2
Improving Data Quality and Integrity
Every organization has quality management activities, whether they have been formally planned or not. An organizational quality standard provides guidance on how to develop a formal system to manage these activities (ISO 9000:2015 (E).2.4.2).
The committee’s fourth task was to develop criteria for assessing laboratory protocols and procedures used by the organizations mentioned in Task 2 and to use them to identify best practices and procedures for U.S. Geological Survey (USGS) laboratories. Laboratory procedures are typically assessed using criteria developed by managers, regulatory agencies, or professional organizations. The criteria may incorporate formal quality standards, consensus-based best practices, input from subject matter experts, or feedback from laboratory personnel, collaborators, users, and scientific peer review.
Numerous assessments of laboratory procedures and recommended best practices have been published, and the committee drew from these to address Task 4. This chapter introduces the use of systematic approaches for assuring quality, explains reasons for implementing quality assurance programs, describes example approaches used to do so in research settings, and discusses the training and support needed to integrate and maintain such systems.
INTRODUCTION TO QUALITY ASSURANCE PROGRAMS
A process or product that conforms to requirements or to a quality standard is recognized as a quality process or product (Crosby, 1979). This conformance to requirements demonstrates the ability to meet expectations and is the foundation for establishing value (Guaspari, 2002). The requirements used to define and measure quality can either be established by management (a lead scientist, group, or institution) or adopted from an externally developed, consensus-based quality standard. In either case, management has complete control over, and flexibility around, what the requirements are and how to change them in response to evolving experience or needs. Once the requirements are defined, a quality assurance program is
developed to direct and coordinate the organizational, managerial, and technical activities needed to ensure that the requirements are met.
The steps to build a quality assurance program generally include the following:
- Identify the quality objectives related to a product;
- Assess the risks that may threaten the quality of a product;
- Establish the requirements to reduce the likelihood that risks will be realized;
- Check processes and outcomes to ensure that requirements are met;
- Evaluate, reduce, and learn from errors or problems that occur; and
- Routinely reevaluate risks, processes, and requirements to capture opportunities for improvement.
This systematic approach to managing quality arose from the field of manufacturing in post-World War II Japan. Quality management based solely on individual product inspection (quality control) was replaced by systems that manage quality (including quality planning, quality control, and quality improvements) across all manufacturing processes (Deming, 1982; Juran, 1986; Ortner, 2000; Smith, 2009). These principles underlie modern quality assurance programs used to maintain and demonstrate the quality of a product or process.
REASONS FOR IMPLEMENTING QUALITY ASSURANCE PROGRAMS
Implementation of a laboratory quality assurance program is a recommended best practice because a standard for work is established to assure that the best quality data are generated. Determining whether the work conforms to the standard is part of quality assurance. Standardizing routine activities has the benefit of aligning laboratory scientists around a consensus-based approach for assuring data quality, while avoiding problems stemming from uncontrolled or unintended variation (GBSI, 2013).
A quality assurance program can be implemented by an organization of any size and applied to any kind of work that produces a product (Cochran, 2008; Westgard and Westgard, 2014). In laboratories, the products are primarily analytical data generated and published in reports or journal articles. These data products are typically the outcome of multiple processes executed by different people under different conditions using similar or different methods. For these reasons, standardized and transparent processes that enable accurate reconstruction of all analytical activities and their associated data and metadata are critical for demonstrating data quality and for testing reproducibility of results within and across laboratory environments.
Laboratory quality assurance programs are systematic, process oriented, and intended to address all aspects of the work being done, including management, personnel, equipment, methods, materials, laboratory records and documentation, data management, error management, and facility and environment. The expectation is that data and metadata (the primary
work products) will be managed to assure data quality and integrity throughout the data life cycle, from generation and recording, to processing and analysis, to use, to archive or destruction, as appropriate (MHRA, 2018). Data and information management processes are put in place to ensure that data and metadata are described (who, what, where, when, how, why), documented (e.g., attributable, accurate, complete, and consistent), and preserved for publication, sharing, and re-use. Collectively, these processes contribute to a data governance system and laboratory culture that supports the generation of a complete, consistent, accurate, and secure research record (Wiggins et al., 2013; WHO, 2016; MHRA, 2018).
