7
Phylogeographic Model Selection Leads to Insight into the Evolutionary History of Four-Eyed Frogs

MARIA TEREZA C. THOMÉ* AND BRYAN C. CARSTENS†‡
Phylogeographic research investigates biodiversity at the interface between populations and species, in a temporal and geographic context. Phylogeography has benefited from analytical approaches that allow empiricists to estimate parameters of interest from the genetic data (e.g., θ = 4Neµ, population divergence, gene flow), and the widespread availability of genomic data allow such parameters to be estimated with greater precision. However, the actual inferences made by phylogeographers remain dependent on qualitative interpretations derived from these parameters’ values and as such may be subject to overinterpretation and confirmation bias. Here we argue in favor of using an objective approach to phylogeographic inference that proceeds by calculating the probability of multiple demographic models given the data and the subsequent ranking of these models using information theory. We illustrate this approach by investigating the diversification of two sister species of four-eyed frogs of northeastern Brazil using single nucleotide polymorphisms obtained via restriction-associated digest sequencing. We estimate the composite likelihood of the observed data given nine demographic models and then rank these models using the Akaike information criterion. We demonstrate that estimating parameters under a model that is a poor fit to the data is likely to produce values that lead to spurious
__________________
* Departamento de Zoologia, Instituto de Biociências, Universidade Estadual Paulista, Campus Rio Claro, 13506900 Rio Claro, SP, Brazil; and †Department of Evolution, Ecology and Organismal Biology, The Ohio State University, Columbus, OH 43210. ‡To whom correspondence should be addressed. Email: carstens.12@osu.edu.
phylogeographic inferences. Our results strongly imply that identifying which parameters to estimate from a given system is a key step in the process of phylogeographic inference and is at least as important as being able to generate precise estimates of these parameters. They also illustrate that the incorporation of model uncertainty should be a component of phylogeographic hypothesis tests.
In biological populations with interbreeding individuals, allele frequencies will inevitably change with time, in both stochastic and systematic manners, through neutral and adaptive processes. These processes—genetic drift, gene flow, mutation, recombination, and natural selection—constitute observable phenomena that lead directly to population structure, population divergence, and eventually speciation. Phylogeography is ideally situated to investigate systems where the microevolutionary processes that act within gene pools begin to form macroevolutionary patterns and has been described as the bridge between population genetics and phylogenetics (Avise et al., 1987). The power of the discipline comes from the consideration of geographic origin of individuals and populations along the continuum between populations and species (Knowles, 2004; Hickerson et al., 2010).
Phylogeographic research has progressed through several stages since Avise et al. (1987) introduced the term. Initial studies were based on information that can be gathered from the genetic data under few assumptions, for example by calculating summary statistics or estimating gene trees. Inferences were then derived from qualitative interpretations about what that information implied about the evolutionary history of the system (e.g., Demesure et al., 1996; Bernatchez and Wilson, 1998). This approach has been criticized as being prone to overinterpretation, because researchers are inclined to propose more detailed and complex historical scenarios than are actually supported by the data (Knowles and Maddison, 2002). The general response to such criticisms has been the widespread adoption of model-based methods to analyze phylogeographic data, particularly models that incorporate coalescent theory (Kingman, 1982) to estimate parameters of interest under a formal framework. Model-based methods of phylogeographic inference clearly represent an advance to the field, but making inferences from these parameter estimates still forces researchers to make subjective decisions. Despite the potential complexity of the demographic models, the actual process of phylogeographic inference remains largely analogous to that of earlier investigations: The relative influence of evolutionary processes is derived from the magnitude of numeric values estimated for parameters that measure what the researchers believe to be important evolutionary processes. For example, subjective decisions regarding estimated rates of gene flow are commonly
used to determine whether populations are reproductively isolated from their sister taxa (e.g., Dolman and Moritz, 2006) or conspecifics (e.g., Runemark et al., 2012).
