7
Data Science
1. INTRODUCTION
The growing availability of data presents an opportunity to improve the resilience and efficiency of food and agricultural production on a scale unimaginable even one decade ago. The convergence of cloud computing, mobile, Internet of Things (IoT), and analytic technologies has resulted in an explosion of data that has been transformational in all sectors of the economy (Cao, 2016). Approximately 90 percent of the data ever created were generated in the past 2 years (Marr, 2018). By 2025, the amount of data created and copied annually is expected to grow to 1 trillion gigabytes (Seagate, 2017). Understanding the connections between the biophysical and socioeconomic elements of agricultural systems through research depends on generating and analyzing massive amounts of data. Data will be at the center of the next revolution in food and agriculture (UN, 2014).
The relationship between data, algorithms, and computing is symbiotic. In practice, (raw) data are processed by computers through mathematical models and algorithms into useable information. The value of data comes when they are analyzed to provide information that is used to make decisions based on insights and understandings derived from data. Advances in data storage, communications, and processing have led to new research methods and tools that were simply not possible just one decade ago (NIH, 2018). Similarly, breakthroughs in agriculture will benefit from and compel state-of-the-art advances in the areas of data, algorithms, and computing. Machine learning and deep learning are two such examples, which will be further discussed in Section 3.1.
Data science is the emerging field that sits at the nexus of data, algorithms, and computing. It is an interdisciplinary field of inquiry in which quantitative and analytical approaches, processes, and systems are developed and used to extract knowledge and insights from increasingly large and complex sets of data (NIH, 2018). Data analysis has fostered knowledge creation for hundreds of years; achieving the science breakthroughs identified in this report will be spurred by data science.
In June 2018, the United States announced a new record in the global competition to build the world’s fastest supercomputer. The petascale machine called “Summit” can perform 200 petaflops (1015 calculations) per second and is more than twice the speed of the incumbent at China’s National Supercomputing Center (Top 500, 2018). The next horizon is exascale computing; a machine capable of executing 1 billion billion (109 × 109 = 1018) calculations per second. Exascale computing will enable more complete and accurate representations in Earth systems modeling needed to improve our understanding of local to global scales for the food system and enable faster simulations that can, for example, more realistically mirror the speeds of biological processes (McDermid et al., 2017).
Figure 7-1 shows a data analytics maturity curve that progresses from descriptive to predictive to prescriptive. In the early stages, information provided in the descriptive stage lends hindsight on what happened. The predictive stage is the next stage that provides insight into what will happen. The last stage of analytics maturity is prescriptive and enables foresight in what we can make happen. Much progress has been made in food and agricultural research in establishing descriptive analytics. Scientific breakthroughs in food and agricultural disciplines in the next 10-15 years will increasingly address the predictive and prescriptive levels of understanding.
Our understanding of the fundamental scientific underpinnings of the biological, chemical, physical, and socioeconomic elements of the food and agricultural system can benefit from better data access, data integration, and data analytics. The previous chapters have indicated specific instances where access to more data and data science can improve the resilience, efficiency, and sustainability of agriculture. This chapter describes the challenges of collecting, integrating, and analyzing a broad range of data types, and ensuring the quality of those data in near real time to afford the research breakthroughs needed. The chapter then proposes research needs and potential breakthroughs specific to data science for the food and agricultural enterprise.
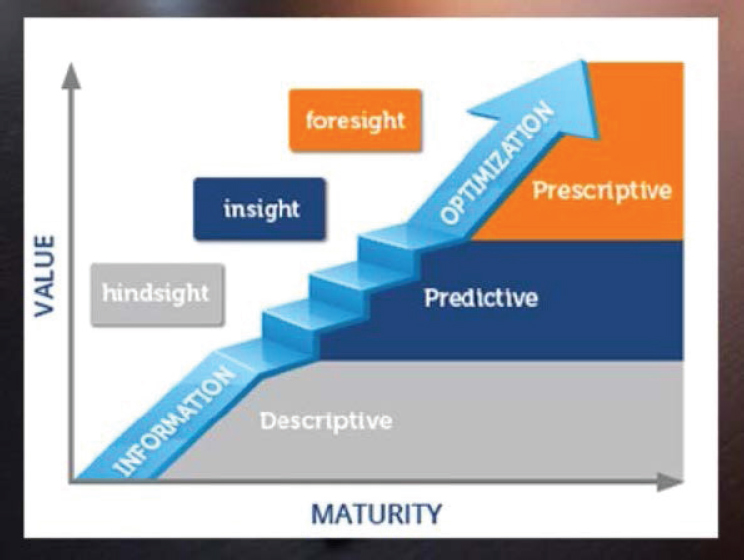
SOURCES: Centurion, 2015, citing INFORMS Analytics Maturity Model User Guide (www.informs.org).
