4
Evidence
As the use of genetic tests and genomic technologies increases in medical practice and through direct-to-consumer marketing, there is an increased need for evidence-based information to guide the use of such tests and the interpretation of their results (Botkin et al., 2010).
This chapter discusses the different types of evidence for genetic tests with regard to the concepts of analytic validity, clinical validity, and clinical utility. Although this report emphasizes clinical utility and is designed to address issues of patient management and clinical care, as noted in the statement of task, evidence of analytic validity and clinical validity remains important in test development and is an informative element of the evidence base. The chapter begins with a brief introduction to the types of evidence and their use in evaluating genetic tests. That is followed by discussion of the current state of the general evidence base on genetic tests. Finally, recommendations for advancing the evidence base are offered.
In many ways, the evidence base on genetic testing is not different from that for other areas of medical care: information is gathered and evaluated through research. The information that is gleaned through research varies in its quantity and quality, which confers some level of certainty about conclusions based on that information. Evidence is generally organized, summarized, and synthesized through systematic reviews or through meta-analyses. Evidence summaries are then used to inform clinical-practice guidelines and policy decisions.
There are ways, however, in which the evidence base on genetic testing is relatively unique, including differences in study designs and often a lack of direct evidence, for example, from randomized controlled trials (RCTs). The rarity of many genetic diseases and variants associated with genetic diseases pose a major challenge to researchers. The very nature of a rare disease makes it difficult to gather enough patients with the disease or variant to conduct a traditional study; thus, the usual sources of evidence are often not available. One way in which the field has responded to that challenge is the establishment of databases that aid in the collection and accumulation of evidence on clinical validity and utility; another potential approach is to provide guidance through decision modeling, for example, ClinGen and ClinVar, and the Genetic Testing Registry (Rubinstein et al., 2013; Rehm et al., 2015; Landrum et al., 2016).
Genomic sequencing presents another type of challenge because of the large number of variants that can be identified. The committee agreed that the evidence for any genomic test (including sequencing) is only as good as the evidence on the genes or variants being
investigated with regard to clinical validity and utility. Because genomic tests return results for many genes, of which only certain genes are pertinent to a specific patient, the level of evidence available for genomic tests varies by patient and clinical scenario (see Chapter 2).
The evidence base on genetic tests is described in this chapter as it applies to the domains of analytic validity, clinical validity, and clinical utility. The sources of evidence (the types of study design) that apply to each domain are described. The sources and quantity of evidence that are relevant and useful might vary by the characteristics of the test, the clinical scenario, and the prevalence, severity, and nature of the disease. For example, observational study designs, such as cohort studies, are appropriate for assessing clinical validity because they can accurately measure the ability to predict or detect disease. In contrast, evidence on the clinical utility of a genetic test is ideally generated by controlled studies, especially RCTs, because their design maximizes internal validity and addresses issues of selection bias and confounding. Although an RCT is ideal for assessing many aspects of clinical utility, the level of evidence sufficient to evaluate clinical utility might depend on the specific clinical indication, the clinical setting, and the perceived value of potential outcomes of the use of the genetic test itself.
Clinical-study designs can generally be organized in a hierarchic fashion on the basis of their inherent value in providing various types of evidence. Study types are often ranked according to their ability to detect true associations (internal validity) in the population of interest (external validity). Internal validity generally refers to the manner in which a study was conducted to minimize risk of bias1 A study with high internal validity has few flaws in its design, conduct, or reporting, so a similar result is likely if the study is repeated in the same way on another sample from the same population. External validity refers to the ability of a study to generate information that is applicable or generalizable to a population of interest (IOM, 2011a). Careful assessment of each piece of evidence is needed in the context of the clinical scenario. Well conducted studies that are lower in the evidence hierarchy might be more valuable than poorly conducted studies that are higher in the hierarchy for the type of evidence sought. Studies with high internal validity might have limited external validity. In some circumstances, lower-level evidence might show larger effects, such as a case study that demonstrates a dramatic change as a result of treatment according to the findings of a genetic test compared with an observational study that has a large effect size and great statistical significance, which might confer greater confidence that the association is valid. Additionally, some study designs might not be feasible for all clinical scenarios.
Evidence that is publicly available and peer reviewed is typically given greater weight than evidence that is not. It is in the nature of publication and peer review that studies must meet reporting criteria set by scientific journals and be objectively and independently evaluated.
ANALYTIC VALIDITY
Analytic validity refers to the accuracy and consistency of the technology used in detecting the true status of genetic variation, that is, is the variant actually present (or is the variant accurately called?). Evidence of analytic validity is generally reflected by such measures as test precision, reliability, accuracy, sensitivity, and specificity. Measures that compare a
___________________
1 Types of bias include allocation or selection bias, attrition bias, performance bias, detection bias, and reporting bias. For more information, see Finding What Works in Health Care: Standards for Systematic Reviews (IOM, 2011a).
genetic test of interest with other relevant tests that are considered the standard of care or a “gold standard” are valuable (Morrison and Boudreau, 2012).
The ability to assess evidence on analytic validity is generally limited in three ways. First, validating genetic tests is often challenging because of a lack of appropriately qualified samples, a lack of gold-standard reference methods, and the constantly emerging and evolving genetic techniques. Thus, data on analytic validity of genetic tests might be lacking, inconsistent, or out of date. The relative newness of the technologies being used and the different technologies used in different laboratories and settings make implementing a gold standard for comparison difficult, although some efforts, such as those conducted by the US Food and Drug Administration (FDA) and Genome in a Bottle (organized by the National Institute of Standards and Technology), are attempting to advance this process (FDA, 2016d; NIST, 2016). Many next-generation sequencing (NGS) laboratories use quality-control measures that are intended to specify the reliability of a genotyping result, but the reliability of the quality-control measures also requires assessment (Gargis et al., 2012; Rehm et al., 2013).
Second, the ability to obtain unbiased, detailed data on the analytic validity of tests that already exist can be challenging. Information about the analytic validity of laboratory-developed tests obtained from manufacturers varies in availability, detail, and credibility. Evidence of analytic validity is often found in the grey literature (such as technical reports) or in FDA documentation of FDA-cleared or approved tests (Jonas et al., 2012; EGAPP, 2014a). In addition to systematic searches of published literature, it might be important to collect information from other sources, such as laboratories and manufacturers, conference proceedings, FDA summaries, materials submitted by manufacturers, the Centers for Disease Control and Prevention’s (CDC’s) Newborn Screening Quality Assurance Program, the CDC Genetic Testing Reference Materials Coordination Program, proficiency-testing programs such as that of the College of America Pathologists (CAP), interlaboratory sample-exchange programs such as those conducted by the Association for Molecular Pathology and CAP, and international programs (Sun et al., 2011). In some cases, evidence pertaining to analytic validity is unavailable because of its proprietary nature. Thus, traditional methods of evidence review are not easily used (EGAPP, 2014a).
