3
Socioeconomic Status, Health, and Mortality in Aging Populations
Angela M. O’Rand and Scott M. Lynch
Duke University
INTRODUCTION
Nearly 25 years ago, Preston and Taubman (1994) summarized major observations from demographic research on socioeconomic differences in adult mortality and health in a National Research Council volume on the Demography of Aging. By then, the associations between socioeconomic status (SES) and health and mortality were widely reported, although the measurement of these phenomena was not firmly established nor were the causal relationships between them. Most notable among the findings they reported was the widening of educational differences in mortality for men over the previous three decades, evident especially in the magnitude of educational differences in heart-disease death rates among White men. Mortality caused by cardiovascular disease had declined overall, but the majority of educational differences in mortality were attributable to differentials in heart disease for all sex–racial/ethnic groupings. Similarly, educational differentials in disability, poor health, and chronic conditions were evident by midlife (ages 45–54), although differences appeared to diminish at later ages. Finally, their evaluation of the growing literature on the effects of numerous intervening, proximate variables such as health behaviors (including smoking), personality, social relationships, environmental factors, and access to medical care revealed the “persistent failure” of these variables to account fully for the relationship between education and health.
The authors considered alternative measures of SES and adopted the most commonly used measure in the literature, education (years of school-
ing), as the most reliable choice for their analyses because of its stability within individuals, its interval measurement scale, and its unique significant association at that time (based mainly on cross-sectional studies) with health behaviors and cardiovascular risk. Education’s apparent effects were widely attributed to cognitive skills and greater access to information. Other measures of SES were considered to be limited by issues of endogeneity, including selectivity and reverse causation. Occupation, for example, excludes persons who do not work (the retired, housewives, the disabled), a status that may be determined by health. Income suffers from the same problem of endogeneity since health may limit hours worked. In addition, income can be measured in several ways (earnings, transfers, savings, other household members’ incomes), each with different implications for health outcomes. They did acknowledge that education may also be affected by long-term disability and its effects on later morbidity and mortality, but they found reverse causation to be less of a problem in the general population.
The robust relationship between SES and health continues as a dominant theme in the demography of aging. Since the publication of the 1994 Preston and Taubman summary (referred to as PT94 in the remainder of this essay), life course theory has emerged as a key organizing framework for examining the relationship between SES and health over longer periods of the life span, during which the onset, duration, co-occurrences, and sequential contingency (if not causal relationship) between SES and health-related events can be observed. The development of life course theory has been facilitated as longitudinal databases have matured and have incorporated increasingly precise measures of health and disease and as they have been augmented via linkages to administrative data such as Social Security, Medicare, and/or patient records. Linkages to administrative data have also improved the measurement of mortality and health costs (especially with access to Medicare records) relative to reported income and to the diverse geographical contexts of aging (using Decennial Census, health and vital statistics, and GPS data). Genomic databases have emerged most recently to add precision to the measurement of the etiology of diseases and may contribute to the resolution of questions regarding causality, especially as gene-by-environment interactions can be more readily modeled. Further, analytic statistical tools for dealing with endogeneity, in particular selectivity and other unobserved heterogeneity, have been developed to better assess causality, although the complexity of this relationship over time poses continuing challenges.
This chapter begins by examining the current relationship between SES, mortality, and health and the major advances in understanding this relationship since PT94. Then we review the contribution of the life course framework to understanding this relationship across the life span and
the analytical challenges in doing so. We then summarize the biological and behavioral pathways in the SES-health-mortality association that are current foci of demographic research and conclude by considering the approaches and challenges of these foci for selected future directions in research.
SES, HEALTH, AND MORTALITY IN THE 21ST CENTURY
In this section we conduct analyses that update PT94. We use data from the Integrated Health Interview Survey (IHIS), a combined collection of the National Health Interview Survey (NHIS) (Minnesota Population Center and State Health Access Data Assistance Center, 2015). NHIS is a nationally representative, repeated cross-sectional survey of community-dwelling persons (National Center for Health Statistics, 2014). We use two major health outcomes in our analyses: all-cause mortality and self-rated health (SRH). Our main goal is to evaluate whether the broad patterns in the association between SES and health that PT94 reported from 1960 through the mid-1980s have changed or have continued in recent decades. Because these are not entirely comparable data to those used in PT94, we cannot perfectly extend the results reported there.
Mortality Analyses
Mortality data have been incorporated into the IHIS from 1986 to 2009, with the survey tracking mortality to 2011 (National Center for Health Statistics, 2015). Using these data, we constructed a person-year dataset (with individuals limited to a 5-year follow-up period) and estimated a number of discrete time logistic regression models (Allison, 1984) predicting mortality by age group (30–44, 45–54, 55–64, 65–74, and 75+ years; measured with four dummy variables). We disaggregated the data and estimated models separately for two time periods (respondents interviewed between 1986 and 1995 and those interviewed between 1996 and 2005) for four different educational attainment groups (<12 years of schooling, 12 years, 13–15 years, 16+ years) and by sex and racial/ethnic group (non-Hispanic Whites and non-Hispanic Blacks; hereafter, just “Whites” and “Blacks”). The models are thus nonparametric. The models predict the 1-year mortality probability for a person in a given 15-year age group whose interview data are in the IHIS in the given period. The mortality probabilities are therefore age group–specific annual period probabilities. Greater detail on the methodology is available from the authors.
Figures 3-1 and 3-2 show the results of the mortality analysis for females and males, respectively. In each figure, the upper panel shows the results for both time periods for Whites, while the lower panel shows
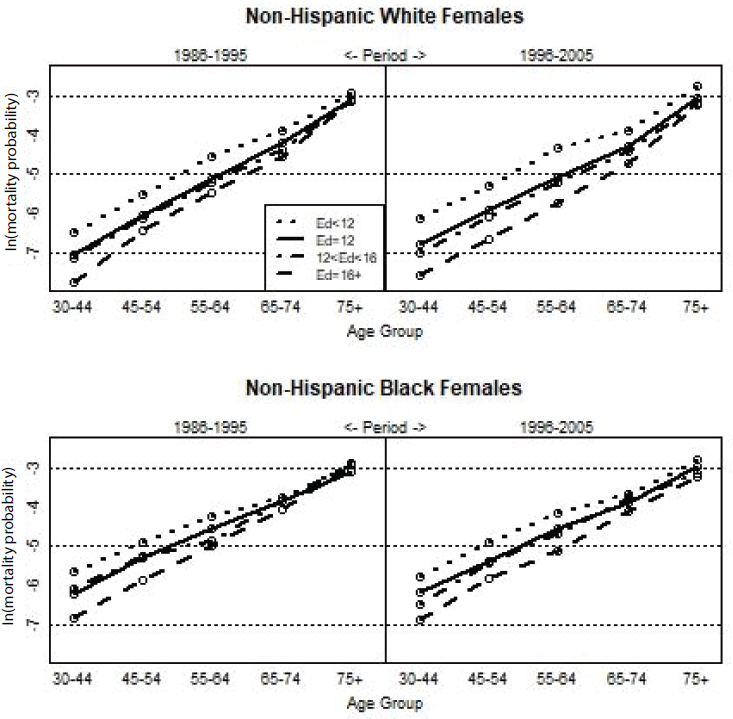
results for Blacks. The figures report mortality probabilities in log scale. In general, log mortality probabilities increase fairly linearly with age. In all cases, there is a clear education gradient in mortality, with persons with a college degree or more (educational attainment ≥ 16 years) evidencing the lowest mortality rates and persons with less than a high school diploma (educational attainment < 12 years) evidencing the highest rates. In most cases, however, there is little difference in mortality risk for persons with a high school diploma (education = 12 years) compared to those with some college (12 < education < 16 years). The education gradient tends to shrink across age for all sex–racial/ethnic groups, so that the largest mortality risk differences are observed for persons aged 30–44. The gradient seems more
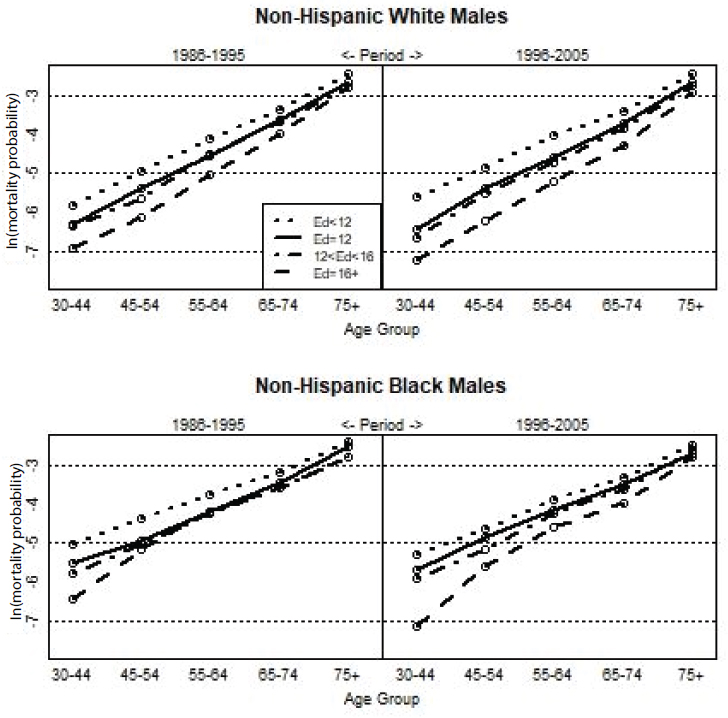
pronounced for Whites than for Blacks, and this is especially so for females. Consistent with all prior research on mortality, White mortality risk is lower than Black mortality risk for both sexes for each education category and for almost all age groups. The Black-White disparity appears to shrink at the oldest ages, however. Finally, the gradient appears to be larger for all sex–racial/ethnic groups in the more recent period.