2. CHALLENGES
2.1 Challenge 1: Data Heterogeneity and Dimensionality
The challenges and opportunities in agricultural and food data can be illustrated by the acronym GEMS, which represents the fact that agriculture yields are modeled as a function of genetic (G), environment (E), management (M), and socioeconomic (S) factors. To understand yield, there needs to be an understanding of the genetics of the plant or animal being cultivated, the environmental factors affecting growth, the management practices of the farm, and the socioeconomic factors, as well as an understanding of the complex interactions among these factors, represented as G × E × M × S (GEMS). Numerous environmental factors may be of interest (e.g., location, soil type, elevation, inclination, rainfall, precipitation, humidity, temperature, and dew point) and each may have positive or negative impacts on genotypes. GEMS data are in a wide variety of formats, in
different spatial and temporal scales, with different degrees of accuracy and precision that are challenging to harmonize for analysis and discovery (Lu et al., 2016). Data curation and harmonization efforts currently constitute 80 percent of the effort related to data use (Crowdflower, 2016).
The curation and analysis of GEMS data lead to the need for data standards and tools to manipulate and analyze the massive geospatial temporal datasets, and pushes the frontier of data, analytics, and computing systems (see Box 7-1). The complexities of data, such as the GEMS data, can be explored in terms of the four basic data attributes: volume, variety, velocity, and veracity (see Box 7-2).
Example: Improvements in Sensor Technology and Data Velocity Enabling Real-Time Continuous Monitoring in Agriculture. Novel sensors can provide accurate measures of soil, plant, animal, and food characteristics at a high frequency and data density and at any given time and location. The next generation of sensors will revolutionize the ability to deploy prescriptive solutions in near real time. For example, Figure 7-2 shows how georeferenced in situ soil sensors could be widely distributed across a farm, measure soil and plant nutrients, and display results in a colored geospatial map that allows farmers to determine site-specific actions (e.g., variable application of water and nutrients). Similarly, microelectromechanical technology is already being used to develop miniature sensors that
can be embedded into plant stems for measuring a plant’s water potential (the hydration relative to its growth and production yield) (Pagay et al., 2014); this could replace traditional labor-intensive, destructive methods that provide only point-in-time measurements. Continuous measurements such as these will improve the calibration and performance of water- and nutrient-use models, and may lead to a new understanding of how plants use water and nutrients. Integrating these farm-scale sensor technologies with seasonal or hyper-local weather forecasts, or with measures of water availability in connected ecosystems via the IoT, creates opportunities to manage farm ecosystems integrated with natural ecosystems (e.g., ecohydrology). For vineyards, such an understanding could lead to more consistent production of high-quality wine grapes with certain flavor and
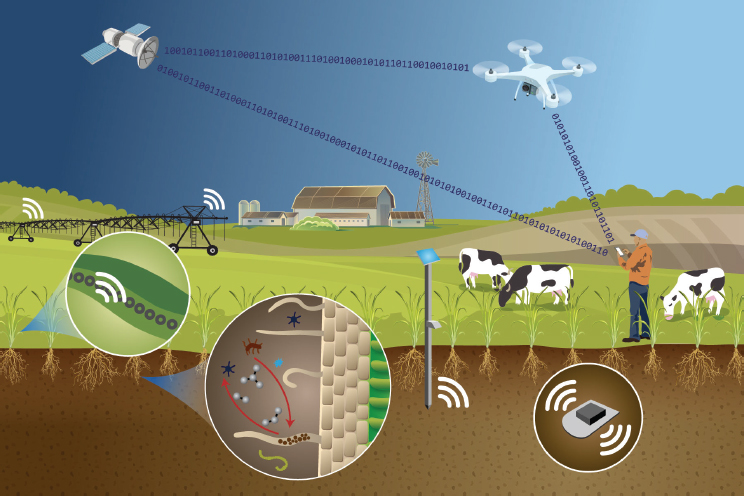
aroma profiles (Campbell, 2016). Likewise, in planta crop health sensors may quantify biochemical changes in plants caused by an insect pest or a pathogen, alerting and enabling the producer to plan and deploy immediate site-specific control strategies before the infestation occurs and damage is visible and widespread across the field. Increased data velocity will enable dynamic control of agricultural equipment in motion in real time—such as precision planters, sprayers, and irrigation—and enable real-time continuous monitoring of individual livestock in a herd using wearable (and other) sensors for precision livestock applications, using advanced technologies such as microfluidics, sound analyzers, image-detection techniques, sweat and salivary sensing, serodiagnosis (diagnosis based on blood sera), and others. Creating efficient online monitoring systems in real time requires the ability to integrate all the available sensor data and run analysis in real time (Neethirajan, 2017).
2.2 Challenge 2: Data Standards and Interoperability
Modern scientific research is becoming a more data-driven, interactive process between multiple stakeholders across the world (EU SCAR, 2015). The term “e-science” has been coined to describe the potential of data-driven and computationally intensive processes to enhance manual laboratory work and fieldwork and reduce dependence on paper-based recording (EU SCAR, 2015). E-science has the potential to enable worldwide collaboration in flexible teams using advanced tools, services, and data repositories. These can include distributed networks or grid computing, high-performance computing, visualizations, simulations, workflows, and provenance documentation (EU SCAR, 2015).
Providing access to data can accelerate and democratize the scientific process. To do so would require infrastructure improvements for supporting the reuse of scientific data in the food and agricultural realm and enhancements for machines to automatically find and use data. While there are some notable cyberinfrastructure initiatives and data-sharing efforts under way (see Box 7-3), these efforts are only able to address some parts of the vast food system. As a set of universally agreed-upon guidelines referring to interoperability among systems or applications, standards support reuse. One of the major hurdles in data standardization and interoperability is the lack of sufficient knowledge representations (e.g., ontologies, semantic nets, and rules) (Jonquet et al., 2018). Knowledge representations provide a representation that a computer system can utilize to solve complex tasks.