Quality assurance programs facilitate effective research and data management in laboratory environments because they address organizational, managerial, and technical activities; support data completeness and accuracy; promote process standardization when possible; and assure work transparency and traceability. The key benefits from implementing quality assurance programs include the following:
- Standards for work are established and communicated;
- Activities are planned, managed, documented, and monitored;
- The assessment and mitigation of risks associated with error and uncontrolled or unintended variation are built in;
- An accurate, complete, transparent, and secure data record is maintained and available;
- Re-work is reduced due to reduction in errors and unaccounted variation; and
- Confidence in laboratory processes and competency is increased (Ehrmeyer, 2015).
Standardization and systematic oversight of laboratory practices are considered “good institutional practice” (Begley et al., 2015) because they are designed to reduce variability, manage risks specific to the work being conducted, increase work transparency, and increase the reliability of results within and across laboratory settings (Adams et al., 1998; Trotter et al., 2012; GBSI, 2013; Freedman and Inglese, 2014).
In some manufacturing, clinical, or other regulated laboratory environments, the quality of processes and products must be strictly controlled according to a specified consensus-based or regulatory standard. However, even research laboratories that do not need to comply with regulatory or accreditation requirements are increasingly integrating quality assurance programs and best practices into their operations (Adams et al., 1998; Holcombe et al., 1999; Vermaercke, 2000; Vermaercke et al., 2000; Abad et al., 2005; Robins et al., 2006; Poli et al., 2015; Baker, 2016a; Dirnagle et al., 2018). One driver of this shift are questions raised about the quality and reproducibility of research in disciplines including chemistry, biology, medicine, psychology, economics, physics, engineering, and earth and environmental sciences (Chalmers and Glasziou, 2009; Gratzer, 2013; Ioannidis et al., 2014; Macleod et al., 2014; Begley et al., 2015; Baker, 2016a,b; Bergman and Danheiser, 2016; Bustin and Nolan, 2016; Freedman et al., 2015, 2017; Moher et al., 2016b; Davies et al., 2017; NASEM, 2018b; Ellis et al., 2019).
In response, a number of papers have called for the development and expanded use of consensus-based standards and standardized vocabularies and approaches that support scientific quality (Steneck, 2006; Zimmerman, 2010; Poste, 2012; GBSI, 2013; Plant et al., 2014; Rüegg et al., 2014; Begley et al., 2015; Giesen, 2015; Nosek et al., 2015; Sené et al., 2017). Many laboratories already use available reference standards (purified and traceable biological, physical, or chemical materials) to calibrate equipment or to validate, verify, or authenticate methods. Similarly, written consensus standards that describe optimal practice for specific methods or tests are available. For example, standardized systems for data collection, analytical methods, quality control, and archive have been developed for some procedures by the Long Term Ecological Research program (e.g., Fahey and Knapp, 2004; Robertson et al., 2009). It will take time for such standards to be developed, approved, and adopted within multiple scientific disciplines. However, the adoption of a quality assurance program can facilitate the development and use of standardized approaches for routine activities in a laboratory or laboratory system. For example, some scientists are using quality assurance activities to reduce the potential for errors and uncontrolled or unintended variation in a wide range of factors (e.g., personnel, methods, equipment, or record keeping) that could affect the quality of data (Robins et al., 2006; Zapata-Garcia et al., 2007; Riedl and Dunn, 2013; Bongiovanni et al., 2015; Baker, 2016a; Dirnagl et al., 2016; Hooper et al., 2018; Molinéro-Demilly et al., 2018).
EVIDENCE THAT QUALITY ASSURANCE PROGRAMS SUPPORT DATA QUALITY
Evidence that quality assurance programs are appropriate and effective strategies for maintaining and demonstrating data quality comes from meta-research and reports by organizations and scientists who have integrated quality assurance best practices or programs into their laboratories. Key results from meta-research are summarized below. Examples of scientists’ experiences integrating quality assurance programs into their laboratories appear in “Options for Designing Laboratory Data Quality Programs” below.
