5
Lifetime Data Costs
To open the second day of the workshop, Alexa McCray, Harvard Medical School, summarized important messages from the first day of the workshop. She said that although data are not created equal, they improve when integrated with other data. Thus, it is essential that the most useful data are preserved. As a resource builds and obtains more data, scientists reclaim value, she continued. Questions remain about how to fund data sharing and preservation—if researchers can demonstrate that science advances as a direct result of sharing data via particular methods and platforms, an increase in long-term funding from the federal government and foundations could be justified.
PANEL DISCUSSION: INCENTIVES, MECHANISMS, AND PRACTICES FOR IMPROVED AWARENESS OF COST CONSEQUENCES IN DATA DECISIONS
Lars Vilhuber, Cornell University, Moderator
John Chodacki, University of California Curation Center, California Digital Library
Melissa Cragin, San Diego Supercomputer Center
Wendy Nilsen, National Science Foundation
Lucy Ofiesh, Center for Open Science
Panel moderator Lars Vilhuber, Cornell University, noted that in July 2019, the American Economic Association updated its data and code
availability policy: Data will now be treated as primary objects, and authors will be required to submit to prepublication verification. However, publication is at the tail end of a research project, and researchers should think about the entire life cycle of research, starting from the conception of an idea to the final publication, he said, as well as the data reuse that might occur afterward.
John Chodacki, University of California Curation Center (UC3), California Digital Library (CDL), explained that UC3 focuses specifically on research data management, digital preservation, and persistent identifiers—part of a larger suite of services that CDL offers across the University of California system. Three years ago, CDL began to consider how to sustain the cost of preservation, what happens after successfully capturing research outputs, and ways in which campuses can get more involved. Although the campus community thinks carefully about how to set up computational environments and support computational research, his team recognized that the preservation policies, as well as plans to make data accessible and reusable over time, were merely ad hoc processes.
He described a pilot at CDL, with the entire University of California system, to address some of these data preservation issues. The pilot included stakeholders from across the campus communities, including the libraries, research entities, and information technology systems. Their goal was to make data more discoverable and more usable, while adhering to FAIR (findable, accessible, interoperable, reusable) principles, reducing hurdles for reuse and for advancement, and building capacity for researchers. The team agreed that storage costs needed to be addressed in this pilot—make upfront capital investments in storage, leverage storage across the system, and create a distributed storage network that is paid for through different budgets.
Chodacki said that while the pilot was ultimately unsuccessful, the process illuminated important lessons. Conversations with diverse stakeholders were crucial to convince three campuses to invest in purchasing or acquiring additional storage as well as to evaluate policies and procedures. One flaw in the pilot was its lack of researcher involvement; without an understanding of front-end processes, a pilot to improve back-end processes could not gain traction. Researchers need to be champions for these types of issues, but it was difficult to demonstrate the value of long-term preservation to researchers, he continued. The team also realized that pilots traditionally try to solve complex problems on a small scale; the pilot might have gained more traction had it been done on a larger scale. With further conversation about alternative approaches, UC3 connected with Dryad—a curated data repository that works across many fields and domains and accepts more than 300 GB per Digital Object Identifier—to discuss its potential support of institutional needs. A partnership with
Dryad emerged, and Dryad will now be offered to all University of California researchers at no cost.
CDL also works on issues related to machine-actionable data management plans (DMPs), which can be used to help model data preservation costs and understand those costs throughout the entire research life cycle. DMPTool,1 a platform with 43 templates for 17 U.S. funders as well as international funders, is used by more than 31,000 researchers at 237 universities across the world. The tool is publicly available, and more than 250 campuses have a custom DMPTool. Chodacki’s team has a grant from the National Science Foundation (NSF) to retrofit DMPTool and other DMP ecosystems to be more machine actionable. He explained that DMPs are active documents. To help with forecasting costs, DMPs need to expose structural information (including data volume) as a project progresses, make information available to the right parties, and be updateable by multiple parties in a decentralized fashion. Chodacki’s team is working with DataCite’s Event Data with Crossref as a means to use scholarly infrastructure to capture controlled information within a DMP and share it through an existing central hub. He emphasized that none of this work would be possible without collaboration: The team has been leveraging the Research Data Alliance2 to build common standards for the format underlying machine-actionable DMPs (see Figure 5.1).