The FAIR data principles are a set of guiding principles that could facilitate data standardization and interoperability for scientific data management and stewardships. The principles are organized around four concepts: findable, accessible, interoperable, and reuseable (Wilkinson et al., 2016). The FAIR principles are complementary to open-data philosophy—data can be open without being FAIR, and vice versa. Open data is the idea that some data should be freely available to everyone to use and republish as they wish, without restrictions from copyright, patents, or other mechanisms of control (Auer et al., 2007). Government-funded awards often require researchers to open their data, but there is little guidance on best practices or dissemination for open data in the United States.
The existence of standards is an often-cited success factor for reuse of data and software (Pasquetto et al., 2017). Standards can be administered in a variety of ways: created de facto by private organizations1 or managed by nonprofit organizations.2 The nonprofit consortium of businesses serv-
___________________
1 The computer file format PDF was created by Adobe in 1993 and was a de facto standard for many years before eventually becoming a formal ISO standard in 2005.
2 The nonprofit organization GS1 is best known for the barcode (universal product code) that is scanned by retailers more than 6 billion times every day.
ing the agriculture sector, AgGateway, has identified the need for standards as essential to promoting and enabling the industry’s transition to highly data-driven agricultural practices and is working to develop their own (AgGateway, 2017; Smith, 2017).
2.3 Challenge 3: Data Privacy
Privacy is a confounding issue for food and agricultural data. In some domains, such as health care, data can be anonymized by removing or encrypting personally identifiable information. The best available privacy protection technology, differential privacy, is inadequate for agricultural
data because of its spatiotemporal nature (Shekhar et al., 2017). Anonymizing geospatial data without distorting its granularity is a known problem which does not yet have an adequate solution. Aggregating data is a frequently used approach to anonymize farm- or field-level data. For instance, data from individual fields and farms are aggregated into county-level metrics that are made public. Aggregation limits the granularity of analysis, making intrafield or site-specific analysis impossible. For example, spatial data that are aggregated for anonymization purposes typically lack the high spatial resolution needed by biophysics-based models to accurately capture or predict the system responses.
3. SCIENTIFIC OPPORTUNITIES
There are emerging technologies related to data that can transform the food and agricultural system. In particular, there are four promising future areas: artificial intelligence, blockchain, IoT, and quantum computing.
3.1 Opportunity 1: Artificial Intelligence
Artificial intelligence (AI) refers to intelligence displayed by machines, as opposed to natural intelligence demonstrated by humans and other animals (Bradford, 2017). The term AI is commonly used when a machine mimics cognitive functions that are typically associated with humans, such as planning, learning, reasoning, problem solving, knowledge representation, perception, motion, manipulation, social intelligence, and creativity. AI combines automation, robotics, and computer vision. Advances in statistics, faster computers, and access to large amounts of data have enabled advances in AI, particularly in the field of machine learning where significant progress has been made in the areas of image and pattern recognition, natural language understanding, and robotics.
The scale and complexity of the food and agricultural system make it well suited for AI, with large amounts of data available to train algorithms. Large amounts of data from ever-more-sophisticated experiments and models are being generated, and food and agricultural researchers are challenged with deciding what data to collect and how to best process the data generated. A 2016 analysis estimated that a new crop protection product requires the analysis of more than 160,000 compounds—the equivalent to more than 11 years of research and development and overall costs that exceed $280 million per commercial product (Brayne et al., 2018). AI will enable new discoveries, not just in analyzing existing data but in augmenting human creativity around planning new experiments and accelerating discovery and improving efficiency.
There are promising applications and indicators for AI in agriculture. In selective breeding programs, the development of high-throughput automated phenotyping capabilities can speed the process of breeding to develop resilient yet high-yielding, high-quality crops. AI and machine-learning research on the massive amount of environmental, growth, and genetic data can help scientists, for example, elucidate the connections between system components, predict the yield of different varieties based on early-season plant attributes, and associate specific desirable traits with genetic markers (Baxter et al., 2007). Robots can be designed to harvest crops at a higher volume and faster pace than human laborers, to monitor crop and soil health using computer vision and deep-learning algorithms that analyze data captured by drones, and to more accurately predict crop
yields using machine-learning models to better understand environmental impacts (Sennar, 2017). Box 7-4 on robotic milking provides an example of integrating AI with sensor technologies in animal agriculture.
3.2 Opportunity 2: Blockchain
Blockchain is a recent technology advancement with potential for addressing the challenge of creating a more transparent, authentic, and trustworthy digital record of the journey that food and other physical products take across the supply chain (Lougee, 2017). Blockchain technology arose out of the efforts to create the cybercurrency Bitcoin, but its potential goes well beyond its original purpose. As discussed in Chapter 4, at its core, the blockchain is a shared, immutable ledger for recording the history of transactions (IEEE, 2018a). It uses a variety of technologies, including public–private key cryptography, distributed databases, decentralized processing, hash functions, and consensus algorithms (Ge et al., 2017).
