corresponding to different levels of abstraction of these problem parts.
Perception is modeled in COGNET using a perception process consisting of perceptual demons. These software modules recognize perceptual events in the simulated environment and post information about them (e.g., messages and hypotheses) on the blackboard.
Tasks in COGNET are independent problem solving agents. Each task has a set of trigger conditions. When those conditions are satisfied, the task is activated and eligible to execute. An activated task competes for attention based on the priority of its associated goal (i.e., the loudness of its shrieks in the "shrieking demon" metaphor). Its priority, in turn, is based on specific blackboard content.
Task behaviors are defined by a procedural representation called COGNET executable language. COGNET executable language is a text-based language, but there is also a graphical form (graphical COGNET representation). COGNET executable language (and by extension, graphical COGNET representation) contains a set of primitives supporting information processing behavior. A goal is defined by a name and a set of conditions specifying the requirements for the goal to become active (relevant) and be satisfied. Goals may be composed of subgoals.
Four types of COGNET operators support the information processing and control behaviors of COGNET models. System environment operators are used to activate a workstation function, select an object in the environment (e.g., on a display), enter information into an input device, and communicate. Directed perceptual operators obtain information from displays, controls, and other sources. Cognitive operators post and unpost and transform objects on the blackboard.
Control operators suspend a task until the specified condition exists and subrogate (turn over control) to other tasks.
COGNET executive language also includes conditionals and selection rules, which are used to express branching and iteration at the level of the lowest goals. Conditionals include IF and REPEAT constructs. Selection rules (IF condition THEN action) can be deterministic (the action is performed unconditionally when the condition is true) or probabilistic (the action is performed with some probability when the condition is true).
The COGNET trigger evaluation process monitors the blackboard to determine which tasks have their trigger conditions satisfied. It activates triggered tasks so they can compete for attention.
The attention focus manager monitors task priorities and controls the focus of attention by controlling task state. A task may be executing (that is, its COGNET executive language procedure is being performed) or not executing. A task that has begun executing but is preempted by a higher-priority task is said to be interrupted. Sufficient information about an interrupted task is stored so that the task can be resumed when priority conditions permit. Using this method, the attention focus manager starts, interrupts, and resumes tasks on the basis of priorities. As mentioned above, COGNET assumes serial behavior with rapid attention switching, so only the highest-priority task runs at any time. Tasks are executed by the task execution process , which is controlled by the attention focus manager. The action effector changes the environment.
Operation
The operation of a COGNET model can be described by the following general example, adapted from Zachary et al. (1992):
-
Perceptual demon recognizes event, posts information on blackboard.
-
New blackboard contents satisfy triggering condition for high-level goal of task B, and that task is activated and gains the focus of attention.
-
B subrogates (explicitly turns control over) to task A for more localized or complementary analysis.
-
A reads information from blackboard, makes an inference, and posts this new information on the blackboard.
-
New information satisfies triggering condition for task D, but since it lacks sufficient priority, cannot take control.
-
Instead, new information satisfies triggering condition for higher-priority task C, which takes over.
-
C posts new information, then suspends itself to wait for its actions to take effect.
-
Task D takes control and begins posting information to blackboard.
-
etc.
Current Implementation
COGNET is written in C++ and runs in Windows 95, Windows 97, and UNIX on IBM PCs and Silicon Graphics and Sun workstations. A commercial version is in development. COGNET models run substantially faster than real time.
Support Environment
The support environment for COGNET is called GINA (for Generator of Interface Agents). Its purpose is the creation and modification of COGNET models, the implementation and debugging of executable software based on those models, and the application of executable models to interface agents. It consists of model debugging and testing tools, COGNET GCR editing tools, and a translator for switching back and forth between the GCR representation used by the modeler and the COGNET executive language version of the procedural representation necessary to run the model.
Once a model has been developed and debugged within the GINA development environment, it can be separated and run as a stand-alone computational unit, as, for example, a component in distributed simulation or an embedded model within some larger system or simulation.
Validation
A specific COGNET model in an antisubmarine warfare simulation received limited validation against both the simulated information processing problems used to develop it and four other problems created to test it. The investigators collected data on task instance predictions (that is, COGNET predictions that a real human operator would perform a certain task) and task prediction lead (that is, the amount of time the predicted task instance preceded the actual task performance). When tested against the data from the problems used to develop the model, COGNET correctly predicted 90 percent of the task instances (that is, what tasks were actually performed) with a mean task instance prediction lead time of 4 minutes, 6 seconds. When tested against the new set of information processing problems, it correctly predicted 94 percent of the tasks with a mean task prediction lead time of 5 minutes, 38 seconds. The reader should keep in mind that antisubmarine warfare is much less fast-paced than, say, tank warfare, and situations typically evolve over periods of hours rather than minutes or seconds.
Applicability for Military Simulations
COGNET models have been used as surrogate adversaries in a submarine warfare trainer and as surrogate watchstanders in a simulated Aegis combat information
center. Although the performance of the models is not described in the documentation available to the panel at the time of writing, the fact that these applications were even attempted is an indication of the potential applicability of COGNET in military simulations. Clearly COGNET is a plausible framework for representing cognitive multitasking behavior. A development environment for creating such models would be of benefit to developers of military simulations. Although validation of COGNET has been limited, the results so far are promising.
COGNET is limited in psychological validity, though its developers are taking steps to remedy that problem. The degree of robustness of its decision making and problem solving capabilities is not clear. Its perceptual models appear to be limited, and it is severely constrained in its ability to model motor behavior, though these limitations may not be significant for many applications. Nevertheless, given its ability to provide a good framework for representing cognitive, multitasking behavior, COGNET merits further scrutiny as a potential tool for representing human behavior in military simulations. Moreover, COGNET is being enhanced to include micro-models from the human operator simulator (see below).
Executive-Process Interactive Control (EPIC)3
EPIC is a symbolic cognitive architecture particularly suited to the modeling of human multiple-task performance. It includes peripheral sensory-motor processors surrounding a production rule cognitive processor (Meyer and Kieras, 1997a, 1997b).
Purpose and Use
The goal of the EPIC project is to develop a comprehensive computational theory of multiple-task performance that (1) is based on current theory and results in the domains of cognitive psychology and human performance; (2) will support rigorous characterization and quantitative prediction of mental workload and performance, especially in multiple-task situations; and (3) is useful in the practical design of systems, training, and personnel selection.
Assumptions
In general, EPIC adheres to the assumptions inherent in the modified stage model (Figure 3.1). It uses a parsimonious production system (Bovair et al., 1990) as the cognitive processor. In addition, there are separate perceptual processors
with distinct processing time characteristics, and separate motor processors for vocal, manual, and oculomotor (eye) movements.
EPIC assumes that all capacity limitations are a result of limited structural resources, rather than a limited cognitive processor. Thus the parsimonious production system can fire any number of rules simultaneously, but since the peripheral sense organs and effectors are structurally limited, the overall system is sharply limited in capacity. For example, the eyes can fixate on only one place at a time, and the two hands are assumed to be bottlenecked through a single processor.
Like the ACT-class architectures (see the earlier discussion of ACT-R) EPIC assumes a declarative/procedural knowledge distinction that is represented in the form of separate permanent memories. Task procedures and control strategies (procedural knowledge) are represented in production rules. Declarative knowledge is represented in a preloaded database of long-term memory elements that cannot be deleted or modified. At this time, EPIC does not completely specify the properties of working memory because clarification of the types of working memory systems used in multiple-task performance is one of the project's research goals. Currently, working memory is assumed to contain all the temporary information tested and manipulated by the cognitive processor's production rules, including task goals, sequencing information, and representations of sensory inputs (Kieras et al., 1998).
Unlike many other information processing architectures, EPIC does not assume an inherent central-processing bottleneck. Rather, EPIC explains performance decrements in multiple-task situations in terms of the strategic effects of the task instructions and perceptual-motor constraints. Executive processes—those that regulate the priority of multiple tasks—are represented explicitly as additional production rules. For instance, if task instructions say a stimulus-response task should have priority over a continuous tracking task, a hand-crafted production will explicitly encode that priority and execute the hand movements of the former task before those of the latter. These are critical theoretical distinctions between EPIC and other architectures (although they may not make a practical difference in the modeling of military tasks; see Lallement and John, 1998).
At this time, there is no learning in the EPIC cognitive processor—it is currently a system for modeling task performance.
Architecture and Functionality
EPIC's cognitive, perceptual, and motor processors work together as follows. EPIC's perceptual processors are "pipelines" in that an input produces an output at a certain time later, independent of what particular time the input arrives. A single stimulus input to a perceptual processor can produce multiple outputs in working memory at different times. The first output is a representation
that a perceptual event has been detected; this representation is assumed to be fixed and fairly short at about 50 milliseconds. This is followed later by a representation that describes the recognized event. The timing of this second representation is dependent on properties of the stimulus. For example, recognizing letters on a screen in a typical experiment might take on the order of 150 milliseconds after the detection time. At present, these parametric recognition times are estimated from the empirical data being modeled. The cognitive processor accepts input only every 50 milliseconds, which is consistent with data such as those of Kristofferson (1967), and is constantly running, not synchronized with outside events. The cognitive processor accepts input only at the beginning of each cycle and produces output at the end of the cycle. In each cognitive processor cycle, any number of rules can fire and execute their actions; this parallelism is a fundamental feature of the parsimonious production system. Thus, in contrast with some other information processing architectures, the EPIC cognitive processor is not constrained to do only one thing at a time. Rather, multiple processing threads can be represented simply as sets of rules that happen to fire simultaneously.
EPIC assumes that its motor processors (voice, hands, oculomotor) operate independently, but, as noted above, the hands are bottlenecked through a single manual processor. Producing motor movement involves a series of steps. First, a symbolic name for the movement to be produced is recoded by the motor processor into a set of movement features. Given this set of features, the motor processor is instructed to initiate the movement. The external device (e.g., keyboard, joystick) then detects the movement after some additional mechanical delay. Under certain circumstances, the features can be pregenerated and the motor processor instructed to execute them at a later time. In addition, the motor processor can generate only one set of features at a time, but this preparation can be done in parallel with the physical execution of a previously commanded movement.
Operation
The inputs to an EPIC model are as follows:
-
A production rule representation of the procedures for performing the task
-
The physical characteristics of objects in the environment, such as their color or location
-
A set of specific instances of the task situation, such as the specific stimulus events and their timing
-
Values of certain time parameters in the EPIC architecture
The output of an EPIC model is the predicted times and sequences of actions in the selected task instances.
Once an EPIC model has been constructed, predictions of task performance are
generated through simulation of the human interacting with the task environment in simulated real time, in which the processors run independently and in parallel. Each model includes a process that represents the task environment and generates stimuli and collects the responses and their simulated times over a large number of trials. To represent human variability, the processor time parameters can be varied stochastically about their mean values with a regime that produces a coefficient of variation for simple reaction time of about 20 percent, a typical empirical value.
Current Implementation
EPIC is currently available for Macintosh and various UNIX platforms. It is written in Common Lisp and therefore easily portable to other platforms. A graphical environment is provided on the Macintosh platform via Macintosh Common Lisp. An interface between EPIC's peripherals and Soar's cognitive processor is available on the UNIX platforms.
