Proceedings of a Workshop
IN BRIEF | |
February 2018 |
Advances in Causal Understanding for Human Health Risk-Based Decision-Making
Proceedings of a Workshop—in Brief
Scientists have long understood that a person’s exposure to substances in the environment is linked to health outcomes. However, efforts to control exposure to environmental pollutants without understanding the ways in which those exposures and the health outcomes are linked may not affect the burden of illnesses or diseases in a population. Successful medical or public health interventions can be informed, in part, on being able to scientifically establish causality—that an environmental exposure gives rise to human health or disease states. Causality is more than a “link”; it is a demonstration that environmental exposure(s) are responsible for specific health outcome(s).
What are the best ways to determine whether an environmental exposure, such as a drinking water contaminant, causes an adverse outcome, such as acute gastrointestinal illness? Information about a given exposure or disease is available from a number of traditional sources, such as animal toxicology and human epidemiology studies, and newer research sources, such as in vitro (e.g., cell-based) assays and the rise of the -omics technologies.
As discussed in a series of related National Academies of Sciences, Engineering, and Medicine (National Academies) reports, Toxicity Testing in the 21st Century: A Vision and a Strategy (2007) and Exposure Science in the 21st Century: A Vision and a Strategy (2012), and Using 21st Century Science to Improve Risk-Related Evaluations (2017), the newest data streams that can yield important information about a disease, health state, or effect of an exposure or stressor include in vitro technologies, toxicogenomics and epigenetics, molecular epidemiology, and exposure assessment. These new molecular and bioinformatic approaches have advanced understanding of how molecular pathways are affected by exposure to environmental chemicals and which pathways are involved in disease. Research from newer and traditional approaches also reveal that multiple different insults and exposures can result in the same disease outcome. The converse, that one particular insult or exposure can lead to different health outcomes, has also been demonstrated. However, the ability of emerging or non-traditional methods to establish causality in public health risk assessments remains unclear. Therefore regulators have still largely relied on more traditional endpoints, such as those observed in animal studies, to assess risk.
Scientific tools and capabilities to examine relationships between environmental exposure and health outcomes have advanced and will continue to evolve. Researchers are using various tools, technologies, frameworks, and approaches to enhance our understanding of how data from the latest molecular and bioinformatic approaches can support causal frameworks for regulatory decisions. For this reason, on March 6–7, 2017, the National Academies’ Standing Committee on Emerging Science for Environmental Health Decisions, held a 2-day workshop to explore advances in causal understanding for human health risk-based decision-making. The workshop, sponsored by the National Institute of Environmental Health Sciences (NIEHS), aimed to explore different causal inference models, how they were conceived and are applied, new frameworks and tools for determining causality, and ultimately discusses gaps, challenges, and opportunities for integrating new data streams for determining causality. Where possible, examples from outside the environmental health field were highlighted and ensuing discussions focused on ways the environmental health field could learn from these examples. This workshop brought together environmental health researchers, toxicologists, statisticians, social scientists, epidemiologists, business and consumer representatives, science policy experts, and professionals

from other fields who utilize different data streams for establishing causality in complex systems to discuss the topics outlined above. This Proceedings of a Workshop—in Brief summarizes the discussions that took place at the workshop.
CURRENT FRAMEWORKS AND THE NEED TO SHIFT
Kim Boekelheide of Brown University described the causal understanding between a person’s exposure to a chemical in the environment and a plausible health outcome as a “black box.” This black box is essentially the focus of the workshop, he explained. The approaches currently in use to ascertain what takes place inside the black box draw on work dating back to the 19th century by pioneers such as John Snow and his work establishing that cholera is a waterborne infectious disease and the Henle-Koch postulates for determining whether an infectious organism causes a particular disease. Later, in mid-20th century, the rise of lung cancer mortality led scientists and public health professionals to search for an explanation. Yet, due to the nature of the disease, they were unable to apply the Henle-Koch postulates, said Jon Samet of the University of Southern California’s Institute for Global Health. This led to the development of guidelines for causal inference that accommodated reliance on observational evidence and were used in the landmark 1964 report of the Surgeon General on smoking and health. This way of thinking about causal inference also led to Sir Austin Bradford Hill publishing a set of criteria in 1965, now known as the “Bradford Hill criteria,” which is the most frequently used framework for causal inference in epidemiologic studies (see Figure 1-1). However, the use of the Bradford Hill criteria is continually challenged as research and technology advance. In recent years, this evolution includes the expanded focus on the totality of exposures that can influence human biology, including psychosocial factors such as stress, poverty, and racial discrimination, as well as new data on genomic and epigenomic vulnerabilities, Boekelheide said.
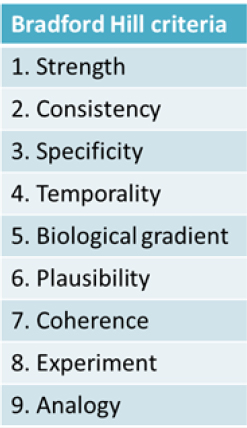
Though not necessary to establish causality, Samet described how six of the Bradford Hill criteria (see Figure 1-1) can aid researchers using newer tools and data to study causality. First, the cause should precede the effect (temporality). Second, stronger links (i.e., statistical associations) are more likely to be causal (strength). Also, increasing the dose should increase the risk of the outcome (biological gradient); removing the exposure should lower the risk of the outcome (experiment); and researchers should be able to consistently replicate findings (consistency). Last, causal associations should be biologically plausible (plausibility).
The new paradigm for investigating links between exposures and disease, which is consistent with a multi-factorial view of disease, involves identifying key precursor events and pathways leading to an apical health endpoint. Expert judgment is currently the best way to integrate in vivo data with in vitro data, such as is generated by -omics tools, according to the 2017 National Academies report Using 21st Century Science to Improve Risk-Related Evaluations. Samet, who chaired the committee that produced that report, stressed a key report finding that insufficient attention has been given to analysis, interpretation, and integration of various data streams from exposure science, toxicology, and epidemiology.