Melissa Cragin, San Diego Supercomputer Center, discussed ways to facilitate access to and use of active data (i.e., data within the research life cycle). She commended the “action leaders” in the United States and Europe (e.g., libraries, research computing departments, campus information technology specialists, and other administrators) who are seeking structural and process changes to encourage the management and stewardship of data within constrained budgets. Other constraints related to research data management include the breadth of services available, the relationships between technology and infrastructure platforms, and the transparency of ownership and costs. She explained that many faculty are interested in making their data available, learning about costs and trade-offs, and planning accordingly. Sustainable models are needed, she continued, which also requires commitment from campus leaders.
Cragin contacted colleagues from campuses across the United States and asked the following questions:
- What is happening on campus in terms of interactions with faculty to help them understand costing?
___________________
1 For more information about the DMPTool, see Dmptool.org, accessed September 25, 2019.
2 For more information about the Research Data Alliance, see rd-alliance.org, accessed September 25, 2019.

- What processes are being used, and are there tools available?
- How are units collaborating, and is there infrastructure on campus?
- What are the costs? Where are they showing up? She described several examples into different service categories (see Table 5.1).
In the unfunded linked-facilitator model, the campus information technology center and the high-performance computing center provide facilitator services to faculty for storage and compute. This includes free, distributed, and manageable storage services at a low level of size. If additional storage and compute are needed, consultations are arranged and fee-based solutions are offered to the researchers. The research unit fee-for-service model has three sustainable and flexible funding options, depending on the needs of the researcher: (1) a researcher uses a grant to pay the fees for storage, compute, and analytics and has complete access until the end of the award period; (2) a researcher pays a fee and his or her department supplements the fee, so that others in the department share the service and the researcher has access beyond the life of a grant; and (3) the campus pays 50 percent of the services, and faculty across campus can buy in. This model offers ways to distribute the costs differently, and can reduce costs across the research process, as researchers participate in evaluating trade-offs for services in out years. In the all-campus coordination model, there is a campus-wide committee: Policies, costs, and service boundaries are all shared, and transparency is paramount. The institutional commitment model requires a significant administrative investment into the library to provide research data management and a data repository. In one example, any student, staff, or faculty member has access to a private 100 GB of storage for 3 years and can publish up to 1 GB of data at no cost—these data will be available for a minimum of 10 years.
TABLE 5.1 Cooperative Approaches to Research Data Services
Linked facilitator model | Research unit model | All-campus coordination model | Institutional commitment model | |
---|---|---|---|---|
Motivation | Continuum of service | Sustainable, flexible shared cost models | Reduce stress and improve trust across units | Data life cycle as a driver |
Service | Campus IT and local HPC Center provide information, “hand-off,” and consulting |
Storage and compute
|
|
|
Benefit |
|
|
Cross-campus representatives to identify best storage solutions |
|
Funding | Unfunded | Fee-for-service | Unit staff time | Administration; external project funding |
NOTE: IT, information technology; HPC, high-performance computing.
SOURCE: Adapted from Melissa Cragin, San Diego Supercomputer Center, presentation to the workshop, July 12, 2019.
Cragin commented that Cornell University’s library has developed an open-source web-based tool, Data Storage Finder, which can be customized for individual campuses. Faculty can use this tool to understand the storage services available on their respective campuses and to make better decisions. She explained that postsecondary institutions are beginning to recognize data as an asset: Campus-based cooperative arrangements are on the upswing; there is a trend toward professionalization of research
staff roles; and an increased number of people and projects are being supported while managing costs. Persistent challenges include the variation of the kinds and extent of services across postsecondary institutions, hidden costs for data services across lifecycle and service groups, differences in procurement processes for multi-institution infrastructure projects that increase costs and necessitate much higher management overhead, and a lack of published empirical data on emergent trends and models.
Wendy Nilsen, NSF, explained that data are continuous, messy, and heterogeneous. Data are collected for long periods of time in large capacities, and biomedical data in particular can be combined to reveal important information about people. She described the success of the NSF-funded Asterisk database, which brings together data from diverse sources. Asterisk is now available on Apache and is being used by industry and researchers alike.
With the explosion of data, Nilsen noted the value of posing questions to data scientists and informaticians about what kind of data they need in order to move forward. She described a recent infrastructure initiative from NSF’s Computer and Information Science and Engineering directorate to better understand the needs of the research community, including data that are usable, accessible, inexpensive, and machine-readable.