Support Environment
EPIC is a relatively new architecture that has not yet been the object of the development time and effort devoted to other architectures, such as ACT-R, Soar, and Micro Saint. Therefore, its support environment is not specific to EPIC, but depends on a conventional Lisp programming environment. The EPIC user can rely on several sources of documentation. In addition to publications describing the architecture and research results, Kieras and Meyer (1996) describe the various components of EPIC and their interaction. EPIC's source code, written in Lisp, is heavily annotated and relatively accessible.
Documentation and source code relevant to EPIC can be accessed at <ftp://ftp.eecs.umich.edu/people/kieras/>.
Validation
As mentioned earlier, EPIC is a relatively new integrative architecture, so it has generated fewer models than older architectures such as ACT-R and Soar. However, there has been rigorous evaluation of the EPIC models against human data. Currently, models that match human data both qualitatively and quantitatively exist for the psychological refractory period (PRP) (Meyer and Kieras, 1997a, 1997b), a dual tracking/stimulus-response task (Kieras and Meyer, 1997), a tracking/decision making task (Kieras and Meyer, 1997), verbal working-memory tasks (Kieras et al., 1998), computer interface menu search (Hornoff and Kieras, 1997), and a telephone operator call-completion task (Kieras et al., 1997).
Applicability for Military Simulations
EPIC has not yet been used for any realistic military tasks; researchers have focused more on rigorous validation of psychological tasks and limited computer-interaction tasks. There is nothing in principle that prevents EPIC from being applied for large-scale military tasks, but its current software implementation would probably not scale up to visual scenes as complex as those in military command and control work areas without an investment in software engineering and reimplementation.
Human Operator Simulator (HOS)4
The human operator simulator (HOS) simulates a single human operator in a human-machine system, such as an aircraft or a tank (Glenn et al., 1992). It models perception, cognition, and motor response by generating task timelines and task accuracy data.
Purpose and Use
HOS was developed to support the design of human-machine systems by allowing high levels of system performance to be achieved through explicit consideration of human capabilities and limitations. HOS is used to evaluate proposed designs prior to the construction of mockups and prototypes, which can be very time-consuming and expensive. It serves this function by generating task timelines and task accuracy predictions.
The HOS user employs a text-based editor to define environment objects, such as displays and controls. The user then analyzes operator tasks to produce a detailed task/subtask hierarchy, which is the basis for the model, and defines task procedures in a special procedural language. HOS models must be parameterized to account for moderator variables and other factors that affect behavior (see Chapter 9). The user then runs the model on representative scenarios to derive timelines and predicted task accuracy. These results are analyzed to determine whether performance is satisfactory. If not, the results may yield insight into necessary changes to the design of the human-machine interface.
Assumptions
HOS is based on several assumptions that have implications for its validity and its applicability to military simulations. First, HOS assumes that the human has a single channel of attention and time-shares tasks serially through rapid attention switching. Second, the operator's activities are assumed to be highly
proceduralized and predictable. Although decision making can be modeled, it is limited. Finally, it is assumed that the human does not make errors.
Architecture and Functionality
The HOS system consists of editors, libraries, data files, and other components that support the development and use of HOS models specialized to suit particular analysis activities. Those components most closely related to the human operator are described below.
The environment of the HOS operator is object-oriented and consists of simulation objects. Simulation objects are grouped into classes, such as the class of graphical user interface (GUI). At run time, instances of these classes are created, such as a specific GUI. These instances have attributes, such as the size, color, and position of a symbol on the GUI.
The HOS procedural language is used to define procedures the operator can perform. A HOS procedure consists of a sequence of verb-object steps, such as the following:
-
Look for an object
-
Perceive an attribute of an object
-
Decide what to do with respect to an object
-
Reach for a control object
-
Alter the state of a control object
-
Other (e.g., calculate, comment)
The HOS procedural language also includes the following control constructs:
-
Block
-
Conditional
-
Loop
-
Permit/prevent interruptions by other procedures
-
Branch
-
Invoke another procedure
At the heart of HOS functionality are its micro-models, software modules that model motor, perceptual, and cognitive processes. Micro-models calculate the time required to complete an action as a function of the current state of the environment, the current state of the operator, and default or user-specified model parameters. HOS comes equipped with default micro-models, which are configurable by the user and may be replaced by customized models.
Default micro-models include those for motor processes, which calculate the time to complete a motion based on the distance or angle to move, the desired speed, the desired accuracy, and other factors. Specific motor processes modeled
include hand movements, hand grasp, hand control operations, foot movement, head movement, eye movement, trunk movement, walking, and speaking.
Micro-models for perceptual processes calculate the time required to perceive and interpret based on user-defined detection and perception probabilities. The default micro-models in this category include perception of visual scene features, reading of text, nonattentive visual perception of scene features, listening to speech, and nonattentive listening.
Micro-models for cognitive processes determine the time required to calculate, based on the type of calculation to be made, or to decide, based on the information content of the decision problem (e.g., the number of options from which to choose). Short-term memory is explicitly modeled; this model calculates retrieval probabilities based on latency.
Physical body attributes, such as position and orientation, are modeled by the HOS physical body model. The desired body object positions are determined by micro-models, and the actual positions are then updated. Body parts modeled include the eyes, right/left hand, right/left foot, seat reference position, right/left hip, right/left shoulder, trunk, head, right/left leg, and right/left arm.
In HOS, channels represent limited processing resources. Each channel can perform one action at a time and is modeled as either busy or idle. HOS channels include vision, hearing/speech, right/left hand, right/left foot, and the cognitive central processor.
HOS selection models associate micro-models with procedural language verbs. They invoke micro-models with appropriate parameters as task procedures are processed.
The HOS attention model maintains a list of active tasks. As each step in a procedure is completed, the model determines what task to work on, based on priority, and decides when to interrupt an ongoing task to work on another. Each task has a base priority assigned by the user. Its momentary priority is based on its base priority, the amount of time it has been attended to, the amount of time it has been idle since initiated, and parameters set by the user. Idle time increases momentary priority to ensure that tasks with lower base priority are eventually completed. Interrupted tasks are resumed from the point of interruption.
The attention model also determines when multiple task activities can be performed in parallel and responds to unanticipated external stimuli. The latter function is performed by a general orienting procedure, which is always active and does not require resources. When a stimulus is present, it invokes an appropriate micro-model to determine stochastically whether the operator responds. If so, the appropriate procedure becomes active.
Operation
HOS operation can be described by the following pseudocode procedure:
-
Put mission-level task on active task list
-
While active task list not empty and termination condition not present do
-
Get next step from procedure of highest-priority task
-
Apply selection model to interpret verb-object instruction
-
Invoke micro-models and initiate channel processing, when able
-
Compute and record task times and accuracies
-
Compute new momentary task priorities
-
HOS in its pure form computes task performance metrics (times and accuracies), not actual behaviors that could be used to provide inputs to, say, a tank model. However, it could be adapted to provide such outputs and is in fact being combined with COGNET (discussed above) for that very purpose.
Current Implementation
The current version of HOS, though not fully implemented, is written in C to run on an IBM PC or compatible. Earlier versions were written in FORTRAN and ran much faster than real time on mainframe computers. Major functional elements of HOS (converted to C++) are being incorporated into a new version of COGNET (see above) that runs on a Silicon Graphics Indy.
Support Environment
The HOS operating environment includes editors for creating and modifying objects, and procedures and tools for running HOS models and analyzing the results.
Validation
Many of the individual micro-models are based on well-validated theories, but it is not clear that the overall model has been validated.
Applicability for Military Simulations
HOS has a simple, integrative, flexible architecture that would be useful for its adaptation to a wide variety of military simulation applications. HOS also contains a reasonable set of micro-models for many human-machine system applications, such as modeling a tank commander or a pilot.
On the other hand, HOS has a number of limitations. It is currently a stand-alone system, not an embeddable module. Also, the current status of its micro-models may prevent its early use for modeling dismounted infantry. For example, many of the micro-models are highly simplified in their default forms and require parameterization by the user. The attention model, though reasonable,
appears to be rather ad hoc. The architecture may also cause brittleness and poor applicability in some applications that differ from those for which it was designed. Moreover, HOS computes times, not behavior, though it should be augmentable. Knowledge representation is very simple in HOS and would not likely support sophisticated perception and decision making representations. Finally, the user must define interruption points to allow multitasking.
The integration of HOS with COGNET should overcome many of the limitations of each.
Micro Saint
Micro Saint is a modern, commercial version of systems analysis of integrated networks of tasks (SAINT), a discrete-event network simulation language long used in the analysis of complex human-machine systems. Micro Saint is not so much a model of human behavior as a simulation language and a collection of simulation tools that can be used to create human behavior models to meet user needs. Yet many Micro Saint models have been developed for military simulations (e.g., Fineberg et al., 1996; LaVine et al., 1993, 1996), and the discussion here, which is based on the descriptions of Laughery and Corker (1997), is in that context. It is worth noting that such Micro Saint models are used as part of or in conjunction with other analysis tools, such as WinCrew, IMPRINT, HOS V (see above), task analytic work load (TAWL), and systems operator loading evaluation (SOLE).
Purpose and Use
Micro Saint is used to construct models for predicting human behavior in complex systems. These models, like the HOS models discussed above, yield estimates of times to complete tasks and task accuracies; they also generate estimates of human operator workload and task load (i.e., the number of tasks an operator has to perform, over time). These are not outputs that could be applied as inputs to other simulation elements, though in theory the models could be adapted to provide that capability.
Assumptions
Micro Saint modeling rests on the assumption that human behavior can be modeled as a set of interrelated tasks. That is, a Micro Saint model has at its heart a task network. To the user of these models, what is important is task completion time and accuracy, which are modeled stochastically using probability distributions whose parameters are selected by the user. It is assumed that the operator workload (which may affect human performance) imposed by individual tasks can be aggregated to arrive at composite workload measures. Further assumptions
are that system behavior can be modeled as a task (or function) network and that the environment can be modeled as a sequence of external events.
Architecture and Functionality
The architecture of Micro Saint as a tool or framework is less important than the architecture of specific Micro Saint models. Here we consider the architecture and functionality of a generic model.
The initial inputs to a Micro Saint model typically include estimates (in the form of parameterized probability distributions) for task durations and accuracies. They also include specifications for the levels of workload imposed by tasks. During the simulation, additional inputs are applied as external events that occur in the simulated environment.
Both human operators and the systems with which they interact are modeled by task networks. We focus here on human task networks, with the understanding that system task network concepts form a logical subset. The nodes of a task network are tasks. Human operator tasks fall into the following categories: visual, numerical, cognitive, fine motor (both discrete and continuous), gross motor, and communications (reading, writing, and speaking). The arcs of the network are task relationships, primarily relationships of sequence. Information is passed among tasks by means of shared variables.
Each task has a set of task characteristics and has a name as an identifier. The user must specify the type of probability distribution used to model the task's duration and provide parameters for that distribution. A task's release condition is the condition(s) that must be met before the task can start. Each task can have some effect on the overall system once it starts; this is called its beginning effect. Its ending effect is how the system will change as a result of task completion. Task branching logic defines the decision on which path to take (i.e., which task to initiate) once the task has been completed. For this purpose, the user must specify the decision logic in a C-like programming language. This logic can be probabilistic (branching is randomized, which is useful for modeling error), tactical (a branch goes to the task with the highest calculated value), or multiple (several subsequent tasks are initiated simultaneously). Task duration and accuracy can be altered further by means of performance-shaping functions used to model the effects of various factors on task performance. These factors can include personnel characteristics, level of training, and environmental stressors (see Chapter 9). In practice, some performance-shaping functions are derived empirically, while some are derived from subjective estimates of subject matter experts.