THE REGULATORY PERSPECTIVE ON NEW DATA STREAMS
The reauthorization of the Toxic Substances Control Act (TSCA) in the United States and the European Union’s Registration, Evaluation, Authorisation and Restriction of Chemicals (REACH) program1 allow for the incorporation of new tools to test the broader universe of thousands of substances that potentially could impact humans or the environment in a different manner. During his presentation, Gary Ankley of the U.S. Environmental Protection Agency (EPA), reiterated a point from a recent publication, “the data needed to address these types of legislated mandates cannot realistically be generated using traditional whole animal testing approaches,” which are historically employed for chemical risk assessment.2 The causality frameworks used by EPA and the International Agency for Research on Cancer (IARC) have and will continue to evolve to accommodate novel data sources, said Vince Cogliano of EPA, who also works with IARC. The
_______________
1 See http://ec.europa.eu/environment/chemicals/reach/reach_en.htm.
2 LaLone, C., Ankley, G.T., Belanger, S.E., Embry, M.R., Hodges, G., Knapen, D., Munn, S., Perkins, E.J., Rudd, M.A., Villeneuve, D.L., et al. (2017). Advancing the adverse outcome pathway framework—An international horizon scanning approach. Environmental Toxicology and Chemistry 36(6):1411-1421.
earliest frameworks addressed epidemiologic studies and bioassays using animal models, which are still considered the standard. During the 1990s and early 2000s, these frameworks evolved to address mechanistic data, as mechanistic understanding grew and new assays came into use. Similarly, when EPA scientists realized that the agency’s Endocrine Disruptor Screening Program, called for by Congress, could not achieve its goals with conventional animal testing, the program pivoted to consider a pathway-based approach, pointed out Stanley Barone of EPA. The EPA began using evidence from various cell lines and systems, as well as animal toxicology and other mechanistic studies, and later began to incorporate that information into a causal model framework, he said. The advent of 21st-century data sources presents similar opportunities, Cogliano said. Ankley emphasized the importance and need to develop cost-effect predictive tools that will provide information regarding a chemical’s potential impact to fill gaps in empirical data. Additionally, utilization of the adverse outcome pathway framework (AOP), a conceptual framework that describes existing information about a sequence of events between a molecular stimulus and an adverse outcome in a manner relevant to risk assessment, could be used to identify key events that warrant further investigation. Ankley also pointed out that the recent explosion in technology in the biological sciences enables the collection of large amounts of pathway-based molecular and biochemical data, including high-throughput in vitro testing that enables the efficient and rapid generation of knowledge concerning the effects of chemical perturbation on biological systems. Ankley suggested that the data generated from these technological advances now need to be applied to the decision-making and risk assessment process.
The National Toxicology Program (NTP) must follow the requirements of the Interagency Coordinating Committee on the Validation of Alternative Methods (ICCVAM) Authorization Act of 2000, said John Bucher of NTP and NIEHS. The Act stipulates that new or alternative tests need to be at least as predictive as the tests that are currently used to support actions by regulatory agencies. He said NTP is finding situations where new assays compare more favorably with human databases than with animal databases, which paradoxically remain the “gold standard”, and are the program’s required comparator for new test development. To make full use of the new data, the scientific community faces several challenges, among them to develop case studies that test approaches for integrating new data into inferences about causality; better understand the linkages between human diseases and the pathways through which they develop; communicate the findings of large datasets to decision-makers and other stakeholders; better understand the uncertainties associated with the use of new data; and promote discussion among scientists and stakeholders about the use of new data in various decision contexts. The scientific community will have to think about how to gain confidence in causality determinations from new data and how much data are enough, Cogliano said. Boekelheide observed that simply clarifying how the new approaches contribute to identifying the hazards and risks of exposures for federal risk assessments can be significant. Several workshop attendees also stressed the importance of finding ways to recognize the impact of decisions not to take action.
Some groups are already beginning to incorporate these novel data streams. For example, IARC’s “2B”criteria for identifying substances “possibly carcinogenic to humans” allows the use of new data, said Kathryn Guyton of IARC. She said that it helps the agency be comprehensive in how it assesses the data for carcinogenesis. Bucher said that NTP is adapting elements of systematic review to address questions in environmental health. This involves sorting out ways to integrate evidence from human (usually observational) studies, experimental animal studies, and mechanistic studies (much of which is coming from new approaches consistent with the technologies put forth in Toxicity Testing in the 21st Century).
California’s Department of Toxic Substances Control includes a program that examines evidence from a number of data streams as to whether compounds included in products raise safety concerns that require the state to take action, explained the agency’s Meredith Williams. In such cases, the agency works with manufacturers to analyze whether a safer alternative exists. It is currently setting policy priorities for regulating product chemical combinations that could impact workers, and recently completed workshops on nail salons, perfluorinated compounds, and the aquatic impacts of compounds of substances containing triclosan and nonylphenol ethoxylates. She outlined the criteria used to prioritize the product chemical combinations, (1) potential exposure to chemical in product, and (2) potential for exposure to contribute significant or widespread adverse impact, while stressing that these criteria are not numerical in nature and not a traditional risk assessment. Williams also suggested that grouping chemicals logically, either by mechanism of action or similar chemistry, would help toward accelerating the pace at which the agency can review and regulate chemicals. She alluded to ongoing work by EPA where it is taking a different approach to systematic review. This was raised at various points throughout the workshop by Barone and others, explaining that instead of looking for all the outcomes of a particular chemical, they were interested in finding all of the chemicals that can produce a known outcome, or stimulate
a particular pathway. Such an approach would allow researchers to feed in information from a multitude of data streams related to a particular outcome.
LEVERAGING EPIDEMIOLOGY
Given that cancers can be caused by a multitude of agents (such as viruses, bacteria, behavioral factors, hormonal factors, and environmental factors) and the human evidence comes from observational studies, researchers at IARC have taken a new approach for incorporating novel data streams. This approach is informed by a new method for systematically organizing and evaluating mechanistic data on human carcinogens based on 10 key characteristics. Martyn Smith of the University of California, Berkeley, explained that the approach grew out of the suspicion that mechanistic data alone might be able to determine if a compound was a carcinogen. The genesis of the project was the work of a team led by Cogliano for Volume 100 of IARC’s Monographs on the Evaluation of Carcinogenic Risk to Humans3 that raised questions about how to globally evaluate what we know about how human carcinogens cause cancer and concordance between human and animal studies. The method’s authors, which include Guyton, Cogliano, and Smith, began by focusing on evaluating the data based on what was known about the hallmarks of cancer, the properties of cancer cells, as well as a computational study4 showing “that chemicals that alter the targets or pathways among the hallmarks of cancer are likely to be carcinogenic.”5 Their work was informed by the efforts of another group, the Halifax Project,6 which used the hallmark framework for studying linkages between mixtures of commonly encountered chemicals and the development of cancer.