This could be valuable in creating transparency in a global food system with multiple disparate actors across the multitiered supply chain.
More information will be required to address research challenges on food and agricultural supply chains and a platform for studying how complex systems interact. Blockchain presents a potential new source of data and new platform to be leveraged to deliver advances toward transparency to the food system and transformations in food safety, fraud reduction, market access, waste reduction, and productivity gains (Ahmed and ten Broek, 2017; Ge et al., 2017; Kim and Laskowski, 2017).
A systematic mapping study designed to understand the current research topics, challenges, and future directions of blockchain technology from a technical perspective examined 41 primary papers (Yli-Huumo, 2016). It showed that most of the current research on blockchain technologies has been focused on security and privacy issues. Issues in scalability, such as performance and latency, need to be addressed in order to realize pervasive use of blockchain technology in the food system.
3.3 Opportunity 3: IoT
IoT is the network of physical devices embedded with electronics, software, sensors, actuators, and connectivity which enables these “things” to connect and exchange data creating opportunities for more direct integration of the physical world into computer-based systems, resulting in efficiency improvements, economic benefits, and reduced human exertions (Wasik, 2013; Morgan, 2014). Among its advantages, IoT addresses the high cost of manual data collection, which impedes the adoption of beneficial data-driven technologies. IoT technologies enable seamless data collection from various sensors, cameras, and drones and are the foundation for intelligent systems, as illustrated in the example above and throughout this report. Farm applications of precision agriculture and precision livestock farming are prime examples of IoT; however, the influence of IoT spans the entire food system (Wolfert et al., 2017).
IoT is still in its infancy in the food and agriculture sector (Verdow et al., 2016). Realizing future subsystems within the food system where IoT devices are capturing and sending data, but in a time-sensitive and synchronized way, “could be stalled by our lack of effective methods to marry computers and networks with timing systems” (NIST, 2015).
Challenging requirements for food and agriculture applications need to be addressed in both the technical and nontechnical arenas. For example, on-site applications in remote outdoor farming locations often lack Internet connectivity needed for communications. Easy access to power and durability and calibration are also essential. The FarmBeats system design explic-
itly accounts for weather-related power and Internet outages, which has enabled 6-month-long deployments in two U.S. farms (Vasisht et al., 2017).
3.4 Opportunity 4: Quantum Computing
Quantum mechanics may play a vital role in biology, as quantum phenomena occur in biological systems. In 2007, it was discovered that photosynthesis operates though quantum coherence, giving rise to the new field of quantum biology (Engel et al., 2007; Ball, 2011). Other examples of quantum phenomena relevant for food and agriculture include olfaction (Turin, 2002), DNA mutation (Lowdin, 1965), and Brownian motors in many cellular processes (Krug et al., 2006). The emergence of quantum computing could be used to explore natural phenomena in the physical world—phenomena that traditional computing paradigms are ill suited to represent at scale.
Quantum mechanics is a subdiscipline of physics that explains the physical world and attempts to explore how it functions (Feynman, 2002). Nature follows the laws of quantum mechanics, in which particles behave in “strange” ways. For example, caffeine molecules in a cup of coffee are difficult to model, and the detailed structure and properties are difficult to understand because the particles can take on more than one state and can even interact with other particles that are far away. The computers currently in use have not been able to take into account such complex properties because classical computers code information in bits that represent 0 or 1 values. On the other hand, quantum computers operate on the basis of qubits and incorporate two key principles of quantum physics: superposition and entanglement. Superposition allows a qubit to simultaneously take on the value of both 0 and 1, and entanglement allows qubits in a superposition to be correlated with one another (the state of one can be dependent on the other). Classical computers use a binary format as on-off switches, while quantum computers use qubits that can act as sophisticated on-off switches and can be used to solve more complex problems in quantum scales (including nature) (IEEE, 2018b).
Quantum computing technology is in its infancy. Many fundamental challenges exist, including developing long-living qubits for computation, scaling the number of qubit processors, and building effective quantum error correction. However, major efforts are under way across the globe in academia and industry and promising early results are unfolding (Knight, 2017; Alibaba, 2018; Google, 2018; IBM Q, 2018; Intel, 2018; Microsoft, 2018). In 2016, the first publicly available quantum computing service on the cloud was made available. Quantum computers may lead to revolutionary breakthroughs in discovery of new materials for agriculture, agrochemical discovery, and artificial intelligence algorithms that could impact
food and agriculture. Molecular modeling has been identified as an area for exploration with quantum computing (Emerging Technology from the arXiv, 2017). Another area in which quantum computing could help with is analyzing genomics data for plants, animals, or the soil microbiome. Quantum computing could make it possible to perform real-time analytics on various types of data (e.g., weather data at high spatial resolution) (Accenture Labs, 2017; Popkin, 2017). This unique juncture in time provides an opportunity for food and agricultural research to help shape the next era of computing.