Once efficient algorithms and computational power became available, researchers applied model-based methods to phylogeographic research with little hesitation (but see Templeton, 2010), with models implemented in software packages being particularly popular. For example, the paper describing a popular method that estimates temporal divergence with gene flow has been cited in more than 500 studies to date (Hey, 2010). Simulation-based techniques are also commonly applied to empirical systems, either to test competing hypotheses such as introgression and lineage sorting (e.g., Reid et al., 2012; Debiasse et al., 2014; Grummer et al., 2015) or to test phylogeographic hypotheses against a null model (e.g., Knowles, 2001; DeChaine and Martin, 2005; Smith et al., 2011). Such methods have been widely adopted by the phylogeographic community because model-based methods offer a path toward estimating putatively relevant parameters, and because the models themselves can be tailored to the particulars of a given system (e.g., Knowles, 2009b; Beaumont MA et al., 2010). Phylogeographic inferences are more transparent when based on parameters estimated under these models, and arguably less subjective. However, simply using a complex demographic model to analyze genetic data is not a guarantee that phylogeographic inferences will be correct.
In the cognitive sciences, researchers have long been mindful of confirmation bias, the tendency to interpret novel information in a manner consistent with preconceived ideas (Nickerson, 1998). People tend to seek out information that supports their preexisting beliefs and are unlikely to consider contradictory information. Particularly problematic is the primacy effect, in which the information that is learned first effectively has more emphasis than information that is obtained at a later date (Nickerson, 1998). Confirmation bias is likely prevalent in phylogeographic research (Carstens et al., 2013), influencing phylogeographic inference by shaping the very questions that are asked by researchers. For example, if initial investigations into a given system used gene trees and phylogenetic thinking, researchers may not consider population processes such as gene flow as being potentially important, and choose to estimate divergence times under a species tree model, which may not actually fit the data (e.g., Reid et al., 2014). Researchers working in temperate systems in the Northern Hemisphere may assume that postglacial expansion is an important process and choose to estimate effective population size under growth models (e.g., Kuhner, 2006), whereas those working on focal taxa that inhabit island systems are likely to consider dispersal to be a key process shaping allele frequencies, and estimate effective population sizes under migration models (e.g., Beerli and Felsenstein, 2001). Such assumptions
will guide choices about which models and software should be used to analyze the data and might also bias their interpretation of the values of parameters estimated under these models. Objective assessment of model fit should be an important component of phylogeographic research, particularly in systems where there is little preexisting information about the demographic history.
WHAT IF THE PHYLOGEOGRAPHIC MODEL IS WRONG?
There is a great asymmetry in terms of the amount of available background information between model and nonmodel systems. In the extreme case of Homo sapiens, the analytical models used for data analysis are informed by the academic output of entire disciplines (e.g., anthropology) as well as thousands of previous genetic investigations. In contrast, the average phylogeographer likely knows very little about the focal organism before an investigation, save what can be inferred from its taxonomy and general habitat. This asymmetry is exacerbated for researchers interested in tropical diversity, which account for the vast majority of organisms: Chances are that even the most basic natural history traits (area of occurrence, density, feeding habitats, maturation age, and reproductive mode) are unknown to science. Given this paucity of information, how should researchers determine which models to use in data analysis?
In their review of statistical methods in phylogeography, Nielsen and Beaumont (2009) argue strongly that population parameters should be estimated under appropriate models to avoid bias in the parameter estimates: “A clear limitation of any model-based method is that the model might be wrong. In fact, the real complexity of the demography of natural populations is unlikely to be captured by any simple model we could propose. In some cases, this may not affect inferences much, but in other cases it will.” If phylogeographic inferences are largely derived from parameter estimates made under complex models, then such inferences are implicitly conditioned on the statistical fit of the model used to estimate these parameters to the empirical data collected from the focal system. To date, there has been too little attention devoted to methods for assessing the statistical fit of phylogeographic models to the data.