As discussed in the 2017 Using 21st Century Science to Improve Risk-Related Evaluations report, the “characteristics include components and pathways that can contribute to a cancer. For example, “modulates receptor-meditated effects” includes activation of the aryl hydrocarbon receptor [(AhR)], which can initiate downstream events many of which are linked to cancer, such as thyroid-hormone induction, xenobiotic metabolism, pro-inflammatory response, and altered cell-cycle control.” Only a few of the 10 characteristics are assessed via the high-throughput in vitro assays in EPA’s Toxicity Forecaster (ToxCast) and the Tox21 program jointly run by EPA, NTP, and the National Institutes of Health Chemical Genomics Center, Smith commented. Bucher said that a number of the Tox21 tests cover characteristics including receptor-mediated endpoints, stress response pathways, and DNA repair. He added that NTP is moving toward a transcriptomic approach that will cover more of the characteristics in the next few years. Smith and Bucher indicated that revisions of and expansions to the programs are merited.
Guyton said that the characteristics can be used as a way to categorize mechanistic data around a specific agent of interest. She characterized the holistic view of the data made possible by the new method as a “revolutionary” way to gain insight into and comprehensively assess a multi-causal disease with complicated network and feedback loops. Indeed, the recent Using 21st Century Science to Improve Risk-Related Evaluations report proposed that scientists develop key characteristics for other adverse outcomes and health hazards similar to those developed for cancer. These could then be used as a basis for understanding assay results for a particular compound and for evaluating the risk associated with that compound.
Mary Beth Terry of the Columbia University Mailman School of Public Health spoke about insights gleaned from her work on breast cancer prevention and risk assessment, which involves data on genetics and environmental exposures. The main genes associated with familial breast cancer are also those that are involved with sporadic disease, which means that the discovery of these types of genes can be valuable for all women, explained Terry. However, she reiterated a point from her recent publication that many epidemiological studies “do not include a substantial proportion of subjects with a cancer family history, and are therefore not enriched for underlying genetic susceptibility.”7 This can limit the ability to detect gene–environment interactions.
_______________
3 See http://monographs.iarc.fr/ENG/Monographs/PDFs/index.php.
4 In Vitro Perturbations of Targets in Cancer Hallmark Processes Predict Rodent Chemical Carcinogenesis, https://doi.org/10.1093/toxsci/kfs285.
5 Smith, M.T., Guyton, K.Z., Gibbons, C.F., Fritz, J.M., Portier, C.J., Rusyn, I., DeMarini, D.M., Caldwell, J.C., Kavlock, R.J., Lambert, P.F., Hecht, S.S., Bucher, J.R., Stewart, B.W., Baan, R.A., Cogliano, V.J., and Straif, K. (2016). Key characteristics of carcinogens as a basis for organizing data on mechanisms of carcinogenesis. Environmental Health Perspectives 124(6):713-721.
6 Harris, C.C. (2015). Cause and prevention of human cancer [Editorial]. Carcinogenesis 36(Suppl 1):S1.
7 Shen, J., Liao, Y., Hopper, J.L., Goldberg, M., Santella, R.M., and Terry, M.B. (2017). Dependence of cancer risk from environmental exposures on underlying genetic susceptibility: An illustration with polycyclic aromatic hydrocarbons and breast cancer. British Journal of Cancer 116:1229-1233.
Terry’s research group uses a family-based population cohort enriched with individuals across the risk spectrum to look at the risk of developing breast cancer when exposed to environmental factors that are suspected carcinogens. They apply the BOADICEA8 breast cancer risk prediction model, which considers only genetic breast cancer risk factors to estimate the association between a measured exposure to polycyclic aromatic hydrocarbons (PAHs), which include suspected carcinogens. Terry found a very strong association between people who have high levels of exposure to PAHs (for example, from air exhaust or cooking fumes) and high levels of genetic risk for breast cancer. “It’s a proof of principle that shows that when you get large exposure and high [genetic] risk, you see much stronger effects,” she explained. She emphasized the utility of using enriched population cohorts as a method to enhance the ability to detect gene–environment interactions.
Paolo Vineis of Imperial College London described his molecular epidemiology research that combines epidemiology with laboratory studies, including molecular biomarkers and other -omics, to study causality. Molecular epidemiology is not intended to find biomarkers, per se, he explained, but to research the continuum of disease development from early exposures, via biomarkers. Biomarker research supports causal reasoning by linking exposures with disease via mechanisms and emphasizing causality as a process, he said. Vineis also described the idea of using a person’s genomic sequence for determining exposures in keeping with the concept of the exposome, which encompasses the sum of a person’s environmental exposures from conception. Sequencing the genome may bring to light somatic mutations linked to a known exposure that could serve as a biomarker, or fingerprint, of an individual’s exposure. Vineis is a member of a research team that recently published a study in Science9 involving the analysis of genetic “signatures” of smoking by looking for somatic mutations and DNA methylation in samples from 5,243 cancers of types for which tobacco smoking confers an elevated risk. The work brought to light at least five distinct ways that smoking can damage DNA, results consistent with the hypothesis that increased somatic mutations caused by smoking can increase cancer risk.