Meta-Research on Scientific Practices Associated with Data Quality
Meta-research (research on research) examines the strengths and weaknesses of scientific practices and looks for opportunities for improvement. Scientists have studied how science is conducted (methods), reported (communication), verified (reproducibility), evaluated, and rewarded, and they have recommended improvements that can be applied across scientific disciplines (Casadevall and Fang, 2012; Bond, 2013; Casadevall et al., 2014; Ioannidis et al., 2014, 2015; Casadevall et al., 2016). For example, the scientific community is being encouraged to adopt “open science” policies that make research data and methods freely available so they can be reused and reproduced (e.g., Nosek et al., 2015; Casadevall et al., 2016; Munafò, 2016; NASEM, 2018a; Kretser et al., 2019). As expectations for open science grow, the
importance of being able to assure and demonstrate the quality and integrity of data and metadata becomes even more critical.
Much meta-research on the quality and integrity of data focuses on the biomedical field, which is highly dependent on laboratory-generated analytical data. However, biomedical laboratory methodologies share commonalities with all research disciplines (e.g., method development, equipment and materials management, quality control, and documentation), and so conclusions of meta-research studies are likely applicable to other types of laboratories.
Meta-research shows that routine and careless errors and poor habits occur frequently enough to affect data reliability. For example, Toker et al. (2016) examined 70 human gene expression studies and found discrepancies between the sex indicated by gene expression and the sex identified in the metadata in nearly half of data sets. They also found that “an alarming degree” of sample mislabeling was not being discovered by laboratories, even though detection is straightforward. Zieman et al. (2016) analyzed 35,175 supplementary Excel files from 18 journals. They determined that Excel default settings converted gene names to dates or numbers in about one-fifth of papers with supplementary files containing lists of genes. This problematic Excel feature has been recognized for more than 10 years and yet the errors continue. Ellis et al. (2019) conducted a study to determine whether previously reported and unexpectedly high steroid concentrations in river water could be confirmed. The authors concluded that calculation error (especially related to spreadsheet use) was the most probable cause of the high values reported in the initial study. However, the errors could not always be traced to the source because processing spreadsheets or written laboratory notebook records were not available. Finally, Martinson et al. (2005) examined survey data from a large and representative sample of early- and mid-career U.S. scientists funded by the National Institutes of Health. A substantial fraction of scientists reported engaging in “inadequate record keeping related to research projects” (28 percent) or “dropping observations or data points from analyses based on a gut feeling that they were inaccurate” (15 percent). Typical quality assurance activities, risk assessment, and standardizing and monitoring routine processes would likely have identified and reduced the frequency of these problematic research practices.
APPROACHES FOR DESIGNING LABORATORY DATA QUALITY PROGRAMS
The design and implementation of any process to assure research data quality requires substantial input from scientists to ensure that the process is science centered (incorporates general and field-specific scientific best practices), realistic for the research environment, and fit for purpose (Leonelli, 2017). Likewise, the design and implementation of a system-level approach to assure data quality across an organization needs to be designed to ensure that scientists have the infrastructure and resources needed to demonstrate that they have identified, established, adopted, and maintained appropriate best practices for the work they do.
A variety of approaches can be taken to assure and maintain data quality in laboratory environments. Options range from highly autonomous scientific oversight programs designed to meet individualized requirements to centrally controlled quality management systems (QMSs) designed to meet the requirements of an organization. The committee created four models to illustrate the range of approaches that could be adopted to provide confidence in the quality of data generated in analytical laboratories. The four approaches are
- Scientist-defined procedures and protocols that are implemented at the individual laboratory level,
- Institution-defined best practices that are implemented at the individual laboratory level,
- Institution-defined QMS requirements that are implemented throughout the institution, and
- Externally-defined QMS requirements that are implemented at the institution or individual laboratory level to demonstrate compliance with an external quality standard.
These four approaches are illustrated in Figure 2.1 and discussed below.