STATISTICAL FRAMEWORKS FOR PHYLOGEOGRAPHY
Phylogeographic research is a historical discipline rather than an experimental one, and evolutionary history cannot be replicated. Because the experimental controls used in classical hypothesis testing are not available (e.g., Neyman and Pearson, 1933), testing hypotheses, even with parametric simulation (e.g., Knowles, 2001; Carstens et al., 2004), forces
phylogeography to conform to a statistical framework that may not be suited to historical research (Cleland, 2001). A more promising strategy for phylogeographic data analysis is to proceed by identifying which of many possible models of historical demography offer the best statistical fit to the observed data, rather than testing null hypotheses, where rejection only tells us that the model representing the hypothesis is a poor fit to the data. If the goal of phylogeography is to infer the evolutionary history of the focal taxon, then ranking a set of models that represent alternative evolutionary scenarios provides a rigorous tool for inference because it will help researchers to avoid confirmation bias. Because the parameters in each model correspond to various evolutionary processes, the relative influence of particular evolutionary processes to the empirical system can be assessed by considering the set of parameters included in the model that offers the best fit to the data. Model selection is a useful framework for phylogeographic inference because it offers an approach that accounts for the uncertainty in the models used to analyze the data.
MODEL SELECTION IN BAYESIAN AND INFORMATION THEORETIC FRAMEWORKS
Fagundes et al. (2007) provided a compelling example of phylogeographic research using model selection in a Bayesian framework, using approximate Bayesian computation (ABC) to evaluate alternative models of human demographic history. Inspired by this work, many researchers have applied a similar approach to a wide range of nonmodel systems (e.g., Tsai and Carstens, 2013; Espindola et al., 2014; Jamamillo-Correa et al., 2015; Peres et al., 2015; Vera-Escalona et al., 2015). However, as with any approach to data analysis, phylogeographic model choice using ABC has limitations, and decisions about which models to include in the comparison set can be challenging. Because ABC loses power to differentiate among models as the number of models in the comparison set increases (Pelletier and Carstens, 2014), one cannot easily evaluate large numbers of models. Fagundes et al. (2007) had the advantage of working in a model system where they could identify three types of models to test based on the results of hundreds of previous investigations, but the lack of similar information in nonmodel systems increases the odds of erroneous model choice and faulty phylogeographic inference.
A solution to evaluating a large number of models representing many possible demographic histories is to use information theory (Burnham and Anderson, 1998) to rank models. Information theory relies on the estimation of the Kullback–Leibler (Kullback and Leibler, 1951) information of a given model using the Akaike information criterion (AIC) (Akaike, 1973), and the subsequent ranking of all models in the comparison set. The model
ranking is achieved by calculating the difference between the AIC score of a particular model and the best model in the set (e.g., ∆i = AICi – minAIC), and subsequent transformation to model likelihoods (wi) by normalizing AIC differences across the set of R models such that they sum to 1.0 see Burnham and Anderson, 1998]. A reasonable interpretation of these model probabilities is that they correspond to posterior probabilities under a uniform prior distribution (Burnham and Anderson, 1998). Information theory is commonly used to select models of DNA nucleotide substitution for analyses of sequence data (as in the software ModelTest; Posada and Crandall, 1998), and has been effectively used to compare among a large number of models in this context. To date, information theoretic approaches have been used in phylogeography to choose the best of several isolation-with-migration models (e.g., Koopman and Carstens, 2010; Rittmeyer and Austin, 2015), to evaluate models of postglacial expansion and colonization (Carstens et al., 2013), and to evaluate models of source-sink migration (Beerli and Palczewski, 2010; Barrow et al., 2015). In this chapter, we briefly illustrate its application using data from the four-eyed frogs of northeastern Brazil.
CASE STUDY: THE PLEURODEMA SYSTEM IN THE BRAZILIAN CAATINGA
Pleurodema alium and Pleurodema diplolister are sister species of four-eyed frogs that inhabit the Caatinga in northeastern Brazil (Faivovich et al., 2012). The Caatinga is a widespread xeric biome, surrounded by the extensive mesic environments of the Amazon, Cerrado, and Atlantic Rainforest. Its climate is highly seasonal and unpredictable, with severe droughts and rainless years. As is typical of amphibians from xeric habitats, Pleurodema persist throughout most of the year by burrowing underground, becoming active only after seasonal heavy rains create ephemeral pools for breeding. Even though the life cycle in Pleurodema depends on precipitation, these frogs cannot maintain populations in more mesic biomes and its distribution is restricted to the Caatinga xeric habitat.