Third, traditional approaches to analyzing the data, once obtained, might be inappropriate given the variability in testing parameters and the types of data considered (Sun et al., 2011). Genetic tests present a number of technical issues that are relevant to assessment of their analytic validity. Technical issues might differ with the type of genetic test and might influence the interpretation of a test result. Those issues include collection, preservation, and storage of samples before analysis; type of assay used and its reliability; types of samples being tested; type of analyte investigated (e.g., single-nucleotide polymorphisms, alleles, genes, or biochemicals), genotyping methods; timing of sample analysis; interpretation of test result; and variability among laboratories or their staff members and their quality-control processes. For a given technology, variability within a run, between runs, within a day, between days, within a laboratory, and among operators, instruments, reagent lots, and laboratories can affect assay reliability and robustness. Thus, pooling data to investigate a class of tests that use a specific technology might not be useful, and it might be necessary to evaluate each test individually (Sun et al., 2011). Technical issues might also differ depending on the specimen being tested. For example, although it is not a focus of this report, there are different considerations when assessing tumor genomes as opposed to germline genomes (Jonas et al., 2012).
Analytic-validity studies evaluate a broad array of testing-performance characteristics, such as analytic sensitivity or specificity, analytic accuracy, precision, reproducibility, and
robustness. Analytic-validity studies of laboratory testing are different in design from other types of studies, such as diagnostic-accuracy studies and studies to evaluate therapeutic interventions, and require different criteria for quality assessment. As recommended by the Agency for Healthcare Research and Quality (AHRQ), an ideal set of quality rating criteria includes not only measures of the internal validity of a study but measures of external validity and reporting quality, and it should be flexible and customizable so that the criteria used are based on the issues being evaluated and the needs of the stakeholders (Sun et al., 2011). Compounding those challenges is the possibility that conclusions will be drawn regarding the analytic validity of the underlying technology or testing platform, such as NGS, or the analytic validity of a single test or testing panel.
Prioritizing Evidence on Analytic Validity
Given the variety of issues that are important in assessing analytic validity of different tests, rapidly advancing technology, and differences between in vitro diagnostic tests, laboratory-developed tests, companion diagnostic tests, and NGS, it is challenging to create a strength-of-evidence hierarchy that is similar to that used for clinical utility or efficacy. However, there are clear characteristics associated with greater confidence or certainty that a test has sufficient analytic validity, such as validation of a large number of well-characterized samples across different laboratory sites. Well-designed and well-applied external proficiency testing schemes and interlaboratory comparison programs, such as those supported by CAP (2016), also provide an increased level of confidence, and peer-reviewed and published data add certainty.
Evidence on analytic validity is available in FDA summaries for genetic tests that function as companion diagnostics for pharmaceuticals (FDA, 2016b) and for genetic tests used as in vitro diagnostic devices (FDA, 2016c), which are reviewed by expert panels. However, most genetic tests are marketed as laboratory-developed tests, which are not reviewed by FDA but might be addressed by application of CAP guidelines.
Finally, the analytic validity of a technology that has been well documented for detecting a number of analytes (such as genetic variants) is often assumed to have certainty similar to that of analytic validity for a new analyte. For genomic sequencing, however, that might not be true inasmuch as some parts of the genome are particularly difficult to sequence (Marx, 2013).
In light of those caveats, higher confidence in the evidence of analytic validity of a genetic test is based on the following:
- number of sites participating in validation
- number of samples included in validation
- application of established proficiency testing and interlaboratory comparisons
- peer-review publication of results
- expert-panel review of regulatory (FDA) information
- underlying testing technology that has well-established analytic validity
Analytic validity is an integral element of clinical validity such that some approaches to evaluation of evidence on genetic tests assume that analytic validity has been demonstrated if clinical validity has been shown; that is, observation of a gene–disease association depends on the ability to classify people correctly as to genetic status (Teutsch et al., 2009; CDC, 2013). However, that assumption is less convincing if a test is conducted by a single laboratory, in a few runs, by the same operator, using one or a few instruments.
CLINICAL VALIDITY
The clinical validity of a genetic test refers to the test’s ability to define or predict the disorder or phenotype of interest accurately and reliably, so it is necessary for there to be an association between the gene or variant and the phenotype. To describe this aspect of clinical validity, the committee outlines several pathways used to identify the genes and variants that cause disease; discusses how evidence of penetrance, pathogenicity, and functional characterization affect the ability to draw causal inferences; and describes how that evidence is collected in databases. The types of studies used to generate evidence of the clinical validity of a genetic test are also described.
When evaluating the clinical validity of a test, the clinical scenario is an important consideration. For example, the validity of a given test can depend on whether it is used in a diagnostic versus a predictive setting. Issues of incomplete penetrance and variable expressivity are particularly important in predictive scenarios compared with diagnostic scenarios, in which a person’s symptoms are associated with a higher prior probability.2
Evidence on Genes and Variants Associated with Disease
It is important to recognize the difference in clinical validity between a test based on a gene or any of its variants that have been clearly associated with a disease or phenotype of interest and a test based on specific variants in that gene, some of which might never or rarely have been seen before. This is especially important in discussions of clinical validity of NGS and other methods that sequence large sections of the genome.
Identifying Disease Genes and Variants
Historically, the identification of disease genes and variants was generated by two related methods: the candidate-gene approach and positional cloning. The candidate-gene approach requires that researchers have some prior understanding of the disease pathophysiology and a plausibly relevant gene to study, but looks for associations between the gene and the disease in small families and case-control studies (Kwon and Goate, 2000). Positional cloning, on the other hand, is useful when no specific gene has been implicated; it includes linkage analysis (the co-segregation of genetic variants with disease in extended families) and linkage-disequilibrium analysis (the latter when many affected people in the population have inherited the identical disease mutation from a common founder) (Puliti et al., 2007). With the decreased cost of sequencing, disease genes are now more commonly identified by sequencing of families or of cases and controls to identify pathogenic variants. Even when the statistical evidence for a gene or variant is very strong, supporting biologic evidence (e.g., the observation of a similar phenotype when the same variant is put into a model system, whether a human cellular model or an animal model) is generally required to provide convincing causal evidence (Marian, 2014).