Approach 1: Scientist-Defined Procedures
Principal investigators and staff scientists establish their data quality procedures through personal oversight of laboratory-specific processes that are commensurate with scientific practices, in which methods are developed, shared, and adapted among research groups and honed through peer review. Laboratory processes may be managed using a combination
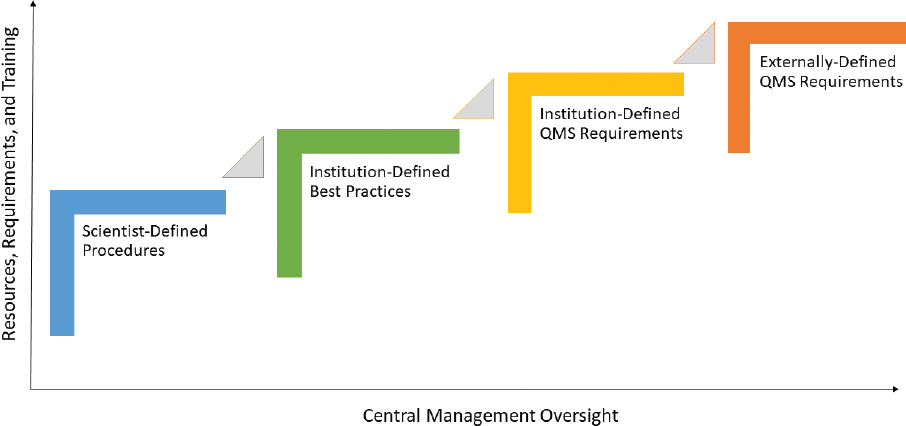
of tools, including written and unwritten policies and procedures, personnel training and mentoring, proficiency testing, the use of electronic laboratory notebooks, automated quality control, and the use of software programs to maintain sample and data records. Examples of this approach are given in Box 2.1.
Advantages and disadvantages of scientist-defined procedures. The primary advantages of this approach to an organization are that
- Scientists have autonomy and flexibility to be creative and innovative in their laboratory methods;
- Scientists develop the procedures needed to address the specific complexity and risk in their environment; and
- Integration of best practices, procedures, and protocols is efficient and inexpensive because scientists follow community practice and there are no additional organizational requirements.
The primary disadvantages of this approach to an organization are that
- Individual practices applied to maintain data quality are highly variable and depend on the commitment, knowledge, training, and resources of the lead researcher and his/her team;
- Quality planning, quality control, and quality improvements may not be integrated across all processes that contribute to data quality and integrity;
- Self-assessment and peer review may not catch data problems quickly;
- Scientists may develop their procedures independently, which creates inefficiencies and may reduce the likelihood for sharing of best practices, tools, and resources within the organization; and
- The lack of consistent or recorded protocols among scientists may hinder the ability to reproduce results across multiple laboratories.
Approach 2: Institution-Defined Best Practices
Best practices are “procedures that have been shown by research and experience to produce optimal results and that are established or proposed as a standard suitable for widespread adoption.”1 In this approach, institutional management establishes and communicates its general expectations for how the quality of data should be maintained and demonstrated across the organization (e.g., Begley et al., 2015). It is then the responsibility of the lead scientist and his or her team to develop a program to meet those expectations. The institutional expectations are directed toward generalized laboratory best practices rather than compliance with a centrally controlled quality standard. For example, the guidelines for nonroutine work (i.e., research) could be flexible and be presented as a toolbox from which relevant best practices could be drawn (Krapp, 2001), whereas guidelines for routine work could include more detailed specifications and policies to assure consistency of the laboratory product.
This approach increases the uniformity of data quality throughout the institution because expectations for quality work and laboratory and data management are communicated and consistent, and awareness of quality assurance is maintained across the scientific organization. In addition, the organizational commitment impels the institution to provide training, tools, and resources to ensure that scientists can successfully meet the expectations.
Many institutions use this approach to encourage the adoption of safety best practices. Safety is frequently managed by requiring individuals to demonstrate that they meet the regulatory or institutional requirements that apply to their specific work. However, scientists are also expected to demonstrate that they have adopted the general best practices that apply to any kind of laboratory work. Typically, institutions offer training and resources to encourage the recognition and adoption of the recommended best practices.
Examples of this approach are given in Box 2.2.
___________________
1 See https://www.merriam-webster.com/dictionary/best%20practice.
Advantages and disadvantages of institution-defined best practices. The primary advantages of this approach to an organization are the following:
- Standardized expectations for data quality and tools, training, and guidelines for scientists should improve the consistency, reliability, and efficiency of processes across the laboratory system.
- The organization demonstrates a commitment to data quality as a core value.