The outputs of a Micro Saint model include mission performance data (task times and accuracies) and workload data.
Operation
Because Micro Saint models have historically been used in constructive (as opposed to virtual) simulations, they execute in fast time (as opposed to real time). The simulation is initialized with task definitions (including time, and accuracy parameters). Internal and external initial events are scheduled; as events are processed, tasks are initiated, beginning effects are computed, accuracy data are computed, workloads are computed, and task termination events are scheduled. As task termination events are processed, the system is updated to reflect task completions (i.e., task ending effects).
Current Implementation
Micro Saint is a commercial product that runs on IBM PCs and compatible computers running Windows. It requires 8 megabytes of random access memory and 8 megabytes of disk space. DOS, OS 2, Macintosh, and UNIX versions are available as well.
Micro Saint is also available in a version integrated with HOS (described above). The Micro Saint-HOS simulator is part of the integrated performance modeling environment (IPME), a network simulation package for building models that simulate human and system performance. The IPME models consist of a workspace design that represents the operator's work environment; a network simulation, which is a Micro Saint task network, and micro-models. These micro-models (which come from HOS) calculate times for very detailed activities such as walking, speaking, and pushing buttons. They provide an interface between the network and the workspace (i.e., the environment), and they offer a much finer level of modeling resolution than is typically found in most Micro Saint networks. The integrated performance package runs on UNIX platforms.
Support Environment
The Micro Saint environment includes editors for constructing task networks, developing task descriptions, and defining task branching decision logic; an expandable function library; data collection and display modules; and an animation viewer used to visualize simulated behavior.
Validation
Since Micro Saint is a tool, it is Micro Saint models that must be validated, not the tool itself. One such model was a workload model showing that a planned three-man helicopter crew design was unworkable; later experimentation with human subjects confirmed that prediction. HARDMAN III, with Micro Saint as
the simulation engine, has gone through the Army verification, validation, and accreditation process—one of the few human behavior representations to do so.
Applicability for Military Simulations
Micro Saint has been widely employed in constructive simulations used for analysis to help increase military system effectiveness and reduce operations and support costs through consideration of personnel early in the system design process. Examples include models to determine the effects of nuclear, biological, and chemical agents on crews in M1 tanks, M2 and M3 fighting vehicles, M109 155-millimeter self-propelled howitzers, and AH64 attack helicopters. Micro Saint models have also been developed for the analysis of command and control message traffic, M1A1 tank maintenance, and DDG 51 (Navy destroyer) harbor entry operations.
Although Micro Saint has not yet been used directly for training and other real-time (virtual) simulations, Micro Saint models have been used to derive tables for human performance decrements in time and accuracy. Those tables have been used in real-time ModSAF simulations.
Micro Saint models of human behavior are capable of computing task times and task accuracies, the latter providing a means for explicitly modeling human error—a feature lacking in many models. The models can also be readily configured to compute operator workload as a basis for modeling multitasking. Used in conjunction with HOS V micro-models, Micro Saint has the capability to represent rather detailed human behaviors, at least for simple tasks. As noted, it is a commercial product, which offers the advantage of vendor support, and its software support environment provides tools for rapid construction and testing of models.
On the other hand, since Micro Saint is a tool, not a model, the user is responsible for providing the behavioral modeling details. Also, in the absence of HOS V micro-models, Micro Saint is most suited for higher levels of abstraction (lower model resolution). Being a tool and lacking model substance, Micro Saint also lacks psychological validity, which the user must therefore be responsible for providing. Knowledge representation is rudimentary, and, other than a basic branching capability, there is no built-in inferencing mechanism with which to develop detailed models of complex human cognitive processes; such features must be built from scratch.
Nevertheless, Micro Saint has already shown merit through at least limited validation and accreditation and has further potential as a good tool for building models of human behavior in constructive simulations. Being a commercial product, it is general purpose and ready for use on a wide variety of computer platforms. It also has been used and has good potential for producing human performance tables and other modules that could be used in virtual simulations. With the micro-model extensions of HOS V and a mechanism for converting
fast-time outputs to real-time events, it is possible that Micro Saint models could even be used directly in virtual simulations.
Man Machine Integrated Design and Analysis System (MIDAS)
MIDAS is a system for simulating one or more human operators in a simulated world of terrain, vehicles, and other systems (Laughery and Corker, 1997; Banda et al., 1991; see also Chapters 7 and 8).
Purpose and Use
The primary purpose of MIDAS is to evaluate proposed human-machine system designs and to serve as a testbed for behavioral models.
Assumptions
MIDAS assumes that the human operator can perform multiple, concurrent tasks, subject to available perceptual, cognitive, and motor resources.
Architecture and Functionality
The overall architecture of MIDAS comprises a user interface, an anthropometric model of the human operator, symbolic operator models, and a world model. The user interface consists of an input side (an interactive GUI, a cockpit design editor, an equipment editor, a vehicle route editor, and an activity editor) and an output side (display animation software, run-time data graphical displays, summary data graphical displays, and 3D graphical displays).
MIDAS is an object-oriented system consisting of objects (grouped by classes). Objects perform processing by sending messages to each other. More specifically, MIDAS consists of multiple, concurrent, independent agents.
There are two types of physical component agents in MIDAS: equipment agents are the displays and controls with which the human operator interacts; physical world agents include terrain and aeronautical equipment (such as helicopters). Physical component agents are represented as finite-state machines, or they can be time-script-driven or stimulus-response-script-driven. Their behaviors are represented using Lisp methods and associated functions.
The human operator agents are the human performance representations in MIDAS—cognitive, perceptual, and motor. The MIDAS physical agent is Jack™, an animated mannequin (Badler et al., 1993). MIDAS uses Jack to address workstation geometry issues, such as the placement of displays and controls. Jack models the operator's hands, eyes, and feet, though in the MIDAS version, Jack cannot walk.
The visual perception agent computes eye movements, what is imaged on the
retina, peripheral and foveal fields of view, what is in and out of focus relative to the fixation plane, preattentional phenomena (such as color and flashing), detected peripheral stimuli (such as color), and detailed information perception. Conditions for the latter are that the image be foveal, in focus, and consciously attended to for at least 200 milliseconds.
The MIDAS updatable world representation is the operator's situation model, which contains information about the external world. Prior to a MIDAS simulation, the updatable world representation is preloaded with mission, procedure, and equipment information. During the simulation, the updatable world representation is constantly updated by the visual perception agent; it can deviate from ground truth because of limitations in perception and attention. Knowledge representation in the updatable world representation is in the form of a semantic net. Information in the updatable world representation is subject to decay and is operated on by daemons and rules (see below).
The MIDAS operator performs activities to modify its environment. The representation for an operator activity consists of the following attributes: the preconditions necessary to begin the action; the satisfaction conditions, which define when an action is complete; spawning specifications, which are constraints on how the activity can be decomposed; decomposition methods to produce child activities (i.e., the simpler activities of which more complex activities are composed); interruption specifications, which define how an activity can be interrupted; activity loads, which are the visual, auditory, cognitive, and psychomotor resources required to perform the activity; the duration of the activity; and the fixed priority of the activity. Activities can be forgotten when interrupted.
MIDAS includes three types of internal reasoning activities. Daemons watch the updatable world representation for significant changes and perform designated operations when such changes are detected. IF-THEN rules provide flexible problem solving capabilities. Decision activities select from among alternatives. Six generalized decision algorithms are available: weighted additive, equal weighted additive, lexicographic, elimination by aspect, satisfying conjunctive, and majority of confirming decisions.
There are also various types of primitive activities (e.g., motor activities, such as ''reach," and perceptual activities, such as "fixate"). Therefore, the overall mission goal is represented as a hierarchy of tasks; any nonprimitive task is considered a goal, while the leaf nodes of the mission task hierarchy are the primitive activities.
MIDAS models multiple, concurrent activities or tasks. To handle task contention, it uses a scheduling algorithm called the Z-Scheduler (Shankar, 1991). The inputs to the Z-Scheduler are the tasks or activities to be scheduled; the available visual, auditory, cognitive, and motor resources; the constraints on tasks and resources (that is, the amount of each resource available and the times those amounts are available); and the primary goal (e.g., to minimize time of completion or balance resource loading). The Z-Scheduler uses a blackboard
architecture. It first builds partial dependency graphs representing prerequisite and other task-to-task relations. Next it selects a task and commits resource time slices to that task. The task is selected on the basis of its criticality (the ratio of its estimated execution time to the size of the time window available to perform it). The Z-Scheduler interacts with the task loading model (see below). The output of the Z-Scheduler is a task schedule. There is also the option of using a much simpler scheduler, which selects activities for execution solely on the basis of priorities and does not use a time horizon.
The MIDAS task loading model provides information on task resource requirements to the Z-Scheduler. It assumes fixed amounts of operator resources (visual, auditory, cognitive, and motor), consistent with multiple resource theory (Wickens, 1992). Each task or activity requires a certain amount of each resource. The task loading model keeps track of the available resources and passes that information to the Z-Scheduler.
Operation
In a MIDAS simulation, declarative and procedural information about the mission and equipment is held in the updatable world representation. Information from the external world is filtered by perception, and the updatable world representation is updated. Mission goals are decomposed into lower-level activities or tasks, and these activities are scheduled. As the activities are performed, information is passed to Jack, whose actions affect cockpit equipment. The external world is updated, and the process continues.
Current Implementation
MIDAS is written in the Lisp, C, and C++ programming languages and runs on Silicon Graphics, Inc., workstations. It consists of approximately 350,000 lines of code and requires one or more workstation to run on. It is 30 to 40 times slower than real time, but can be simplified so it can run at nearly real time.
MIDAS Redesign
At the time of this writing, a new version of MIDAS was in development (National Aeronautics and Space Administration, 1996). The following brief summary is based on the information currently available.
The MIDAS redesign is still object-oriented, but is reportedly moving away from a strict agent architecture. The way memory is modeled is better grounded in psychological theory. MIDAS' long-term memory consists of a semantic net; its working memory has limited node capacity and models information decay.
The modeling of central processing (that is, working memory processes) is
different from that of the original MIDAS. An event manager interprets the significance of incoming information on the basis of the current context (the goals being pursued and tasks being performed). The context manager updates declarative information in the current context, a frame containing what is known about the current situation. MIDAS maintains a task agenda—a list of goals to be accomplished—and the agenda manager (similar to the Z-Scheduler in the previous MIDAS) determines what is to be done next on the basis of the task agenda and goal priorities. The procedure selector selects a procedure from long-term memory for each high-level goal to be accomplished. The procedure interpreter executes tasks or activities by consulting the current context to determine the best method. Motor behavior is executed directly. A high-level goal is decomposed into a set of subgoals (which are passed to the agenda manager). A decision is passed to the problem solver (see below). The procedure interpreter also sends commands to the attention manager to allocate needed attentional resources. The problem solver, which embodies the inferencing capabilities of the system, does the reasoning required to reach decisions.
The redesigned MIDAS can model multiple operators better than the original version. Like the original, it performs activities in parallel, resources permitting, and higher-priority tasks interrupt lower-priority tasks when sufficient resources are not available. It models motor error processes such as slips and it explicitly models speech output.