In summary, our mortality analysis shows that the broad patterns of socioeconomic differences in mortality found by PT94 continue: Educational differentials are large, continue to become more pronounced over time, and disproportionately benefit those with the most schooling. These
patterns are true for both Whites and Blacks but are more apparent for Whites.
SRH Analyses
SRH is a commonly used measure of general health, and SRH data have been collected in NHIS since 1972. We use data from 1986 forward to obviate the need to collapse the measure because of a change in outcome category measurement in 1982 and to keep consistent with the periods used in our mortality analyses. From 1982 forward, the survey asked respondents to rate their health as “excellent,” “very good,” “good,” “fair,” or “poor.” We coded these outcomes sequentially with integers from 4 to 0, respectively, for our analyses.
We use SRH as our health measure for several reasons. First, SRH is the only health measure that has been collected in every wave of NHIS. Second, SRH is well known to be a reliable and valid measure of general health, and it does not suffer from selection problems that some other measures have, such as asking about health limiting the respondent’s ability to work. That is, measures regarding work limitations are only relevant for persons who work, and limitations may depend on the kind of work one does. Third, we feel that a general measure is better than any specific one for assessing long-term trends because, in any period, specific health conditions may differentially increase or decrease in incidence and prevalence, thereby providing only a partial picture of health patterns.
Our analyses of SRH follow the same strategy used in our mortality analyses. We created five age groups and four education groups as before, but we separated the data into three periods: 1986–1995, 1996–2005, and 2006–2015. We estimated separate linear regression models for each sex-by-race-by-period-by-education group, with dummy variables for age groups in each model. We then computed predicted scores for all combinations. As with the mortality models, the models are nonparametric, given the extent of our disaggregation.
Figures 3-3 and 3-4 show the results. Figure 3-3 shows the results for females, while Figure 3-4 shows the results for males. In both figures, the upper plot shows results for Whites and the lower plot shows results for Blacks. The results show a strong educational gradient in health. Persons with educational attainment of a college degree or more have the best health, whereas those with less than a high school diploma have the worst health. Unlike the results from the mortality models, the models for health reveal a clear difference in health for persons with a high school diploma compared to those with some college for all subgroups, with the exception of Black males, ages 65–74, in the earliest period (1986–1995).
In all plots, the gradient in health appears to widen across age groups

up to 55–64 before narrowing in subsequent age groups. This result is consistent with numerous studies published since the 1990s (e.g., House et al., 1994; Lynch, 2003) and appears to be the result of a more rapid decline in health in early adulthood for persons with less schooling than for persons with more schooling, coupled with a floor effect on the measure. That is, while health continues to decline across age for persons with higher levels of schooling, the health decline for persons with low levels of schooling begins to level off at ages beyond midlife, leading toward convergence in health across education groups in later life. The underlying cause of the leveling-off of health for the least educated could be selective survival of the most robust members of the least-educated population, or it could be that health ratings are increasingly made in reference to one’s peers at older ages; both arguments have been made in the literature.
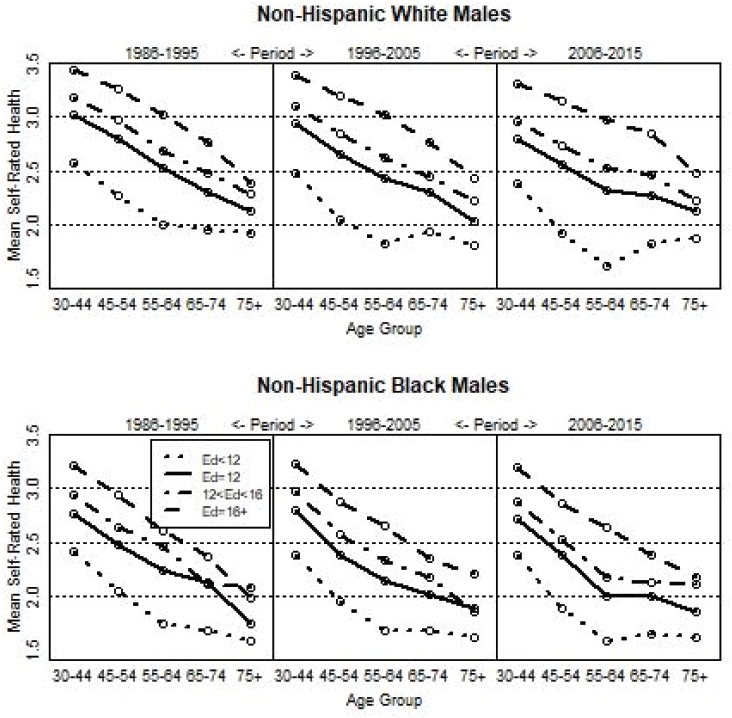
As with the results of the mortality model, the gradient tends to be stronger for Whites than for Blacks, for both men and women. Additionally, health for Whites is better than health for Blacks, with the exception that health for Blacks with less than a high school diploma is often better than health for Whites at the same level of schooling. Finally, health appears to have been fairly stable across the three periods within educational attainment groups, in some case even evidencing a slight decline. Given that overall population health has generally improved, increasing educational attainment has thus masked education-specific declines. That is, those with the most schooling have the best health, so that slight declines in health even at the highest levels of schooling are offset at the aggregate level by a greater percentage of persons obtaining more schooling. Further,
large observed educational gains over the last several decades imply that the low-education groups are becoming increasingly negatively selective: those who do not obtain higher levels of schooling are increasingly a less healthy subset of the overall population.
Overall, our mortality and health analyses, as well as a large recent literature, show that the patterns and trends observed in PT94 have continued. Most notably, the educational gradient in health and mortality is strong and growing, and Whites tend to have a stronger gradient than Blacks.
THE LIFE COURSE LENS ON SES, HEALTH, AND MORTALITY
Findings from the social, psychological, epidemiological, and biological sciences have converged regarding patterns in the associations among SES, health, and mortality. They have converged generally along two lines. First, they have independently observed that adult disparities in health and mortality are significantly correlated with childhood socioeconomic conditions and health (Schafer et al., 2011; McEwen and McEwen, 2017). Developmental and epidemiological studies hypothesize that the enduring impact of these early conditions may reflect “critical” or “sensitive” periods of biological and behavioral development that are highly responsive to environmental factors and that these changes tend to endure over the life course (Ben-Shlomo and Kuh, 2002; Shanahan and Hofer, 2011). The critical period hypothesis attempts to identify the limited time window during development in which an individual is subject to adverse or protective influences on structural development. The Barker hypothesis has identified the prenatal period as a critical period when nutritional or other environmental deprivation or damage produces lifelong consequences for health including gene transcription (Barker, 1995). The sensitive period hypothesis posits that an organism experiences more than one developmental period with an elevated vulnerability to disease risks. Exposure to persistent socioeconomic adversity and health risks in childhood, adolescence, or young adulthood can lead to the “biological embedding” of diverse behavioral and biological phenotypes that endure throughout adulthood (Hertzman and Boyce, 2010).