Floristically, the Caatinga is one of the isolated nuclei in the Seasonally Dry Tropical Forests (SDTFs) of South America. The history of the SDTFs is debated, with some evidence suggesting that they were formerly continuous and recently fragmented [during the Last Glacial Maximum (LGM); Prado and Gibbs, 1993], and other evidence favoring an older (Tertiary) fragmentation (Pennington et al., 2000). Environmental niche modeling results in contrasting maps ranging from a largely continuous to a fragmented Caatinga, depending on the approach used (Werneck et al., 2011; Collevatti et al., 2013). Regardless of the broader continental trends
of the SDTFs, there is abundant geologic evidence that the Caatinga has been recurrently invaded (and at least partially replaced) by mesic forest throughout its history (de Oliveira et al., 1999; Auler et al., 2004).
P. alium and P. diplolister were recently the subject of phylogeographic investigation. Thomé et al. (2016) collected >350 samples, sequenced the mitochondrial cytochrome oxidase I (COI) gene, and genotyped 12 microsatellite loci. Using these data, they were able to confirm that the species were distinct at the genetic level (both at COI and microsatellite markers), and that they have partly sympatric distributions: P. alium is restricted to the southern Caatinga, whereas P. diplolister is widespread in the biome, occurring also in pockets of Caatinga embedded within the Cerrado (Fig. 7.1). The population genetic structure within the broadly distributed P. diplolister reflected the distribution of its sister species, in that the P. diplolister samples that were sympatric with P. alium formed a separate genetic cluster.
Given the available information, a wide range of evolutionary processes (and therefore parameters) could be incorporated into a demographic model of P. alium and P. diplolister. Temporal divergence likely represents an important component, supported by the deep divergence in the COI data (Thomé et al., 2016). Effective population sizes are likely to differ between species, because P. diplolister has a much larger geographic range than P. alium, and probably a corresponding difference in census population size. Although range size and effective population size are not necessarily correlated, the difference in geographic range provides justification for allowing for the possibility of differences in effective population size among species, so long as we assume that the mutation rate does not vary between species. In addition to the processes of temporal divergence and different population sizes, other evolutionary processes could be important: population size change within species (such as population bottlenecks or exponential population growth), gene flow, and/or natural selection.
We specified nine demographic models for analysis, which were designed to represent a range of demographic histories. All models included lineage divergence between the sister taxa P. alium and P. diploister and some combination of the following demographic processes: population expansion or contraction, population bottlenecks, gene flow, and population-specific θ values (Fig. 7.2). There are hundreds of ways that the divergence of two species from a common ancestor could be parameterized (see Pelletier and Carstens, 2014); here, we hope to specify models that span the range of possible models but include those that we believe to be plausible (e.g., we do not include n-island models that lack temporal divergence, because we consider divergence time to be an essential parameter to include in any model that contains sister species).

Sampling and Molecular Protocols
We sampled 183 individuals of Pleurodema from 55 locations in the core, isolates, or peripheral regions of the Caatinga, comprising most of its distribution in the Caatinga biome (see Thomé et al., 2016). SNPs were collected via genomewide sampling using restriction enzymes (double-digest RADseq; Peterson et al., 2012). DNA digestion and barcode ligation were performed individually for each sample using 300 ng of freshly extracted DNA, the restriction enzymes Sbf1-HF and MspI, the ligation enzyme Ligase T4, and eight different barcoded Illumina adaptors. The digestion–ligation reactions were then pooled in groups of eight and purified with Agencourt AMPure beads, and PCR (12 cycles) was used to amplify the fragments containing barcodes using six different Illumina
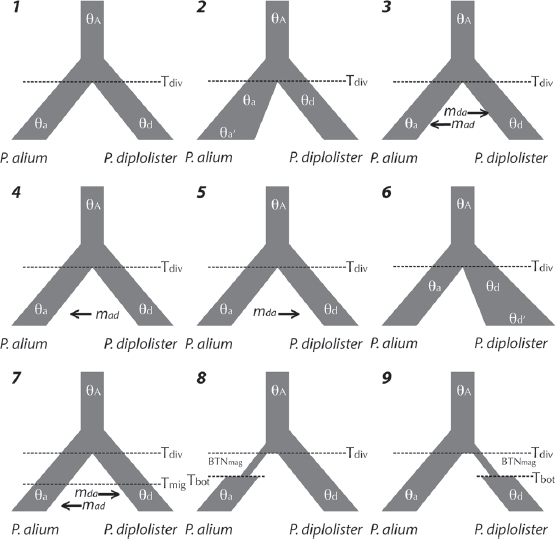
indexed primers and Phusion DNA polymerase. PCR products were quantified with Qubit Fluorometric Quantitation (Invitrogen), equimolar quantities of six groups containing eight samples each were pooled, and 250-bp to 500-bp fragments were selected using a Blue Pippin Prep. The fragment sizes were confirmed with an Agilent 2100 Bioanalyzer (Agilent), and 100-bp, single-end, sequencing reactions were conducted using an Illumina HiSEq 2000 at Beckman Coulter Genomics.