A variant associated with a disease implicates not only itself in disease pathogenesis but the gene in which it lies. Identification of other, distinct mutations in the same gene in patients who have the same phenotype, also with supporting biologic evidence, greatly strengthens the
___________________
2 The prior probability of an event is the probability of the event that is computed before the collection of new data.
causal inference. Mutations that disrupt the normal protein sequence by causing an early termination by a stop codon or frameshift are considered more likely to be causal.
Whole exome sequencing and whole genome sequencing have identified many new potential gene–disease relationships that are based on the association of rare, de novo, or inherited variants in the same gene that are predicted to be pathogenic in patients with the same phenotype from independent families. Probabilities of identifying independent de novo mutations can be calculated on the basis of the background mutation frequency and the intolerance of the gene and protein domain to genetic variation. Further support of the association of the gene with the disease is provided when the gene of interest is functionally disrupted in animal models and the disruption results in a phenotype comparable with that found in humans.
Gene Panels Versus Whole Exome or Whole Genome Tests
Some genetic tests involve gene or variant panels that are restricted to one or a few genes and a specified list of well-characterized variants (new or poorly documented variants are not included). For example, FDA approved cystic fibrosis testing by NGS for a specific panel of variants in the CFTR gene because there was good evidence of the analytic validity and clinical validity for the variants (based on statistical evidence on the frequency of the variants in patients versus controls and functional predictions).
In contrast, whole-genome or whole-exome tests, such as those performed with NGS, present a situation in which many genes, perhaps the entire genome, are examined simultaneously. When they are used in a diagnostic or prognostic setting, the same rules apply as those described above in connection with causal evidence on a particular gene and particular mutations for the disease or phenotype under consideration. Because of a variety of additional questions, such as those involving penetrance, the use of such expansive testing for making disease predictions requires additional evidence, which is often lacking.
Monogenic Versus Polygenic Disease
The report is focused on Mendelian (monogenic) diseases or traits, which are characterized by single genes that have large effects. However, over the last decade, many genome-wide association studies of common diseases and traits have yielded large numbers of genes and common variants whose modest effects underlie disease susceptibility. Genetic risk scores can be calculated for these common, single-nucleotide polymorphisms (SNPs), as well as for a number of available and marketed panels that combine tests for several SNPs. However, the increased risk associated with any one SNP is small, such that even the combination of several SNPs in a panel cannot provide sufficient predictive ability (Khoury et al., 2013). For example, the Evaluation of Genomic Applications in Practice and Prevention (EGAPP) Working Group confirmed this with two separate genetic risk profile evidence reviews and recommendations, finding insufficient evidence to support cardiovascular disease risk profiling or type 2 diabetes risk profiling (EGAPP, 2010, 2013b). However, more recent studies have shown that genetic risk scores for coronary artery disease are associated with greater cardiac risk that can be mitigated by healthy lifestyle (Khera et al., 2016), and polygenic risk scores have been associated with risk perception, medical decision making, and improved lifestyle choices (Krieger et al., 2016).
With additional research and discovery of more related SNPS, polygenic risk assessment might provide clinical value in the future. For example, a recent study suggested that genetic-based risk models might provide predictive value in minimizing radiotherapy for oncology
patients (Kerns et al., 2015). However, at the current time, given the lack of evidence that population-level polygenic risk profile strategies have sufficient predictive value or demonstrated clinical utility, they are not discussed further here.
Inferring of Causality for Genes and Variants
With NGS analysis, many novel variants are seen, and establishing causality becomes a critical issue as inferences regarding novel variants are often drawn on the basis of analogy with well-documented causal variants. It is important to note the distinction between evidence supporting an association between a gene and a disease and evidence supporting an association between a specific genetic variant and a disease. The standard for implicating a gene in a disease is the unequivocal association and causal interpretation of one or more variants in the gene. In other words, it is well established that some mutations in a gene can lead to disease but that not every variant in a gene will lead to disease. Evidence on individual variants comes either from direct empirical clinical studies or, more weakly, from informatics predictions, especially by analogy with known disease-causing variants. Mode and pattern of inheritance and allele frequency are also sometimes invoked to support an association. For example, segregation of a novel variant in a family that has multiple affected members (usually in the case of a dominant disorder) can provide such evidence (akin to linkage analysis). It is more typical that a detected novel variant is de novo, that is, without documented inheritance. In such a case, it is presumed that DNA variants that lead to severe diseases that impair reproduction are not transmitted and thus appear as de novo mutations not carried by parents. That depends on an inherent assumption that the penetrance of such mutations is high.
Pragmatically, the clinical validity of a genetic test has to depend on evidence of the association of the gene—rather than of all the variants found in the gene—with the disease of interest. Otherwise, nearly all genes would be excluded through usual methods of determining causality. A test that is valid might be characterized by a great deal of uncertainty and have diminished clinical utility because of uncertain pathogenicity of many observed variants. FDA notes in its draft guidance on NGS-based testing that processes for evaluating the clinical validity of variants, and not just genes, are critical to the validity of a test (FDA, 2016b). As described below, it is a smaller problem for diagnostic and prognostic tests and a greater problem for predictive tests.
Effects of Penetrance and Expressivity
The concepts of penetrance and expressivity are extremely important in clinical genetics. Penetrance refers to the fact that people who carry a genetic variant or variants associated with a disease might never manifest the observable features of the disease (Cooper et al., 2013). The norm is that a gene might have numerous variants; some of them might always be associated with the disease (complete penetrance). For example, Huntington’s disease is an autosomal dominant disease with complete penetrance. The manifestation of the disease and the age of onset vary greatly depending on genetic factors (the number of CAG trinucleotide repeats) and other environmental factors (Wexler et al., 2004; Gayán et al., 2008; Kay et al., 2016). But other variants might not always be associated with disease (reduced penetrance). A weaker association between variant and disease will be observed in the case of variants that have reduced penetrance (Geneletti et al., 2011).