Nilsen’s team considers how to develop infrastructure to collect and preserve relevant data. Questions to determine the relevance of data relate to quantity, reproducibility, cost (e.g., rare group samples are expensive), existence, and completeness. Her team evaluates analytics, crowdsourced value, diverse community governance, repetition, and feedback from users to determine the usefulness of data. She mentioned that 20 percent of NSF awards are now dedicated to outreach so that the researchers have the opportunity to get user feedback on their data. Expertise is also needed in computing and information science to reduce barriers to data access, maintain safety, increase data quality (e.g., metadata, validity, reproducibility), decrease costs (both time and money), and build sustainable models, she continued.
Lucy Ofiesh, Center for Open Science (COS), described the mission of COS as to increase openness, integrity, and reproducibility of research through three interconnected functions: infrastructure, metascience, and community (see Figure 5.2).
COS’s metascience team studies the reproducibility of research, evaluates interventions, and works on large-scale reproduction projects. The technology team builds infrastructure, including several web-based solutions, that enables researchers to enact reproducible behaviors across the research life cycle. For example, OSF.io is a project management platform that has been implemented at 60 universities and research institutions across the world. Five million files were downloaded from OSF.io in 2018;
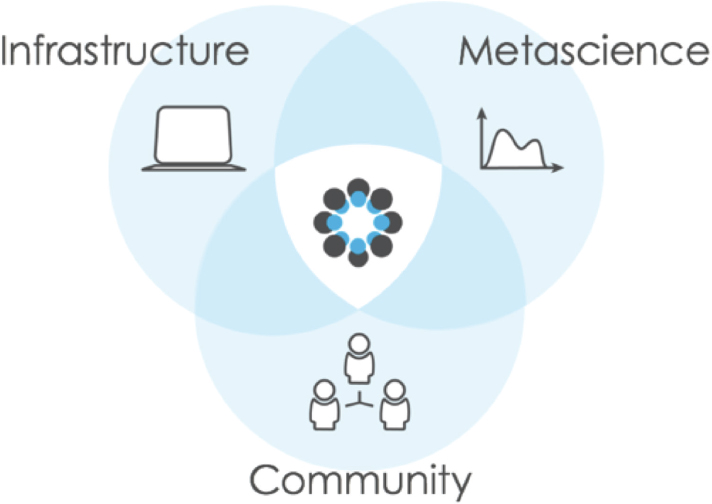
in 2019, that number climbed to nearly 12 million. She noted that OSF.io study registrations will likely be close to 40,000 by the close of 2019. People also use OSF.io to discover and repurpose files, papers, and data sets.
The community and policy team engages with existing research communities (and fosters new ones) to identify pain points in the research process and to develop best practices. The community and policy team is guided by eight transparency and openness guidelines:
- Data citation,
- Design transparency,
- Research materials transparency,
- Data transparency,
- Analytic methods transparency,
- Preregistration of studies,
- Preregistration of analysis plans, and
- Replication.
Data are multiplicatively more effective with open code, open materials, and preregistration of studies, Ofiesh explained. COS strives to
motivate stakeholders, including publishers and funders, to recommend, require, and/or enforce sharing policies, which, with the help of technology solutions, will drive behavior change among members of the research community (see Figure 5.3).
Ofiesh emphasized that the first step to changing behavior is to understand the unique needs of each research community and develop relevant incentives. COS lends visibility to desired actions, thus promoting the adoption of ethical behavior. It is also exploring ways for researchers to earn credit, be acknowledged for their practice, and be viewed as credible (e.g., via the badging program for researchers who exhibit a best practice such as open data, open materials, or preregistration), as well as ways to support the use of open repositories and open registries. She noted that the proportion of preregistered open materials recently increased from 3 percent to 40 percent for the 60 journals that have adopted the badging program.
COS is also piloting programs related to registered reports, in which the researcher, journal, and funder are working together at the study design phase as well as engaging in peer review after both the study design phase and the report writing phase. This cooperative approach privileges rigorous scientific research and removes pressure for researchers to produce results that are “publishable.” It also ensures that funders work in partnership with both the researcher and the journal throughout the research life cycle. Two hundred journals currently accept registered reports, Ofiesh said.