The second line of multidisciplinary convergence is that the differential accumulation of exposures to lower SES and to related successive health risks over time produces differential trajectories of health across adulthood. These independent observations of accumulation processes have yielded multiple terminologies across disciplines, resulting in some confusion. (Ferraro and Morton [2016] provide clarifications and guidelines.) Chains of risk, cumulative exposure, cumulative adversity, and weathering are terms, among others, that are intended to capture how one exposure increases the likelihood of subsequent exposures and how the chain of such
exposures additively accumulate to produce poor health. Epidemiologists think of this as cumulative exposure, where risks accumulate within the body as one is exposed to adverse conditions over the life course. Cumulative disadvantage theory provides the dominant sociological framework for these observations of widening heterogeneity and inequality observed within aging cohorts experiencing differential opportunities and risks over time (Dannefer, 1987; DiPrete and Eirich, 2006). Early-life conditions matter in this theory, but broader institutional and environmental sources of inequality throughout the life course are also implicated. Institutional arrangements such as educational systems, institutional racism, health care policies, and labor market conditions can serve to reinforce, amplify, or reduce inequalities in the population. Linking cumulative exposure and disadvantage, some scholars identify the biology of disadvantage as the current frontier of aging research (Adler and Stewart, 2010; Wolfe et al., 2012). An integrative theory of cumulative inequality now proposes a deductive framework for hypothesis testing based on this biosocial linkage (Ferraro and Shippee, 2009). Cumulative inequality is depicted as a path-dependent biosocial pattern in the life course with persistent direct links between earlier and later socioeconomic and health conditions.
Despite considerable recent interdisciplinary agreement regarding divergence of health trajectories, PT94 reported that educational health disparities peaked at ages 55–65 and then declined, a finding that replicated earlier results (Kitagawa and Hauser, 1973) and concurrent research (House et al., 1994), including ours above. Why disparities decline after midlife at older ages has been a focus of some debate since 1994. Almost every empirical study, especially those using cross-sectional data, has confirmed that health disparities narrow in later life. A common explanation for this pattern is that aging levels the playing field in health, both biologically and socially. And, with the exceptions of automobile accidents, sudden heart attacks, and other health shocks, biological processes dominate social processes and everyone’s health eventually declines prior to death. Furthermore, social policies such as Medicare may equalize access to health care, effectively slowing health decline for older persons of lower SES (House et al., 1994). An alternative to this “age-as-leveler” view is mortality selection: persons of lower statuses who are particularly susceptible to the detriments of lower status are selected out of the population via mortality at a faster rate than persons who are less susceptible, leaving a robust subset of those with lower status (Dupre, 2007). The result is that aggregate health disparities appear to diminish at older ages, although the health trajectories for, say, any pair of low-versus-high status individuals continue to diverge, as expected by the cumulative disadvantage perspective (Lynch, 2003; Willson et al., 2007).
Numerous studies continue to support the cumulative disadvantage hypothesis through middle age by documenting the persistent effects of SES
and childhood health conditions on mortality, disability, disease risk, and perceived well-being (e.g., Elo and Preston, 1992; Hayward and Gorman, 2004; Hamil-Luker and O’Rand, 2007). Many of these analyses draw on data from the Health and Retirement Study (HRS), which collects repeated, multiple measures of health and economic status but also depends on retrospective accounts of childhood conditions related to parental education, parental employment, financial strain, welfare receipt, and father’s absence. The latter data pose potential challenges to validity based on biased or poor memories, diminished cognitive capacities, or depressive symptoms at the time of survey. Although some disagreements on the validity of these data persist, the recall of conditions of severe adversity and poor health appears to be largely valid after controlling for current cognitive level (see Haas, 2007; Vuolo et al., 2014).
THE CAUSALITY CONUNDRUM
Nearly as soon as the association between SES and health was discovered, scholars began questioning whether the relationship was causal. Early research questioned the causal direction of the relationship, whereas more recent research asks whether the association is causal in general. The PT94 discussed the selection-causation problem in their original chapter, but research on the topic at the time was limited in two major ways. First, most research on the relationship between SES, health, and mortality involved cross-sectional data, so there were few avenues for resolving the dilemma. Second, most research was limited to adults and few surveys asked about early-life SES or health, both of which precede status and health in adulthood. Indeed, much of the research at that time in demography, epidemiology, and sociology assumed a causation perspective and sought to identify intervening mechanisms that explained the SES-health relationship. This assumption was often explicitly made as a justification for using educational attainment as the measure of SES. Research over the last two decades, however, has shown that, although early-life socioeconomic conditions affect later-life health, early-life health also affects cognitive development, thereby playing a major role in influencing educational attainment and therefore occupational status and earnings (Case et al., 2002). Thus, we consider the original selection-causation question to be overly simple: it is clear that the relationship between SES and health is reciprocal across the life course of individuals and is, in fact, intergenerational.
Contemporary research has turned to assessing more generally the extent to which the relationship between SES and health is causal. This growing attention has been enabled by the collection of several long-term panel datasets, such as the HRS and the National Longitudinal Survey of Youth, as well as by the development of methods and software for analyz-
ing panel data. The most prevalent methods in use for analyzing panel data include fixed effects and random effects methods. The former are preferred in economics, and justifiably so.
Fixed effects methods control out all sources of time-invariant unobserved heterogeneity that may confound relationships between SES and health outcomes. However, there are some key limitations to applying these methods in this genre of research. First, fixed effects methods require measures to be time varying, and education is fixed by age 30 for more than 95 percent of the population. Studies that apply fixed effects methods to education data therefore may resolve endogeneity due to omitted variables at the expense of inducing sample selection–based endogeneity. Second, although income is also commonly used as a measure of SES, income is volatile. So sample selection issues are less problematic. However, treating income as a predictor of health in a fixed effects model begs the question of causal direction, and lagging income does not resolve the problem and may even exacerbate it (Vaisey and Miles, 2017).
Random effects methods are commonly used in research outside of economics, in part because of the requirement that variables must be time varying in fixed effects models. Over the last two decades, countless studies have been published using random effects methods, including studies using random intercept models, random coefficient (or [latent] growth) models, and latent class models. Although these studies have taught researchers much about how SES is associated with long-term patterns of health, random effects methods are not causal methods, despite common misconceptions. Random effects methods merely yield efficient measures of association, net of controlled variables. For example, growth models yield unique intercepts and slopes for individual health trajectories, but this simply resolves the violation of the non-independence of errors assumption of regression modeling by clustering a portion of the error term. In short, the random effects are part of the error term, and so the key assumption of these models is that the random effects are uncorrelated with other regressors. Thus, the “random effects assumption” does not address any endogeneity concerns due to omitted variable bias or reciprocality.
In economics, the use of natural experiments is becoming increasingly common in an attempt to establish the causality of the relationship between SES and health. Several studies have attempted to exploit policies, such as changes in compulsory schooling, in this effort (e.g., Lleras-Muney, 2005). The argument is that because individuals do not generally influence large-scale schooling laws, changes in the age at which students can drop out of school are exogenous shocks that differentiate attainment for younger birth cohorts over older birth cohorts. Thus, mortality (or health) differences between cohorts that cannot be explained by other, remaining factors
must be a result of the change in educational attainment. In general, this strategy may resolve issues regarding both direction of causality and omitted variables.
Despite their celebration in economics, we are not sanguine regarding the usefulness of natural experiments in this genre of research for several reasons. A key problem with natural experiments is that they almost surely violate the stable-unit-treatment-value assumption (SUTVA) of causal modeling (Imbens and Rubin, 2015). The essence of SUTVA is that the effect of a treatment is not influenced by treatment of others. In the context of the relationship between education and health, SUTVA implies that one person’s receipt of additional schooling has no implication for the effect of the receipt of another person. One of the key arguments for using educational attainment as a measure of SES is that it is strongly related to, and prior to, two other key measures: occupation and income. Yet employers make hiring decisions and wage determinations at least in part on the basis of educational attainment. If everyone entering the labor market gains additional schooling, especially at a relatively low level, such gains do not change the relative position of anyone in the labor market, and so the policy change is likely to have minimal observable effect on health through occupation and income, two major factors implicated in the education-health association.