The symptoms or disease manifestations in people who carry predisposing genotypes can also vary widely; this is known as expressivity. For example, Gaucher disease results from
reduced activity or absence of the enzyme glucocerebrosidase. Genotypes that include mutations that lead to complete elimination of enzyme activity are associated with an early-onset, severe form of the disease. Genotypes that lead to a partial function of the enzyme generally have a later onset and a milder form of the disease. People who have two mutations associated with decreased enzymatic activity might have a very mild form of Gaucher disease with late onset or even no symptoms. Different people who have Gaucher disease might have different manifestations of the disease—so-called variable expressivity. Some will have bone pain, and others will have a severe neurodevelopmental disorder (Pastores and Hughes, 2000). Incomplete penetrance and variable expressivity are hallmarks of many genetic diseases that affect the ability to observe associations and the ability to draw causal inferences accurately. The penetrance of most variant–disease associations has not been well documented and can lead to false positives (MacArthur et al., 2014).
Understanding of the penetrance of a particular genetic variant is critical for predictive testing of unaffected people (especially population-based testing of those who are not known to be at increased risk). It is less important in diagnostic or prognostic genetic testing when a person already has symptoms and a test is used to establish or confirm a diagnosis. For predictive testing, a test might have clinical validity if the gene and individual variants are well established, but there might still be a great deal of uncertainty if the penetrance of the different clinical manifestations is not well described in the target population.
For many genetic conditions, estimates of penetrance might not be available. The best study design for estimating age-related penetrance is a longitudinal cohort study, preferably prospective. Case-control designs can also be used, but they require more attention to potential confounders (in this case, mostly genetic ancestry). For many Mendelian conditions, studying penetrance is difficult because the rarity of such mutations limits the size and power of all study designs (MacArthur et al., 2014).
For rare variants, prospective or retrospective study of relatives, in whom the frequency of a specific mutation is enriched, can be useful; however, sample sizes are necessarily limited by variant prevalence. Much better data generally are available on founder mutations, for which more mutation carriers are available. For example, three breast and ovarian cancer variants, BRCA1 185delAG and 5382insC and BRCA2 6174delT variants, are present collectively in about 2% of Ashkenazi Jews (Moslehi et al., 2000). Those mutations have been studied by case–cohort analysis and in extended pedigrees, which have yielded somewhat different estimates of penetrance (Chen and Parmigiani, 2007). Nonetheless, this example is the exception rather than the rule. Empirically derived risk estimates for individual mutations are generally lacking, and assumptions about penetrance are often based on analogy; for example, combining all truncating alleles because they would be predicted to lead to early protein termination and loss of protein function.
Pathogenicity
Pathogenicity is often used in human genetics to refer to the notion that genetic variants can cause disease. But it is inherent in this notion that such variants can be highly penetrant or weakly penetrant and, especially in the latter case, influenced by environmental and other genetic factors. Weak penetrance is also often reflected in uncertainty regarding causal effects of genetic variants, especially when such inference is based not on empirical clinical information but on indirect information in the form of predictions from biologic information or informatics (such as the degree of evolutionary conservation of the DNA sequence) and possibly on analogy with
other well-documented variants. Predictions of pathogenicity based on such indirect information are imperfect, especially in the case of missense variants. Pathogenicity is used to define variants in a clinical setting, but it is a complex term because of the potential of reduced or variable penetrance. It might also be difficult to distinguish between low penetrance and lack of pathogenicity (Minikel at al., 2016). Direct evidence of pathogenicity of variants is necessary to maximize the ability to differentiate disease-causing variants from the many rare nonpathogenic variants that are present in all human genomes (MacArthur et al., 2014).
Functional Characterization
Finally, in the absence of empirical clinical data to support a mutation’s association with disease, biologic and informatics approaches are often used to predict the functional effects of a variant on gene products and the resulting effects at the cellular and clinical levels (pathogenesis). Those approaches are improving, but there are still important limitations.
Genetic Databases
A number of databases report genetic variants in genes for Mendelian diseases, such as the Human Gene Mutation Database3 and ClinVar.4 The databases are used extensively in practice to provide evidence on particular variants that are identified by a genetic test. Because they are critical for the application of NGS-based tests, FDA has issued draft guidance on the use of such databases for clinical validation (FDA, 2016a). However, the databases are incomplete and contain numerous errors (MacArthur et al., 2014). The ExAC database includes 60,000 exome sequences from people who were not selected for disease and has been used to show that some variants previously believed to be disease causing or pathogenic are benign or of low penetrance (Lek et al., 2016). Such initiatives as ClinGen have begun to address some of those issues through the curation and interpretation of evidence on reported variants (Rehm et al., 2015). Variant databases used for clinical practice are continually being improved and expanded. FDA has proposed the versioning of such databases to keep track of the changes and improvements (FDA, 2016a).
Even if a variant that appears in a database is pathogenic, the penetrance of the variant, particularly among individuals without a family history, is often unclear. Without data on penetrance, the databases are useful primarily for diagnostic and prognostic tests and far less clinically useful for predictive tests.
Increased submission of data on detected mutations and associated clinical information to such databases and the curation of data in the databases will help to improve the evidence base on the clinical validity of genomic tests (MacArthur et al., 2014; Brookes and Robinson, 2015). However, filling the evidence gap as to the clinical validity of predictive genomic tests is much more complicated, expensive, and time consuming, particularly because it requires a careful study design and accumulation of many subjects who share the same rare mutation (or genotype).
___________________
3 Available at: http://www.hgmd.cf.ac.uk/ac/index.php (accessed November 8, 2016).
4 Available at: https://www.ncbi.nlm.nih.gov/clinvar (accessed November 8, 2016).
Study Designs for Clinical Validity
The sources of evidence on the clinical validity of a test are generally observational studies in which investigators simply observe the course of events. Observational designs are useful in situations in which it is unethical, unfeasible, or inefficient to conduct an RCT, and they can be more helpful in collecting data on long-term outcomes (Goddard et al., 2012). Observational studies are subject to confounding and other biases, but their use in genetics is less susceptible to confounding because genetic variation is correlated with few other factors except those related to ancestry. Studies that provide evidence of clinical validity often report such measures as diagnostic sensitivity, diagnostic specificity, positive predictive value, negative predictive value, likelihood ratios, and comparisons with other tests or methods that are considered the “standard of care” (Morrison and Boudreau, 2012).