Data Processing
Illumina outputs from Pleurodema samples were processed using the pyRAD pipeline (Eaton, 2014). Except for the initial demultiplexing step, which was conducted separately on each library, we processed data for all samples together with the following parameter specifications: 10× minimal coverage, four or fewer unknown bases per sequence, minimum
similarity of 0.90, a maximum ratio of shared polymorphisms of 20%, and a minimum coverage taxon of 70%. The number of reads that passed quality control was plotted against the number of loci obtained in each sample to establish a minimum number of reads for a sample to be considered. Because the number of loci stabilizes above 300,000 reads, we eliminated the 18 samples that were below this threshold before conducting a final SNP calling step in the remaining 165 samples. This scheme yielded 6,027 alignments containing SNPs.
Missing Data
After excluding SNPs that were possibly under selection (Supporting Information1), our dataset consisted of 5,810 sequenced regions containing one or more SNPs. However, every region was not sequenced in each sample. Population-level data collected using RADseq and related protocols typically consist of data matrices with some degree of missing data (e.g., Rubin et al., 2012; Wagner et al., 2013), and these missing data can lead to biased estimates of effective population size and other parameters (Arnold et al., 2013; Gautier et al., 2013). Missing data are likely to be particularly problematic for analytical methods that rely on estimates of allele frequencies because rare alleles may be undercounted. However, it is not clear how to best conduct analyses in a manner that accounts for the missing data. Missing data might be related to mutations in the recognition site of the enzymes, and removing all individuals that contain missing data about a certain threshold would be equal to removing the most divergent individuals, which could artificially homogenize the dataset and dramatically change the estimates of the number of rare alleles. Alternatively, removing all loci that contain missing data will dramatically reduce the size of any observed RADseq dataset and negate some of the advantages of collecting such data in the first place. Because we analyze our data using a method that relies on estimates of the population site frequency spectra (discussed below), it is important to account for missing data in a manner that does not bias our estimate of these frequencies. To accomplish this, we choose SNPs (one per locus) and individuals at random from our full data and then replicated this downsampling 10 times using a Python script provided by Jordan D. Satler, The Ohio State University, Columbus, OH (Supporting Information). After the downsampling procedure, our replicate data matrixes consisted of approximately one-third of the total SNPs in one-half of the individuals and enabled us to calculate confidence intervals by comparing estimated parameters across replicates.
__________________
1 Supporting information for this chapter, which includes Table S1, is available online at www.pnas.org/lookup/suppl/doi:10.1073/pnas.1601064113/-/DCSupplemental.
Model Selection
We estimated the composite likelihood of the probability of the observed data given the specified model using fastsimcoal2 (FSC2) (Excoffier et al., 2013). FSC2 estimates parameters specified by the user (including θ = 4Neµ, population size change, gene flow, and population divergence) from the site frequency spectrum (SFS). Demographic processes will influence the site frequency distributions; for example, gene flow will produce an abundance of shared SNPs, population bottlenecks will result in a reduction of genetic diversity and thus fewer low-frequency SNPs, and so on. After the demographic model is specified, FSC2 selects initial parameter values at random from a range specified by the user and simulates data using the demographic model and parameter values. Composite likelihoods are calculated following Nielsen (2000), who demonstrated that there is a relationship between the branch lengths of the genealogy and the probability of observing a SNP of a certain frequency distribution. Parameter optimization was conducted using the Brent algorithm implemented in FSC2, which identifies parameter values that maximize the likelihood estimate of the observed SFS given the demographic model. Finally, the maximized likelihood observed across all iterations is used in model comparison.