Our more general criticism of natural experiments applies not just to them but also to all methods attempting to determine “the” causal effect of SES on health. We question whether the notion that there is a causal effect is reasonable for two main reasons. First, as PT94 found, and Lynch (2003) also found, the association between SES and health has strengthened across birth cohorts born in the last century. Thus, the effect of SES on health is not a single, stable quantity: it is context dependent. Second, Link and Phelan (1995) argued that SES was a “fundamental cause” of disease: over time, status is ALWAYS related to health. However, the predominant health outcomes extant in a society, and the mechanisms that link SES to them, change over time.
This argument also suggests that there is no single causal effect of SES on health for two additional reasons. First, how education affects downstream measures of status such as occupation and income differs by birth cohorts. For example, although Mirowsky and Ross (2003) made a strong case for measuring educational attainment as years of schooling, for use in health disparities research, because education’s primary effect is via cognitive development, Lynch (2006) showed that an increasing proportion of the association between education and health across birth cohorts is accounted for by education’s association with income. Further, more-recent research by Hayward et al. (2015) showed that education’s association with mortality is increasingly nonlinear and follows a stair-step (or “sheepskin”)
effect, indicating that education’s key contribution to mortality reflects the importance of the acquisition of credentials (i.e., high school diplomas and college degrees). Changes in the importance of credentials (versus years of schooling) across birth cohorts for several other health outcomes, including SRH, obesity, and activity limitations, should probably be addressed further.
Second, how status relates to particular health outcomes changes over time. As PT94 noted, death rates due to heart disease declined dramatically from 1960 to 1994 and persons in different educational categories experienced differential rates of decline. In contrast, cancer deaths did not evidence the same rates of decline at either the population level or the education subgroup level. Thus, a study examining “the causal effect” of a policy change affecting educational attainment (for example) on one health outcome may reach a very different conclusion from one investigating a different health outcome, especially if such studies involve different birth cohorts.
In summary, we think that some progress has been made and will continue to be made in resolving—or at least delimiting—the causality conundrum. However, despite the fact that long-term longitudinal data and clever methods are increasingly available, the increasing complexity of theory—reflecting our increasing awareness of the complexity of the SES-health association—will most likely limit our ability to resolve the conundrum fully.
THE BIOLOGY OF DISADVANTAGE: BIOLOGICAL AND BEHAVIORAL PATHWAYS LINKING SES AND HEALTH
Increasing integration of survey data with medical biomarkers and other biological data over the last two decades has broadened life course research to include more precise consideration of physiological, psycho-dynamic, and neurocognitive processes that unfold across different contexts of development and aging. However, the literature in this highly interdisciplinary area is vast and varied, often yielding either incomparable findings based on different study designs (e.g., nationally representative surveys versus clinical or community studies) or seemingly inconsistent results across studies with similar designs (e.g., health selection versus SES effects on midlife health outcomes using national surveys). Consequently, the field still has not yet fully explained the SES-health-mortality association.
Nationally representative longitudinal surveys of different cohorts have added and updated biomarker and genomic data since the mid-1990s (e.g., HRS, Wisconsin Longitudinal Study, the Midlife in the United States study, the National Social Life, Health, and Aging Project, and Add Health) and
some researchers have linked these data to other information sources such as health records, death indices, and Medicare files to augment self-reported (or proxy-reported) data and enhance validity. But the addition of these data has come later in the lives of study participants than would be ideal for purposes of explanation, and typically only subsamples of participants have been involved. Long-running epidemiological databases (NHIS and the National Health and Nutrition Examination Survey) continue to add new samples and biomarkers of biological processes and new measures of acute and chronic health outcomes, although their demographic information remains relatively sparse, thus limiting the inclusion of potentially confounding variables. Smaller community and clinical studies abound, often focused on specific measures of biological processes such as inflammation and on health outcomes such as cardiovascular disease or hypertension, but their dependence on cross-sectional data and their sample selectivity limit their comparability. Taken as a whole by our assessment, what emerges from these widely varying efforts are the following empirical generalizations:
- SES differentials vary by behavioral or biological process (e.g., on average, smoking is more influenced by SES than is obesity).
- SES differentials vary for different health outcomes (e.g., on average, cardiovascular disease is more influenced by SES than is hypertension).
- N-way interactions (e.g., SES × race × gender × region) make interpretations of SES differentials a challenge, especially across studies.
Hence, precise biosocial linkages are still not firmly established, and challenges in the collection of biomarker and genomic data and their integration with other life course data persist (Gruenewald, 2013). Nevertheless, the formative role of environments, including SES and other social factors across the life course, remains central to the agenda of the demography of aging. The major question that persists is, “How do socially graded environmental factors elicit biological and behavioral responses that are cumulative and have durable effects on health and longevity?” The answer to this question will enable the answer to the next: “How can social-medical interventions alter adverse health trajectories?”
Biological Pathways
Several biological pathways of dysregulation in major physiological systems have been identified as associated with SES (see Wolfe et al., 2012). These pathways consist of disease precursors measured by biomarkers related to stress, inflammation, and immunosenescence; metabolic
syndrome; and neurocognitive function that develop over the life course, long before they are typically diagnosed. Dysregulation in one system can effect changes in other systems and probably follows “a progression from neuroendocrine hormonal dysregulation and chronic inflammation (primary mediators) to dysregulated cardiovascular and metabolic systems (secondary mediators) to organ system dysfunction and disease (tertiary outcomes)” (Wolfe et al., 2012, p. 45). Therefore, these pathways appear to follow different age trajectories, with some racial/ethnic and gender variations, ultimately influencing differential onsets of life-threatening diseases and death (Crimmins et al., 2006). They are also intertwined with the relative adaptive capacities of individuals to resist stress and to recover “normal” physiological states as a result of medical and social interventions (Yashin et al., 2016).
Because these physiological processes must be measured with samples from saliva or blood or by instrumental readings of vital processes (e.g., blood pressure), data collection is often limited to cross-sectional, non-representative samples—that is, clinical, community, and older populations after the onset of disease—or data are collected on national samples without demographically rich information on the life course (Wolfe et al., 2012). Data collection has become more cost-effective as a result of technologies that can garner more information from single collections; this is leading to greater incorporation of these measures in longitudinal social surveys even though data collection occurs later in the life courses of participants. The HRS has added these data recently on subsamples of recent waves, to improve precision of measurements of these processes across cohorts in the U.S. aging population (Crimmins, 2013).
Stress and Allostatic Load
The stress process tradition in the social sciences (Wheaton et al., 2013) has focused most on the roles of acute and chronic stress on allostatic load (Juster et al., 2010), which was once measured primarily via cortisol levels but is now usually measured as a composite of biomarkers from several physiological systems, such as C-reactive protein, glycated hemoglobin, diastolic and systolic blood pressure, total cholesterol, high-density lipoproteins, body mass index (BMI) and hip-to-waist ratios, and resting pulse rate. Allostatic load in older populations has been treated as an indicator of frailty: the depleted capacity to resist stress or to rebound to normal functioning (Crimmins et al., 2006).
Cumulative life stresses associated with lower SES and poverty have been a major focus in the demography of aging research that emphasizes the biology of disadvantage. Stress is treated as a predominant means by which the immediate and perceived environment “gets under the skin.” Disadvan-
taged childhood conditions, including poor health, anchor lifetime trajectories of exposures to risks and behavioral and biological responses to them that persist and proliferate across physiological systems. The life course consequences among the disadvantaged include earlier aging and premature death (Belsky et al., 2017; Crimmins et al., 2009; Levine and Crimmins, 2014), earlier onset of disability and disease (Hamil-Luker and O’Rand, 2007), lower adult income associated with more rapid disease or disability progression (Herd et al., 2007), and greater disability and shorter life expectancy across U.S. contexts (Montez et al., 2017; Chetty et al., 2016).