- The approach meets the diverse needs of the laboratory network because scientists have autonomy, creativity, and flexibility in how they meet organizational expectations.
- The alignment of centralized expectations creates opportunities to share training, tools, and resources.
The primary disadvantages of this approach to an organization are the following:
- Institutional management, in accordance with their objectives and in consultation with its scientists, must make the effort to identify the best practices necessary to assure effective data management and quality.
- Scientist-designed programs and their effectiveness will vary across the institution and will be affected by the commitment, training, or resources of the individual researcher.
- If programs do not include an independent or external audit activity, then self-assessment and peer review may not catch data problems quickly. In addition, opportunities to improve working habits may not be realized.
- Costs, support, implementation time, and oversight are higher than for scientist-defined procedures because scientists have to adapt their processes to the recommended guidelines. They will likely need extra time and support to change their routine approach.
Approach 3: Institution-Defined QMS Requirements
An institution-defined QMS is a centrally controlled system designed to assure that the quality objectives of the institution are met. In the laboratory setting, a QMS describes the manner in which a laboratory manages operations to assure the quality of the test results. The system is intended to ensure that all processes that contribute to the laboratory production of data (e.g., raw materials, training, instruments, procedures, sample collection and handling, quality control, and proficiency testing) are managed, conducted, documented, and monitored so that results meet specified quality criteria (Westgard and Westgard, 2014).
This approach differs from the two approaches discussed above because responsibility for managing and demonstrating research quality is shared among (1) the scientists that supervise research activities, (2) the independent quality assurance specialists that support and monitor the quality assurance processes, and (3) the organizational management that establishes the quality standard and provides the resources and oversight necessary to sustain the QMS.
This centralized approach to managing quality is used to achieve consistency, efficiency, and a shared quality culture across the research network. A centralized QMS approach will increase the cost and complexity of research activities because quality assurance professionals are needed to coordinate and monitor multiple managerial and technical activities (e.g., document control, change control, internal auditing, equipment, environment, and error management) across the organization. In addition, staff will require training and support as they take on new or additional quality assurance activities as required within a QMS. The USGS is following this approach. Other examples are given in Box 2.3.
Advantages and disadvantages of institution-defined QMS requirements. Advantages of this approach to an organization include the following:
- A QMS is a recognized and accepted method for assuring confidence in laboratory results.
- The use of a centralized QMS should improve quality, reliability, work transparency, and consistency across the institution.
- An internally defined quality standard can be customized to address the specific needs of an organization.
- Quality assurance experts are available to help scientists implement required QMS procedures and identify and solve data quality problems before they become a larger concern.
- An effective QMS promotes opportunities for self-assessment and improvement of work habits through independent auditing and process review.
Some disadvantages of this approach to an organization include the following:
- QMS requirements established for an entire system may be more applicable to some laboratory activities than to others. For example, laboratory activities directed toward exploratory research or method development will not require the same level of management oversight as routine analytical testing for clients will require. This mismatch may lead to inefficiencies, increased costs, and the loss of flexibility and creativity in exploratory research environments.
- Scientists may be reluctant to adopt a system that they perceive as adding work or restricting their autonomy, flexibility, and creativity (e.g., Vermaercke, 2000; Riedl and Dunn, 2013).
- A fully centralized QMS that spans a diverse and distributed network of laboratories is complex and requires substantial ongoing commitment of management, dedicated quality assurance personnel, and financial resources to implement and sustain.
- Quality assurance specialists with the necessary information management expertise may be hard to find (Rüegg et al., 2014), especially in the numbers needed to support QMS implementation, audit activities, and training initiatives.
Approach 4: Externally-Defined QMS Requirements
With this approach, an institution adopts one or more externally-defined quality standards and develops its QMS to ensure that the requirements of those standards will be met. A laboratory or laboratory system may choose to comply with an external standard because it seeks accreditation by an external agency to carry out a particular line of work, enhance credibility, or meet the specific requirements of clients, collaborators, or regulatory agencies. The laboratory has some flexibility in how it addresses the requirements of the standard in light of its resources, skills, and mission. However, adopting an external quality standard is the most stringent approach for implementing a QMS because the laboratory undergoes accreditation or credentialing assessments.