Using FSC2, the analysis of each of the 10 downsampled datasets was replicated 50 times (Excoffier et al., 2013). The individual run settings of each replicate included 100,000 simulations for the calculation of the composite likelihood and 50 cycles of the Brent algorithm (for parameter optimization). FSC2 analyses were conducted using massively parallel computing resources provided by the Ohio Supercomputer Center. After the maximum likelihood was estimated for each model in every replicate, we calculated the AIC scores and converted to model probabilities as above. This transformation allows us to measure the probability of each model given the observed data across replicates (e.g., Table S1), which we interpret as a measure of the degree of support for a particular model following Anderson (2008).
RESULTS AND DISCUSSION
The results of the FSC2 analysis were consistent in the sense that only three models, all isolation with migration, have any appreciable model probability (i.e., >0.001; Table S1). The model with ongoing gene flow from P. diplolister to P. alium has the highest model probability. The secondary contact model and the model asymmetric gene flow between P. diplolister and P. alium have similar log-likelihoods given the data to the best model but lower AIC scores due to having additional parameters. Additionally, parameter estimates suggest that these models may be more similar than
they seem (Table 7.1). For example, in the secondary contact model (i.e., model 7) parameter estimates of the time that gene flow begins are closer to the divergence of these species from their common ancestor than to the present, and in model 3 (i.e., the model with asymmetric gene flow) the rate of gene flow from P. alium to P. diplolister is estimated to be much lower than the rate of migration in the opposite direction (although these estimates are not perfectly comparable because the duration of gene flow is not the same under these models). Because of the similarity in parameters estimated by these models, our phylogeographic inferences are based on model-averaged parameter values (i.e., the value of a given parameter estimated under a particular model weighted by the model probability of that model, averaged across models that share the particular parameter; Table 7.1).
There are several striking features of the divergence with gene flow models. Assuming a mutation rate of 2.1 × 10–9 substitutions per site per generation (Gottscho et al., 2014) to convert parameter estimates, the ancestral effective population size (averaged across replicates and models) was estimated to be small (~12,500 individuals). P. alium and P. diplolister began to diverge from their common ancestor during the last glacial cycle of the Pleistocene (~58,900 y BP) but continued to exchange alleles via migration. The rate of migration into each species from the other was not equal; roughly 10 times as many P. diplolister migrants entered the P. alium gene pool than the reverse (2 Nmda = 0.78; 2 Nmad = 0.07). Finally, whereas the current effective population size of each species is estimated to be larger than the ancestral population, current effective population sizes in P. diplolister are substantially larger than in P. alium (Nd = 1.34 × 106; Na = 6.9 × 104), consistent with differences in their geographic ranges.
Perhaps the most surprising result from our analysis is how much parameter estimates depend on the model used to estimate the parameters. For example, divergence time is estimated to be two orders of magnitude more ancient when estimated under model 6 (~3,280,000 y BP) than under the best-ranked model (Table 7.1), whereas the ancestral effective population size was estimated to be much smaller (2.65 × 102). Given the lack of previous estimates for these parameters in this system, there would be little reason to be suspicious of these values absent an assessment of model fit. This example illustrates the importance of performing phylogeographic model selection before any attempt to make inferences about the evolutionary history of a system, especially those based on parameter estimates.