Inflammation and Immunosenescence
Inflammation is a response at the cellular level to trauma or infection that, when sustained at chronic levels, leads to mitochondrial damage, a degradation of the immune and endocrine systems, and chronic disease. Biomarkers of inflammation include C-reactive protein, interleukin-6, insulin resistance, type 2 diabetes, and T-cell markers of aging, some of which are associated with other biological pathways such as allostatic load and metabolic syndrome. The inflammation response is initially protective: intended to destroy pathogens, repair the affected tissues, and return the organism to normal functioning. But chronic inflammatory levels (immunosenescence) associated with poverty and other disadvantaged environments appear to increase the pace of aging (Crimmins and Finch, 2006; Crimmins et al., 2009). For example, disadvantaged environments increase the exposure to infections and the susceptibility to chronic infections that are implicated later in life in the negative association between income and T-cell markers of aging (Aiello et al., 2016).
Metabolic Syndrome
This syndrome includes a group of risks that strongly predict heart disease, diabetes, and stroke. These risks include high fasting blood sugar and insulin resistance, abdominal obesity, high BMI, high blood pressure, high triglyceride levels, and low LDL cholesterol. The prevalence of this syndrome appears to be (a) spreading in the population globally (GBD Collaboration, 2014); (b) emerging at higher rates earlier in the life course among successive U.S. cohorts, especially among women (Reither et al., 2009); and (c) becoming a candidate to surpass smoking as a leading killer (GBD Collaboration, 2014).
Health behaviors (summarized below) are strongly implicated in this pathway. The effect of excessively high BMI (BMIs well into the obese range) on risks of disease and all-cause mortality has now been observed across gender and age groups in U.S. and other populations (Freedman et
al., 2006). Lower SES in childhood, lower education, and lower income in adulthood are associated with high BMI, insulin resistance and diabetes, and death related to these causes (Wolfe et al., 2012), although a good deal of this research comes from small cross-sectional samples.
Neurocognitive Development and Decline
Neuroscientists are relying on additional metrics besides biomarkers (e.g., beta-amyloids) to assess the relationship between SES and cognitive development in the young and cognitive decline in the old. These metrics include electroencephalography, which measures electrical activity on the scalp and partially reflects activity of neurons and other brain cells, and magnetic resonance imaging, which produces images of the brain’s anatomy and responses in the brain to sets of stimuli including tasks and/or visual images. These techniques provide data on the development and organization of the brain and the dynamics associated with cognition (e.g., visual-spatial information, language and syntax, executive functioning) and emotion (e.g., fear), which develop in childhood and adolescence (e.g., Hanson and Hackman, 2012).
A large literature reports that SES is positively associated with children’s cognitive and emotional development (e.g., McEwen and McEwan, 2017). This substantial literature also reports that SES is negatively associated with adverse physical and psychological environments to which children are exposed as they develop (Evans, 2004), including toxins, poor nutrition, stress, parental neglect, lack of cognitive stimulation and nurturance, and neighborhood violence. Issues of causality are now prime preoccupations of this literature, much as they are for most life course research.
In later life, SES has been associated with measures of cognition (e.g., positively with working memory and negatively with processing time), but its relationship to rates of cognitive decline, especially with dementia and Alzheimer’s disease is less clear (Wilson et al., 2006). Some evidence exists that higher SES enables easier adaptation to and compensation strategies for normal cognitive decline (Alwin and Hofer, 2011). However, although Alzheimer’s disease and related dementias increase with age into the eighth and ninth decades of life, SES differentials have not been clearly identified at the oldest ages. In the case of these diseases, some progress has been made in biological research identifying genetic and inflammatory (beta-amyloid) precursors (Hardy and Selkoe, 2002).
Social Genomics
The relationship between genetics and aging has been a decades-long interest pursued initially through twin-sibling studies and then via target or candidate gene approaches. Another long-running focus has been on telomere length as a biomarker of aging. Telomeres, nucleoprotein structures at the ends of eukaryotic chromosomes, have been observed to shorten during cellular aging. Associations between telomere attrition and several indicators of disease and of mortality have been observed across (mainly cross-sectional and clinical) human studies (Mather et al., 2011). Thus, this area demands more longitudinal research on representative populations. New approaches in social genetics have shifted to genome-wide association studies (GWASs) that leverage DNA sequence variations called single-nucleotide polymorphisms to detect variation in disease susceptibility, as in the well-known case of the apolipoprotein E mutation associated with higher risk for Alzheimer’s disease. More recent discoveries of SES-related genomic variations include asthma in children (Chen et al., 2006) and depression and other complex phenotypes (Bulick-Sullivan et al., 2015). Multiple polymorphisms are now being used to construct polygenic scores to predict risks for complex mental and physical diseases (Domingue and Belsky, 2017), as well as major killers such as cancer and (currently) Alzheimer’s.
Epigenetics has emerged as the most recent approach to studying the genetic bases of aging and disease and of gene-environment interactions that influence the rate of aging (Belsky et al., 2017). The principal focus of epigenetics is on how environmental conditions and factors, including stress exposure, affect methylation of DNA (and related processes that lead to the masking of DNA subsequences), thus influencing the transcription process in cellular replication. This process then affects phenotypic expression of genes, producing health disparities. Given that epigenetics involves the interaction of genes with environment, it presents a new approach to adjudicating the nature-nurture debate in favor of a gene-environment interaction paradigm.
Social genomics researchers are also exploring the controversial topic of the genetic bases of educational attainment itself, using GWAS methods. The first study of this kind claimed the identification of a range of alleles associated with educational attainment that fell on a continuum yielding a polygenic score (Rietveld et al., 2013). This approach has since been applied in other studies reporting findings that suggest that polygenic scores predict adult achievement beyond educational attainment (Belsky et al., 2016).
Behavioral Pathways
Social surveys have investigated the impact of diverse behaviors on health and mortality, but three sets of behavioral traits have emerged repeatedly as significantly correlated with SES: health behaviors, social connectedness, and cognitive and noncognitive behavioral traits. While social connectedness and cognitive and noncognitive traits may not be treated as “behavioral pathways” typically in the social sciences, we propose that they are observed patterns of behavior or performance that are repeatedly associated with SES and health outcomes.
Health Behaviors
The relationships between poor health behaviors—such as smoking, obesity, excess drug and alcohol consumption, and physical inactivity—and adult health and mortality risk have been widely studied, but with mixed results regarding the role of SES for each behavior. Reviews of studies of socioeconomic differences suggest that SES has uneven effects on different health behaviors—for example, generally large effects on smoking and physical inactivity and more moderate effects on obesity—and that different health behaviors influence health and mortality in ways that are difficult to isolate from other factors, measured or otherwise. The association between SES and alcohol use is complicated by the distinction between high alcohol use (at higher SES) and problem drinking (associated with lower SES) (see Pampel et al., 2010, for a general review).
Because these health behaviors appear to be most strongly associated with preventable or more socially graded diseases such as cardiovascular disease, educational attainment is the widely advocated explanation for these findings because it is a marker of better access to knowledge, more effective problem solving, and greater self-control, among other cognitive and noncognitive (or personality) traits (Mirowsky and Ross, 2005). Also, since these behaviors usually emerge early in the life course, often in adolescence when lifelong behavioral tendencies are established (Moffitt et al., 2011), they are considered cumulative stocks of health-damaging behaviors that are inextricably intertwined with day-to-day living.
Other factors that have been identified as contributors to damaging health behaviors include deprivation and stress, which motivate stress-relieving or comfort-seeking dependence on these behaviors (Eibner and Evans, 2005); peer relationships, usually consisting of individuals of similar SES backgrounds who encourage and reinforce these behaviors (Smith and Christakis, 2008); geographic locations (neighborhoods, communities, states, etc.) where the concentration of low SES and prevalence of different health behaviors varies for a wide range of factors related to lifestyles, social
networks, safety, drug availability, recreational opportunities, health care resources, and access to healthier foods (Boardman et al., 2005; Christakis and Fowler, 2007), and where variations in the income gradient are correlated with smoking behavior but not with other environmental conditions (Chetty et al., 2016); and cohort differences observable in changing habits of daily physical activity (in work or recreation), diet, and exposure to alcohol and drugs (Case and Deaton, 2017). Recent observations of significant geographic and cohort variations among non-Hispanic Whites living outside of urban areas in increased death rates from self-destructive health behaviors (suicide, drug overdose, alcoholism) have raised serious concerns about the sustained despair experienced in economically depressed regions (Case and Deaton, 2017). All of the above factors interact in complex ways that call for further research.