Support Environment
The MIDAS support environment has editors and browsers for creating and changing system and equipment specifications, and operator procedures and tools for viewing and analyzing simulation results. Currently much specialized knowledge is required to use these tools to create models, but it is worth noting that a major thrust of the MIDAS redesign is to develop a more self-evident GUI that will allow nonprogrammers and users other than the MIDAS development staff to create new simulation experiments using MIDAS. In addition, this version will eventually include libraries of models for several of the more important domains of MIDAS application (rotorcraft and fixed-wing commercial aircraft). The intent is to make the new version of MIDAS a more generally useful tool for human factors analysis in industry and a more robust testbed for human performance research.
Validation
The original version of MIDAS has been validated in at least one experiment involving human subjects. MIDAS was programmed to model the flight crew of a Boeing 757 aircraft as they responded to descent clearances from air traffic control: the task was to decide whether or not to accept the clearance and
if so, when to start the descent. The model was exercised for a variety of scenarios. The experimenters then collected simulator data with four two-pilot crews. The behavior of the model was comparable to that of the human pilots (0.23 ≤ p ≤ 0.33) (Laughery and Corker, 1997).
Applicability for Military Simulations
In both versions, MIDAS is an integrative, versatile model with much (perhaps excess) detail. Its submodels are often based on current psychological and psychomotor theory and data. Its task loading model is consistent with multiple resource theory. MIDAS explicitly models communication, especially in the new version. Much modeling attention has been given to situation awareness with respect to the updatable world representation. There has been some validation of MIDAS.
On the other hand, MIDAS has some limitations. Many MIDAS behaviors, such as operator errors, are not emergent features of the model, but must be explicitly programmed. The Z-Scheduler is of dubious psychological validity. The scale-up of the original MIDAS to multioperator systems would appear to be quite difficult, though this problem is being addressed in the redesign effort. MIDAS is also too big and too slow for most military simulation applications. In addition, it is very labor-intensive, and it contains many details and features not needed in military simulations.
Nevertheless, MIDAS has a great deal of potential for use in military simulations. The MIDAS architecture (either the original version or the redesign) would provide a good base for a human behavior representation. Components of MIDAS could be used selectively and simplified to provide the level of detail and performance required. Furthermore, MIDAS would be a good testbed for behavioral representation research.
Neural Networks
In the past decade, great progress has been made in the development of general cognitive systems called artificial neural networks (Grossberg, 1988), connectionistic networks (Feldman and Ballard, 1982), or parallel distributed processing systems (Rumelhart et al., 1986b; McClelland and Rumelhart, 1986). The approach has been used to model a wide range of cognitive processes and is in widespread use in cognitive science. Yet neural network modeling appears to be quite different from the other architectures reviewed in this section in that it is more of a computational approach than an integrative human behavior architecture. In fact, the other architectures reviewed here could be implemented with neural networks: for example, Touretzky and Hinton (1988) implemented a simple production system and an experimental version of Soar was implemented in neural nets (Cho et al., 1991).
Although the neural network approach is different in kind from the other architectures reviewed, its importance warrants its inclusion here. There are many different approaches to using neural nets, including adaptive resonance theory (Grossberg, 1976; Carpenter and Grossberg, 1990), Hopfield nets (Hopfield, 1982), Boltzman machines (Hinton and Sejnowski, 1986), back propagation networks (Rumelhart et al., 1986a), and many others (see Arbib, 1995, or Haykin, 1994, for comprehensive overviews). The discussion in this section attempts to generalize across them. (Neural networks are also discussed in the section on hybrid architectures, below, because they are likely to play a vital role in overcoming some weaknesses of rule-based architectures.)
Purpose and Use
Unlike the rule-based systems discussed in this chapter, artificial neural networks are motivated by principles of neuroscience, and they have been developed to model a broad range of cognitive processes. Recurrent unsupervised learning models have been used to discover features and to self-organize clusters of stimuli. Error correction feed-forward learning models have been used extensively for pattern recognition and for learning nonlinear mappings and prediction problems. Reinforcement learning models have been used to learn to control dynamic systems. Recurrent models are useful for learning sequential behaviors, such as sequences of motor movements and problem solving.
Assumptions
The two major assumptions behind neural networks for human behavior modeling are that human behavior in general can be well represented by self-organizing networks of very primitive neuronal units and that all complex human behaviors of interest can be learned by neural networks through appropriate training. Through extensive study and use of these systems, neural nets have come to be better understood as a form of statistical inference (Mackey, 1997; White, 1989).
Architecture and Functionality
A neural network is organized into several layers of abstract neural units, or nodes, beginning with an input layer that interfaces with the stimulus input from the environment and ending with an output layer that provides the output response interface with the environment. Between the input and output layers are several hidden layers, each containing a large number of nonlinear neural units that perform the essential computations. There are generally connections between units within layers, as well as between layers.
Neural network systems use distributed representations of information. In a
fully distributed system, the connections to each neural unit participate (to various degrees) in the storage of all inputs that are experienced, and each input that is experienced activates all neural units (to varying degrees). Neural networks are also parallel processing systems in the sense that activation spreads and flows through all of the nodes simultaneously over time. Neural networks are content addressable in that an input configuration activates an output retrieval response by resonating to the input, without a sequential search of individual memory locations.
Neural networks are robust in their ability to respond to new inputs, and they are flexible in their ability to produce reasonable outputs when provided noisy inputs. These characteristics follow from the ability of neural networks to generalize and extrapolate from new noisy inputs on the basis of similarity to past experiences.
Universal approximation theorems have been proven to show that these networks have sufficient computational power to approximate a very large class of nonlinear functions. Convergence theorems have been provided for these learning algorithms to prove that the learning algorithm will eventually converge on a maximum. The capability of deriving general mathematical properties from neural networks using general dynamic system theory is one of the advantages of neural networks as compared with rule-based systems.
Operation
The general form of the architecture of a neural network, such as whether it is feed-forward or recurrent, is designed manually. The number of neural units and the location of the units can grow or decay according to pruning algorithms that are designed to construct parsimonious solutions during training. Initial knowledge is based on prior training of the connection weights from past examples. The system is then trained with extensive data sets, and learning is based on algorithms for updating the weights to maximize performance. The system is then tested on its ability to perform on data not in the training sets. The process iterates until a satisfactory level of performance on new data is attained. Models capable of low-level visual pattern recognition, reasoning and inference, and motor movement have been developed.
The neural units may be interconnected within a layer (e.g., lateral inhibition) as well as connected across layers, and activation may pass forward (projections) or backward (feedback connections). Each unit in the network accumulates activation from a number of other units, computes a possibly nonlinear transformation of the cumulative activation, and passes this output on to many other units, with either excitatory or inhibitory connections. When a stimulus is presented, activation originates from the inputs, cycles through the hidden layers, and produces activation at the outputs.
The activity pattern across the units at a particular point in time defines the
state of the dynamic system at that time. The state of activation evolves over time until it reaches an equilibrium. This final state of activation represents information that is retrieved from the memory of the network by the input stimulus. The persistence of activation produced by stimulus is interpreted as the short-term memory of the system.
Long-term storage of knowledge is represented by the strengths of the connections from one neural unit to another, which are called connection weights. The initial weights represent prior knowledge before training begins. During training, the weights are updated by a learning process that often uses a gradient ascent algorithm designed to search for a set of weights that will maximize a performance function.
Most neural network systems are stand-alone systems that do not have a real-time connection to an information source or the outside world, but some systems do interact with the real world. For instance, the NavLab project uses neural networks for its road-following navigation system and has built 10 robot vehicles (three vans, two HMMWVs, three sedans, and two full-sized city buses), one of which traveled 98.2 percent of the trip from Washington, D.C. to San Diego, California, without human intervention, a distance of 2,850 miles (Jochem et al., 1995). However, even if performance is in real time, the training prior to performance is usually computationally intensive and done off-line.
Current Implementation
Packages for designing and simulating neural network behavior are available from many sources. Some textbooks come packaged with software, for example, McClelland and Rumelhart, 1988, and its successor PDP++ from the Center for the Neural Basis of Cognition (Carnegie Mellon University). Commercial packages abound (and can be found on the Internet by searching for "neural networks"), but they are often not extensible. One useful Windows and menu-based package is available from the commonly used engineering programming language MATLAB. However, it is relatively easy to develop models using any mathematical programming language that includes matrix operators (such as MATHEMATICA).
Support Environment
There are numerous excellent textbooks that can help in designing and programming neural networks (see, e.g., Anderson, 1997; Golden, 1996; Haykin, 1994; Levin, 1991; McClelland and Rumelhart, 1988). Programming documentation for software is available from MATLAB, PDP++, and commercial packages. Many of the newer packages have GUI interfaces and associated editing and debugging tools. Courses in neural networks are commonly taught in cognitive
science, or engineering, or computer science departments at major universities.
Validation
Neural networks applied to particular tasks have been extensively and rigorously tested in both cognitive psychology experiments and engineering applications. A comprehensive review of this large literature is beyond the scope of this chapter, but some examples include word perception and individual word reading (McClelland and Rumelhart, 1981; Seidenberg and McClelland, 1989; Hinton and Shallice, 1991; Plaut et al., 1996), many memory tasks (e.g., Knapp and Anderson, 1984; McClelland and Rumelhart, 1985), control of automatic processes (Cohen et al., 1990), problem solving and reasoning (Rumelhart et al., 1986c; Rumelhart, 1989) and motor control (e.g., Burnod et al., 1992; Kawato et al., 1990).5 The ART architecture, in particular, is supported by applications of the theory to psychophysical and neurobiological data about vision, visual object recognition, auditory streaming, variable-rate speech perception, cognitive information processing, working memory, temporal planning, and cognitive-emotional interactions, among others (e.g., Bradski et al., 1994; Carpenter and Grossberg, 1991; Grossberg, 1995; Grossberg et al., 1997; Grossberg and Merrill, 1996).
Application for Military Simulations
Most neural network applications have been designed to model a very small part of the cognitive system, often sensory/motor processes. We know of no work that models human behavior at the level of reasoning about military strategy and tactics or performance of the tasks to carry out those decisions. Researchers differ in their opinions as to whether it is possible for neural networks to support a general cognitive architecture with high-level reasoning skills involving structured information: see Sharkey and Sharkey (1995) and Touretzky (1995) for opposing views. Given this open research issue, systems composed of sets of neural networks might be feasible for some tasks, or hybrid systems of neural networks and symbolic architectures (discussed further in the last section of this chapter) might be most suitable for military applications. For instance, the NavLab project on autonomous driving (though not a model of human driving behavior) combined neural networks for reactive tasks, such as road-following and obstacle avoidance, with symbolic knowledge and processes to plan and
execute those plans (Pomerleau et al., 1991). An additional issue is that for high-knowledge domains, e.g., such as the responsibilities of a platoon leader, the off-line training required for a neural net system would be very time consuming and computationally expensive.
Operator Model Architecture
The operator model architecture (OMAR) models human operators in complex systems, such as command and control systems, aircraft, and air traffic control systems (Deutsch et al., 1993, 1997; Deutsch and Adams, 1995; MacMillan et al., 1997).
Purpose and Use
The primary purpose of OMAR is the evaluation of operator procedures in complex systems. It is also intended to be used to evaluate system design (in particular the design of displays and controls) and as a testbed for developing human behavior models.