TABLE 7.1 Comparison of Parameter Estimated Using FSC2 Under Four Models
Model (wi) | Nancestral | Nalium | Ndiplolister | Tdiv | 2Nm12 | 2Nm21 | Nfound | Tevent | Gexp |
---|---|---|---|---|---|---|---|---|---|
3 (0.21) | 1.48 × 104 | 6.86 × 104 | 1.34 × 106 | 5.86 × 104 | 0.069 | 0.904 | — | — | — |
4 (0.56) | 1.43 × 104 | 6.98 × 10v | 1.33 × 106 | 5.88 × 104 | 0.072 | — | — | — | — |
7 (0.23) | 5.59 × 103 | 6.92 × 104 | 1.36 × 106 | 5.93 × 104 | 0.078 | 0.738 | — | 2.97 × 104 | — |
6 (0.00) | 2.65 × 102 | 8.20 × 104 | 2.3 × 106 | 3.28 × 106 | — | — | 73 | 1.09× 104 | –4.6 × 10–5 |
Model average | 1.25 × 104 | 6.94 × 104 | 1.34 × 106 | 5.89 × 104 | 0.073 | 0.783 | — | — | — |
Lower confidence interval | 1.12 × 104 | 6.61 × 104 | 1.31 × 106 | 5.75 × 104 | 0.063 | 0.643 | — | — | — |
Upper confidence interval | 1.37 × 104 | 7.26 × 104 | 1.37 × 106 | 6.02 × 104 | 0.083 | 0.887 | — | — | — |
NOTES: Shown for all models are estimates of population sizes (Nancestral, Nalium, Ndiplolister), population divergence (Tdiv), and gene flow (2Nm). Additional parameters estimated under model 6 are the size of the founding population (Nfound), the time that expansion begins (Tevent), and the magnitude of population size change (Gexp). The additional parameter estimated under model 7 is the time that gene flow begins (Tevent). The model probability of each model is shown in parentheses after the model number. Also shown for six parameters are the model averaged estimate values and the upper and lower 90% confidence interval. All parameters were converted to real units assuming a mutation rate of 2.1 × 10–9. See Table S1 for additional information regarding the results from all models.
Phylogeographic Inferences
There are several advantages to basing phylogeographic inferences on the results of model selection exercises. Such analyses allow researchers to identify which evolutionary processes have shaped genetic diversity. In Pleurodema, the divergence of the sister taxa P. alium and P. diplolister is occurring despite ongoing gene flow. This inference stems directly from results of the model selection exercise: All of the models that have good AIC scores and thus receive any appreciable support include some gene flow between these species. This inference is not based on the magnitude of the parameter estimates, but solely on the inclusion of the gene flow parameters in the highest-ranked models. In addition, the results of the model selection analysis prevent us from overinterpreting our data (sensu Knowles and Maddison, 2002). In Pleurodema, previously collected evidence suggested that population expansion could represent an important feature of this system (Thomé et al., 2016), but none of the population size change or bottleneck models offered a good fit to the empirical data. As much as we expected expansion to be a dominant force shaping these data, there is no evidence for the influence of this process in the SNP dataset. We attribute this discrepancy to one of two causes. It could be that there is an actual difference in the signal between the SNP data analyzed here and the microsatellite and COI data analyzed by Thomé et al. (2016). Each of these markers evolves at a different rate and thus will be informative at different timescales. Thus, it is possible that faster markers perform better in detecting demographic expansions as recent as 4,240 y BP (de Oliveira et al., 1999). However, because these analyses differed in the number of individuals included (approximately three times as many in the microsatellite analysis as here), as well as in details of each analysis, this difference could result from some combination of these differences.
What factors may have caused the initial divergence of P. alium and P. diplolister? Results from analyses of environmental (climatic) niche modeling provide two important clues. First, the environmental niche of P. alium does not differ from that of P. diplolister (see Box 7.1). This makes it unlikely that these species are undergoing adaptive diversification, a result that is supported by an outlier loci analysis (e.g., a BayeScan analysis detects only 14 out of 6,027 loci as being potentially under selection; Supporting Information). Second, species distribution modeling supports the hypothesis of a dynamic distribution for the Caatinga, as the predicted distribution of these species has changed over the last 130,000 years, including being notably smaller at the mid-Holocene, and somewhat reduced at the LGM (Fig. 7.3). These historical distributions are at odds with previous paleomodeling of the SDTFs but consistent with the palynological record, which indicates that the present-day distribution of the Caatinga established very recently in the late Holocene (4,240 y BP; de Oliveira et
al., 1999). The dynamic range of these species supports the idea that these lineages have been periodically fragmented, possibly isolated, with secondary contact inhibiting the formation of reproductive isolation.