Social Connectedness
The extent to which individuals (a) are socially integrated in, or isolated from, their families and communities, (b) perceive positive or negative support from family members and communities, and (c) experience social strain in their relationships has been found to have diverse influences on health across the life course. Social relationships constitute the day-to-day social capital available to individuals to enable problem solving, facilitate access to resources, and reinforce social identities and cultural values. Among disadvantaged groups, social connectedness can compensate for fewer economic resources and buffer against poor health. However, connectedness to networks of disadvantage can also limit access to resources and inhibit efforts to access resources, as a result of cultural values and community norms. The distrust with which socially disadvantaged groups view health institutions is one way that health-seeking behavior is constrained and poor health is reproduced in these communities (Cook and Stepanokova, 2007). Thus, social connectedness can have countervailing effects on health (Smith and Christakis, 2008).
Few studies have examined these relational patterns and their outcomes across the life course. Fewer still have linked them to physiological processes that influence health and life expectancy. One recent study draws on four national surveys (Add Health, the National Health and Nutrition Examination Survey, the Midlife in the United States study, and the National Social Life, Health, and Aging Project) with biomarker data that measure precursors of inflammation and metabolic dysregulation at successive life course phases in different samples (Yang et al., 2016). The principal findings are that higher levels of social integration are associated with lower levels of physiological dysregulation in adolescence and later life. Lower levels of social integration are concomitantly associated with elevated risks
for dysregulation. In the older population, the effect of social isolation on hypertension exceeds that of clinical factors such as diabetes.
Social isolation is a growing risk for poor health and mortality in the general population (Hawkley and Cacioppo, 2010). Social isolation is often intertwined with poverty, social inequality, and life chances in rural and urban contexts across countries and across age groups (Wilkinson and Marmot, 2003). In the older population, the risk of social isolation grows as members experience the loss of spouses, siblings, and friends and as financial resources become depleted over time and limit social activities and interactions. The perception of isolation is accompanied by feelings of loneliness, which predict impaired mental health, including depression associated with physiological dysregulation and mortality (Luo et al., 2012; Lynch and George, 2002).
Cognitive and Noncognitive Traits
SES has been demonstrated to affect children’s brain development, measured intelligence, language skills, and executive function (Wolfe et al., 2012). The relationship between SES and cognitive development and abilities, through its associations with educational attainment and later adult achievement, is also well established. Small but significant gaps in early skills in basic mathematics, reading, and problem solving associated with SES grow into wider disparities as children and adolescents move through the educational system, which sequentially rewards earlier achievement and, with time, amplifies initial SES differences through sorting and tracking structures. These disparities are reinforced by SES differences in resources for nonschool learning opportunities in summer camps, music lessons, tutoring, and the like (Crosnoe and Benner, 2016). This early, institutionalized widening of inequality has motivated economists to calculate the costs of investing in early childhood education as opposed to later educational programs and remedial job training; they have found investments in disadvantaged children to be the most cost-effective (Heckman, 2006).
At the other end of the life course, cognitive abilities such as word recall and counting backwards have been associated with SES in childhood (Case and Paxson 2008), but the rate of cognitive decline with age and the onset of dementias have not. Research in this area has identified considerable heterogeneity and plasticity in the age trajectories of numerous cognitive abilities over the life course (Alwin and Hofer, 2011). The influence of SES across the life course on these patterns has yet to be fully investigated.
Noncognitive traits and “soft skills” (Heckman and Kautz, 2012) have been implicated in explaining educational attainment and SES across the life course. These factors include diverse measured traits such as conscientiousness, self-control, and delayed gratification, as identified by developmental
psychologists (Moffitt et al., 2011), and mastery, self-efficacy, personal control, and planful competence, as identified by sociologists (Hitlin and Kwon, 2016). Self-control and conscientiousness in childhood have been found to predict health and well-being in adulthood (Moffitt et al., 2011). The educational system also amplifies these noncognitive patterns of social behavior that endure into adulthood and predict damaging health behaviors that increase the risks for disease, influence patterns of achievement in the workplace, affect compliance with the health care system, and constrain patterns of well-being (Heckman et al., 2006).
NEW DIRECTIONS IN RESEARCH: APPROACHES AND CHALLENGES
The SES-health-mortality association will continue to preoccupy the demography of aging. The results of our analyses, and others available in this large literature, indicate continued relationships among these variables. Educational differentials in mortality are significant and continue over time. Those with the least schooling are experiencing increases in mortality risk. While we know that some objective indicators of health (e.g., cardiovascular disease) have improved over time, probably in part as a result of increases in educational attainment in the population generally, educational differentials in SRH persist. The precise mechanisms by which this is occurring remain puzzles.
This essay has pointed throughout to new directions for research in the demography of aging. Biological data, linkages to administrative data that add information on geographical context, mortality, health records, analytic strategies to deal with causal relationships, and a life course framework all have emerged in the last two decades to enhance the demography of aging. We can nevertheless highlight major future directions in research that include the following:
Educational and other social status differentials will continue to demand attention as representative longitudinal survey datasets mature and become more enriched with the introduction of detailed health (biological) measures and administrative records and as epidemiological and medical datasets incorporate more demographically and socially rich data to augment their clinical records. More cooperation between these major data collection approaches is overdue. In the case of samples for nationally representative longitudinal surveys of aging, the introduction of biological data often comes late in the lives of participants (and usually on voluntary subsamples of the panel), after underlying biological processes have been set in motion. Longitudinal datasets collected on younger samples offer one long-term solution to this challenge. For instance, the National Longitudinal Study of Adolescent to Adult Health includes biomarker data along
with rich, fine-grained demographic information on multiple life transitions. Dependence on voluntary subsamples for biomarker data is still a limitation of most surveys.
In the case of epidemiological and clinical data, the addition of demographically rich data is essential to assist in dealing with selectivity bias. For example, both the critical period and sensitive period hypotheses examined in these studies, which are often tested on limited samples, are difficult to assess empirically in the general population due to limited information on later-life outcomes in clinical studies. A promising approach is to link clinical data on these early periods to follow-up surveys and clinical data collections later in life to capture patterns over the life course. Similarly, these studies depend on selective approaches to solicit participation and subsequently on voluntary participation by subjects. Innovative data collection/analytic designs that seek to identify underlying selection processes for study participation are overdue.
The apparent tightening interdependence of SES and health over the life course will continue to present inferential challenges calling not only for statistical and experimental innovations but also for more interdisciplinary synthesis of research findings to establish “robust associations” that may not meet some criteria of causality but can still inform health and social policies. In this context, the SES construct itself will require some interrogation along at least two lines. First, as cohort differences in educational levels and in their consequences for occupational placement, earnings, and health outcomes shift, the explanation for education’s effect will be problematic and require multilevel strategies. Some clues lie in recent research cited in this essay that identifies credential effects rather than years of schooling effects. What are the period or structural factors bearing on the effect of education? Second, if educational stratification becomes even more differentiated to the extent that, for example, even heretofore advantaged college-educated populations become more finely stratified in health in later life by selectivity of degree and marketability of college major, how will the effects of education be interpreted along cognitive or social dimensions? Along these lines, age-period-cohort analyses are more feasible than ever, with nearly a century of poolable cross-sectional surveys and over a quarter-century of panel datasets and new analytic strategies to analytically decompose the effects of these major life course variables.
The creative concatenation of multiple panel datasets from different cohorts may be a useful strategy to compensate for single-cohort studies with limited generalizability for the longer life course. Single cohort panels have moved demography a good distance toward understanding the aging process, but datasets start at different ages (adolescence, young adulthood, later life), in spite of their respective longitudinal designs, and are thus limited in their generalizability. We have cited one recent effort to compensate
for this with the concatenation of multiple cohort-based datasets (Yang et al., 2016). This approach faces several challenges, including the linkage of different sampling designs that follow different individuals over successive phases of the life course and the harmonization of measures, among others. Yet, the successful integration of multiple datasets may move life course theory forward.
Reported in the most recent studies in the demography of aging summarized earlier are clear regional variations (both across states and rural-urban) in the SES-health-mortality relationship that have provoked new questions. Indeed, across locales in the United States, life expectancy at birth varies by more than 10 years, and health disparities are equally large. Thus, the geography of inequality is probably as important as the biology of inequality. Much remains to be investigated to understand the source of these disparities, but part of the explanation is surely to be attributable to both SES disparities and to differences in how SES influences health across regions as a result of economic restructuring, migration, and technological transformations that have accompanied globalization.