Assumptions
OMAR is based on certain assumptions about attributes of human behavior. The first is that much human behavior is proactive, that is, goal directed. Humans perform multiple tasks concurrently, so OMAR includes explicit representations of goals. Multiple concurrent tasks contend for limited sensory, cognitive, and motor resources, so OMAR models include task instances. Parallel behavior is limited to cases in which some of the concurrent tasks are overlearned and almost automatic, requiring no conscious thought; therefore, in OMAR the execution of such "automatic" procedures is done in parallel with a cognitive task. Some behaviors are rule based, and humans display differing levels of skill in performing activities. A final and very significant assumption is that operators work in teams, so OMAR has provisions for modeling multiple communicating operators.
Architecture and Functionality
The major architectural components of OMAR are a set of knowledge representation languages, a knowledge acquisition environment (including editors and browsers), the target system model, scenario representations, one or more human operator models, compilers, an event-based simulator, online animation tools, and post-run analysis tools.
OMAR can be described as a set of interrelated layers. The bottom layer is the core simulation layer. It is built in the Common Lisp object system. The
simple frame language, used to define simulation objects, adds essential features to the Common Lisp object system. The SCORE language, which is an extension of Common Lisp, adds robust parallel syntax and semantics to support the parallel processing required by the model. Also included is a forward-chaining rule-based language. The core layer simulator is a discrete-event simulator, which uses time-sorted queues of future events called closures. It allows individual threads of execution (parallel execution) and implements asynchronous event monitoring (closure-condition associations).
The perceptor/effector model layer is a place-holder for detailed perceptor (sensory) and effector (motor) models. Default models are provided, and specialized perceptor/effector models have also been developed for event types of particular workplace environments (e.g., commercial aircraft flight decks and air traffic control workstations) and for in-person, telephone, and party-line radio communication to support team-based activities.
At the cognitive level, OMAR consists of agents, which are entities capable of executing goals, plans, and tasks. Generally, a human operator model consists of a single agent, but operator model components (e.g., perception) can be modeled as agents as well. Goals, plans, tasks, and procedures represent proactive components of the human operator model. A goal is an explicit statement of what the agent is to do, specifying the activation circumstances and the procedure for computing the goal's priority (also called activation level). Goals are decomposed into and/or trees. A plan is the set of leaves of a goal tree. A task is the instantiation of a plan; it encapsulates and activates a network of procedures to perform the task and describes the resources needed by the procedures. The goal achievement simulator attempts to execute subgoals and plans that make up goal trees. OMAR tries to catch the interplay between proactive and reactive behaviors: goals and plans set up proactive capabilities, forming a dynamically structured network of procedures responsive to sensory input that "guides" the reactive response to events through pattern matching to appropriate goal-directed behaviors.
In OMAR, tasks compete for perceptor, cognitive, and effector resources. There may be contention within classes of tasks (e.g., two tasks competing for the eyes) or between classes of tasks (e.g., a task to speak vs. a task to listen). Task contention takes into account the dynamic priorities (activation levels) of competing tasks, the class membership of competing tasks, how close tasks are to completion, and the cost of interrupting an ongoing task (inertia). Deliberative (or thoughtful) task scheduling is modeled as well.
Operation
As OMAR runs, stimuli simulated in the external world are processed by perceptor models. Task priorities or activation levels are dynamically computed on the basis of existing conditions. The task with the highest activation level runs
until it is finished or is preempted by another task that has acquired sufficient activation credits. The execution of a task is performed by a network of procedures, approximating a data flow system.
The OMAR operator generally runs serially, with some parallelism for tasks with high levels of automaticity. Effector models simulate behavioral responses that change the simulated environment, and new goals are then created.
Current Implementation
The current implementation of OMAR is programmed in Common Lisp and extensions, as described above, and runs on Sun and Silicon Graphics workstations. At the time of this writing, it was being ported to IBM PCs, and to increase portability, all interface code was being converted to Java. For many applications, the current implementation runs faster than real time.
Support Environment
OMAR's developers have provided a toolkit to facilitate building models of human performance. It consists of a concept editor for creating and modifying concepts (objects and relations that comprise networks), a procedure browser for viewing procedures and procedure networks, a simulator for running the model, and tools for analyzing model performance both during and after a run. A complete manual is available (BBN, 1997).
Validation
Many OMAR submodels are well grounded in psychological theory, but there has been little or no validation of the model as a whole. However, OMAR has been used in mixed human-in-the-loop and model simulations, and the OMAR models seem to interact well with the real human operators.
Applicability for Military Simulations
As noted above, OMAR is an integrative architecture that is well grounded in psychological theory. It appears to be flexible and versatile. A powerful toolkit is available for creating and exercising models. OMAR provides much detail and, important for the simulation of unit behavior, can be used to model teams of operators. Its limitations are that it is a very complex model and may be difficult for users not well trained in behavioral modeling. It is also likely to be labor intensive. Nevertheless, OMAR offers one of the best tradeoffs between capabilities and limitations of all the integrative architectures reviewed here.
Situation Awareness Model for Pilot-in-the-Loop Evaluation (SAMPLE)
SAMPLE is the most recent version of a line of models that began with the procedure oriented crew (PROCRU) model. SAMPLE and its antecedents have been used to represent individual operators, as well as crews of complex human-machine systems (Baron et al., 1980; Zacharias et al., 1981, 1994, 1996). The following discussion takes a general perspective, and not all of the characteristics described apply to every variant.
Purpose and Use
SAMPLE and its antecedents have been used in the examination of crew procedures. Some variations are used to model single operators. Variations of SAMPLE have been used in analysis of the approach to landing procedures of commercial transport aircraft (PROCRU), analysis of antiaircraft artillery system procedures (AAACRU), evaluation of nuclear power plant control automation/decision aiding (crew/system integration model [CSIM]), and air combat situation awareness analysis. Most recently, SAMPLE has been used to evaluate air traffic alerting systems in a free flight environment.
Assumptions
Assumptions basic to SAMPLE are that the behavior of the crew (in some cases an individual operator) is guided by highly structured, standard procedures and driven by detected events and assessed situations. Some variants assume a multitasking environment, though this aspect is less emphasized in the later model. In all cases, however, the crew is concerned primarily with performing situation assessment, discrete procedure execution, continuous control, and communication.
Architecture and Functionality
The SAMPLE architecture consists of a system model and one or more human operator models.
The system model includes system dynamics (e.g., ownship, the plant, or a target), which are modeled by partial differential equations of motion (e.g., point mass equations for vehicle trajectory). The system dynamics can be modeled at any level of complexity desired.
A human operator model exists for each crew member. It consists of sensory and effector channels and several processors.
The sensory channels model visual and auditory sensing. Both are based on an optimal control model with no perceptual delay. They model limitations due to observation noise and stimulus energy with respect to defined thresholds. All
auditory information is assumed to be heard correctly. The operator cannot process all sources of information simultaneously, so the model must decide what to attend to. The attention allocator uses suboptimal attention allocation algorithms for this purpose.
The information processor monitors the system and environment based on inputs from the rest of the simulation. It consists of two time-varying Kalman filters: the continuous state estimator produces estimates and other statistics of continuous system state; the discrete event detector generates occurrence probabilities of operationally relevant event cues.
The situation assessor uses event cue probabilities to generate occurrence probabilities of possible situations facing the operator. Earlier versions of SAMPLE used a fixed, predefined set of possible situations facing the pilot or operator; more recent versions allow for flexible situational definitions by building up a situation from a number of situational attributes (e.g., an overall situation may be defined by the component threat situation, friendly situation, fuel situation, armament situation, and so on). The situation assessor uses belief nets to model the process of situation assessment. Belief net nodes represent situations, events, and event cues detected by the discrete event detector. Belief net arcs (or arrows) represent associative and inferential dependencies between nodes. Situation awareness is modeled as an inferential diagnostic process: event cues are detected, events are deduced, and situations are inferred using Bayesian logic. (See Chapter 7 for a more detailed discussion of this material.)
Procedures form the core of the SAMPLE human operator model. The modeled operator faces a number of procedures or tasks that can be performed, either continuously or in response to events. All crew actions (except decisions about what procedures to perform) are determined by procedures. Procedures may comprise subprocedures; they are interruptible; and they are assumed to be performed essentially correctly, though a wrong procedure can be selected, and errors can result from improper decisions based on lack of information quantity or quality.
There are several classes of procedures, depending on the domain application. In early PROCRU efforts, there are six classes: system control procedures (e.g., aircraft or antiaircraft system control), system monitoring procedures (for system status and events), verbal request and callout procedures, subsystem monitoring and control procedures (e.g., servicing an altitude alert subsystem), verbal acknowledgment procedures, and miscellaneous procedures (for modeling convenience). Procedures are represented by both procedural code and rules. In more recent applications of SAMPLE, procedural classes have been extended to cover a number of tactical air combat procedures.
The execution of a procedure results in a sequence of actions that affect the modeled system and/or its environment. Procedures also compute the time required to complete each associated action. Completion times may be deterministic or sampled from a probability distribution. As mentioned above, procedure execution is assumed to be error free.
The procedure selector is the component that determines which procedure the operator model will execute next. Given an assessed situation, it generates selected procedures, determining which procedure to execute next as a function of expected gain. A procedure's expected gain is a function of a constant relative ''value" of the procedure, the situational relevance of the procedure at the current instant, and time. Expected gain increases with time until the procedure is executed or is declared as missed.
Procedure actions are implemented by the procedure effector, which generates attention requests, control actions, and communication actions. Attention requests are internal "actions" that guide the attentional focus of the sensory channels toward specific information sources. Control actions (which, for example, control the airplane) may be continuous or discrete. Communication actions transfer information to some extrinsic node in the simulation. The information may be about the system state, it may be a command, or it may be about some event.
SAMPLE is implemented as an object-oriented software agent. Each of the key system components (sensory channel, information processor, situation assessor, decision maker, and procedure effector) is a software object that communicates with its neighbors via message passing. The topology of the model is defined by specifying the functionality of each component (which may have its own hierarchy of subcomponents linked via message passing) and the connectivity among the components. Multiple agents communicate with each other by means of the same message-passing methodology, which is based on the command and control simulation interface language (CCSIL) framework used in distributed interactive simulation.
In SAMPLE, no restrictions are placed on the algorithmic composition of any of the object classes, provided they conform to a well-defined class interface. The software interface is structured to facilitate integration and run-time linking of third-party software libraries (e.g., expert system languages such as NASA's CLIPS, numerical algorithms, database access, and any other custom software tools). The topology is defined using a set of ASCII data files that specify the contents and connectivity of each system block.
Operation
SAMPLE operates in the following manner. Continuous system control procedures are always enabled. Events in the system or environment are detected by the discrete event detector, and discrete procedures are enabled by event detections. Enabled procedures compete for the operator's attention on the basis of expected gain, and the individual operator can execute only one procedure at a time. Procedure execution results in actions that affect the simulated system and its environment.
The model generates several outputs, most of them time series. These include system state trajectory information, each crew member's estimate of the
system and environment state, each crew member's attention allocation, system control inputs, a list of significant events, assessed situations, a procedural time line denoting the procedures being executed by each crew member, a message time line indicating communication traffic and auditory signals, and a milestone time line showing important milestones and events as defined by the modeler.
Current Implementation
Recent variants of SAMPLE are written in C++. They run on an IBM PC under the Windows 95 operating system.