Multiple standards that apply to laboratory testing have been developed. Examples include the International Organization for Standardization (ISO) standards and the National Environmental Laboratory Accreditation Conference (NELAC) Institute (TNI) standard. Compliance with the ISO 17025 standard (as demonstrated by accreditation status) enables
laboratories to “demonstrate they operate competently, and are able to generate valid results”2 using the accredited method. Compliance with ISO 9001 enables laboratories to demonstrate that they have implemented an effective QMS to ensure that customer and regulatory requirements are met.3 The TNI standard encompasses ISO 17025 requirements, but focuses specifically on environmental data. It is used by the National Environmental Laboratory Accreditation Program to “foster the generation of data of known and documented quality” through the accreditation of environmental laboratories according to consensus standards “representing the best professional practices in the industry.”4
It is possible for laboratories to comply with multiple standards within the same environment. For example, some TNI-accredited laboratories may also have to comply with the additional quality standards established by their own organization. In addition, it is possible for some methods within laboratories to be accredited, while others are not. For example, some quality standards like ISO 17025 are used to accredit individual tests within laboratories, and some standards like ISO 9001 are used to accredit the entire laboratory management system. Experience with either standard will typically build the culture of quality and drive best practices throughout the laboratory (e.g., Abad et al., 2005; Zapata-Garcia et al., 2007; Cutler and Scott-Dupree, 2016).
Examples of the use of externally-defined QMS requirements are described in Box 2.4.
Advantages and disadvantages of externally-defined QMS requirements. The primary advantages of this approach to the institution are the following:
- Compliance with an external quality standard allows a laboratory to conduct analyses that meet regulatory or other consensus-based requirements (e.g., from a professional society) to support high-risk applications and to demonstrate a high level of research accountability through accreditation by independent and external assessors.
- Most formal consensus-based standards are written with the understanding that there are many ways to comply with a given requirement. Therefore, the laboratory can customize how it will meet the requirements.
- Accreditation provides external recognition that the measurement was made under conditions that optimize the likelihood that the measurement is verifiable.
- A laboratory may have both accredited and nonaccredited test methods. If so, the QMS put in place to support the accredited tests is likely to enhance the management of the nonaccredited tests as well.
___________________
2 See International Organization for Standardization ISO/IEC 17025:2017(E); General requirements for the competence of testing and calibration laboratories (current edition 2017-11).
3 See International Organization for Standardization ISO/IEC 9001: 2015-09-15; Quality management systems- Requirements.
4 See National Environmental Laboratory Accreditation Conference Institute (TNI), Management and technical requirements for laboratories performing environmental analysis (2016). http://nelac-institute.org/content/NELAP/index.php.
The primary disadvantages to the institution are the following:
- Demonstrating compliance with an external quality standard requires substantial resources to address the complex array of management, performance, and monitoring requirements.
- An organization may need to maintain more than one quality standard if the scope of the external QMS accreditation does not cover the entire quality system. The result can be (a) a confusing array of requirements, which could lead to inconsistent adoption of requirements, or (b) universal application of potentially unnecessary requirements, which raise costs.
Choosing the Right Approach
Individuals or organizations will choose an approach to maintain laboratory data quality based on their commitment to quality objectives, the inherent risks associated with laboratory activities and outcomes (data, results, reports), their implementation timeline, and the availability of resources needed to plan, implement, and sustain their program. Approaches 1–4 discussed above reflect the increasing complexity, cost, requirements, and oversight needed to assure confidence in the data. Approaches 2–4 are quality assurance programs and recommended best practices, with centralized expectations that a sufficient management system will be in place for all laboratories, and that the effectiveness of the program can be demonstrated throughout all laboratory processes. Scientist-defined procedures and institution-defined best
practices offer the most flexibility and can be implemented at the lowest cost. The centrally controlled QMS approaches provide the most stringent strategies for maintaining and demonstrating data quality, but require the greatest institutional adjustments, the most resources (time, expertise, and funding), and the highest level of support and collaboration.