4. BARRIERS TO SUCCESS
Data science is a rapidly evolving field and data science skills for working with Big Data are in high demand in all sectors, including food and agricultural research and agricultural economics (Woodward, 2016). Data science tools need to become more useable by non–computer science domain experts. Traditional agricultural and food science programs are evolving to better integrate the variety of disciplines needed; for instance, the digital agriculture initiative at Cornell University is aimed at generating innovating research at the intersection of agriculture, computing, and engineering (Cornell University, 2018). New partnerships, prizes, and conferences are needed to spark convergence between those who are new to food and agriculture and current food and agricultural researchers. Initiatives such as the Syngenta Crop Challenge in Analytics awarded by the Analytics Society of INFORMS show promise in fostering much-needed cross-industry collaboration (INFORMS, 2018). Reward systems for generating and sharing data in research environments may need to be considered, for example, creating incentive structures or changing the approach to tenure and promotion evaluations (USDA-NIFA, 2017b). It will be important to train a workforce that can manage, analyze, and manipulate large datasets and enable the workforce’s convergence with food and agricultural scientists. Furthermore, it may be necessary to develop a culture that supports and rewards sharing of data (including combinations of private and public data) by sets of communities of researchers, standardizes protocols, harmonizes experimental designs, and addresses ownership, privacy, and security concerns unique to the food and agricultural enterprise. Funding sources, public or private, need to be available for developing the standards and protocols that need to be harmonized for agricultural datasets to make them interoperable.
The collection of data from numerous stakeholders is required to create the pool of Big Data needed to well represent the multidimensional food system. To incentivize data sharing by individual stakeholders or researchers, reward systems may require new technologies that can iden-
tify and appropriately attribute value derived from the pool of Big Data to the individual contributions. These value attribution technologies may be conceptually similar in spirit to marketing attribution, the process of identifying a set of user actions (events, touchpoint) that contribute in some manner to a desired outcome, and then assigning a value to each of these (Priest, 2018), but will need to account for the unique complexities in the food and agricultural domain.
Ownership of food and agricultural data can be a confounding issue, especially when it comes to IoT data. Ownership is a legal concept related to property, and U.S. law recognizes various categories of property (for example, real property, personal property, and intellectual property). However, data generated in the food and agricultural sectors may not belong in any of those categories of property. For example, farming data is a compilation of data generated from field operations (real property) using sensors on equipment (personal property) and represents intellectual property on maximizing yield. Questions emerge around legal ownership of data (which confers control) and how the data can be used. There are currently few legislative or judicial rulings for guidance. The American Farm Bureau Federation developed the Privacy and Security Principles for Farm Data (AFBF, 2014), which lays out 13 data principles, but these are only guidelines. Users of data will need to consider the implications of data ownership, including protecting ownership of data, abiding by legal usage, and ensuring appropriate sharing with others. Ambiguity and uncertainty around ownership can cause inefficiencies and limit sharing and value creation.
5. RECOMMENDATIONS
Advances in data sciences can transform how data can be better collected, analyzed, and used for food and agricultural research. While there are many opportunities, the following actions merit high priority:
- Accelerate innovation by building a robust digital infrastructure that houses and provides FAIR (findable, accessible, interoperable, and reuseable) and open access to agri-food datasets.
- Develop a strategy for data science in food and agricultural research, and nurture the emerging area of agri-food informatics by adopting and influencing new developments in data science and information technology in food and agricultural research.
- Address privacy concerns and incentivize sharing of public, private, and syndicated data across the food and agricultural enterprise by investing anonymization, value attribution, and related technologies.
REFERENCES
Accenture Labs. 2017. Innovating with Quantum Computing: Enterprise Experimentation Provides View into Future of Computing. Available at https://www.accenture.com/t00010101T0000000w/br-pt/acnmedia/PDF-45/Accenture-Innovating-QuantumComputing-Novo.pdf (accessed April 26, 2018).
AFBF (American Farm Bureau Federation). 2014. Privacy and Security Principles for Farm Data. Available at https://www.fb.org/issues/technology/data-privacy/privacy-and-security-principles-for-farm-data (accessed April 26, 2018).
AgGateway. 2017. AgGateway Releases Annual Report and 5-Year Plan: Enable Companies to Increase Efficiency, Agility and Profitability. Available at http://www.aggateway.org/Newsroom/2017PressReleases/AgGatewayReleasesAnnualReportand5-YearPlan.aspx (accessed April 26, 2018).
Ahmed, S., and N. ten Broek. 2017. Food supply: Blockchain could boost food security. Nature 550(7674):43.
Alibaba. 2018. Alibaba Cloud and CAS Launch One of the World’s Most Powerful Public Public Quantum Computing Services. Available at https://www.alibabacloud.com/pressroom/alibaba-cloud-and-cas-launch-one-of-the-worlds-most (accessed July 9, 2018).
Allan, G., J. Erdmann, A. Gustafson, A. Joglekar, M. Milligan, G. Onsongo, K. Pamulaparthy, P. Pardey, T. Prather, S. Senay, K. Silverstein, J. Wilgenbusch, Y. Zhang, and P. Zhou. 2017. G.E.M.S: An innovative agroinformatics data discovery and analysis platform. Available at https://rdmi.uchicago.edu/papers/08162017165531paperwilgenbusch081617.pdf (accessed July 9, 2018).
Auer, S. R., C. Bizer, G. Kobilarov, J. Lehmann, R. Cyganiak, and Z. Ives, Z. 2007. DBpedia: A Nucleus for a Web of Open Data. The Semantic Web. Lecture Notes in Computer Science. 4825. P. 722. doi: 10.1007/978-3-540-76298-0_52.