Cohort Studies
In cohort studies, groups that have particular characteristics or are receiving particular interventions (e.g., premenopausal women who are receiving chemotherapy for breast cancer) are monitored to observe an outcome of interest. The outcomes are then compared between groups on the basis of whether people had an exposure, disease, or intervention of interest. A cohort study might be conducted prospectively or retrospectively, for example, through the use of databases (IOM, 2011b). Cohort studies are retrospective if exposure information is known for a well-defined population that has already been followed for the outcome of interest. The major advantages of the cohort design are that the exposure is known in advance of the outcome and that patient selection by exposure level is not influenced by outcome status. Cohort studies are often the design of choice for rare exposures because selecting a sample on the basis of exposure offers the possibility of creating a study that has enough at-risk people. In the specific situation considered here, the “exposed” group is made up of people who carry the genotype of interest. Following such people longitudinally could provide risk estimates for disease or trait outcomes as a function of age and possibly other variables, including environmental ones. Thus, cohort studies are the design of choice for providing risk estimates that are necessary for predictive genetic testing. However, cohort studies can be expensive because they require continuous follow-up unless they can be constructed retrospectively. For rare genetic variants, constructing a cohort of family members is one approach to increase the number of people who have the genetic variant of interest but might be confounded by relatedness or similarity of environment.
Case-Control Studies
In case-control studies, groups made up of people who do and do not have an event, outcome, or disease are examined to see whether an exposure or characteristic is more prevalent in one group than in the other (IOM, 2011b). Case-control studies in the genetic context compare the odds of having a genetic variant, for example, among cases (those who have a health condition) and among controls (those who do not have the condition) from the same source population. In the case of genetic research, cases and controls should be matched on race or ancestry because genetic variants differ in frequency by ancestry within a population. If unmatched, allele-frequency differences between cases and controls can be related to ancestry rather than to the presence of the disease of interest in the cases and its absence in the controls. The case-control design is generally the most common design used to demonstrate an association between a genetic variant and a disease outcome. That is especially true for rare diseases.
Because case-control studies start with cases and controls, they cannot provide a direct estimate of disease risk for people who carry the relevant genetic variant, so they are most useful for clinical validation of diagnostic testing rather than for predictive testing. However, under the assumption that the disease of interest is rare, the odds ratio derived from a high-quality case-control study provides a good estimate of the relative risk of the disease in genetic-variant carriers versus noncarriers. If the overall lifetime risk of the disease is known, absolute risks can be estimated from the relative risks. That approach is often necessary because of the challenges and expense associated with mounting a well-designed cohort study.
Case Series and Case Reports
Case reports and case series (multiple cases described together) are retrospective descriptions of individual accounts that document a disease, an exposure, an intervention, or an aspect of care. They are not systematically collected, there is no comparison group, and there is no statistical analysis or hypothesis testing. The observations are useful for reporting possible new gene–disease associations, particularly for rare diseases observed through the use of whole-genome sequencing. With regard to genetic research, case reports often report family-based data, not only data on individual people (Samuels et al., 2008).
Prioritizing Evidence on Clinical Validity
For clinical validity, hierarchy levels are based on dimensions of replication, study design and quality, and biologic plausibility (see Figure 4-1). Evidence from multiple studies gives greater confidence and has higher priority than evidence from single studies. Study quality, as described earlier in this chapter, plays an important role in one’s confidence about a gene–disease association, so studies that have flaws of concern, such as lack of control for confounding or biases are lower in the hierarchy. Biologic plausibility represents the extent of understanding of the gene–disease relationship. Evidence of clinical validity is stronger when the molecular and biologic mechanisms leading to the disease are understood than when mechanisms are not understood.
Evidence on clinical validity in distinct groups or small datasets can provide proof of concept, but additional data representative of the entire range of the intended clinical population provide much stronger evidence on clinical validity (Teutsch et al., 2009).

CLINICAL UTILITY
The clinical utility of a genetic test refers to the ability of a genetic test result to improve measurable clinical outcomes and add value to patient-management decision making compared with current management in the absence of genetic testing (Teutsch et al., 2009). A screening or diagnostic genetic test does not itself have inherent clinical utility; rather, it is the intervention (therapeutic or preventive) that is guided by the test results that influences clinical outcomes and determines clinical utility (Grosse and Khoury, 2006).
The gold standard of clinical utility is the evaluation of results from prospective trials that have randomized subjects to genetic testing or no genetic testing in an effort to compare different genetically informed treatments with usual care or from genetically stratified trials that compare treatment outcomes between different genetic groups. Prospective trials to study genetic tests are not common, but there are a few examples, such as the use of warfarin-dosing algorithms informed by genetic testing for variants in the CYP2C9 and VKORC1 genes (Anderson et al., 2007, 2012; Burmester et al., 2011; Pirmohamed et al., 2013).
In some cases, prospective trials are not available, and clinical utility has been determined by using a “chain of indirect evidence5 consisting of assessments of studies showing alteration of patient management . . . and the influence these changes had on outcomes” (Dotson et al., 2016). For example, EGAPP examined genetic testing strategies for patients who had newly diagnosed colorectal cancer to identify cases of Lynch syndrome, thus determining clinical validity of the test. And EGAPP found “adequate evidence” to support increased testing in Lynch syndrome relatives, better adherence to recommended surveillance guidelines, and follow-up and effectiveness of routine colonoscopy for preventing colon cancer. Thus, EGAPP’s “chain of evidence” supported the use of genetic testing strategies to reduce mortality and morbidity in Lynch syndrome relatives, supporting the clinical utility of the test for the relatives (EGAPP, 2009a).
In other cases, the chain of evidence linking a genetic test and improved clinical outcomes for rare, highly penetrant, single-gene disorders is slight or nonexistent. In those cases, the clinical utility of a test might be demonstrated if there is sufficient evidence that etiologic diagnoses are “actionable”6 and result in clear benefit to patient outcomes or that testing ends the “diagnostic odyssey” (see Chapter 1). For some families, clinical utility can be demonstrated if a genetic test results in a diagnosis that enables at-risk family members to know whether they carry a causative mutation so that they might plan early interventions or make reproductive decisions. For society at large, the clinical utility of a genetic test can be demonstrated through the understanding of the etiology of a genetic disorder and early identification of people’s at-risk years, which might inform more efficient health-system use (ACMG Board of Directors, 2015). Those types of evidence can be generated by a variety of observational and experimental study designs.