In some cases, hybrid approaches may meet the needs of an institution. For example, an organization may add independent internal or external auditing to institution-defined best practices to assure that data of known and documented quality are produced and that opportunities for improvement are created. Such monitoring could take the form of independent peer audit, quality assurance review at periodic intervals, round robins (sharing samples at multiple laboratories), or benchmarking (systematically comparing an organization’s practices and procedures with those in other organizations). A quality approach that encompasses both basic research and method development (nonroutine) and production (routine) activities needs to be specifically and carefully designed to be fit for its purpose in each environment. Organizations may also use more than one quality approach. For example, a laboratory may use an institution-defined QMS for most of its methods and externally-defined standards for selected methods. These options may help institutions and scientists balance their needs for flexibility, creativity, productivity, and sustainable quality.
Inherent in the development of any approach is the understanding that not all aspects of research activity can be predicted or predetermined. Science progresses through frequently changing conditions. As a result, the approach used to support and manage data quality needs to be efficient, adaptable, and flexible while also enhancing the scientist’s ability to generate accurate, transparent, complete, and reproducible data (Herman and Usher, 1994; Mathur-De-Vre, 1997; Holcombe et al., 1999; Petit and Muret, 2000; Krapp, 2001; Robins et al., 2006; Abdel-Fatah, 2010; Dirnagl et al., 2018).
MAINTAINING DATA QUALITY PROGRAMS
Implementing quality assurance programs as an organizational strategy to maintain and demonstrate data quality requires a strong and sustained commitment from leadership, management, and laboratory scientists. Every published account describing the integration of quality assurance programs in basic research laboratories stresses the critical need for effective training, communication, collaboration, and support to ensure success and participant buy-in as these changes to laboratory culture and practice are introduced. Scientists and quality assurance professionals need to be able to work together to establish quality assurance programs that will add value to the individual laboratory and to the organization as a whole. They can also combine their subject matter expertise to develop a training program that works. Training is required not only for the data quality approach an organization initially chooses, but also to support different approaches that may be implemented later. These training opportunities are indicated as the triangles between steps in Figure 2.1. For example, adoption of
institution-defined best practices would be strengthened by providing training programs that illustrate how the guidelines can be implemented within individual laboratories, and by creating self-assessment, independent peer-assessment, or quality assurance assessment opportunities and resources. Examples are trainer and trainee handbooks (WHO, 2010a,b) intended to support adoption of the World Health Organization’s quality practices in basic biomedical research (WHO, 2006).
Maintaining quality assurance programs also requires an infrastructure that supports the types of activities needed to comply with the program. For example, quality assurance activities can be supported through the use of tools that make it easier and faster to meet quality requirements. Examples include the use of electronic notebooks, centralized data collaboration and sharing sites, quality management software (record repository and working environment for quality assurance activities), and equipment or laboratory information systems that make the automatic collection of data and generation of records more consistent and reliable. Multiple publications provide examples of programs, procedures, checklists, tools, or guidelines that can be applied in research, development, and production-oriented laboratories to improve data quality (e.g., Herman and Usher, 1994; Adams et al., 1998; Holcombe et al., 1999; Volsen et al., 2004, 2009; Robins et al., 2006; Berwouts et al., 2010; Zimmerman, 2010; Davis et al., 2012; Trotter et al., 2012; Westgard and Westgard, 2014; Giesen, 2015; Dirnagl et al., 2016; Davies et al., 2017; Sené, 2017; Lanati, 2018; Plant et al., 2018)5 or its management (e.g., WHO, 2006; Wiggens et al., 2013; Goodman et al., 2014; Nussbeck et al., 2014; Rüegg et al., 2014; Sandle, 2014; Perkel, 2015; Dirnagl and Przesdzing, 2016; Wilkinson et al., 2016; MHRA, 2018).6 An example of a checklist is given in Table 2.1.
Quality assurance programs succeed when the activities become a routine and rewarded part of the laboratory culture. Therefore, an incentive structure needs to recognize scientist efforts to improve and document data quality (e.g., Casadevall and Fang, 2012; Rüegg 2014; Begley et al., 2015; Moher et al., 2016a). New strategies may be needed to encourage a culture that embraces quality assurance activities.