Ball, P. 2011. Physics of life: The dawn of quantum biology. Nature 474:272-274.
Baxter, I., M. Ouzzani, S. Orcun, B. Kennedy, S. S. Jandhyala, and D. E. Salt. 2007. Purdue Ionomics Information Management System. An integrated functional genomics platform. Plant Physiology 143(2):600-611.
Bradford, A. 2017. Empirical evidence: A Definition. LiveScience. Available at https://www.livescience.com/21456-empirical-evidence-a-definition.html (accessed July 9, 2018).
Brayne, S., S. McKellar, and K. Tzafestas. 2018. Artificial Intelligence in the Life Sciences & Patent Analytics: Market Developments and Intellectual Property Landscape. London: IP Pragmatics Ltd. Available at https://www.ip-pragmatics.com/media/1049/ip-pragmaticsartificial-intelligence-white-paper.pdf (accessed July 9, 2018).
Brouček, J., and P. Tongel’. 2015. Adaptability of dairy cows to robotic milking: A review. Slovak Journal of Animal Science 48(2):86-95.
Campbell, G. 2016. The Tensiometer: Micro-sized. Environmental Biophysics. Available at http://www.environmentalbiophysics.org/tensiometers-micro-sized (accessed July 9, 2018).
Cao, L. 2016. Data science and analytics: A new era. International Journal of Data Science and Analytics 1(1):1-2.
Centurion, C. 2015. Moving Along the Analytics Maturity Curve. River Logic Blog. Available at https://blog.riverlogic.com/moving-along-the-analytics-maturity-curve (accessed July 9, 2018).
Cornell University. 2018. Digital Agriculture: Cornell University Agricultural Experiment Station. Available at https://cuaes.cals.cornell.edu/digital-agriculture (accessed April 26, 2018).
Crowdflower. 2016. Data Science Report. Available at http://visit.crowdflower.com/rs/416ZBE-142/images/CrowdFlower_DataScienceReport_2016.pdf (accessed July 9, 2018).
de Koning, K. 2010. Automatic milking—Common practice on dairy farms. Pp. V59-V63 in Proceedings of the First North American Conference on Robotic Milking. Elora, ON, Canada: Precision Dairy Operators.
Emerging Technology from the arXiv. 2017. Google Reveals Blueprint for Quantum Supremacy. MIT Technology Review. Available at https://www.technologyreview.com/s/609035/ google-reveals-blueprint-for-quantum-supremacy (accessed July 9, 2018).
Engel, G. S., T. R. Calhoun, E. L. Read, T. K. Ahn, T. Mancal, Y. C. Cheng, R. E. Blakenship, and G. R. Fleming. 2007. Evidence for wavelike energy transfer through quantum coherence in photosynthetic systems. Nature 466:782-786.
EU SCAR (European Union Standing Committee on Agricultural Research). 2015. Agriculture Knowledge and Innovations Systems Towards the Future: A Foresight Paper. Strategic Working Group AKIS-3 Report. Available at https://ec.europa.eu/research/scar/pdf/akis-3_end_report.pdf (accessed March 15, 2018).
Feynman, R. 2002. Richard Feynman on quantum physics and computer simulation. Los Alamos Science No. 27. Los Alamos National Laboratory. Available at http://permalink.lanl.gov/object/tr?what=info:lanl-repo/lareport/LA-UR-02-4969-02 (accessed July 9, 2018).
Ge, L., C. Brewster, J. Spek, A. Smeenk, and J. Top. 2017. Blockchain for Agriculture and Food: Findings from the Pilot Study. Wageningen Economic Research. Available at https://www.wur.nl/upload_mm/b/3/d/df37c4ff-14f3-43b9-a34d-6ed8599d8aba_2017-112%20Ge_def.pdf (accessed July 9, 2018).
Google. 2018. A Preview of Bristlecone: Google’s New Quantum Processor. Available at https://ai.googleblog.com/2018/03/a-preview-of-bristlecone-googles-new.html (accessed July 9, 2018).
IBM Q. 2018. What Is Quantum Computing? Available at https://www.research.ibm.com/ibm-q/learn/what-is-quantum-computing (accessed March 15, 2018).
IEEE (Institute of Electrical and Electronics Engineers). 2018a. Blockchain Overview. Available at https://blockchain.ieee.org/about (accessed March 15, 2018).
IEEE. 2018b. Quantum Computers Strive to Break Out of the Lab. Available at https://spectrum.ieee.org/computing/hardware/quantum-computers-strive-to-break-out-of-the-lab (accessed March 15, 2018).
INFORMS (Institute for Operations Research and the Management Sciences). 2018. Syngenta Crop Challenge. Available at http://connect.informs.org/analytics/news-events/news-events-articles/news-events-news-events-articles-syngenta (accessed April 26, 2018).
Intel. 2018. Intel Starts Testing Smallest “Sping Qubit” Chip for Quantum Computing. Available at https://www.intc.com/investor-relations/investor-education-and-news/investor-news/press-release-details/2018/Intel-Starts-Testing-Smallest-Spin-Qubit-Chip-forQuantum-Computing/default.aspx (accessed March 15, 2018).