The association of SES with health and mortality is a complex, multivariate, interactive, and multilevel puzzle for the demography of aging. This review only touches upon the nuances of this complexity. It will require genuine interdisciplinary research going forward, research in which boundaries are crossed between different styles of research and conceptual formulations.
REFERENCES
Adler, N.E., and Stewart, J. (Eds.). (2010). The Biology of Disadvantage: Socioeconomic Status and Health. New York: Wiley-Blackwell.
Aiello, A.E., Feinstein, L., Dowd, J.B., Pawelec, G., Derhovanessian, E., Galea, S., Uddin, M., Wildman, D.E., and Simanek, A.M. (2016). Income and markers of immunological cellular aging. Psychosomatic Medicine, 78, 657–666.
Allison, P.D. (1984). Event History Analysis: Regression for Longitudinal Event Data (Sage University Paper Series: Quantitative Applications in the Social Sciences, No. 07-46). Beverly Hills, CA: Sage.
Alwin, D.F., and Hofer, S.M. (2011). Health and cognition in aging research. Journals of Gerontology: Psychological and Social Sciences, 66B(S1), 9–15.
Barker, D.J.P. (1995). The fetal origins of coronary heart disease. British Medical Journal, 311, 171–174.
Belsky, D.W., Moffitt, T.E., Corcoran, D.L., Domingue, B., Harrington, H., Hogan, S., Houts, R., et al. (2016). The genetics of success: How single nucleotide polymorphisms associated with educational attainment relate to life-course development. Psychological Science, 27(7), 957–972.
Belsky, D.W., Caspi, A., Cohen, H.V., Kraus, W.E., Ramrakha, S., Poulton. R., and Moffitt, T.E. (2017). Impact of early personal-history characteristics on the pace of aging: Implications for clinical trials of therapies to slow aging and extend healthspan. Aging Cell, 16, 644–651.
Ben-Shlomo, Y., and Kuh, D. (2002). A life course approach to chronic disease epidemiology: Conceptual models, empirical challenges, and interdisciplinary perspectives. International Journal of Epidemiology, 31, 285–293.
Boardman, J.D., Saint Onge, J.M., Rogers, R.G., and Denney, J.T. (2005). Race differentials in obesity: the impact of place. Journal of Health and Social Behavior, 46(3), 229–243.
Bulick-Sullivan, B., Finucane, H.K., Anttila, V., Gusev, A., Day, F.R., Loh, P.R., et al. (2015). Nature Genetics, 47(11), 1236–1241.
Case, A., and Deaton A. (2017). Mortality and Morbidity in the 21st Century (Brookings Papers on Economic Activity (BPEA) Conference Drafts, March 23-24). Washington, DC: Brookings Institution.
Case, A., and Paxson, C. (2008). Height, health and cognitive function at older ages. American Economic Review: Papers and Proceedings, 98(2), 463–467.
Case, A., Lubotsky, D., and Paxson, C. (2002). Socioeconomic status and health in childhood: The origins of the gradient. American Economic Review, 92, 1308–1344.
Chen, E., Hanson, M.D., Paterson, L.Q., Griffin, M.J., Walker, H.A., and Miller, G.E. (2006). Socioeconomic status and inflammatory processes in childhood asthma: The role of psychological stress. Journal of Allergy and Clinical Immunology, 117(5), 1014–1020.
Chetty, R., Stepner, M., Abraham, S., Lin, S., Scuderi, B., Turner, N., Bergeron, A., and Cutler, D. (2016). The association between income and life expectancy in the United States, 2001–2014. Journal of the American Medical Association, 315, 1750–1766.
Christakis, N.A., and Fowler, J.H. (2007). The spread of obesity in a large social network over 32 years. New England Journal of Medicine, 357, 370–379.
Cook, K.S., and Stepanikova, J. (2007). The health care outcome of trust: A review of empirical evidence. In J. Brownlie, A. Greene, and A. Howson (Eds.), Researching Trust and Health (pp. 194–214). New York: Routledge.
Crimmins, E.M. (2013). HRS Documentation Report: Documentation of Biomarkers in the 2006 and 2008 Health and Retirement Study. Available: http://hrsonline.isr.umich.edu/sitedocs/userg/Biomarker2006and2008.pdf [March 2018].
Crimmins, E.M., and Finch, C.E. (2006). Infection, inflammation, height and longevity. Proceedings of the National Academy of Sciences of the United States of America, 103(2), 498–503.
Crimmins, E.M., Johnson, M.L., Hayward, M., and Seeman, T. (2006). Age differences in allostatic load: An index of frailty. In Z. Yi, E.M. Crimmins, Y. Carriere, and J.-M. Robine (Eds.), Longer Life and Healthy Aging (pp. 111–140). New York: Springer.
Crimmins, E.M., Kim, J.K., and Seeman, T.E. (2009). Poverty and biological risk: The “earlier” aging of the poor. Journals of Gerontology: Biological and Medical Sciences, 64A(92), 286–292.
Crosnoe, R., and Benner, A.D. (2016). Educational pathways. In M.J. Shanahan, J.T. Mortimer and M.K. Johnson (Eds.), Handbook of the Life Course: Volume II (pp. 179–200). New York: Springer.
Dannefer, D. (1987). Aging as intracohort differentiation: Accentuation, the Matthew effect, and the life course. Sociological Forum, 2, 211–236.
DiPrete, T.D., and Eirich, G.M. (2006). Cumulative advantage as a mechanism for inequality: A review of theoretical and empirical developments. Annual Review of Sociology, 32(1), 271–297.
Domingue, B.W., and Belsky, D.W. (2017). The social genome: Current findings and implications for the study of human genetics. PLoS Genetics, 13(3), e1006615.
Dupre, M.E. (2007). Educational differences in age-related patterns of disease: Reconsidering the cumulative advantage and age-as-leveler hypotheses. Journal of Health and Social Behavior, 48(1), 1–15.
Eibner, C., and Evans, W.N. (2005). Relative deprivations, poor health habits, and mortality. Journal of Human Resources, 40(3), 591–620.
Elo, I.T., and Preston, S.H. (1992). Effects of early life conditions on adult mortality: A review. Population Index, 57, 186–212.
Evans, G.W. (2004). The environment of childhood poverty. American Psychologist, 59(2), 77–92.
Ferraro, K.F., and Morton, P.M. (2016). What do we mean by accumulation? Advancing conceptual precision for a core idea in gerontology. Journals of Gerontology: Psychological and Social Sciences, 73B(2), 269–278.
Ferraro, K.F., and Shippee, T.P. (2009). Aging and cumulative inequality: How does inequality get under the skin? The Gerontologist, 49, 333–343.
Freedman, D.M., Ballard-Barbash, R., Doody, M.M., and Linet, M.S. (2006). Body mass index and all-cause mortality in a nationwide U.S. cohort. International Journal of Obesity, 30, 822–829.
GBD Collaboration. (2014). Global, regional and national prevalence of overweight and obesity in children and adults, 1980-2013. Lancet, 384(9945), 766–781.
Gruenewald, T.L. (2013). Opportunities and challenges in the study of biosocial dynamics in healthy aging. In National Research Council, New Directions in the Sociology of Aging (pp. 217–237). Washington, DC: The National Academies Press. doi: https://doi.org/10.17226/18508.
Haas, S.A. (2007). The long-term effects of poor childhood health: An assessment and application of retrospective reports. Demography, 44, 113–135.
Hamil-Luker, J., and O’Rand, A.M. (2007). Gender differences in the link between childhood socioeconomic conditions and heart attack risk in adulthood. Demography, 44(1), 137–158.
Hanson, J., and Hackman, D.A. (2012). Cognitive neuroscience and disparities in socioeconomic status. In B. Wolfe, W. Evans, and T.E. Seeman (Eds.), The Biological Consequences of Socioeconomic Inequalities (pp. 158–186). New York: Russell Sage.
Hardy, J., and Selkoe, D.J. (2002). The amyloid hypothesis of Alzheimer’s disease: Progress and problems on the road to therapeutics. Science, 297, 353–356.
Hawkley, L.C., and Cacioppo, J.T. (2010). Loneliness matters: A theoretical and empirical review of consequences and mechanisms. Annals of Behavioral Medicine, 40(2), 218–227.