SUMMARY AND ANSWER TO TASK 4
The committee’s fourth task was to develop criteria for assessing laboratory protocols and procedures and to identify relevant best practices and procedures for USGS laboratories. An extensive literature in biomedical research and other fields evaluates scientific practices that affect data quality, assesses laboratory procedures, and recommends best practices for laboratories. These publications show that errors and poor habits occur frequently enough to affect data reliability, and that this problem could be mitigated by systematically managing
___________________
5 See also the Michelson Prize and Grants Research Quality Assurance toolkit website, https://www.michelsonprizeandgrants.org/resources/quality-assurance-toolkit/.
TABLE 2.1 Laboratory Quality Assurance Documentation Checklist
Project Management: Establish research plan and assess risk to data quality and integrity | Yes | No |
---|---|---|
1. Project plan (roles and responsibilities, objectives, timeline) |
||
2. Research management plan/process/procedures (communication, facilities, environment, security, confidentiality, etc.) |
||
3. Data governance plan/process/procedures |
||
4. Risk assessment and research review plan/process/procedure |
||
5. Research publication plan/process/procedure |
||
Personnel Records: Ensure that records can be traced to competent and appropriate personnel | Yes | No |
1. Job descriptions, resumes, or CVs |
||
2. Signature and initials identification log |
||
3. Training and ongoing competency policies, standard operating procedures (SOPs) for processes related to data management, methods, equipment, quality assurance, and quality control |
||
Equipment Records: Ensure that data and records are traceable to critical equipment | Yes | No |
1. Equipment inventory log (unique identification) |
||
2. Equipment use, maintenance, verification, and calibration records |
||
3. SOPs for use, care, and management of equipment |
||
4. Computer systems used to capture, process, generate, and report data are secure, working as expected, and fit for their intended purpose |
||
Method/Procedure Records: Ensure that data can be traced to methods or procedures that are approved, described, working as expected, and fit for their intended purpose | Yes | No |
1. SOPs for routine research methods |
||
2. Laboratory notebook (paper or electronic) procedure for non-routine research records |
||
3. Method validation records |
||
4. Quality control records |
||
5. Research monitoring records (other quality checkpoints throughout the research life cycle) |
||
Standard Operating Procedures: Ensure that procedures are performed consistently, changed as needed, and maintained as historical records | Yes | No |
1. Routine work instructions for laboratory and data management, established technical procedures, and equipment use are documented and controlled |
||
2. Document control procedures are in place (creation, revision, and archive) to ensure that only the current and approved method is in use |
||
3. SOPs are linked to associated data recording forms |
Other Research Records (paper/electronic): Ensure that research data and critical work (who, what, where, when, how, and why) can be reconstructed | Yes | No |
---|---|---|
1. Reagent inventory, authentication and preparation records (receipt, verification, storage, expiration, and disposition), supply records |
||
2. Facilities data (temperature, water/air quality) if quality critical |
||
3. Unique identification for specimens and sources (data and metadata) |
||
4. Sample handling and storage instructions (SOPs) and records |
||
5. Transparent, reliable, and traceable records (accurate, legible, contemporaneous, original, attributable, consistent, available, and secure) |
||
6. Error management procedures (detecting, recording, managing errors, outliers, and nonconforming work or data) |
NOTE: Modified from Davies et al., 2017.
laboratory processes to assure the quality and integrity of data and records. Quality assurance programs are designed to ensure that the requirements for assessing and improving laboratory performance are met, and that best practices for laboratory activities are routinely identified and adopted. The goal is to establish a quality assurance program that is valued by scientists because of the opportunities it presents to safeguard and demonstrate the quality of their data, rather than one that is resented because it may limit their freedom and creativity.
Approaches to laboratory management vary according to the scope and objectives of the laboratory and organization overseeing it. Best practices relevant to the USGS include
- Institution-defined best practices that are implemented at the individual laboratory level,
- Institution-defined QMS requirements that are implemented throughout the institution, and
- Externally-defined QMS requirements that are implemented to demonstrate compliance with an external quality standard.
Each of these approaches to laboratory data quality has strengths and weaknesses. What they have in common is the expectation that all laboratories will have a sufficient management system in place, and that the effectiveness of that system can be demonstrated throughout all laboratory processes. The success of any of these approaches depends on the full support of management, staff commitment, acceptance of a quality culture, and a system that is simple, flexible, modular, non-redundant, self-sustaining, and value-adding (Vermaercke, 2000).
This page intentionally left blank.