New Data, Better Methods, and Improved Inferences from Nonmodel Organisms
One of the pressing issues facing the discipline of phylogeography in the past was the limited amount of genetic data that could be collected from most systems, and the poor quality of parameter estimates that
resulted from analysis of these data (Edwards and Beerli, 2000; Brumfield et al., 2003; Felsenstein, 2006). In the last decade, advances in sequencing technology have led to dramatic improvements in the amount of data that can be collected from nonmodel systems (McCormack et al., 2013; Garrick et al., 2015). Given modest levels of funding, researchers can now collect more data from any system than are likely required to accurately estimate parameters of interest (e.g., Felsenstein, 2006; Carling and Brumfield, 2007). With next-generation datasets, phylogeography is well positioned to address a more important question: Which parameters are important to estimate in a given system? Whereas many of the methods applied by phylogeographic investigations were developed initially for the analysis of data from model systems (e.g., Excoffier et al., 2013), scientists working in nonmodel systems have been forced to confront the question of model fit, and in response they are developing creative solutions to identifying models that fit a particular system.
Some approaches to model selection are built into the framework of existing analytical methods. For example, IMa (Hey and Nielsen, 2007), which implements a divergence with gene flow model, can be used to conduct model selection using either likelihood ratio tests (e.g., Hey and Nielsen, 2007) or information theoretic approaches (Carstens et al., 2009). Similarly Migrate-n (Beerli and Palczewski, 2010), which implements an n-island model, can be used to select among many migration models (Beerli and Palczewski, 2010; Barrow et al., 2015). In addition, there are a number of approaches to species delimitation that incorporate model selection. These include methods that identify the optimal species delimitation using likelihood ratio tests (Knowles and Carstens, 2007), reversible-jump Markov chain Monte Carlo (Yang and Rannala, 2010; Solís-Lemus et al., 2015), information theory (Ence and Carstens, 2011), ABC (Camargo et al., 2012), and marginalized likelihoods (Leaché et al., 2014). Methods for analyzing comparative phylogeographic data are also under active development, including the use of hierarchical Bayesian models to test simultaneous divergence (Hickerson et al., 2007; Oaks et al., 2013) or simultaneous population expansion (Chan et al., 2014; Xue and Hickerson, 2015).
Although methods that implement model selection are extremely useful, they lack the flexibility of simulation-based approaches, which provide researchers with the capacity to customize their models to the particular details of nearly any empirical systems. ABC continues to be a useful approach to model selection, particularly when implemented in computational environments such as R (e.g., Csilléry et al., 2010) that can be easily used by researchers. Other methods are available that calculate the probability of SNP data. In addition to FSC2, used here, model selection can be conducted using diffusion approximation in the software dadi (Gutenkunst et al., 2009).
CONCLUSIONS
Testing the statistical fit of our models given the data enabled us to address a major limitation of model-based phylogeography (Beaumont MA et al., 2010). By deriving our phylogeographic inferences from parameters estimated under suitable models, we avoided confirmation bias and overinterpretation. Parameter estimation was of central importance to our phylogeographic inference process, but only after we made an objective determination about which parameters to estimate. Perhaps the greatest advantage of this approach to phylogeography is that while the inferences themselves do not rely solely on parameter estimates, the parameters that are estimated via model averaging are likely to be more representative of the actual population values. It is incumbent on researchers who do not conduct model selection as part of their phylogeographic investigations to ask whether their phylogeographic inferences are based on a model of historical demography that is appropriate for their empirical system.
ACKNOWLEDGMENTS
We thank Célio F. B. Haddad, Miguel T. Rodrigues, José Pombal, Jr., and Marcelo Nápoli for donation of samples; ICMBio for the collecting permit (30512); and Francisco Brusquetti for help in the field. We also thank members of the B.C.C. laboratory and two reviewers for comments that improved this manuscript prior to publication. Financial support was provided by Fundação Grupo Boticário de Proteção à Natureza Grant 0909_20112 and São Paulo Research Foundation Grants 2012/50255-2, 2011/51392-0, and 2013/09088-8. Computational resources were provided by the Ohio Supercomputer Center. Files used in the analysis have been deposited at DRYAD (doi:10.5061/dryad.8m6j3).