IOM and NRC (Institute of Medicine and National Research Council). 2015. A Framework for Assessing Effects of the Food System. Washington, DC: The National Academies Press.
Jonquet, C., A. Toulet, E. Amund, S. Aubin, E. D. Yeumo, V. Emonet, J. Graybeal, M. Laporte, M. A. Musen, V. Pesce, and P. Larmande. 2018. AgroPortal: A vocabulary and ontology repository for agronomy. Computers and Electronics in Agriculture 144:126-143.
Kim, H. M., and M. Laskowski. 2017. Agriculture on the blockchain: sustainable solutions for food, farmers, and financing. Available at http://dx.doi.org/10.2139/ssrn.3028164.
Knight, W. 2017. IBM Raises the Bar with a 50-Qubit Quantum Computer. MIT Technology Review. Available at https://www.technologyreview.com/s/609451/ibm-raises-the-bar-with-a-50-qubit-quantum-computer (accessed March 15, 2018).
Krug, H., H. Brune, G. Schmid, U. Simon, V. Vogel, D. Wyrwa, H. Ernst, A. Grunwald, W. Grunwald, and H. Hofmann. 2006. Nanotechnology: Assessment and Perspectives. Springer-Verlag Berlin and Heidelberg GmbH & Co. K. Pp. 197-240.
Lougee, R. 2017. Are “Bytes” and “Blocks” the Secret Ingredients to Transforming Food Safety? SwissRe Institute. Available at http://institute.swissre.com/research/library/Food_Safety_Robin_Lougee.html (accessed March 15, 2018).
Lowdin, P. 1965. Quantum genetics and the aperiodic solid. Some aspects on the biological problems of heredity, mutations, aging and tumours in view of the quantum theory of the DNA molecule. Advances in Quantum Chemistry. Volume 2. Pp. 213-360. Academic Press.
Lu, S., L Shao, M. Freitag, L. Klein, J. Renwick, F. Marianno, C. Albrecht, and H. Hamann. 2016. IBM PAIRS Curated Big Data Service for Accelerated Geospatial Data Analytics and Discover, 2016 IEEE International Conference on Big Data. Available at https://ieeexplore.ieee.org/document/7840910 (accessed March 15, 2018).
Marr, B. 2018. How Much Data Do We Create Every Day? The Mind-Blowing Stats Everyone Should Read. Available at https://www.forbes.com/sites/bernardmarr/2018/05/21/how-much-data-do-we-create-every-day-the-mind-blowing-stats-everyone-should-read/#1d51008860ba (accessed July 9, 2018).
McDermid, S., L. Mearns, and A. Ruane. 2017. Representing agriculture in Earth systems models: Approaches and priorities for development. Journal of Advances 9(5):2230-2265.
Microsoft. 2018. Microsoft Quantum: Research. Available at https://www.microsoft.com/enus/research/lab/quantum (accessed March 15, 2018).
Morgan, J. 2014. A Simple Explanation of “The Internet of Things.” Available at https://www.forbes.com/sites/jacobmorgan/2014/05/13/simple-explanation-internet-things-thatanyone-can-understand/#958725c1d091 (accessed July 9, 2018).
Neethirajan, S. 2017. Recent advances in wearable sensors for animal health management. Sensing and Bio-Sensing Research 12:15-29.
NIH (National Institutes of Health). 2018. NIH Strategic Plan for Data Science. Available at https://commonfund.nih.gov/sites/default/files/NIH_Strategic_Plan_for_Data_Science_Final_508.pdf (accessed March 15, 2018).
NIST (National Institute of Standards and Technology). 2015. Lack of Effective Timing Signals Could Hamper “Internet of Things” Development. Available at https://www.nist.gov/news-events/news/2015/03/lack-effective-timing-signals-could-hamper-internet-thingsdevelopment (accessed March 15, 2018).
Pagay, V., M. Santiago, D. A. Sessoms, E. J. Huber, O. Vincent, A. Pharkya, T. N. Corso, A. N. Lakso, and A. D. Stroock. 2014. A microtensiometer capable of measuring water potentials below −10 MPa. Lab on a Chip 14(15):2806-2817.
Pasquetto, I., B. Randles, and C. Borgman. 2017. On the reuse of scientific data. Data Science Journal 16:8.
Popkin, G. 2017. Quantum computer simulates largest molecule yet, sparking hope of future drug discoveries. Science. Available at http://www.sciencemag.org/news/2017/09/quantum-computer-simulates-largest-molecule-yet-sparking-hope-future-drug-discoveries (accessed March 15, 2018).
Priest, C. 2018. How Machine Learning Can Help Marketers Measure Multi-Touch Attribution. Available at https://www.ama.org/partners/content/Pages/how-machine-learningcan-help-marketers-measure-multi-touch-attribution.aspx (accessed July 9, 2018).
Salfer, J., M. Endres, W. Lazarus, K. Minegishi, and B. Berning. 2017. Dairy Robotic Milking Systems—What Are the Economics? eXtension. Available at http://articles.extension.org/pages/73995/dairy-robotic-milking-systems-what-are-the-economics (accessed April 26, 2018).