Thus, evidence of clinical utility might include demonstrated benefits and harms associated with a test, improvements in patient care, effects on patient outcomes, and effects on
___________________
5 A chain of indirect evidence is used in situations where direct evidence showing improved clinical outcomes related to a genetic test is not available; however, evidence linking the test to an intermediary that is associated with improved clinical outcomes may suffice.
6 Medically actionable test results, such as incidental findings, provide information that influences patient care to avoid morbidity or mortality. The determination of what is actionable is generally based on expert consensus and can be subjective (Berg et al., 2013).
patient or family member decision making. Different stakeholders might not view all of those equally. Finally, although the analytic validity and clinical validity of many genetic tests have been established for many genetic tests, the clinical utility of many tests in many contexts remains uncertain (Teutsch et al., 2009; Feero et al., 2013).
Study Designs for Clinical Utility
The sources of evidence on clinical utility are dominated by experimental-study designs. In an experimental study, the investigators actively intervene to test a hypothesis, generally in the format of controlled trials, specifically RCTs. Controlled trials have several variations that are important contributors to the evidence base on genetic testing. Controlled trials are characterized by a group that receives the intervention of interest while one or more comparison groups receive an active comparator, a placebo, no intervention, or the standard of care, and the outcomes are compared (IOM, 2011a). In genetic testing, controlled trial designs consist of one group of patients that receives the genetic test and a control group that does not.
Randomized Controlled Trials
As in a controlled trial, groups of RCT participants are compared, but RCTs randomly allocate participants to the experimental group and the comparison group. RCTs and meta-analyses of RCTs are viewed as the ideal approach to evaluating efficacy because randomization and other procedures reduce the risk that confounding and bias will be introduced and because the results are less likely to be due to error. However, they are generally limited to small groups of patients selected using defined procedures that might not be representative of real-world patients or clinical care (Goddard et al., 2012). Prospective study design also limits the length of follow-up available, so long-term outcomes are not usually assessed (Goddard et al., 2012). RCTs are useful for investigating the effectiveness of one intervention over another (Sox and Goodman, 2012).
Pharmacogenomic Trials
Pharmacogenomic trials use a variety of study designs, both observational and experimental, including RCTs, to investigate whether a genetic test result might be associated with a better response to a drug. They include
- prospective stratified trials that first test and identify participants for the presence or absence of a genetic variant and then randomize within each group (genotype-positive or genotype-negative) to active versus placebo or compare one drug with another,
- prospective screened trials that randomize people to genotype-guided treatment or a nonguided treatment (such as usual care or a single standard drug treatment), and
- retrospective trials in which testing is conducted after a study has concluded and associations between drug response and genotype are evaluated for evidence of effect modification (clinical validity) (IOM, 2011b).
Of those methods, prospective screened trials are better suited to evaluate clinical utility in that they evaluate the effectiveness of genetic testing in guiding treatment choice. However, prospective stratified trials take advantage of knowledge about the members of a test population and allow enrichment of exceptionally rare genotype groups and balancing of treatment assignment. Retrospective trials are useful for hypothesis generation when candidate genetic
variants are unknown and for replication; they are practical in that they leverage extant data from multiple RCTs, which can be systematically reviewed and subjected to meta-analysis.
In a small number of diseases, treatment depends on a molecular diagnosis of a particular gene or variant causing the disease. One example is the companion diagnostic test of CFTR genetic testing for cystic fibrosis patients and the use of an FDA-approved medication, Ivacaftor, for the G551D variant (and nine additional variants). Genetic testing in that example confirms the clinical diagnosis that could have been made by a nongenetic means, such as sweat testing. However, genetic testing can specify the variant that is necessary for making treatment decisions that are demonstrated to be effective as evidenced by the data submitted for FDA approval of the medication (Accurso et al., 2010).
Pharmacogenomic tests (genetic tests used to guide drug therapies) are often investigated through industry-sponsored clinical trials that present some unique challenges in assessing evidence. Data from pharmacogenomic trials are often unavailable because companies do not publicly release data from trials on tests that are not in the precompetitive space, they neither publish nor share genotyped samples from investigator-initiated research, and they tend not to provide such data on request by reviewers. Systematic reviews and meta-analyses conducted without inclusion of all available results of RCTs are systematically biased, and this can lead to spurious or misleading effect-size estimates (Dwan et al., 2013; Mills et al., 2013; Ioannidis et al., 2014).
Pragmatic Clinical Trials
Pragmatic (or practical) clinical trials study the effectiveness (rather than the efficacy) of an intervention in clinical practice with the goal of showing improvements in simple outcomes, such as health outcomes, patient satisfaction, and costs (Tunis et al., 2003). Pragmatic clinical trials can be randomized or nonrandomized. The advantages of the pragmatic approach to clinical trials are that they are more reflective of patients and practice, they are more efficient and less burdensome, and their results are more likely to be generalizable. However, pragmatic clinical trials are experimentally less rigorous by design, usual care is not a stable comparator, and increased heterogeneity makes greater sample sizes necessary as compared with clinical trials (IOM, 2011b). For example, researchers investigated the impact of genotype and several antidepressants on remission of depression and drug side effects in patients under usual circumstances through a pragmatic trial (Schatzberg et al., 2015).
Prioritizing Evidence on Clinical Utility
The sources of evidence often used to show clinical utility can be generally organized into the hierarchy shown in Figure 4-2.
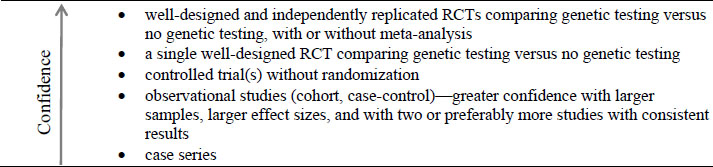
Studies that incorporate randomization are prioritized over those that do not, and studies that incorporate appropriate control groups are preferred to ones that do not. Emphasis is placed on quality and number of studies, and multiple RCTs have the strongest evidence. The evidence of clinical utility demonstrates that a test has a favorable effect on a patient, family, or community. In addition, studies designed to address the question of interest directly have greater weight than studies designed for other purposes but provide indirect evidence about the test (Teutsch et al., 2009). Although this is not reflected in Figure 4-2, the nature of a study to answer the question of interest directly is important. A study that was designed to answer the question directly, even if of lower quality, can provide better evidence on the clinical utility of a test than a higher-quality study that provides only indirect evidence.