Support Environment
SAMPLE has a limited programming environment, but work is under way on developing a graphical editor for model specifications.
Validation
SAMPLE and its descendants have been exercised in a wide variety of analysis projects, so their performance has come under the scrutiny of individuals familiar with human operator performance. However, there has been no formal validation.
Applicability for Military Simulations
The SAMPLE architecture provides a general framework for constructing models of operators of complex systems, particularly in cases in which the operators are engaged in information processing and control tasks. SAMPLE draws heavily on modern control theory, which has enjoyed considerable success in the modeling of human control behavior. The belief net core of the situation assessor of later variants appears to have considerable potential for representing situation awareness. However, procedure development for SAMPLE models would appear to be quite labor-intensive since there seems to be no high-level procedure representation language.
Soar
Soar is a symbolic cognitive architecture that implements goal-oriented behavior as a search through a problem space and learns the results of its problem solving (Newell, 1990; see also Chapters 5 and 8). Complete discussions of Soar describe a hierarchy from an abstract knowledge level down to a hardware- or wetware-dependent technology level (Polk and Rosenbloom, 1994). However, the two middle levels—the problem-space level and the architecture level—are of primary
interest when one is comparing Soar with other cognitive architectures for human behavior representation; these levels are the focus of the following discussion.
Purpose and Use
As a unified theory of cognition, Soar is used to model all the capabilities of a generally intelligent agent. It has the capability to work on the full range of tasks expected of an intelligent agent, from highly routine to extremely difficult, open-ended problems. It interacts with the outside world, either simulated or actual. It represents and uses many different kinds of knowledge, such as that thought of as procedural, declarative, or episodic. Finally, it learns all of these types of knowledge as it performs tasks, learning from instruction, from success and failure, through exploration, and so on.
Soar's development has always involved two factions: (1) artificial intelligence researchers and system builders who wish to use all of Soar's capabilities to solve problems as efficiently as possible, and (2) cognitive psychology researchers who wish to understand human behavior by modeling it in the Soar architecture. The artificial intelligence contingent has driven the development of Soar to very large-scale models that perform rationally, run efficiently in real time, and interact with a real environment (e.g., robots or intelligent forces in a simulated theater of war). The psychological contingent has driven Soar to cognitive plausibility in many areas, such as multitasking performance under time pressure, problem solving, learning, and errors. Although any one Soar program may emphasize the goals of only one of these contingents, both sets of goals could in principle be served by a single Soar model.
Assumptions
Soar assumes that human behavior can be modeled as the operation of a cognitive processor and a set of perceptual and motor processors, all acting in parallel. In general, Soar is another example of a modified stage model (Figure 3.1), with the minor exception that all processors, including the motor processors, communicate through the contents of working memory (not directly from cognition as shown in Figure 3.1). Working memory is represented symbolically and holds the current interpretation of the state of knowledge, which can be considered a Soar model's situation awareness as defined in Chapter 7. However, Soar assumes that working memory cannot be understood independently of learning and long-term memory (Young and Lewis, forthcoming); these memories and processes work together to generate situation awareness.
Soar's research approach has involved adding a few more assumptions, applying the philosophy that the number of distinct architectural mechanisms should be minimized. For example, in Soar there is a single framework for all tasks and subtasks (problem spaces); a single representation of permanent knowledge (productions),
so that there is no procedural/declarative distinction; a single representation of temporary knowledge (objects with attributes and values); a single mechanism for generating goals (automatic subgoaling); and a single elementary learning mechanism (chunking). To date, Soar has been able to model a broad range of human behavior without the need for more complex architecturally supported mechanisms.
Finally, Soar makes quantitative predictions about human behavior, as well as qualitative predictions about which knowledge, associations, and operators will come into play during performing and learning. In particular, Soar assumes that each elementary cognitive operator takes approximately 50 milliseconds to complete. This parameter allows predictions of the time to perform, the time to learn, and interesting interactions with a changing environment (e.g., errors occur because of time pressure).
Architecture and Functionality
At the problem-space level, Soar can be described as a set of interacting problem spaces, where each problem space contains a set of operators that are applied to states to produce new states. A state is a set of attribute-value pairs that encodes the current knowledge of the situation. A task, or goal, in a problem space is modeled by the specification of an initial state and one or more desired states. (Note that the desired states do not have to be completely specified; that is, open-ended problems can be represented by desired states that simply contain more knowledge than the initial state, without the particular type or content of that knowledge being specified.) When sufficient knowledge is available in the problem space for a single operator to be selected and applied to the current state, the behavior of a Soar model is strongly directed and smooth, as in skilled human behavior. When knowledge is lacking, either search in additional problem spaces may be necessary to locate the knowledge or decisions must be made without the knowledge, leaving open the probability of errors and thus error-recovery activities. This more complex branching and backtracking performance models human problem solving behavior.
The architecture is itself a hierarchy. At the lowest level, Soar consists of perceptual and motor modules that provide the means of perceiving and acting upon an external world. At the next level, associations in the form of symbolic productions match the contents of working memory (comprising the inputs of perception, the output parameters for the motor modules, and purely internal structure) to retrieve additional information from long-term memory. In contrast with most classical production systems, Soar's associations match and fire in parallel,6 are limited in their action repertoire to the generation of preferences for
the activation of working-memory structure, and automatically retract these preferences when their conditions no longer match. Associations repeatedly fire and retract until no further associations are eligible to do so; Soar's decision-level process then weighs all the active preferences and chooses a new operator or state. Whenever the activations are not sufficient to allow a unique choice, the architecture responds by setting a subtask to resolve this impasse, and the entire process recurs. If the recursive processing adds new preferences that are active in the original task, Soar's architectural learning mechanism (called chunking) creates a new association between those working-memory structures in the original task that led, through a chain of associations in the subtask, to the new preferences in the original task. Thus chunking effectively transfers knowledge from the subtask space to the original task space. It straightforwardly produces speedup, but can also inductively acquire new knowledge (Rosenbloom et al., 1991).
Operation
Soar has been used in many different ways: as a stand-alone model in which time has no real meaning; in simulated real time, with each decision cycle being defined as taking 50 milliseconds and able to run in synchronicity with other systems constrained to the same time scale; or in real time, interacting with a totally separate, independently acting system. In all models, initial knowledge is encoded by the human modeler in production rules. In many models, the initial state of the model is coded manually, but other models start with no knowledge of the problem state and must acquire that knowledge from the external environment. In some models, the termination state (desired state) is coded manually; in others, the model simply runs until the human modeler stops it. External events can be simulated wholly within Soar with a prototyping facility such as Tcl/Tk, or the model can be linked to a totally independent external system. When run, all Soar models can output a trace of what operators were selected and applied and what knowledge was used to perform the task. In addition, some Soar models literally change the external environment, interacting with real-world systems (e.g., controlling robots, flying a flight simulator, participating in a simulated theater of war). If learning is turned on, the new productions produced by chunking are also a system output; the human modeler can directly examine what the model learned.
Current Implementation
The currently supported version of Soar is Soar 7, a C-based implementation
available for UNIX and Macintosh platforms. Several releases of Soar 6 are available for UNIX, Macintosh, and DOS platforms. Soar 7 replaces the user interface of Soar 6 with Tcl.
Both Soar 6 and Soar 7 have been used with external environments, for example, to control robots and to participate in simulated theaters of war. Depending on the complexity of the task, they can run faster than real time.
Support Environment
Soar is a highly developed programming environment with full-fledged user and reference manuals and program editing, tracing, and debugging facilities. Soar users are an active group, and many programming tools have been developed and shared. For instance, there are emacs-based development environments for UNIX users and a Tcl/Tk environment for those who prefer a GUI. There are also a Macintosh-specific environment specifically targeted at supporting the development of psychological models and a tool for evaluating the match between the behavior of a Soar model and verbal protocol data collected from humans.
Tutorials on Soar are held periodically in both the United States and Europe, and tutorial material is available on the World Wide Web. Workshops are held approximately yearly in the United States and Europe at which Soar users share their models and results. There are also active mailing lists for soliciting assistance and sharing results, and a frequently asked questions (FAQ) site to help users get started.
In addition, there are user libraries of general capabilities and models that can be adopted into new models. For instance, MONGSU is a UNIX socket package enabling external systems to interact more easily with Soar. The SimTime system simulates the passage of real time as a function of the cognitive behavior of a Soar model so independent external events can be simulated without linking up to an external environment. NL-Soar is a general theory of natural-language comprehension and generation that can be incorporated into new models. (Note, however, that according to the Soar theory, natural-language use is highly integrated into the performance of a task, so using this code in the service of modeling military systems such as command and control communication will not be as simple as adding new vocabulary and calling a subroutine.) All of this material can be accessed from the Soar World Wide Web site: <http://www.isi.edu/soar/soar.html>.
Validation
Soar has been evaluated extensively as a cognitive architecture against human behavior in a wide variety of tasks. Although a comprehensive list of Soar models and their validation is beyond the scope of this chapter, some examples of these
models are natural-language comprehension (Lewis, 1996, 1997a, 1997b), syllogistic reasoning (Polk and Newell, 1995), concept acquisition (Miller and Laird, 1996), use of a help system (Peck and John, 1992; Ritter and Larkin, 1994), learning and use of episodic information (Altmann and John, forthcoming), and various human-computer interaction tasks (Howes and Young, 1996, 1997; Rieman et al., 1996). In addition, other work that compares Soar models with human behavior include models of covert visual search (Weismeyer, 1992), abductive reasoning (Krems and Johnson, 1995), a simplified air traffic controller task (Bass et al., 1995), the job of the NASA test director (Nelson et al., 1994a, 1994b), job-shop scheduling (Nerb et al., 1993), and driving a car (Aasman, 1995).
Recent work has joined the Soar cognitive architecture to EPIC's perceptual and motor processors (Chong and Laird, 1997). The resulting perceptual-cognitivemotor model has been validated against human dual-task data and shown to be as accurate as EPIC alone, despite the differences between the cognitive processing of EPIC and SOAR. This approach of incorporating the mechanisms of other architectures and models and "inheriting" their validation against human data promises to result in rapid progress as parallel developments by other architectures emerge.
Applicability for Military Simulations
Soar has been used extensively for military problems. Most notably, the agents of Soar-intelligent forces (Soar-IFOR) have been participants in simulated theaters of war (STOW-E in 1995, STOW-97 in October 1997). These agents have simulated the behavior of fixed-wing and rotary-wing pilots on combat and reconnaissance missions, competing favorably with human pilots. They have acted as very large expert systems, using Soar's architecture to encode knowledge prior to performance and then acting on it, or solving problems using that knowledge. They use this knowledge to act as individuals and as groups of cooperating individuals. However, these systems have not yet run with learning capabilities.
Other research Soar systems have demonstrated more far-reaching capabilities and would require some additional research before becoming robust, real-time systems for military use. An example is the system noted above that integrated natural-language capabilities, visual search, and task knowledge to model the decision process of the NASA test director in simulated real time (slower than actual real time) (Nelson et al., 1994a, 1994b). Another system used ubiquitous learning to store and recall situations and decisions during operation for post-mission debriefing (Johnson, 1994). Although some work has been done on modeling frequency learning in laboratory tasks (Miller and Laird, 1996), it is doubtful that Soar's purely symbolic learning mechanism will extend far enough to capture human-like adaptivity to the frequency of occurrences in real-world environments. In addition, as with most of the other architectures reviewed here, no actual modeling has been done to account for the effects of moderator variables,
although this is an active area of interest for the Soar research community (see Chapter 9).