Molecular epidemiology approaches also have promise for shedding light on obesity, a complex disease with a number of different potential causes, said Jessie Buckley of the Johns Hopkins Bloomberg School of Public Health. She noted that opportunities exist in exploring biologic pathways, phenotyping, and innovative study designs. However, more work is needed to determine the public health relevance of associations with molecular markers of obesity. Being able to identify exposures that give rise to obesity will help determine the impact of public health interventions aimed at reducing exposures or countering the effects of exposures. “We want to know how many cases of obesity could be avoided,” Buckley explained. Understanding the effect of an intervention allows for a more informed cost–benefit analysis. Buckley described a systems approach of compiling data in order to shed light on the types of interventions that will be useful and successful. One connection made via this systems framework was the potential to increase individual physical activity among the general population within a city by following traffic control measures that limited the number of privately owned vehicles on the road and forced more to utilize public transit systems or walk. In comparison, a recent study suggested that the most effective way to reduce childhood obesity is for mothers to exclusively breastfeed for 6 months, which, Buckley added, is an intervention that has been demonstrated not to be very practical and unlikely to be a successful strategy to reduce obesity.
Eric Tchetgen Tchetgen of the Harvard T.H. Chan School of Public Health has been working on using negative controls for indirect adjustment of unobserved confounding in causal inference from observational data. Initially employed by experimental biologists, the approach generally involves repeating an experiment in such a way that is it expected to support the null hypothesis and then confirming that it does. Tchetgen Tchetgen explained that the negative controls can be employed in the observational studies used in epidemiology “to help to identify and resolve confounding as well as other sources of error, including recall bias or analytic flaws,” which was outlined in his 2010 publication.10 For example, he described a 2006 study11 by Jackson et al. that investigated whether confounding played a role in an observational analysis of the effect of influenza vaccination in the elderly. The study suggested “a remarkably large reduction in one’s risk of hospitalization for pneumonia/influenza hospitalization and also in one’s risk of all-cause mortality in the
_______________
8 BODICEA stands for Breast and Ovarian Analysis of Disease Incidence and Carrier Estimation Algorithm.
9 Alexandrov, L.B., et al. (2016). Mutational signatures associated with tobacco smoking in human cancer. Science 354(6312):618-622.
10 Lipsitch, M., Tchetgen, E.T., and Cohen, T. (2010). Negative controls: A tool for detecting confounding and bias in observational studies. Epidemiology 21(3):383-388.
11 Jackson, L.A., et al. (2006). Evidence of bias in estimates of influenza vaccine effectiveness in seniors. International Journal of Epidemiology 35(2):337-344.
following season.” One of Jackson’s negative control analyses was based on the fact that people are often vaccinated in autumn, while influenza transmission is often minimal until winter. The researchers reasoned that the vaccine protective effect should be strongest during the influenza season, if there is a causal link. When the team analyzed a cohort study with a Cox proportional hazards model they found that the protective effect was strongest before the influenza season and weakest after the season, suggesting that confounding accounts for a substantial part of the observed protection. That confounding was most likely due to changes in the injury and trauma hospitalization rates among the study group and most likely independent of the vaccine. This gave the appearance of a vaccine protective effect against hospitalizations, emphasizing a need to consider protective effects limited to outcomes plausibly linked to influenza.
STATISTICAL MODELING AND COMPUTATIONAL TOOLS
The use of statistical modeling to determine causality has evolved to become useful in situations where there are a lot of variables, such as genes, but not much other knowledge, to compute plausible hypotheses that can be explored experimentally or otherwise, said Richard Scheines of Carnegie Mellon University.
Originating in work done in causal graphical models by Judea Pearl, Peter Spirtes, Clark Glymour, and Richard Scheines in the early 1990s, the methods underlying the models Scheines’ group uses are designed to operate within the reality that model space grows “super-exponentially” with the number of variables. The models are designed to search through variables that represent effects to calculate the distribution of the probability that the variables are causal. The main goal is to suggest causal hypotheses that are novel, significant, and likely valid, Scheines explained. These hypotheses can then be tested experimentally. For example, the method was used to identify causal influences in cellular signaling networks involving measurements of multiple phosphorylated protein and phospholipid components. This was done by applying machine learning to create a Bayesian network of the signaling components sampled using thousands of individual primary human immune system cells to uncover relationships and predict novel interactions. The tools can be useful with both observational and experimental data in a wide variety of research contexts, including systems with latent confounding, systems with feedback, time series, non-linear systems, and psychometric measurement models.
Kristen Beck of IBM explained how IBM is using computational tools to process enormous datasets using machine learning for detecting food standard safety concerns. The company’s Metagenomics Computation and Analysis workbench is an informatics service capable of analyzing metagenomics and metatranscriptomic sequence data to identify potential microbial hazards and confirm the authenticity of food products. The project is helping researchers understand the microbiome of food ingredients so that with careful study they will be able to detect changes and monitor potential contamination that could lead to supply chain disruption. This use of -omics tools in this manner requires a robust computing infrastructure and the use of reliable and well-curated reference databases, said Beck.
DEMONSTRATING THE CHALLENGE OF DETERMINING CAUSALITY
The workshop included mock debates in order to illustrate the challenges faced by decision-makers who must use expert judgment to assess evidence in the scientific literature in order to establish causality. The first debate focused on the challenge of relying on existing epidemiology studies where there are conflicting results. The second debate examined the challenge faced by those wanting to apply a read-across approach for similar chemicals. The third debate explored the use of in vitro human cell-based tests to replace animal models. The following statements made by the speakers in the debates should not be taken as their professional opinion, but as their fulfillment of a role assigned to them for this illustrative exercise.
Debate 1: Methylmercury is a contributing cause to cardiovascular disease; thus, this effect should be considered in methylmercury cost–benefit analyses.
In support of the debate statement, Gary Ginsberg of the Connecticut Department of Public Health presented several studies that highlighted the potential of mercury exposure to cause cardiovascular disease. He began with epidemiological studies by Roman and colleagues12 that recommended the development of a dose–response function relating methylmercury with myocardial infarction (MI) for use in regulatory benefits analysis. He then presented two prospective studies in Finnish populations were presented as part of the evidence that supports the relationship between
_______________
12 Roman, H.A., et al. (2011). Evaluation of the cardiovascular effects of methylmercury exposures: Current evidence supports development of a dose–response function for regulatory benefits analysis. Environmental Health Perspectives 119(5):607-614.