Decisions about the clinical utility of a genetic test can be based on lower levels of evidence, as noted in Figure 4-2. Such decisions are inherently subject to a greater risk of bias and carry a greater degree of uncertainty (EGAPP, 2014a). The ideal approach to conclusions about clinical utility would be to base decisions about genetic tests on controlled trials, but in reality such decisions are routinely based on less rigorous levels of evidence. In practice, the sufficiency of evidence needed to support a decision about the clinical utility of a genetic test can depend on the clinical scenario; the potential net benefit of testing, including the outcomes considered; the test itself; the setting and values of the person or organization making the decision; and the consequences of the decision. Decision modeling is a useful tool for assessing those issues, as described below.
Decision Modeling
In many instances of evidence evaluation, the gaps in the evidence base make decisions about clinical recommendations and coverage difficult. Several national groups have developed methods that use decision modeling7 to supplement decision making in the setting of an evidence base that challenges traditional approaches to evidence-based recommendations. The US Preventive Services Task Force (USPSTF), for example, has formally recognized the potential of using decision modeling to address important issues that have not been or cannot be adequately answered by clinical trials. In 2016, the USPSTF published a methods paper that outlines how it uses decision modeling as a complement to systematic reviews to integrate evidence on different questions in its analytic framework, which allows it to
- quantify potential net benefit more precisely or specifically than systematic evidence review alone;
- assess the effect of data uncertainty on estimates of benefits, harms, and net benefits;
- compare alternative testing intervals, strategies, scenarios, and technologies (e.g., starting and stopping ages for testing);
- estimate outcomes beyond the time horizon available in outcomes studies;
- assess net benefit to clinically relevant groups (e.g., those at higher or lower risk for benefits or harms); and
___________________
7 A decision model is a “systematic, quantitative, and transparent approach to making decisions under uncertainty” through visualization of “the sequences of events that can occur following alternative decisions (or actions) in a logical framework, as well as the health outcomes associated with each possible pathway. Decision models can incorporate the probabilities of the underlying (true) states of nature in determining the distribution of possible outcomes associated with a particular decision” (Kuntz et al., 2013).
- assess net benefit stratified by sex, race or ethnicity, comorbid conditions, or other clinically important characteristics (Owens et al., 2016).
The EGAPP Working Group used a decision model informed by studies of different aspects of the Lynch syndrome clinical scenario mentioned above and concluded there was sufficient evidence to support a moderate population health benefit. They also used a cost–consequences economic model to compare different Lynch syndrome genetic testing strategies. No strategy emerged as superior, but the comparison of any testing strategy versus no testing supported the working group’s recommendation regarding the potential value of such testing in patients who are at increased risk (EGAPP, 2009a).
The Department of Health and Human Services Secretary’s Advisory Committee on Heritable Disorders of Newborns and Children (the Health Resources and Services Administration panel that determines the conditions on the Recommended Uniform Screening Panel for the United States) routinely considers evidence reviews on rare conditions that are being considered for inclusion on the screening panel. As in the case of rare conditions, such as those considered for genetic testing, the evidence base (especially from screening trials) is often critically small—the precise situation in which modeling can be particularly informative. In the latest update of its methods, the advisory committee stated that a decision model will supplement the evidence review for any new condition under assessment. The decision model will estimate the upper and lower bounds of predicted net benefit and harm for screening for the condition compared with usual care. The model will account for the prevalence of the condition, performance of the screening test, predicted outcomes of treatment (detected as a result of screening versus clinically observable disease) and harms of screening and treatment, and the inherent uncertainties of all data inputs. The advisory committee plans to make decisions in that way when a paucity of evidence challenges the traditional evidence approaches (Kemper et al., 2014).
Various cautions and caveats associated with decision models should be considered. Such studies can be complex, and transparency in data inputs, assumptions, model structure, validation, and analyses is essential. Results depend on the accuracy and quality of the input data and assumptions (Guzauskas et al., 2013). Results are always subject to some degree of uncertainty, so extensive, rigorous one-way, multiway, probabilistic, and other methods of sensitivity analysis are required and must be reported to inform interpretation of model results. Transparency can be achieved through peer review, detailed modeling reports, and access to the programmed models. Procedures should be in place to minimize potential conflicts of interest and other biases in model development and conduct of analyses.
Personal Utility
The committee noted that some experts have proposed a category of outcomes that they term personal utility, referring to outcomes that do not meet the traditional measures of clinical utility and might not have been examined adequately in research. Various organizations have evaluated genetic tests or developed clinical guidance for genetic tests—for example, EGAPP, the American Society of Clinical Oncology (ASCO), the National Society of Genetic Counselors (NSGC), AHRQ, and USPSTF—but they have not examined “personal utility” outcomes. Personal utility takes into account such issues as psychosocial effects, family planning, lifestyle changes, future decision making, and the value of information to the patient (Broadstock et al., 2000). It also includes the desire in patients or family members to decrease uncertainty about
health conditions and personal interest in having genetic information whose significance might not be known.
Outcomes identified in the category of personal utility share a degree of subjectivity that challenges researchers in devising appropriate measures and research designs. Some personal-utility outcomes—such as anxiety, quality of life, consequences for decision making, and costs—might be amenable to research that augments the evidence base on clinical utility. Other outcomes—such as satisfaction, well-being, and personal value placed on information that do not have a direct measurable effect and vary considerably from patient to patient—will require further development of reliable measures and methods to contribute to clinical utility, and to be amenable to systematic study (Stein and Riessman, 1980; Ende et al., 1989; McCullough et al., 1993; Hays et al., 1994; Grosse et al., 2008; Heshka et al., 2008; Foster et al., 2009).
Because the concept of personal utility is still in development and is not generally accepted as a separate and valid category of outcomes relevant to genetic tests, the committee did not reach conclusions about how best to address it. Some outcomes proposed in the category might be brought within the mainstream of research on clinical outcomes that patients would notice and value. Others will require further development of appropriate measures and research designs. Such organizations as the Patient-Centered Outcomes Research Institute (PCORI) are well positioned to assist in developing methods that will allow rigorous evaluation (PCORI, 2015; Stuckey et al., 2015). The Clinical Sequencing Exploratory Research Consortium has recently published its intentions to include aspects of personal utility in its collaborative research program (Green et al., 2016).