In summary, the Soar architecture has been shown to be sufficiently powerful to represent believable individual human behavior in military situations and sufficiently robust to run very large-scale models connected to real-world systems in real time (without learning). However, this is not yet true for all aspects of learning or moderator variables.
COMPARISON OF ARCHITECTURES
Table 3.1 presents a summary of the integrative architectures reviewed in this chapter, comparing them across several dimensions that should help the reader assess their relative potential in meeting the needs of intended applications. The following sections describe each of these dimensions in turn, attempting to point out features that distinguish some architectures from others.
The reader should keep two things in mind when comparing the architectures. First, our assessments are based on our interpretations of the materials available to us at the time of writing, and in most cases we have very limited experience in working directly with the architectures. Second, from direct conversations with developers and users of the architectures, it is clear that there were very promising developments taking place at the time of this writing for every architecture reviewed. Each therefore warrants additional scrutiny based on the reader's needs.
The following dimensions are included in Table 3.1 and discussed below: original purpose; submodels (sensing and perception, working/short-term memory, long-term memory, motor); knowledge representation (declarative, procedural); higher-level cognitive functions (learning, situation assessment, planning, decision making); multitasking; multiple human modeling; implementation (platform, language); support environment; and validation.
Original Purpose
The purpose for which the architecture was originally created may influence its usefulness for certain applications. Architectures such as HOS and OMAR were created primarily for evaluating equipment and procedure designs as part of the process of human-machine system development. Though they may have many attractive elements, certain features, such as their execution speed and the type of output they produce, may make them less desirable for modeling human problem solving, which is the express purpose of other architectures, such as ACT-R and Soar.
Submodels
Sensing and Perception
Some of the architectures, such as EPIC and MIDAS, have fairly detailed submodels of human sensory and perceptual processes. Such submodels make these architectures particularly applicable in situations where failures in stimulus detection or discrimination are likely to have a significant effect on overall human performance. Other architectures, such as Micro Saint, model such processes only in terms of times and accuracy probabilities, and still others, such as COGNET, have only very abstract representations of such processes, though they may be perfectly acceptable for applications in which detection and discrimination are not limiting factors. Even in cases in which detection and discrimination are limiting factors, most of the architectures offer modelers the capability to incorporate more sophisticated sensing and perception modules.
Working/Short-Term Memory
As discussed in Chapter 5, working or short-term memory plays a major role in human information processing. Applications in which attention and decision making are important considerations are likely to benefit from veridical representations of working memory. The most sophisticated working memory submodels among the architectures reviewed by the panel are those of ACT-R and the newer version of MIDAS, both of which treat working memory as an area of activation of long-term memory. Other architectures, such as Micro Saint, do not model working memory explicitly, and are therefore incapable of directly capturing performance degradation due to working memory capacity and duration limitations.
Long-Term Memory
All the architectures provide some means of long-term information storage. Some, however, such as HOS and Micro Saint, do not explicitly model such a store. Rather, such information is implicitly stored in procedures and task networks, respectively.
Motor
The architectures reflect a wide range of motor submodels. The motor submodels of HOS and Micro Saint provide time and accuracy outputs. EPIC, ACT-R, and Soar have effectors that change the environment directly. MIDAS and OMAR drive the Jack animated mannequin, though the computational costs of that submodel may be too great for most simulations. OMAR has also developed
TABLE 3.1 Integrative Architectures
|
Submodels |
|||||
Architecture |
Original purpose |
Sensing and Perception |
Working/Short-Term Memory |
Long-Term Memory |
Motor |
Outputs |
ACT-R |
Model problem solving and learning |
Perceptual processors: visual, auditory, tactile |
Activation-based part of long-term memory |
Network of schema-like structures plus productions |
Motor processors: manual, vocal, oculomotor |
Behaviors |
COGNET |
Develop user models in intelligent interfaces, surrogate users and adversaries |
Abstract—perceptual daemons, with provision of user-defined models |
Extended working memory through multipanel blackboard |
Multipanel blackboard |
Abstract, with provision for user-defined models |
Behaviors |
EPIC |
Develop and test theories of multiple task performance |
Perceptual processors: visual, auditory, tactile |
Unlimited capacity and duration |
Propositions and productions |
Motor processors: manual, vocal, oculomotor |
Behaviors |
HOS |
Generate timelines for HMS analysis and evaluation |
Visual and auditory sensing and perception micro-models |
Micro-model with limited capacity and decay |
Not explicitly modeled |
Eye, hand, trunk, foot, etc., micro-models |
Metrics |
Micro Saint based network tools |
Evaluate systems and procedures |
Detection/identification probabilities, times, and accuracies |
Not explicitly modeled |
Not explicitly modeled |
Times and accuracies plus micro-models |
Metrics |
MIDAS |
Evaluate interfaces and procedures |
Visual perception agent |
Not explicitly modeled |
Semantic net, updatable world representation |
Jack-animated mannequin |
Behaviors |
MIDAS Redesign |
Evaluate interfaces and procedures |
Visual and auditory perception |
Subset of nodes in long-term memory, limited capacity and decay |
Frames |
Jack-animated mannequin |
Behaviors |
Neural network based tools |
Multiple constraint, satisfaction in memory, language, thought, pattern recognition |
Visual and auditory perception |
Activation-based limited capacity |
Connection weights |
Sensor/motor integration, occularmotor |
Behaviors |
OMAR |
Evaluate procedures and interfaces |
Default perceptor models |
Emergent property—not explicitly modeled |
Not explicitly modeled—network of frames |
Default effector models |
Behaviors |
SAMPLE |
Evaluate crew procedures, equipment |
Visual, auditory channels (optimal control model-based) |
Not explicitly modeled |
Procedural knowledge base |
Time-delayed procedural actions |
Behaviors |
Soar |
Model problem solving and learning |
Perceptual processors: visual, auditory |
Unlimited capacity, duration tied to goal slack |
Productions |
Motor processors: manual, vocal, oculomotor |
Behaviors |
|
Knowledge Representation |
Higher-Level Cognitive Functions |
||||
Architecture |
Declarative |
Procedural |
Learning |
Planning |
Decision Making |
Situation Assessment |
ACT-R |
Schema-like structures |
Productions |
Weight adjustment, production strength adjustments, new productions, new schemas |
Creates new plans |
Knowledge-based, Bayesian |
Overt and inferred |
COGNET |
Blackboard elements, semantic network |
Goal hierarchies, COGNET task description language |
No learning |
Instantiates general plans |
Knowledge-based |
Overt and inferred |
EPIC |
Propositions |
Productions |
No learning |
Instantiates general plans |
Knowledge-based |
Overt and inferred |
HOS |
Object attributes stored in short-term memory |
HOS procedural language |
No learning |
No planning |
None |
Overt |
Micro Saint based network tools |
Global variables |
Micro Saint language, task networks |
No learning |
No planning |
Knowledge-based |
Overt |
MIDAS |
Objects |
Functions, daemons, rules, decision procedures |
No learning |
Instantiates general plans |
Knowledge-based, Bayesian |
Overt |
MIDAS Redesign |
Frames |
Plan frames, rules, functions |
No learning |
Instantiates general plans |
Knowledge-based, Bayesian |
Overt |
Neural network based tools |
Active: pattern activation Latent: connection weights |
Sequences of activation patterns over time |
Weight updating by gradient ascent on objective function |
Motor planning |
Competitive systems of activation |
Primarily inferred |
OMAR |
Augmented frames (built on CLOS) |
SCORE language (built on Common Lisp) |
No learning |
Instantiates general plans |
Knowledge-based, Bayesian |
Overt |
SAMPLE |
Objects |
Production rules |
No learning |
Instantiates general plans |
Knowledge-based, Bayesian |
Overt |
Soar |
Productions |
Productions |
Learning by chunking (flexible—see text) |
Creates new plans |
Knowledge-based |
Overt and inferred |
|
Multitasking |
||||
Architecture |
Serial/Parallel |
Resource Representation |
Goal/Task Management |
Multiple Human Modeling |
Implementation Platform |
ACT-R |
Serial with switching (no interruptions) |
Amount of declarative memory activation |
None (one goal at a time) |
Potential through multiple ACT models |
Mac, PC (limited) |
COGNET |
Serial with switching and interruptions |
Limited focus on attention, parallel motor/perceptual processors |
Pandemonium model—priorities based on task context |
Through multiple COGNET models |
PC, SGI Sun |
EPIC |
Parallel |
Limited perceptual and motor processors, unlimited cognitive processor |
Priority-based task deferment |
No |
Lisp platforms |
HOS |
Serial with switching, plus parallel movement possible |
Speech, hand, foot, and cognitive channels |
Priority-based attention switching |
No |
PC |
Micro Saint based network tools |
Parallel with switching to serial resources limited |
Visual, auditory, cognitive, psychomotor workload |
Simple dynamic prioritization |
Yes |
PC, Mac, Unix platforms |
MIDAS |
Resource-limited parallel |
Visual, auditory, cognitive, motor resources |
Z-Scheduler uses time, resource constraints |
Limited |
SGI |
MIDAS Redesign |
Resource-limited parallel |
Visual, auditory, cognitive, motor resources |
Agenda Manager uses time and resource constraints, goal priorities |
Yes |
|
Neural network based tools |
Contention scheduling via competition among components |
Units and connections allocated to a task |
Competitive systems of activation |
Potential through multiple networks |
MAC, PC, Unix platforms |
OMAR |
Serial with some parallelism for automatic tasks |
Perceptor, cognitive, effector resources |
Tasks compute own priorities |
Yes |
Sun, SGI, PC (future) |
SAMPLE |
Serial with interruptions |
Sensory, cognition, and action channels |
Priority based on base value + ''situational relevance" |
Yes |
PC, Unix |
Soar |
Serial with switching and interruptions |
Serial cognitive processor, limited perceptual and motor resources |
Preference-based attention allocation |
Yes |
Mac, PC, Unix platforms |
Architecture |
Language |
Support Environment |
Validation |
Comments |
ACT-R |
Lisp |
Editors, debuggers |
Extensive at many levels |
ACT-R models focus on single, specific information processing tasks; has not yet been scaled up to complex multitasking situations or high-knowledge domains |
COGNET |
C++ |
Graphical editors, syntax/semantic checkers, debuggers, structural SPI to external processes |
Full model |
Used in low-fidelity submarine training simulations and high-fidelity AEGIS CIC training simulations to provide surrogate operators and adversaries |
EPIC |
Lisp |
Lisp programming environment tools |
Extensive for reaction time |
EPIC models focus on simple, dual-task situations; has not yet been scaled up to complex multitasking situations or high-knowledge domains |
HOS |
FORTRAN |
Editors (mostly text-based) |
Components (micro-models) |
Currently capable of scripted behaviors only |
Micro Saint based network tools |
C, C++ |
Editors, debuggers |
Some micro-models; at least one implementation |
Used extensively in military simulations |
MIDAS |
Lisp, C, C++ |
Graphical editors, graphical data displays |
Full model |
Currently capable of scripted behaviors only |
MIDAS Redesign |
C++ |
Similar to original MIDAS |
None |
In development |
Neural network based tools |
C, NETLAB |
Commercial products, GUIS |
Extensive for component tasks |
Focus on sensory/motor integration, have not yet been scaled up to complex multitasking situations or high-knowledge domains |
OMAR |
Lisp |
Compilers, editors, browsers, online animation tools, post-run analysis tools |
Components |
|
SAMPLE |
C++ |
Editors |
Control tasks (OCM) |
Has been used in small-scale military simulations |
Soar |
C |
Editors, debuggers |
Extensive at multiple levels |
Has been used in military simulations (e.g., synthetic theater of war-Europe [STOW-E], STOW-97) |
KATE, a highly-simplified stick figure mannequin that can execute OMAR tasks. Many of the architectures can be extended by building custom motor modules.