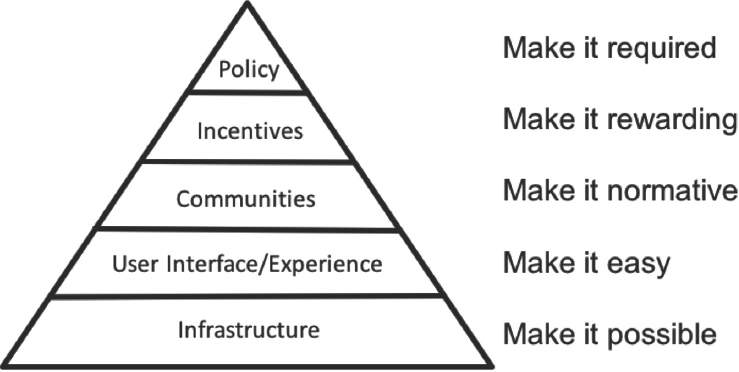
COS’s long-term objectives are to (1) enable best practices with tools, communities, and policies; and (2) remain sustainable with technology solutions that ensure the security and duplicability of data. Ofiesh described a partnership between COS and Internet Archive to replicate all registrations on the COS website. This partnership provides an alternative to commercial infrastructure lock-in. COS strives to build products that meet researchers’ needs, as those needs and the surrounding landscape continue to evolve.
Vilhuber observed that all four panelists emphasized that researchers are motivated by straightforward and inexpensive processes. However, it is difficult for postsecondary institutions to sustain such processes. He asked how to determine, from the researcher’s perspective, which data are useful. Chodacki responded that this community’s desire to solve all of the problems at once creates a multifaceted challenge. Instead, the community should figure out how to build systems to capture information consistently before making value judgments about data, he asserted. Cragin said that it is possible to determine the usefulness of some data with a long-tail perspective (i.e., thinking about integrated and interoperable data sets); but, there remains a need for theoretically based frameworks for decision making. Conversation about the implementation of standards and collection procedures is one way to engage with communities, although sectors within communities could have varied opinions (e.g., social scientists do not all share the same view of secondary uses of data). She added that it is important to think more broadly about data quality (e.g., the use of data for the public good versus the use of data at the institutional level). Nilsen agreed and said that it is crucial to understand what data are available and how they can be used. She reiterated that communities should share their experiences and pain points as a first step in eliminating barriers to data sharing. Ofiesh added that the adoption of any best practice begins with awareness, includes training, and results in refinement. If data are not shared, she cautioned, communities might miss opportunities to use data from other communities.
Maryann Martone, University of California, San Diego, asked Cragin whether the level of information technology support provided to research laboratories drives data management. She also wondered if disciplines have varied levels of interest in and need for such support. Cragin noted that the level of service offered varies by domain; for example, at Oregon State University’s Center for Genome Research and Biocomputing, the high level of analytics support provided is attractive to and valuable for researchers. Chodacki echoed a comment from the first day of the workshop that research is inspired by creativity and curiosity. He noted an increased return on investment for the Carpentries and other training opportunities, which bring higher-level tools or skills into laboratories and feed this curiosity.
William Stead, Vanderbilt University Medical Center, asked about the cost to make Dryad freely available to all University of California researchers, and McCray wondered about the costs involved in COS’s partnership with Internet Archive. Chodacki said that Dryad has an institutional membership model, with small ($3,000 per year), medium, and large ($13,000 per year) tiers. Ofiesh explained that the Institute of Museum and Library Services provided a 2-year $250,000 grant to build a connection to the COS application programming interface (API). Since Internet Archive is the lead on this project, COS is working with Internet Archives’ API to push registries—a task that has the greatest cost. The cost to maintain the API is minimal; COS spends $200,000 a year to host and store data, but incremental costs over the long term will need to be supported.
In response to a question from Ilkay Altintas, University of California, San Diego, Cragin said that no good published research exists on the decision-making process to develop services and allocate resources across campus; most evidence is anecdotal. Altintas added that the definition of data is changing to include “services around data,” which could change the cost model. Cragin clarified that her definition of services includes cyber infrastructure and software services and tools, which can be expensive to maintain, scale, and make interoperable. Robert Williams, University of Tennessee Health Science Center, explained that Dropbox has enabled a successful multi-institutional collaborative framework for data sharing. He described COS as an intermediary between Dropbox and Github, the latter of which is too complicated for use in his field. Chodacki observed that definitions of preservation also vary, further complicating the notion of forecasting costs for preservation. Adam Ferguson, University of California, San Francisco, pointed out that it is difficult to enforce data preservation policies on faculty who have 5-year grant cycles and little career stability. Sarah Nusser, Iowa State University, said that postsecondary institutions should be supporting preservation efforts regardless of faculty interest. Chodacki added that discussing how to forecast data preservation costs from a funder perspective is challenging, especially for cases in which the funder cannot allocate money for data preservation.