EVALUATION OF EVIDENCE ON GENETIC TESTS
Numerous organizations evaluate evidence on genetic tests. Many (such as EGAPP, ASCO, NSGC, and CAP) have drawn on widely used methods for systematic evidence reviews, such as those proposed by the Grading of Recommendations Assessment, Development and Evaluation (GRADE) working group, the Institute of Medicine, AHRQ, and USPSTF (Simon et al., 2009; Teutsch et al., 2009; AHRQ, 2012; ASCO, 2013a,b,; Caudle et al., 2014; CAP, 2015; NSGC, 2015). Several organizations that evaluate evidence on genetic tests and their approaches are summarized in Appendix C. However, traditional methods (systematic evidence reviews) are difficult to apply to genetic tests. In the United States, for example, EGAPP has developed a tailored approach to the unique aspects of genetic tests (Khoury et al., 2010). The EGAPP process provided a foundation for evidentiary standards to guide decision making in genetic testing and included a multidisciplinary, independent assessment of evidence with a focus on appropriate outcomes, methods for assessing individual study quality, methods for assessing the adequacy of the evidence for each component of the framework, and methods for assessing the overall body of evidence (Teutsch et al., 2009).
As of June 2016, only a handful of systematic reviews had been published, including 5 from the Cochrane Collaboration (Twisk et al., 2006; Hilgart et al., 2012; Alldred et al., 2015; Hussein et al., 2015; Walter et al., 2015), 1 USPSTF guideline (Moyer, 2014); and 10 EGAPP reviews (EGAPP, 2007, 2009a,b,c, 2010, 2011, 2013a,b, 2014b, 2015). Many stakeholder organizations rely on less systematic approaches and use expert consensus (Green et al., 2013; Ross et al., 2013) or other methods to conduct evidence reviews and make evidence-based decisions (such as the American Congress of Obstetricians and Gynecologists and the Association for Molecular Pathology).
Finally, regarding the evidence base on genetic tests, there are relatively few sources of synthesized evidence (systematic or not), compared with the large number of tests available. CDC lists 257 documents pertaining to genetic tests—a mixture of guidelines, commentaries and, reviews (CDC, 2016); the National Library of Medicine’s MedGen resource lists 428 international professional guidelines (NCBI, 2016). The National Institutes of Health’s (NIH’s) Genetic Testing Registry notes that there are 48,916 tests, 10,683 conditions, and 16,210 genes for which there are tests (Rubinstein et al., 2013; NCBI, 2017). GeneTests, another database of genetic tests, reports that there are 63,962 tests, 4,647 disorders, and 5,629 genes that are targeted by tests (Pagon, 2006; BioReference Laboratories Inc., 2016). The difference between the number of tests available and the number of available evidence reviews indicates that most tests have not been evaluated and demonstrates the need for additional systematic reviews.
RECOMMENDATION
There are challenges to evidence-based decision making regarding the use of genetic tests, including the paucity of the types of research studies on which evidence-based medical decisions depend, and they are exacerbated by the accelerated pace of development of new tests, discovery of variants, and technologic discoveries. RCTs to establish clinical utility are expensive and take a long time, so decisions are often based on lower levels of evidence. When addressing those challenges, evidence requirements should be risk based and should depend on the specific test and clinical scenario being looked at; and priorities for evidence generation should be based on feasibility and the likelihood that the evidence generated will improve clinical practice and patient outcomes. In other words, the evidence base needs to be advanced by looking for reasonable opportunities to engage in evidence generation practically and thoughtfully. Identifying opportunities and gaps in evidence for specific tests in certain clinical scenarios will require conduct of systematic reviews. The Department of Defense (DoD) could take several actions to contribute to the generation of evidence in practical ways.
DoD could undertake or otherwise support prospective studies of clinical utility when promising tests are identified. Studies should include a broad array of potential outcomes that reflect the clinical utility of genetic testing, including ones that extend beyond clinical outcomes, such as well-being, quality of life, and impacts on family members. DoD could conduct prospective studies, such as RCTs, in its facilities or affiliated institutions or could sponsor outside research.
A second approach would be for DoD to implement processes for data collection and analysis of its own experience with genetic testing. Establishing an infrastructure for data collection will facilitate tracking and monitoring of diagnostic outcomes and provide an opportunity to refine established policies for testing on a continuing basis. That process might include evaluating test use through claims or clinical databases, assessing test findings through collaboration with testing laboratories and providers, identifying clinical-practice changes related to use of test information, and tracking likely effects of test-informed treatment changes on patient outcomes. Through TRICARE, DoD has access to information on medical care and outcomes in a large population of active-duty members, retirees, and their family members. That information could be helpful in answering questions about clinical utility that many researchers and organizations struggle to assess. Access to long-term follow-up data on a particular population and its experience with genetic tests could be a valuable resource for generating evidence on clinical utility.
In both approaches, DoD resources might be more useful if DoD partnered with other organizations (such as PCORI, AHRQ, and NIH) to facilitate studies that focus on clinical utility. Many initiatives that are under way are complementary to those efforts to provide evidence for clinical utility. For example, the NIH National Human Genome Research Institute has organized several research programs to sponsor the development of evidence on clinical utility, including the Clinical Sequencing Exploratory Research program and the Implementing Genomics in Practice consortium (NHGRI, 2016a, 2017).
To generate evidence of clinical validity of genomic sequencing, DoD could participate fully in evidence repositories (such as ClinVar) and work with partners to improve their clinical relevance and usefulness (see Manolio et al., 2013; Rehm et al., 2015; FDA, 2016a). Given the large population covered by DoD (through TRICARE) and DoD’s data systems, such participation has the opportunity to advance the evidence base on genomic testing substantially.
DoD can also contribute to the clinical validity of tests by supporting discovery efforts related to the evidence base on NGS, particularly regarding the association between variants and phenotypes. That requires many people to undergo testing; DoD is in a position to provide such data on its population.
Finally, as the DoD implements a framework to evaluate evidence and make decisions regarding genetic tests (such as the recommended framework in Chapter 5), the evidence gaps identified might be used to directly inform their research priorities.
The committee recommends that the Department of Defense advance the evidence base on genetic tests through independent and collaborative research, including
- supporting high-quality studies of clinical validity and clinical utility (including patient-centered outcomes) of promising tests;
- implementing processes for data collection and analysis of its own experience with genetic testing;
- supporting evidence-based systematic reviews of genetic tests;
- contributing genetic variants and associated clinical data to public evidence repositories; and
- partnering with other organizations to facilitate these recommendations.