Most of the architectures generate outputs in the form of behaviors of the human being represented (e.g., motions, gestures, utterances) that can or could be used as inputs to other simulation modules, such as tank or aircraft models. HOS (in its original version) and Micro Saint, however, generate performance metrics, such as task start and end times and task accuracy data. The former are more applicable for virtual simulations, which need to generate visible images of human and system behaviors, while the latter are more applicable to constructive simulations. In principle, however, it should be possible to modify the two systems to produce such behaviors; in fact, as discussed earlier, modified HOS micro-models have recently been added to COGNET for that very purpose.
Knowledge Representation
Declarative
Representation of declarative knowledge ranges from simple variables to complex frames and schemas. Those applications in which complex factual knowledge structures must be represented explicitly would be better served by the more sophisticated techniques of such architectures as ACT-R and OMAR.
Procedural
All the architectures, except neural net architectures, have a means of representing procedural knowledge beyond that provided by the languages in which they are written. The production rule languages of ACT-R and Soar appear to be the most flexible and powerful, though the related complexity of such languages may not be warranted for those applications in which behavior is highly procedural (perhaps even to the point of being scripted).
Higher-Level Cognitive Functions
Learning
Only three architectures offer learning: ACT-R, neural net architectures, and Soar. Of these, ACT-R provides the most flexibility.
Situation Assessment
In most of the architectures, situation assessment is overt, in the sense that
the programmer/modeler must explicitly code all its aspects. Some of the architectures, however, have the capability of inferring details of the situation from other information. This capability obviously reduces the programmer's burden, provided the mechanism is suitable for the application.
Planning
HOS and Micro Saint do not provide a planning function (though presumably the programmer can implement it). Several of the architectures (e.g., MIDAS) have the capability of instantiating general plans based on the specifics of a given situation. ACT-R and Soar models can create new plans. This capability is obviously valuable for some applications; however, for those applications not requiring planning (i.e., where procedures can be initiated in a prespecified way), the development and computational overhead may be undesirable.
Decision Making
HOS does not provide decision making capability, except to account for the time required for the operator to make a decision given certain characteristics of the decision problem. Most of the other architectures provide for knowledge-based decision making, with a few offering Bayesian techniques. Some form of decision making capability seems essential for most military simulations, and architectures such as ACT-R, OMAR, and Soar seem most capable in this regard.
Multitasking
All the architectures permit multitasking in some sense, though ACT-R enforces strict serial execution of tasks with no interruptions allowed. The degree of multitasking varies from that of HOS, which allows only a single cognitive task (with parallel, ballistic body motion possible) to EPIC, which permits any number of concurrent tasks provided resource requirements are not exceeded. From the standpoint of multitasking flexibility, then, architectures such as EPIC and MIDAS appear to offer the greatest potential. However, there is disagreement over the psychological validity of such systems, and architectures such as OMAR that limit the operator to only one task requiring conscious thought may be more realistic. It is clear, however, that interruptability is an important feature; all architectures, except ACT-R, have this feature.
Multiple Human Modeling
In principle, multiple copies of any of the architectures could be integrated to allow modeling of multiple operators. However, only about half of the architectures
explicitly provide this capability. It is worth noting that this capability was a major driving force in the development of OMAR and the MIDAS redesign.
Implementation
Platform
Most of the architectures have been implemented on one or only a few computer platforms. COGNET and OMAR are more flexible, and Micro Saint and Soar are available for almost any platform a user could require.
Language
For those users needing to modify the architectures to fit the needs of their applications, the language in which the architectures are written is of some concern. To many military simulation modelers who have done most of their work in FORTRAN and C, architectures such as ACT-R, EPIC, and OMAR (written in Lisp) may pose some (small) problems.
Support Environment
The software provided to assist the modeler in creating models in the various architectures ranges widely. At one extreme is EPIC, for which there exists very little such software; at the other extreme is OMAR, whose toolkit appears well thought out and tailored to the user not intimately familiar with OMAR's inner workings. To their credit, though, ACT-R and Soar both have a very large base of users, many of whom have developed additional tools to facilitate the use of the two systems.
Validation
Some of the architectures are too new for any substantive validation to have been done. In other cases (e.g., HOS), certain submodels have been extensively validated. Some of the architectures have been validated in their entirety ("full model" validation in Table 3.1), some of these extensively. At least one (Micro Saint) has instances that have received military accreditation. Unfortunately, most of the validation of "full models" has been based on subjective assessments of subject matter experts, not real human performance data. This last consideration dictates considerable caution on the part of users.
HYBRID ARCHITECTURES: A POSSIBLE RESEARCH PATH
As discussed above, the various architectures have different strengths and
weaknesses with respect to their relevance for military simulations. To model all the details of the complex behavior involved in the tank platoon's hasty defense described in the vignette of Chapter 2, an architecture would have to encompass all the phenomena addressed in all the following chapters: attention and multitasking, memory and learning, decision making, situation assessment, and planning, all subject to behavioral moderators. No existing architectures approach this all-encompassing capability. This does not make these architectures useless—many have been demonstrated to be quite useful despite their limitations—but it does suggest areas of improvement for each.
One research and development path is obvious: start with one of the existing architectures, and expand it in the areas in which it is weak. For example, an emerging ACT architecture, ACT-R/PM, adds EPIC-like perception and motor capabilities to the ACT-R architecture (Byrne and Anderson, 1998). Each of the reviewed architectures could be expanded in this way, as needed for task performance. This approach represents a time-honored path with almost guaranteed incremental payoff, but may eventually encounter boundaries as the architectures reach their limits of expressibility.
Another research and development path might prove more fruitful: combine the strengths of two or more architectures to produce a hybrid that better encompasses human phenomena. A simple example, the combination of Soar's cognitive processor with EPIC's perceptual and motor processors, has already been mentioned. In contrast with ACT-R/PM's reimplementation of EPIC-like processors in the ACT architecture itself, neither Soar nor EPIC was rewritten, but communicate through a shared working memory. More fundamental combinations of architectures are the subject of an ongoing basic research program at the Office of Naval Research (Hybrid Architectures as Models of Human Learning), which supported the infancy of several hybrid architectures. To address the effects of environmental frequency in Soar, that architecture was combined with Echo (Thagard, 1989), a statistical technique for belief updating. Neural nets were augmented with a symbolic explanation-based learning system (Dietterich and Flann, 1997) to address the learning of long procedures when the effects of actions are widely separated from the actions, credit and blame are difficult to assign, and the right combination of moves should count more toward learning than the myriad of failures along the way. The CLARION architecture (Sun, 1995) integrates reactive routines, generic rules, learning, and decision making to develop versatile agents that learn in situated contexts and generalize resulting knowledge to different environments. Gordan (1995) extends Marshall's schema theory of human learning, supplementing its high-level planner with a low-level stimulus-response capability. Marshall (1995) is using a neural net for the identification component of schema theory and ACT-R for the elaboration, planning, and execution components. Cohen and Thompson (1995) use a localist/connectionist model to support rapid recognitional domain reasoning, a distributed
connectionist architecture for the learning of metacognitive behavior, and a symbolic subsystem for the control of inferencing that mediates between the domain and metacognitive systems.
These preliminary architectural explorations are promising examples of an area ripe for research. Through extensive use of the reviewed architectures, researchers and developers in the field now know enough about their relative strengths and weaknesses to judiciously explore plausible combinations for achieving the greatest impact of human behavior models.
CONCLUSIONS AND GOALS
None of the architectures described in this chapter is precisely what is needed for military simulations. Collectively, however, they offer a foundation on which to build models that will be truly useful and practical for military simulations. Once thorough task analyses of the combatant's activities have been conducted to determine exactly what behaviors are to be modeled, these architectures offer frameworks and a wide variety of detailed models of specific perceptual, cognitive, and motor processes that can be used to represent these behaviors. Elements of the various architectures could be combined to yield representations with psychological validity, model fidelity, and computational efficiency.
The problem with these architectures is not what they do not model. Among the architectures reviewed here, there is hardly an interesting and potentially useful phenomenon that is not considered in some way. The problem is that the architectures are not validated. Many of these architectures have submodels that are well validated and have reached a level of maturity suitable for application in military simulations. However, few of the architectures have been validated overall, and their emergent properties are not well understood. More experience with them is needed. A careful, measured expansion of their application in military simulations may just be the proper path to take.
Short-Term Goals
-
Make validation of integrative architectures a priority. Continued use of any integrative architecture or tool should be predicated on its validation. Early effort will be required to define performance measures and standards for use in the validation, as well as scenarios to be used as test cases. Data from exercises and human-in-the-loop simulations can be collected in preparation for validation of models to be developed, and human behavior models currently used in military simulations can be tested against these data.
-
As a first cut at upgrading these architectures, gradually incorporate the concepts, theories, and tools presented in subsequent chapters of this report into existing simulations to incrementally improve existing models. One approach to this end would be to use a simplified stage model to augment current human
-
behavior representations. Such an augmented representation should incorporate, at a minimum, a module representing human perceptual processes, a module representing motor processes, a means for representing multiple active tasks (e.g., planning, decision making, communicating, moving), and a mechanism for selecting a subset of active tasks for execution at any given time. The perceptual module could be based on simple detection and identification probability data derived from existing psychological data. Similarly, the motor process module could be based on simple movement models that yield time and accuracy estimates, or on time and accuracy probabilities derived from human performance data. All current human behavior representations have some means of representing tasks, and most of these could be modified to permit the existence of more than one task at a time. The mechanism for selecting among competing tasks could be based on a simple, static priority system with task priorities derived from task analyses. Such an approach would yield simple architectures with greater face validity than that of current architectures. Although it is unlikely that such architectures would be entirely satisfactory, they would give modelers more experience in developing and validating human behavior representations.
Intermediate-Term Goals
-
Continue validation into the intermediate term as more sophisticated integrative architectures are developed. This generation of architectures can be expected to draw on the integrative architectures reviewed in this chapter, as well as newer architectures that will emerge after this report is published.
-
Continue the development of hybrid architectures, such as those described in this chapter, combining the best elements of existing and emerging integrative architectures. For such hybridization to proceed in a timely manner, it will also be necessary to conduct research and development activities to modularize existing architectures and yield interchangeable components.
-
Apply these architectures in sustained and intensive development of human behavior representations that incorporate specific military tasks in selected domains, such as tank warfare.
-
Compare the different modeling approaches by developing alternative architectures for a domain and comparing them against data from field exercises and human-in-the-loop simulations.
Long-Term Goals
-
Continue architecture validation.
-
Continue to refine new architectures created in the intermediate term.
-
In addition to continued efforts to improve the quality of existing modeling approaches, explore entirely new approaches that will result in architectures as yet unconceived.