Seagate. 2017. Data Age 2025. Available at https://www.seagate.com/www-content/our-story/trends/files/Seagate-WP-DataAge2025-March-2017.pdf (accessed March 15, 2018).
Sennar, K. 2017. AI in Agriculture—Present Applications and Impact. Techemergence. Available at https://www.techemergence.com/ai-agriculture-present-applications-impact (accessed March 15, 2018).
Shekhar, S., J. Colletti, F. Munoz-Arriola, L. Ramaswamy, C. Krintz, L. Varshney, and D. Richardson. 2017. Intelligent Infrastructure for Smart Agriculture: An Integrated Food, Energy and Water System. Computing Community Consortium white paper. Available at https://arxiv.org/ftp/arxiv/papers/1705/1705.01993.pdf (accessed March 15, 2018).
Smith, W. 2017. Uncertainty and Opportunity: Recent News Clips of Interest. Presentation, AgGateway 2017 Annual Conference: Efficiency-Opportunity-Profitability, November 6-9, 2017, San Diego, California.
Stephens, Z. D., S. Y. Lee, F. Faghri, R. H. Campbell, C. Zhai, M. J. Efron, R. Iyer, M. C. Schatz, S. Sinha, and G. E. Robinson. 2015. Big data: astronomical or genomical? PLoS Biology 13(7):e1002195.
Top 500. 2018. Available at https://www.top500.org/lists/2018/06 (accessed March 15, 2018).
Turin, L. 2002. A method for the calculation of odor character from molecular structure. Journal of Theoretical Biology 216(3):367-385.
UN (United Nations). 2014. A World That Counts. Available at http://www.undatarevolution.org/report (accessed March 15, 2018).
USDA-NIFA (U.S. Department of Agriculture’s National Institute of Food and Agriculture). 2017a. Big Data Yields Big Opportunities in Agriculture. Blog. Available at https://www.usda.gov/media/blog/2017/07/17/big-data-yields-big-opportunities-agriculture (accessed March 15, 2018).
USDA-NIFA. 2017b. Executive Summary: Changing the Face, Place, and Space of Agriculture. Available at https://nifa.usda.gov/sites/default/files/resource/Data%20Summit%20Summary.pdf (accessed March 15, 2018).
Vasisht, D., Z. Kapetanoic, J. Won, X. Jin, R. Chandra, A. Kapoor, S. Sinha, M. Sudarshan, and S. Stratman. 2017. FarmBeats: An IoT Platform for Data-Driven Agriculture. 14th USENIX Sympostium on Networked Systems Design and Implementation, March 27-29, 2017. Boston, MA. Available at https://www.usenix.org/system/files/conference/nsdi17/nsdi17-vasisht.pdf (accessed March 15, 2018).
Verdow, C., S. Wolfert, and B. Tekinerdogan. 2016. Internet of Things in Agriculture. Available at https://www.researchgate.net/publication/312164156_Internet_of_Things_in_ agriculture (accessed March 15, 2018).
Wasik, B. 2013. In the Programmable World, All Our Objects Will Act as One. Available at https://www.wired.com/2013/05/internet-of-things-2 (accessed July 9, 2018).
Wilkinson, M. D., M. Dumontier, I. J. Aalbersberg, G. Appleton, M. Axton, A. Baak, N. Blomberg, J.-W. Boiten, L. Bonino da Silva Santos, P. E. Bourne, J. Bouwman, A. J. Brookes, T. Clark, M. Crosas, I. Dillo, O. Dumon, S. Edmunds, C. T. Evelo, R. Finkers, A. Gonzalez-Beltran, A. J. G. Gray, P. Groth, C. Goble, J. S. Grethe, J. Heringa, P. A. C ’t Hoen, R. Hooft, T. Kuhn, R. Kok, J. Kok, S. J. Lusher, M. E. Martone, A. Mons, A. L. Packer, B. Persson, P. Rocca-Serra, M. Roos, R. van Schaik, S.-A. Sansone, E. Schultes, T. Sengstag, T. Slater, G. Strawn, M. A. Swertz, M. Thompson, J. van der Lei, E. van Mulligen, J. Velterop, A. Waagmeester, P. Wittenburg, K. Wolstencroft, J. Zhao, and B. Mons. 2016. The FAIR Guiding Principles for scientific data management and stewardship. Scientific Data 3:160018.
Wolfert, S., L. Ge, C. Verdouw, and M. Bogaardt. 2017. Big Data in smart framing—A review. Agricultural Systems 153:69-80.
Woodward, J. 2016. Big data and ag-analytics: An open source, open data platform for agricultural and environmental finance, insurance, and risk. Agricultural Finance Review 76(1):15-26.
Yang, Z., G. Yu, L. Di, B. Zhang, W. Han, and R. Mueller. 2013. Web service-based vegetation condition monitoring system—VegScape. Pp. 3638-3641 in 2013 IEEE International Geoscience and Remote Sensing Symposium (IGARSS). Institute of Electrical and Electronics Engineers.
Yli-Huumo, J., D. Jo, and S. Choi. 2016. Where is current research on blockchain technology? A systematic review. PLoS ONE. Available at https://doi.org/10.1371/journal.pone.0163477 (accessed March 15, 2018).