MI and methylmercury and found both temporal and dose–response associations: Virtanen et al. (2005)13 and Salonen et al. (2000);14 the latter going as far as to deem mercury a stronger risk factor for MI than cigarette smoking. Another study,15 using a case-control paradigm also supported a dose–response association. Ginsberg continued with three other epidemiological studies to support the association between contributors to cardiovascular disease in Faroese men,16 Polish chemical workers,17 and Italian fish eaters.18 Lastly, a contrary prospective study19 was also presented, explaining a potential protective effect of methylmercury, as measured in toenail clippings, was likely due to the beneficial effects of consuming fish with high levels of omega-3 fatty acids. Ginsberg also pointed to the analogous decision advising pregnant women to limit their fish consumption due to the effects of methylmercury on neurodevelopment, despite a similar preponderance of mixed results from epidemiology studies; asking the question, if the evidence is sufficient to protect infants why is it not sufficient to protect against MI?
Ginsberg ended his argument by discussing the plausibility of an adverse outcome pathway from animal and in vitro studies, including evidence that mercury exposure can impact pathways involved in oxidative stress and protein denaturation, to demonstrate a biologically plausible mechanism. He discussed a study20 that demonstrated exposure to methylmercury can decrease levels of serum paraoxonase/arylesterase 1 (PON1), and having low levels of this enzyme is known to be a risk factor for MI. Mercury has been shown to impact oxidative stress leading to an increase in phospholipase activity and Cyclooxygenase (COX) pathway activation and decreases in nitric oxide. Given that endothelial cells are sensitive to oxidants, the effects could plausibly lead to damage demonstrating a plausible biological pathway linking mercury to MI. In fact, Ginsberg concluded by stating that Arctic fishermen have been shown to have decreases in PON1 as their methylmercury levels increase.21
Melissa Perry of The George Washington University’s Milken Institute School of Public Health began her rebuttal of Ginsberg’s argument by reviewing the Bradford Hill criteria (as outlined in Figure 1-1) and considering the evidence for mercury and cardiovascular disease. She stated that it was clear that confounding, bias, or additional variables could be influencing whether or not there is a relationship between mercury exposure and MI, given the studies outlined by Ginsberg. The hypothesis that mercury causes MI through an oxidative stress pathway is biologically plausible, but the mixed findings of the prospective studies do not clearly show that the outcome is preceded by the effect, negating support for the demonstration of temporality. No randomized controlled trials showed that MI could be induced by methylmercury dosing or that MI rates could be decreased by reducing methylmercury exposure. Perry also argued that the strength of association is not terribly strong given that both studies supporting the link had odds ratios of 2 or less. She added that analyzing the biological gradient, or dose–response, also was not terribly persuasive.
Considering other issues that regulators generally take into account also does not provide a clear picture, referring to reproducibility, specificity, analogy, and coherence. From the perspective of reproducibility Perry argued that only a handful of studies with mixed findings are available to demonstrate the association. The specificity and ability to control for confounders of the studies could be called into question. When considering the analogy raised by Ginsberg on the effects of neurodevelopment, Perry pointed to the long and established history of toxicology studies (animal and in
_______________
13 Virtanen, J.K., et al. (2005). Mercury, fish oils, and risk of acute coronary events and cardiovascular disease, coronary heart disease, and all-cause mortality in men in eastern Finland. Arteriosclerosis, Thrombosis, and Vascular Biology 25(1):228-233.
14 Salonen, J.T., et al. (2000). Mercury accumulation and accelerated progression of carotid atherosclerosis: A population-based prospective 4-year follow-up study in men in eastern Finland. Atherosclerosis 148(2):265-273.
15 Guallar, E., et al. (2002). Mercury, fish oils, and the risk of myocardial infarction. New England Journal of Medicine 347(22):1747-1754.
16 Choi, A.L., et al. (2009). Methylmercury exposure and adverse cardiovascular effects in Faroese whaling men. Environmental Health Perspectives 117(3):367-372.
17 Skoczynska, A., et al. (2010). The cardiovascular risk in chemical factory workers exposed to mercury vapor. Medycyna Pracy 61(4):381-391.
18 Buscemi, S., et al. (2014). Endothelial function and serum concentration of toxic metals in frequent consumers of fish. PLoS ONE 9(11):e112478.
19 Mozaffarian, D., and J.H. Wu (2011). Omega-3 fatty acids and cardiovascular disease: Effects on risk factors, molecular pathways, and clinical events. The Journal of the American College of Cardiology 58(20):2047-2067.
20 Ginsberg, G., et al. (2014). Methylmercury-induced inhibition of paraoxonase-1 (PON1)-implications for cardiovascular risk. Journal of Toxicology and Environmental Health, Part A 77(17):1004-1023.
21 Ayotte, P., et al. (2011). Relation between methylmercury exposure and plasma paraoxonase activity in inuit adults from Nunavik. Environmental Health Perspectives 119(8):1077-1083.
vitro) demonstrating the biological link between neurodevelopment deficits and methylmercury exposure. The findings between human and animal studies related to cardiovascular endpoints have been thus far inconclusive, raising concerns about coherence. Perry closed her remarks by indicating the need to continually examine the existing evidence and design new studies to be able to better address a given issue, citing the self-correcting nature of science and the need to give regulators an opportunity to return to a decision once newer evidence is generated that may result in a change of decision.
Debate 2: Read-across from in vitro data and in-silico modeling provides a sufficient basis for presumption of endocrine activity of dodecylphenol and derivation of health reference values.
Lesa Aylward of Summit Toxicology presented the supporting argument for the use of the “read-across” technique. She introduced read-across techniques as a way to apply data from a tested chemical for a particular property or effect, such as cancer or reproductive toxicity, to predict potential effects of an untested chemical. Read-across can be applied qualitatively or quantitatively, particularly for groups of similar chemicals, and it is being developed as a risk assessment tool. The European Chemicals Agency (ECHA) guidelines22 call for basing read-across strategies in in vivo data using source compounds with structures and properties that mirror those of the target compound. The recommendations for quantitative read-across in the recently released Using 21st Century Science to Improve Risk-Related Evaluations call for researchers to identify appropriate analogs and investigate their toxicity data; select a point of departure and adjust it on the basis of pharmacokinetics or structural activity data; and then to apply appropriate uncertainty factors. She explained that this case study explores the use of in vitro data in lieu of bracketing in vivo data for a quantitative risk assessment. Aylward also stressed that the specific context matters when deciding to apply the read-across technique.