Hayward, M.D., and Gorman, B.K. (2004). The long arm of childhood: The influence of early-life social conditions on men’s mortality. Demography, 41(1), 87–107.
Hayward, M.D., Hummer, R.A., and Sasson, I. (2015). Trends and group differences in the association between educational attainment and U.S. adult mortality: Implications for understanding education’s causal influence. Social Science & Medicine, 127, 8–18.
Heckman, J.J. (2006). Skill formation and the economics of investing in disadvantaged children. Science, 312, 1990–1902.
Heckman, J.J., and Krautz, T.D. (2012). Hard evidence for soft skills. Labor Economics, 19, 451–464.
Heckman, J.J., Stixrud, J., and Urzua, S. (2006). The effects of cognitive and noncognitive abilities on labor market outcomes and social behavior. Journal of Labor Economics, 24(3), 411–482.
Herd, P., Goesling, B., and House, J.S. (2007). Socioeconomic position and health: The differentials effects of education versus income on the onset versus the progression of health problems. Journal of Health and Social Behavior, 48(3), 223–238.
Hertzman, C., and Boyce, T. (2010). How experience gets under the skin to create gradients in developmental health. Annual Review of Public Health, 31, 329–247.
Hitlin, S., and Kwon, H.W. (2016). Agency across the life course. In M.J. Shanahan, J.T. Mortimer, and M.K. Johnson (Eds.), Handbook of the Life Course (pp. 431–449). New York: Springer.
House, J.S., Lepkowski, J.M., Kinney, A.M., Mero, R.P., Kessler, R.C., and Herzog, A.R. (1994). The social stratification of aging and health. Journal of Health and Social Behavior, 35(3), 213–234.
Imbens, G.W., and Rubin, D.B. (2015). Causal Inference for Statistics, Social, and Biomedical Sciences: An Introduction. New York: Cambridge University Press.
Juster, R.P., McEwen, B.S., and Lupien, S.J. (2010). Allostatic load biomarkers of chronic stress and impact on health and cognition. Neuroscience and Biobehavioral Reviews, 35, 2–16.
Kitagawa, E.M., and Hauser, P.M. (1973). Differential Mortality in the United States: A Study in Socioeconomic Epidemiology. Cambridge, MA: Harvard University Press.
Levine, M.E., and Crimmins, E.M. (2014). Evidence of accelerated aging among African Americans and its implications for mortality. Social Science and Medicine, 118, 27–32.
Link, B.G., and Phelan, J. (1995). Social conditions as fundamental causes of disease. Journal of Health and Social Behavior, 35, Extra Issue, 80–94.
Lleras-Muney, A. (2005). The relation between education and adult mortality in the United States. The Review of Economic Studies, 72(1), 189–221.
Luo, Y., Hawkley, L.C., Waite, L.J., and Cacioppo, J.T. (2012). Loneliness, health, and mortality in old age: A national study. Social Science and Medicine, 74(6), 907–914.
Lynch, S.M. (2003). Cohort and life course patterns in the relationship between education and health: A hierarchical approach. Demography, 40(2), 309–331.
Lynch, S.M. (2006). Explaining life course and cohort variation in the relationship between education and health: The role of income. Journal of Health and Social Behavior, 47, 324–338.
Lynch, S.M., and George, L.K. (2002). Interlocking trajectories of loss-related events and depressive symptoms among elders. Journals of Gerontology: Social Sciences, 57B, S117–S125.
Mather, K.A., Jorm, A.F., Parslow, R.A., and Christensen, H. (2011). Is telomere length a biomarker of aging? A review. Journals of Gerontology: Biomedical Sciences, 66A2, 202–213.
McEwen, C.A., and McEwen, B.S. (2017). Social structure, adversity, toxic stress, and intergenerational poverty: An early childhood model. Annual Review of Sociology, 43, 445–472.
Minnesota Population Center and State Health Access Data Assistance Center. (2015). Integrated Health Interview Series: Version 6. Minneapolis: University of Minnesota.
Mirowsky, J., and Ross, C.E. (2003). Education, Social Status, and Health. New York: Aldine de Gruyter.
Mirowsky, J., and Ross, C.E. (2005). Education, learned effectiveness and health. London Review of Education, 3, 205–220.
Moffitt, T.E., Arseneault, L., Belsky, D.W., Dickson, N., Hancox, R.J., and Harrington, H. (2011). A gradient of childhood self-control predicts health, wealth, and public safety. Proceedings of the National Academy of Sciences of the United States of America, 108(7), 2693–2698. https://doi.org/10.1073/pnas.1010076108.
Montez, J.K., Zajacova, A., and Hayward, M.K. (2017). Contextualizing the social determinants of health: Disparities in disability by educational attainment across U.S. states. American Journal of Public Health, 107(7), 1101–1108.
National Center for Health Statistics. (2014). Survey Description, National Health Interview Survey. Hyattsville, MD: National Center for Health Statistics.
National Center for Health Statistics. (2015). Office of Analysis and Epidemiology, Public-use Linked Mortality File. Hyattsville, MD: National Center for Health Statistics.
Pampel, F.C., Krueger, P.M., and Denney, J.T. (2010). Socioeconomic disparities and health behaviors. Annual Review of Sociology, 36, 349–370.
Preston, S.H., and Taubman, P. (1994). Socioeconomic differences in adult mortality and health status. In National Research Council, Demography of Aging (pp. 279–318). Washington, DC: National Academy Press.
Reither, E.M., Hauser, R.M., and Yang, Y.C. (2009). Do cohorts matter? Age-period-cohort analyses of the obesity epidemic in the U.S. Social Science and Medicine, 69(10), 1439–1448.
Rietveld, C.A., Medland, S.E., Derringer, J., Yang, J., Esko, T., Martin, N.W., Westra, H.-J., et al. (2013). GWAS of 126,599 individuals identifies genetic variants associated with educational attainment. Science, 340, 1467–1471.
Schafer, M.H., Ferraro, K.F., and Mustillo, S.A. (2011). Children of misfortune: Early adversity and cumulative inequality in perceived life trajectories. American Journal of Sociology, 116, 1053–1091.
Shanahan, M.J., and Hofer, S.M. (2011). Molecular genetics, aging and the life course: Sensitive periods, accumulation, and pathways models. In R.H. Binstock and L.K. George (Eds.), Handbook of Aging and the Social Sciences. New York: Elsevier
Smith, K.P., and Christakis, N.S. (2008). Social networks and health. Annual Review of Sociology, 34, 405–429.
Vaisey, S., and Miles, A. (2017). What you can—and can’t—do with three-wave panel data. Sociological Methods & Research, 46(1), 44–67.
Vuolo, M., Ferraro, K.F., and Morton, P.M. (2014). Why do older people change their ratings of childhood health? Demography, 51(6), 1999–2023.
Wheaton, B., Young, M., Montazer, S., and Stuart-Lahman, K. (2013). Social stress in the twenty-first century. In C.S. Aneshensel, J.C. Phelan, and A. Bierman (Eds.), Handbook of the Sociology of Mental Health (pp. 299–323). New York: Springer.
Wilkinson, R.G., and Marmot, M. (Eds.). (2003). Social Determinants of Health: The Solid Facts. Copenhagen: World Health Organization.
Willson, A.E., Shuey, K.M., and Elder, G.H. (2007). Cumulative advantage processes as mechanisms of inequality in life course health. American Journal of Sociology, 112(6), 1886–1924.
Wilson, R.S., Li, Y., Bienas, J.L., and Bennett, D.A. (2006). Cognitive decline in old age: Separating retest effects from the effects of growing older. Psychology and Aging, 21(4), 774–789.
Wolfe, B., Evans, W., and Seeman, T.E. (Eds.). (2012). The Biological Consequences of Socioeconomic Inequalities. New York: Russell Sage.
Yang, Y., Boen C., Gerken, K., Li, T., Schorpp, K., and Harris, K.M. (2016). Social relationships and physiological determinants of longevity across the human life span. Proceedings of the National Academy of Sciences of the United States of America, 113(3), 578–583.
Yashin, A., Stallard, E., and Land, K.C. (Eds.). (2016). The Biodemography of Aging (Springer Series on Demographic Methods and Population Analysis). New York: Springer.
This page intentionally left blank.