SUMMARIES OF SMALL-GROUP DISCUSSIONS
Connecting the Dots: Planning Tools for Data Support and Research Computing
Altintas said that her group discussed methods to encourage National Institutes of Health (NIH)-funded researchers to consider, update, and track lifetime data costs, although the group debated whether “lifetime” is the best way to describe what happens to data. She noted that training
can be implemented to reduce costs and to motivate researchers. She suggested that training begin early (during scholarship) and be offered at the institutional level. She also proposed the adoption of a “train-the-trainers” model that follows existing practices, such as those used by the Carpentries. Another topic that emerged during the group’s discussion was the need to align individual and institutional practices with expectations from federal agencies to develop economies of scale. The group also discussed cost reduction incentives embedded in new initiatives, such as NIH’s Science and Technology Research Infrastructure for Discovery, Experimentation, and Sustainability.
Altintas explained that this community could learn lessons from other communities with decadal studies and best practices for planning. It is also imperative to define this community more precisely—who touches the data and when? She mentioned that existing methods to create reusable archives have not been standardized, and she noted the important difference (especially in terms of cost) between data preservation and data hoarding. One way to reduce the cost of data preservation is to make data inactive (i.e., suspended animation or “dehydrated” data). This requires little energy and ensures that the data have captured knowledge and are findable. An inactive archive could then be refreshed at a low cost to improve the value of the data and to prevent loss. Another way to reduce cost is to create an ecosystem of universities and national funding agencies that could distribute the responsibilities to support the operation of repositories, she continued. A related problem that needs to be addressed is that funding for data generation does not always align with funding for data acceptance; thus, repositories are responsible for finding creative ways to reduce the cost of their operations in order to accept more data.
Practices for Using Biomedical Data Knowledge Networks for Life-cycle Cost Forecasting and Updating
Clifford Lynch, Coalition for Networked Information, said that his group first tried to develop an understanding of the phrase “biomedical data knowledge networks.” The group framed its understanding of this concept in terms of the complex and important connection between (1) communities that work with classes of data; and (2) platforms that store, analyze, preserve, and share data for communities. The group discussed several ways to mobilize and leverage communities and platforms to help with cost forecasting. Because these communities are powerful engines for developing standards and establishing practices and norms, he noted that they could predict expected production rates from instrumentation. Lynch explained that in order to forecast access and preservation costs, it is crucial to understand the extent to which access and
preservation encompass the platforms themselves—in other words, what is the life span of platforms before the data go into “hibernation”?
He noted that the best platforms (i.e., the ones that provide incentives and build and strengthen the community) are not passive places for storing and taking data. Rather, they are environments that actively improve and add value to data as well as offer tools to analyze and compare data. Lynch explained that tools in a common environment provide benchmarks against which progress in the field can be measured. He noted that the group considered how to move resources that have risen to a certain level of importance into a more stable, long-term funding cycle. The group also discussed the need for governance of these platforms as well as the need to better understand the relationships between platforms and journals. In response to a topic raised by the previous group, Lynch’s group noted that scholarly societies could play a role in helping to codify membership in this “community.”
Incentivizing Researchers to Determine the Costs of Interoperability
Charles Manski, Northwestern University, explained that several members of his group defined interoperability as the “production-level dissemination” of research. He noted that issues of semantics illustrate the divide between the data community and the research community. For example, he said that many researchers do not use the term “interoperability” or the acronym “FAIR.” With different uses of language, communication between the two groups can become more difficult, he continued.
Manski shared a series of comments made by group members. One participant said that data scientists should be engaged at the start of the research process to assist with dissemination and data sharing. Another participant said that it is difficult to ask researchers to comply with ever-changing specifications for making data available. Researchers are not funded for such activities, and, with the continual change, the process loses value and more closely resembles bureaucracy. Another participant suggested that scientific papers should make science interoperable. The group also discussed ways to incentivize researchers to see that data have value; perhaps the onus should be on the postsecondary institutions, not the researchers, to generate the data, Manski explained. Lastly, the group discussed the sharing of clinical data: Some group members thought that doing so violates patient privacy, while others thought that privacy was simply a mask for data blocking and territoriality.