Turning to the chemical of interest, Aylward explained that dodecylphenol is considered an alkylphenol, a class of high-production volume chemicals used as intermediates in the production of a wide variety of chemical products. Endocrine activity has been demonstrated for well-studied analogs of dodecylphenol. Aylward argued that both qualitative and quantitative data exist for both in vitro and in vivo testing of analog compounds and that multiple in vitro and in silico tools suggest likely endocrine activity. As a group, para-alkylphenol compounds have been identified as having the potential to cause developmental and reproductive toxicity (DART) effects: “alkyl groups with 7 to 12 carbons (branched and unbranched) show relatively strong estrogen receptor (ER) binding effects.”23 Based on its chemical structure, para-dodecylphenol is similar to the para isomers of butylphenol, octylphenol, and nonylphenol, each of which has in vivo toxicity data. These compounds have been tested in the ToxCast program in a variety of high-throughput screening in vitro assays relevant to potential ER activity. EPA scientists have developed an ER pathway model that integrates results from 18 in vitro assays to identify true ER agonists/antagonists with high sensitivity and specificity, validated against in vivo data.24 The model produces a semi-quantitative assessment of the activity related to 17-alpha-ethinyl estradiol. The integrated assessment of potential ER activity finds that dodecylphenol’s activity is likely similar to octylphenol and nonylphenol, which is plausible because of the similarity in their structures.
Aylward concluded by saying that in keeping with the report’s recommendations, the in vivo toxicity data for p-nonylphenol can be used as a point of departure and uncertainty factors can be applied to obtain a health reference value. These uncertainty factors may be adjusted to account for uncertainty regarding relative pharmacokinetics of the two compounds and reliance on in vitro data.
Patrick McMullen of ScitoVation began his opposing argument by discussing how read-across is anchored in in vitro data. He explained that this new approach of read-across is using the observation of a health endpoint in vivo for a tested chemical, comparing how the tested chemical performs in in vitro assays to the untested chemical, and using that knowledge to infer the untested chemical’s health endpoint. He went on to state that the prospect of using this approach quantitatively requires structural similarity to be established. However, the alkylphenols produced by chemical manufacturers are mixtures of branched isomers with poorly characterized physical, chemical, and toxicological properties. Given that the composition of these mixtures is likely to vary substantially across sources and batches, their toxicological
_______________
22 See https://echa.europa.eu/support/guidance.
23 Wu, S., et al. (2013). Framework for identifying chemicals with structural features associated with the potential to act as developmental or reproductive toxicants. Chemical Research in Toxicology 26(12):1840-1861.
24 Judson, R.S., et al. (2015). Integrated model of chemical perturbations of a biological pathway using 18 in vitro high-throughput screening assays for the estrogen receptor. Toxicological Sciences 148(1):137-154.
properties are also like to vary. This presents challenges in establishing structural similarity, given that branched and straight-chain alkylphenols are structurally distinct and can differ in their three-dimensional space. The chain lengths of molecules also have a large impact on physical chemical properties. In fact, while referring to a study by Mansouri and colleagues (2016),25 McMullen stated that the estimated octanol water partition coefficients for p-dodecylphenol and p-nonylphenol differ by two orders of magnitude.
McMullen next emphasized that when considering the toxicokinetics of the two compounds, a difference in the lipophilicity of the untested chemical compared to the tested chemical is likely to impact serum binding, uptake, and metabolism, as well as in vitro kinetics to ensure that what the assays are measuring is a realistic representation of the dose being tested. Specifically, for this example, he stated that alkylphenol clearance is highly dependent on chain length and there is much less transport of higher-chain molecules than of ones with shorter chains.26
Finally, when comparing the values from the ToxCast estrogen receptor battery of assays for p-dodecylphenol and p-nonylphenol, the data demonstrate that the values are near the cytotoxicity limit and are activating non-specific endpoints at similar concentrations. McMullen argued that this suggests the current in vitro data provide little specificity in understanding p-dodecylphenol’s mode of action. He concluded his remarks by stating that while there is value in incorporating in vitro data into any weight of evidence framework, but it is important to be realistic about the assumptions made and the magnitude of the uncertainty surrounding them.
Debate 3: Animal models provide a sufficient basis for presumption for toxicity in safety testing.
Reza Rasoulpour of Dow AgroSciences presented the supporting argument by comparing the success of the in vitro assays to model the action of the ER to the difficulty of modeling the immune system in vitro. He began by discussing how the action of the immune system is much more complex and therefore harder to model with in vitro assays. For example, the immune system includes both innate and adaptive immunity, physical and chemical barriers, multiple cell types and tissues, and this system changes throughout the life stages and in response to different exposures.
Rasoulpour then described skin sensitization assays as an example of the ways that immune system function is evaluated in vivo. Specifically, the local lymph node assay, a mouse model used to study skin sensitivity, measures the replication and expansion of immune cells in the local lymph nodes. This reaction following a skin exposure is a hallmark of a skin sensitization response. Rasoulpour went on to explain that among the in vitro alternatives to the local lymph node assay is the KeratinoSens™ assay.27 It uses a luciferase reporter gene under the control of the antioxidant response element (ARE) that skin sensitizers have been reported to induce. Small electrophilic substances such as skin sensitizers can act on the sensor protein, Keap1 (Kelch-like ECH-associated protein 1), resulting in its dissociation from a transcription factor that activates ARE. The activation of the ARE induces the transcription of the luciferase reporter gene, providing a positive result. The KeratinoSens™ assay was validated for use as part of the European Union’s Integrated Approaches to Testing and Assessment.
He argued that while this assay appears to work well, he cautioned that we often oversimplify when we try to mimic the outcomes of in vivo assays with in vitro test methods. He continued that the range of possible indicators of immunotoxic effect that can occur following exposure to chemicals include increased mortality due to infections, neoplasia, altered hematology, changes in tissue structure, and changes in histopathology. How can one recapitulate these outcomes in in vitro systems?
Rasoulpour next argued that immunity is difficult to recapitulate and the scientific community is simply not there yet, referencing work by Hartung and Corsini, which identified 13 major challenges that need to be overcome to address immunotoxicology with in vitro tools. Some of these challenges are the interference of the test materials with the in vitro assay systems and the ability to study biotransformation, toxicokinetics, the interaction of different cell types, and the induction of the memory response in in vitro systems. He described it as an example of a situation that is good in theory but bad in practice.
_______________
25 El Mansouri, L., et al. (2016). Phytochemical screening, antidepressant and analgesic effects of aqueous extract of anethum graveolens l. from southeast of Morocco. American Journal of Therapeutics 23(6):e1695-e1699.
26 Daidoji, T., et al. (2003). Glucuronidation and excretion of nonylphenol in perfused rat liver. Drug Metabolism and Disposition 31(8):993-998.
27 See http://www.oecd.org/chemicalsafety/testing/Draft_Keratinosens_TG_16May_final.pdf.
Rasoulpour ended his presentation of the supporting argument by posing a series of questions that still need to be addressed. Looking at one particular aspect of a molecular pathway, if a signal is seen in an in vitro setting, does that actually mean anything when it is translated to a whole animal system? Will some small perturbation actually result in pushing the spectrum one way or another? In vitro tools may provide a snapshot into aspects of a pathway in toxicology, but can they integrate the continuum between immune enhancement and immunosuppression that would occur within an in vivo system? For these in vitro tools, to what extent do we know the difference and the statistical significance or even the signal of something happening and to what extent can we put it into context with biological significance? Ultimately, he concluded that animal models should remain the gold standard for immunotoxicology; otherwise, we will simply have a false sense of security.
Norbert Kaminski of Michigan State University presented the counter argument for this debate beginning with stating that the target species for which scientists are doing regulatory assessments is Homo sapiens. However, historically, scientists have chosen to do their studies using animals for ethical reasons. He posed the question of whether a rodent, the most common animal used in these studies, is equivalent to a human, and explained that very little human data exist for many areas of research, but a great deal of literature exists comparing animal testing and human responses in clinical trials. He concluded that often it is found that the animal response does not replicate the human response.
Kaminski went on to discuss a large study demonstrating that genomic responses in mouse models poorly mimic human inflammatory responses. This study analyzed differences in the immune responses among humans following blunt trauma, burn injuries, or exposures to low-dose bacterial endotoxins, and also compared the human immune responses to the mouse immune responses to the same three stressors. The study demonstrated a correlation between the gene expression changes in humans following burn and trauma injuries, respectively. A similar, yet more moderate, correlation was found when comparing the gene expression in humans after endotoxin exposure to the gene expression following burn and trauma injuries. Kaminski went on to point out that there was no correlation between the mouse immune response to endotoxin exposure and mouse trauma. More telling, this study demonstrated virtually no correlation between the gene expression for mouse burns and human burns, mouse trauma and human trauma, or mouse endotoxemia and human endotoxemia.
In another example, Kaminski next explained that following exposure to 2,3,7,8-Tetrachlorodibenzo-p-dioxin (TCDD), an environmental pollutant that stimulates the AhR, only 28 genes were differentially expressed in primary B cells from humans, mice, and rats. However, not all of the genes expressed necessarily had anything to do with B cell function, suggesting “that despite the conservation of the AhR and its signaling mechanism, TCDD elicits species-specific gene expression changes.”28 He continued that, in fact, comparing the human and mouse genomes from the Encyclopedia of DNA Elements (ENCODE) project reveals significant differences. Particularly, in the regulatory regions some of the transcription factor binding elements appear in different places. The situation is similar for enhancer regions: different transcription factors are controlling the regulatory regions of orthologous genes.
However, Kaminski posited that none of this should be surprising because humans diverged from rodents between 70 and 75 million years ago. He concluded his presentation of the counter argument by stating that animal models, especially rodents, may be poor surrogates of human biological responses to exogenous stimuli. Additionally, scientists are now able to recapitulate much of what is known about the cell-to-cell interactions of the immune system “in the in vivo situation” in vitro.
THE WAY FORWARD
During the discussion that followed the debates both Aylward and Ginsberg commented that the decision- to take no action because insufficient evidence is available to quantitatively assess an endpoint is essentially the same as a determination of no risk. Aylward and Ginsberg both stressed that making a decision not to regulate or otherwise take action about a potentially harmful compound can have significant impacts. Aylward went on to say that regulatory scientists do not generally factor the consequences of no action or being wrong into their decision-making processes.
Perry added that the gold standard of prospective studies to establish temporality takes a tremendous amount of time, energy, and investment before a decision is made. Holding back decisions until causality has been established is unrealistic at times and regulators need to be more nimble in order to make decisions in the context of the best available evidence. Rasoulpour suggested that the current regulatory environment is not sufficiently agile, transparent, or quan-
_______________
28 Kovalova, N., R. Nault, R. Crawford, T.R. Zacharewski, and N.E. Kaminski. (2017). Comparative analysis of TCDD-induced AhR-mediated gene expression in human, mouse and rat primary B cells. Toxicolology and Applied Pharmacology 316:95-106.
titative enough to be able to be adjusted appropriately for some kinds of decisions. How the situation may change, in terms of both process and the use of more new data, as the result of the TSCA reform is not yet clear.
In the current situation where expert judgment plays a crucial role, it is important to bear in mind that two experts confronted with the same set of data may interpret it quite differently, Rasoulpour said. For example, the same set of data for a given molecule can be judged differently by scientists in the United States, Brazil, and various European countries. Perry expressed a similar sentiment when asking, to what extent do we abandon deductive and inductive reasoning in our approaches when computational approaches could be extracting out actual associations? She pointed out that the agnostic nature of utilizing computational methods to draw associations between outcomes and exposures may get the field to move away from such reliance on human judgment.
McMullen indicated that the field is beginning to capitalize on the large amounts of data already available for some compounds, such as ones suspected of impacting the endocrine system, to produce proofs-of-principle to illustrate the value of using in vitro tools in some contexts by showing how the results can be comparable to in vivo testing. He added that many methods exist for manipulating cell-based in vitro tests to characterize variability using the tests themselves, as well as computationally, to simulate the impact of a range of exposures on a population. Smith agreed on the need for and value of such tests for aiding in the identification of risk from new and untested chemicals. He also commented on the potential value of designing medium-throughput models to identify both in vitro and in vivo effects in models such as zebrafish and perhaps genetically modified mice. Approaches like chemoproteomics may allow researchers to quickly amass large amounts of information that could help identify and prioritize what to focus on regarding epidemiology and toxicology studies, he said.
Kaminski acknowledged that better in vitro models are needed because the existing models do not simulate all biological processes or effects in the intact organism. He pointed out that the organ-on-a-chip tools being developed hold promise in the future for helping scientists more quickly and reproducibly collect data on the impacts that are difficult to mimic with single-cell systems, such as cell-to-cell interactions and interactions across organ systems. He emphasized that there will not be one-size-fits-all approach and thinks there is a need for criteria to decide which approaches and tools should ultimately be used to make difficult decisions. Rasoulpour said that Dow AgroSciences is leveraging toxicogenomics and transcriptomics data on hundreds of molecules from in vitro and in vivo tests to have the most comprehensive reference dataset possible to assist in the evaluation of future chemicals. Kaminski added researchers should consider human cells and tissues, when possible, be used as an adjunct to animal testing, arguing that many of the assays typically conducted in vivo now can be done in vitro using human cells. He also pointed out the importance of acquiring new genomic information that may help explain the differences seen across species and provide important information about the mechanisms governing responses.
The regulatory requirement to recapitulate animal models in testing is holding back the field, Rasoulpour argued with agreement from some attendees. Rasoulpour suggested that if companies shared more about their internal processes, which are based mainly on predicting human outcomes, not animal models, it could help move the paradigm forward. For example, he said that the approach used at Dow AgroSciences to screen for impacts on the aromatase pathway, which is involved in a key step in the biosynthesis of estrogens, could easily be used for regulatory prioritization of untested chemicals or perhaps even in decision-making.
Weight of evidence, frameworks, systematic reviews, and key characteristics for carcinogens are all efforts to develop organizing principles that can allow people in the scientific community to put this evidence together in a systematic way so that those in the regulatory community and other areas can examine the evidence and use it in ways that are appropriate for their context, Aylward said. Barone agreed. Boekelheide raised the issue of publication bias preventing the dissemination of negative results that could shed further light on the number of studies and links (or no links) found when considering the scientific literature. Perry suggested that sharing more information about the knowledge gleaned from research “failures,” including models that do not work, could help the cause. She suggested that decision-makers and the public need to be more open to decision changes when newer evidence becomes available, in the event it supports the changes. In light of the current importance of expert judgment in making decisions about causality related to newer data streams and tools, Kevin Elliott of Michigan State University suggested that scientists should lay out the evidence as clearly as possible to aid regulators in interpreting it.
Terry thought that the use of negative controls should be employed more widely in observational studies to detect potential sources of error. She also commented on the value of using computational causal discovery as a way to identify variables that could be causal. “From a public health point of view, even when we don’t know everything in that black
box, we can still use some of the information for at least understanding who would have greater susceptibility,” she said.
Samet suggested there is a need to develop a research agenda to address the issues raised during the meeting, inspired by the Using 21st Century Science to Improve Risk-Related Evaluations report. He considered that such a research agenda should include the development of case studies that reflect various scenarios of decision-making and data availability, catalogue evidence evaluations and decisions that have been made on various agents so that expert judgments can be tracked and evaluated, and determine how statistically based tools for combining and integrating evidence, such as Bayesian approaches, can be used for incorporating 21st-century science into all elements of risk assessment.
DISCLAIMER: This Proceedings of a Workshop—in Brief was prepared by Kellyn Betts and Andrea Hodgson as a factual summary of what occurred at the workshop. The planning committee’s role was limited to planning the workshop. The statements made are those of the rapporteurs or individual meeting participants and do not necessarily represent the views of all meeting participants, the planning committee, the Standing Committee on Emerging Science for Environmental Health Decisions, or the National Academies of Sciences, Engineering, and Medicine. The Proceedings of a Workshop—in Brief was reviewed in draft form by George Daston, Procter & Gamble; Bill Farland, Colorado State University; Kristi Pullen Fedinick, Natural Resources Defense Council; and Ana Navas-Acien, Columbia University to ensure that it meets institutional standards for quality and objectivity. The review comments and draft manuscript remain confidential to protect the integrity of the process.
PLANNING COMMITTEE FOR ADVANCES IN CAUSAL UNDERSTANDING FOR HUMAN HEALTH RISK-BASED DECISION-MAKING: Kim Boekelheide, Brown University; Weihsueh Chiu, Texas A&M University; Kristi Pullen Fedinick, Natural Resource Defense Council; Gary Ginsberg, Connecticut Department of Public Health; Reza Rasoulpour, Dow AgroSciences.
SPONSOR: This workshop was supported by the National Institute of Environmental Health Sciences.
ABOUT THE STANDING COMMITTEE ON EMERGING SCIENCE FOR ENVIRONMENTAL HEALTH DECISIONS
The Standing Committee on Emerging Science for Environmental Health Decisions is sponsored by the National Institute of Environmental Health Sciences to examine, explore, and consider issues on the use of emerging science for environmental health decisions. The Standing Committee’s workshops provide a public venue for communication among government, industry, environmental groups, and the academic community about scientific advances in methods and approaches that can be used in the identification, quantification, and control of environmental impacts on human health. Presentations and proceedings such as this one are made broadly available, including at http://nas-sites.org/emergingscience.
Suggested citation: National Academies of Sciences, Engineering, and Medicine. 2018. Advances in Causal Understanding for Human Health Risk-Based Decision-Making: Proceedings of a Workshop—in Brief. Washington, DC: The National Academies Press. doi: http://doi.org/10.17226/25004.
Division on Earth and Life Studies
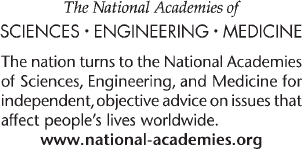
Copyright 2018 by the National Academy of Sciences. All rights reserved.